Modern Artificial Intelligence
ECE special seminar series
addressing the most important new research in the world of artificial intelligence (AI)
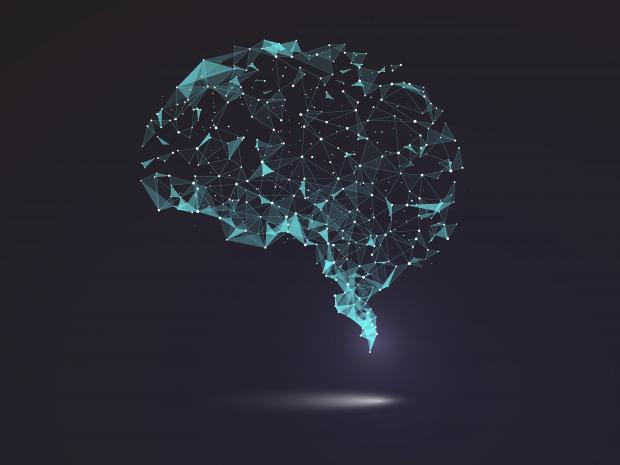
About
The Seminar Series in Modern Artificial Intelligence is hosted by the Department of Electrical and Computer Engineering at NYU Tandon. Organized by Professor Anna Choromanska, the series aims to bring together faculty and students to discuss the most important research trends in the world of AI. The speakers include world-renowned experts whose research is making an immense impact on the development of new machine learning techniques and technologies and helping to build a better, smarter, more-connected world.
View the AI Seminar Series Playlist on YouTube
Spring 2025

Mark Schmidt
University of British Columbia
"Why does Adam work so well for LLMs? And can we find optimal per-variable step sizes?"
February 18, 2025
Fall 2024
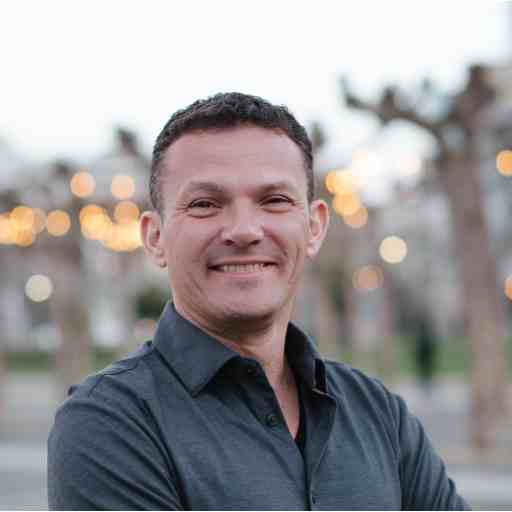
Jose Alvarez
NVIDIA
"Towards Robust and Reliable Autonomous Vehicles with Foundation Models"
October 8, 2024
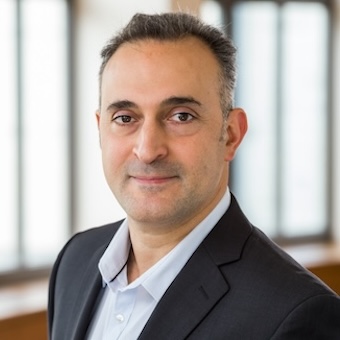
Elad Hazan
Princeton University
"Spectral Transformers"
November 26, 2024
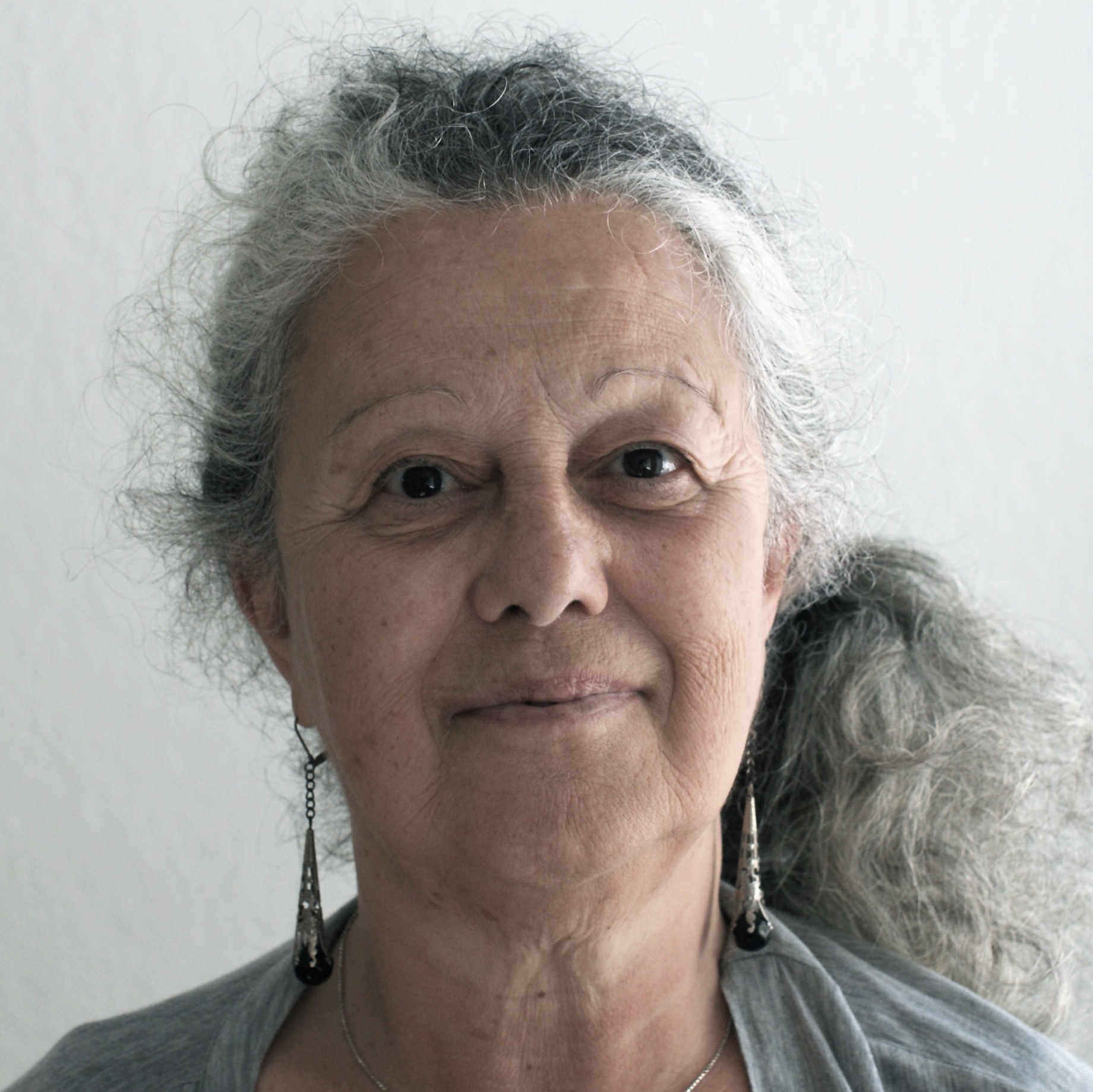
Sara A. Solla
Northwestern University
"Statistical physics, neural networks, and neuroscience: from then to now"
December 3, 2024
Spring 2024
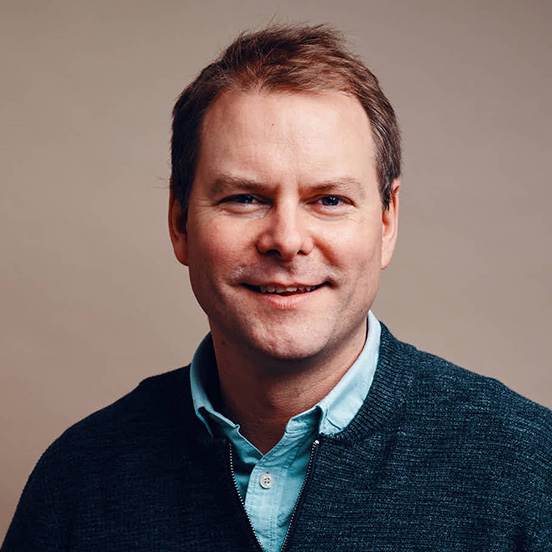
Jamie Shotton
Wayve Technologies
"Frontiers in Embodied AI for Autonomous Driving"
February 27, 2024
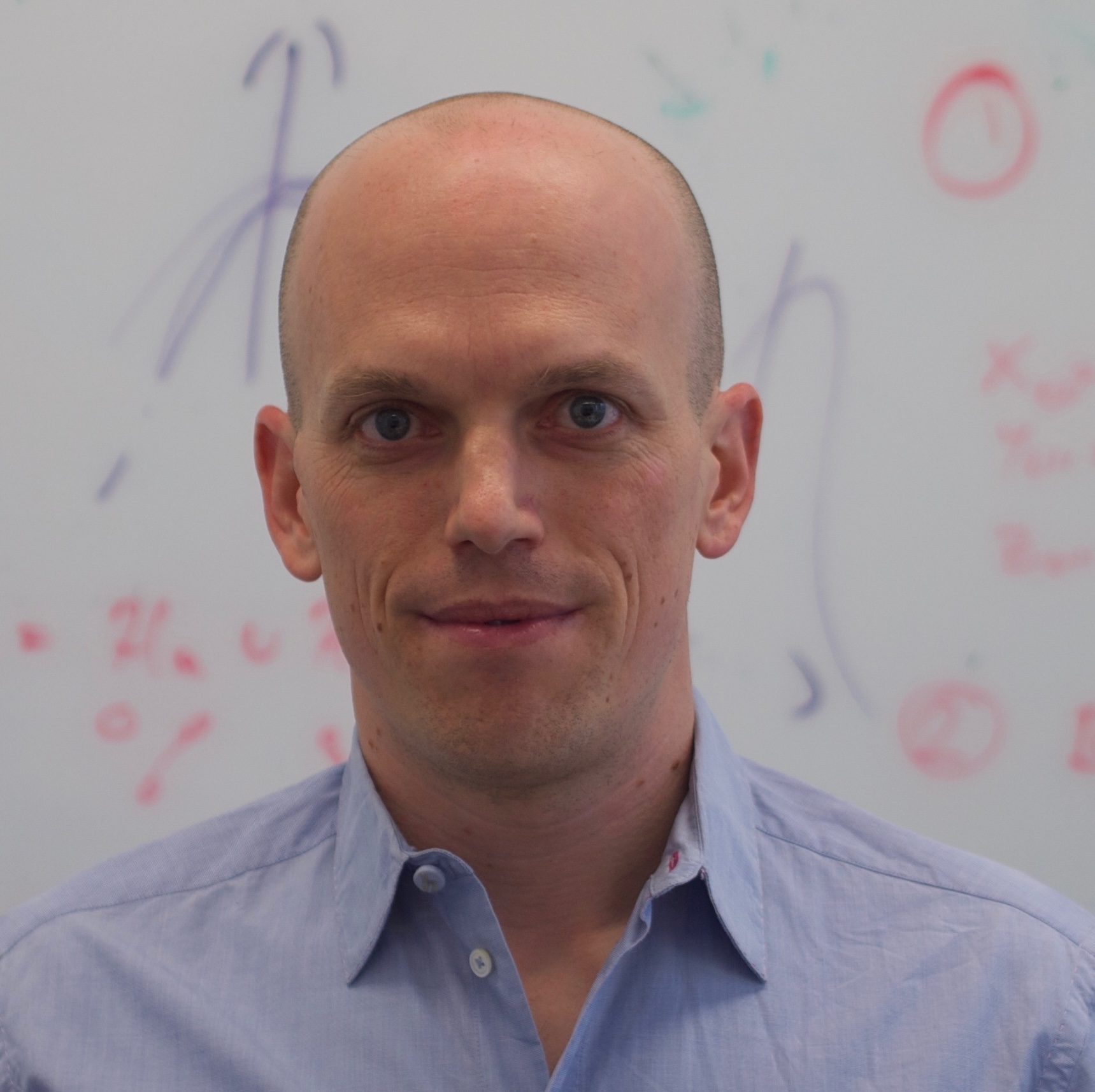
Arthur Gretton
University of College London
"Learning to act in noisy contexts using deep proxy learning"
March 11, 2024
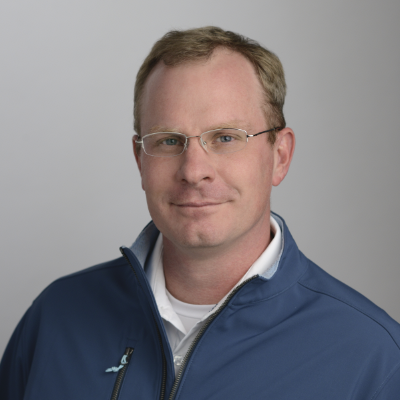
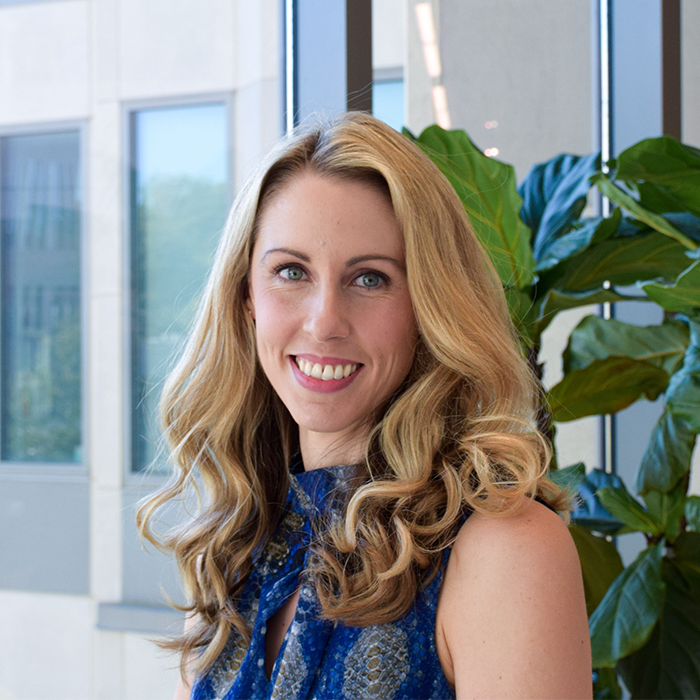
Dr. Samantha Wood
Indiana University Bloomington
"Reverse-Engineering the “Recipe” for Object Perception"
April 30, 2024
Fall 2023
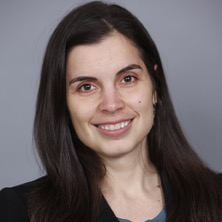
Maria Florina Balcan
Carnegie Mellon University
"Machine Learning for Algorithm Design"
September 26, 2023
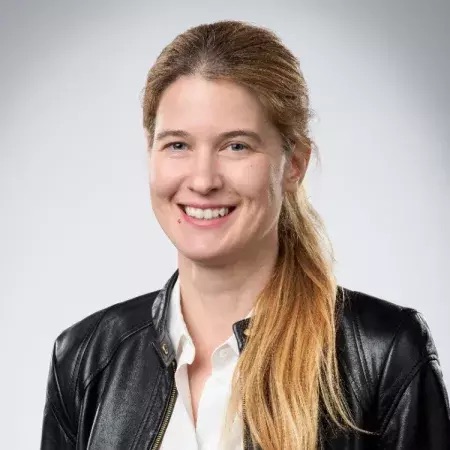
Sanja Fidler
University of Toronto
"Generative AI for 3D Content"
October 24, 2023
Spring 2023
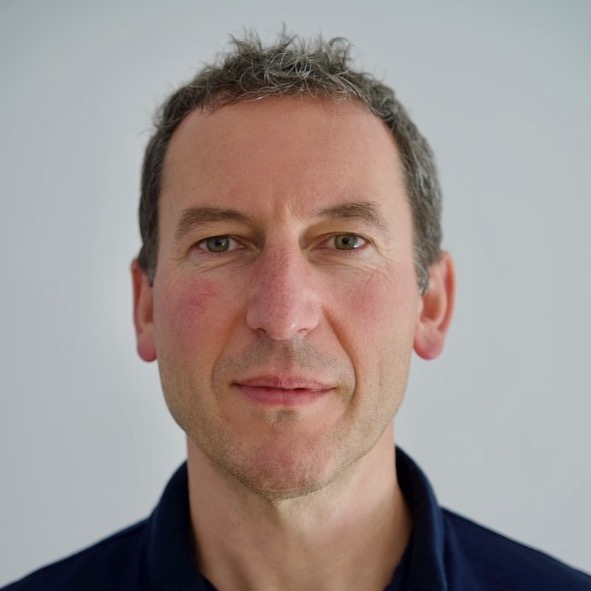
Alex Smola
Amazon Web Services
"AutoGluon: Empowering (Multimodal) AutoML for the Next 10 Million Users"
February 8, 2023
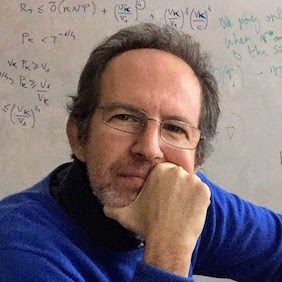
Nicolò Cesa-Bianchi
University of Milan and Polytechnic University of Milan, Italy
"Online learning, bandits, and digital markets"
March 28, 2023
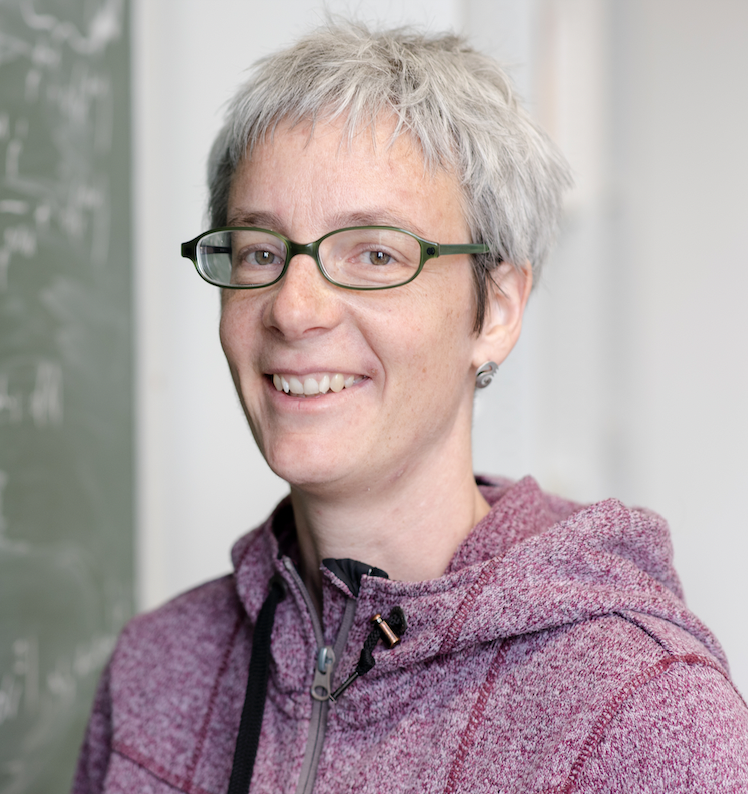
Ulrike von Luxburg
University of Tuebingen, Germany
"Explainability and regulation"
May 9, 2023
Fall 2022
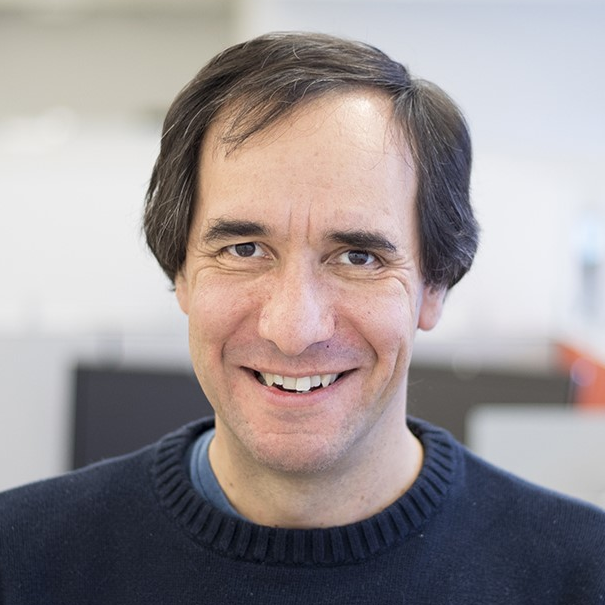
Robert Schapire
Microsoft Research
"Convex Analysis at Infinity: An Introduction to Astral Space"
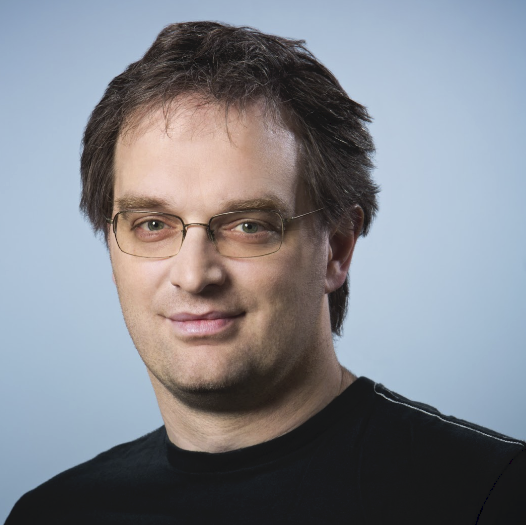
John Langford
Microsoft
"Discovering an agent's controllable latent state"
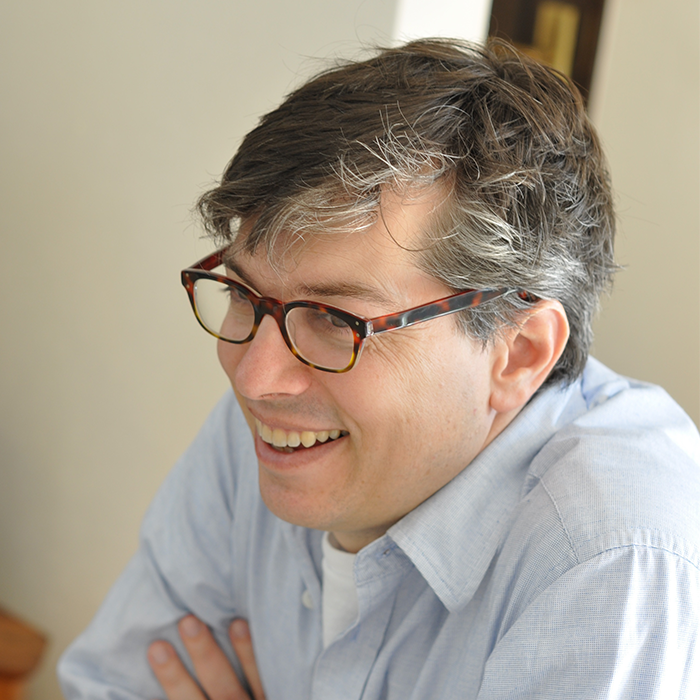
Chris Wiggins
Columbia University, New York Times
"Data Science at The New York Times"
Fall 2021
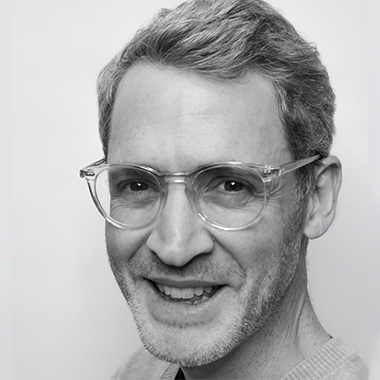
Michael Friedlander
University of British Columbia
"Geometric Duality in Optimization"
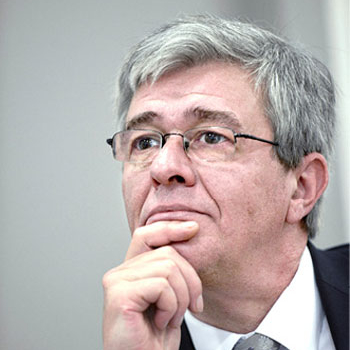
Karl J. Friston
Queen Square Institute of Neurology, University College London
"Deep Inference"
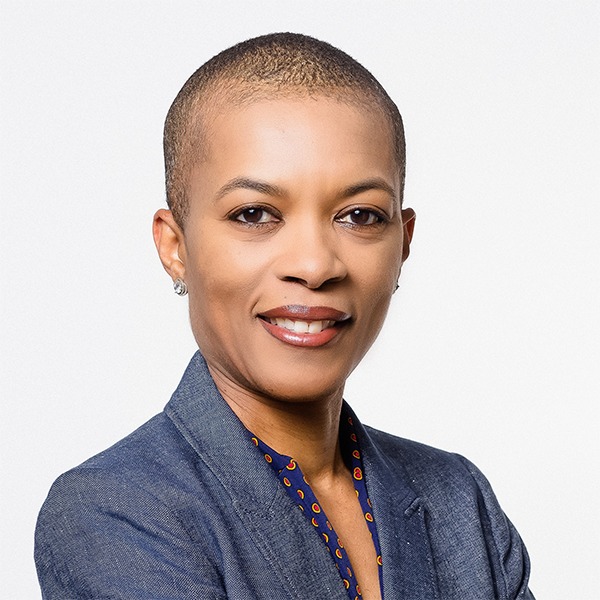
Dr. Fay Cobb Payton
North Carolina State University
"Coding, Coded & Counting: A Bias Continuum"
Spring 2021
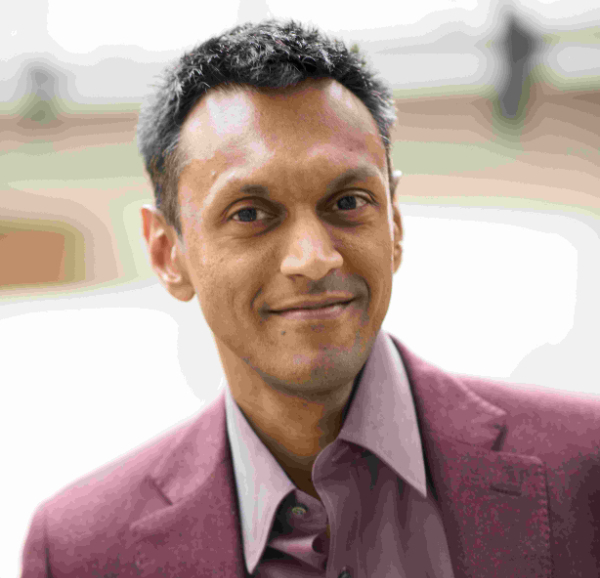
Sham Kakade
University of Washington
"Towards a Theory of Generalization in Reinforcement Learning"
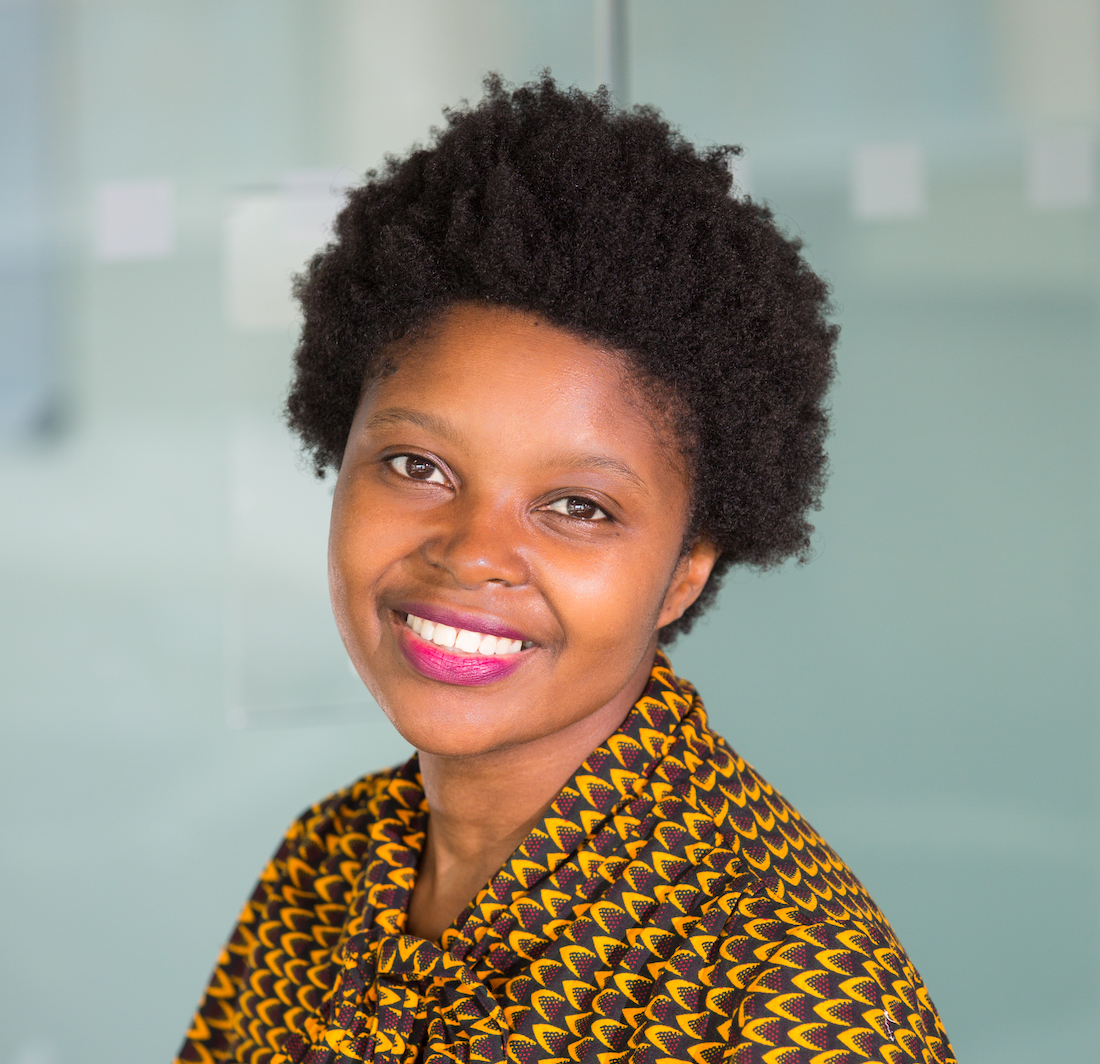
Mutale Nkonde
Founding Director of AI for the People
"Elections, Online Chatter and Content Moderation"
Joelle Pineau
Facebook and McGill University
"Building Reproducible, Reusable, and Robust Deep Reinforcement Learning Systems"
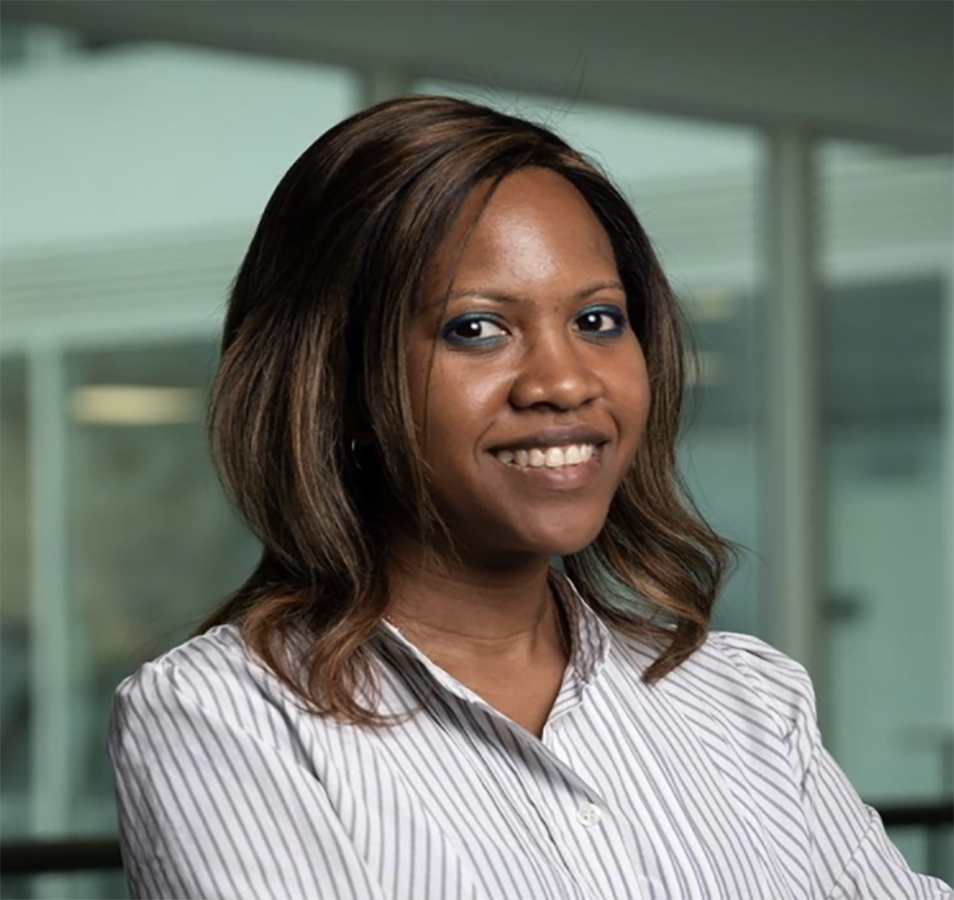
Danielle Belgrave and Niranjani Prasad
Microsoft Research Cambridge
"Machine Learning for Personalised Healthcare: Opportunities, Challenges and Insights"
Spring 2020
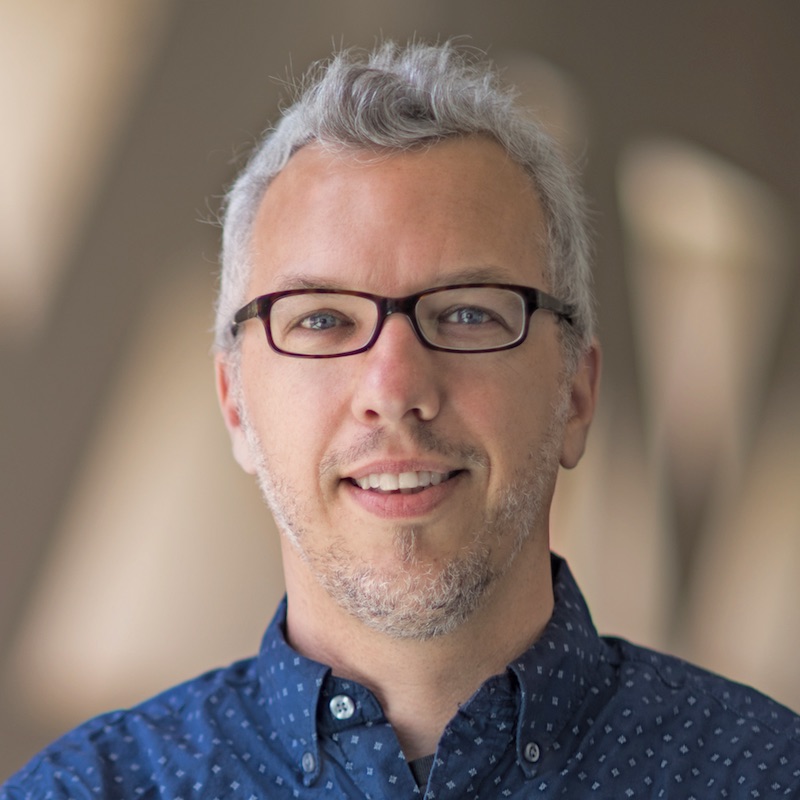
Jan Kautz, VP of Learning and Perception Research at NVIDIA
"Generative Models for Image Synthesis"
February 13, 2020
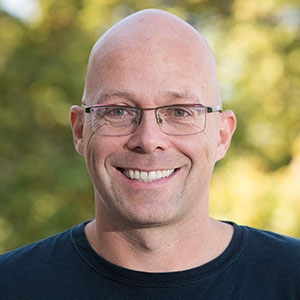
Gabor Lugosi, Pompeu Fabra University
"Archeology of Random Trees"
Thursday, March 5, 2020
Fall 2019
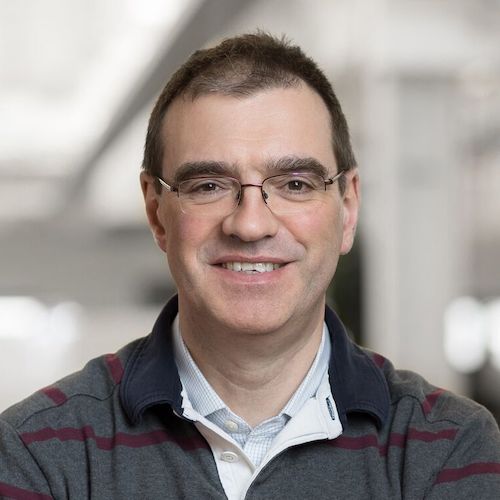
Leon Bottou, Facebook AI Research
"Learning Representations Using Causal Invariance"
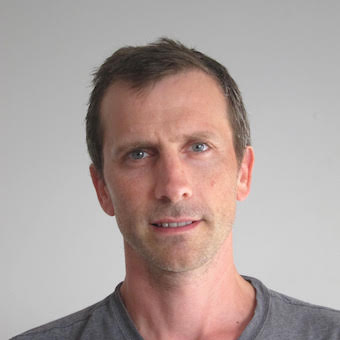
Francis Bach, INRIA, Paris France
"Distributed Machine Learning over Networks"
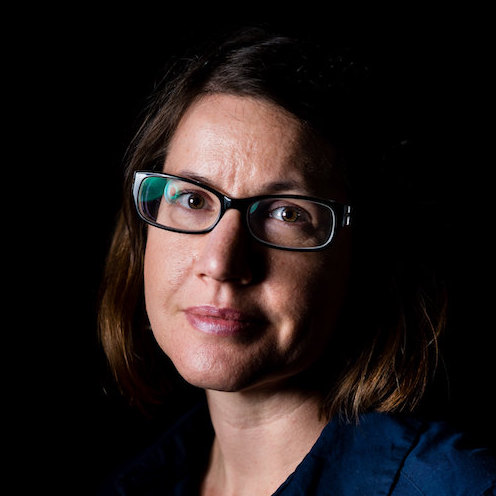
Raia Hadsell, Head of Robotics Research at DeepMind
"Challenges for Deep Reinforcement Learning in Complex Environments"
Spring 2019
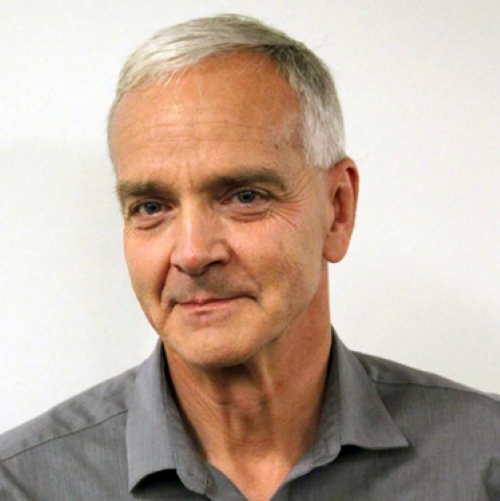
Martial Hebert, Carnegie Mellon University
"Research challenges in using computer vision in robotics systems"
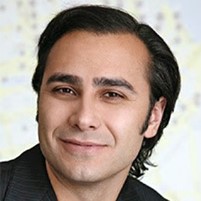
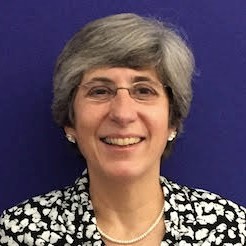
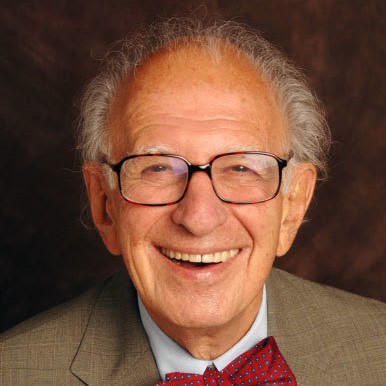
Fall 2018
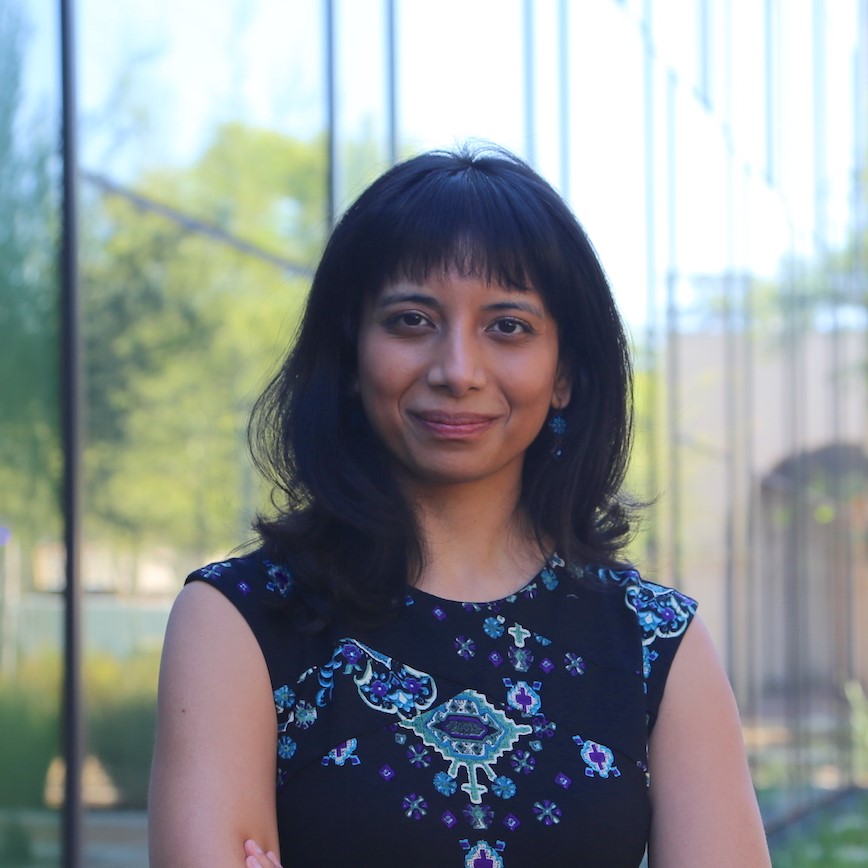
Anima Anandkumar, Caltech
"The AI Trinity: Data + Algorithms + Infrastructure"
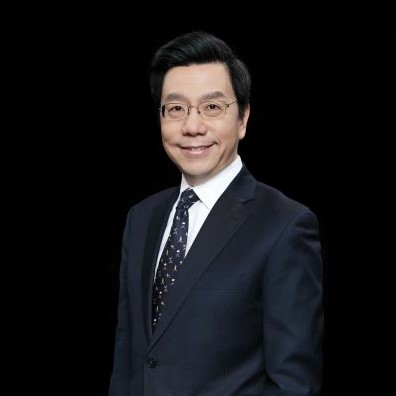
Kai-Fu Lee, Sinovation Ventures
"The Era of Artificial Intelligence"
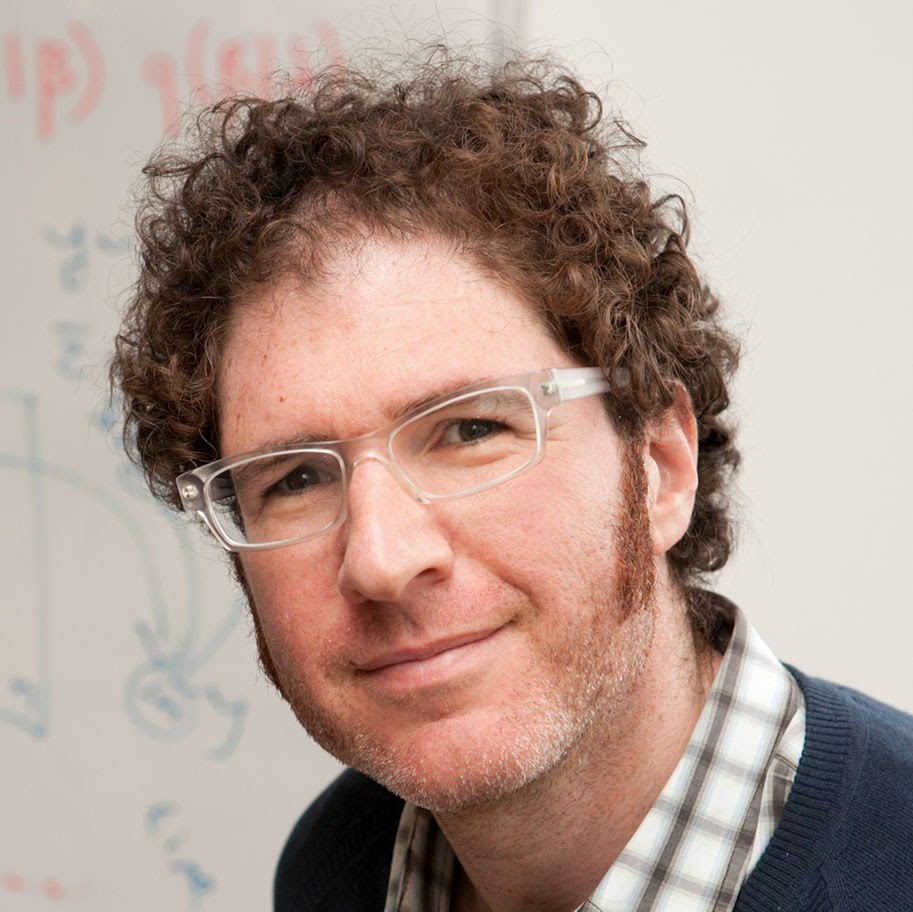
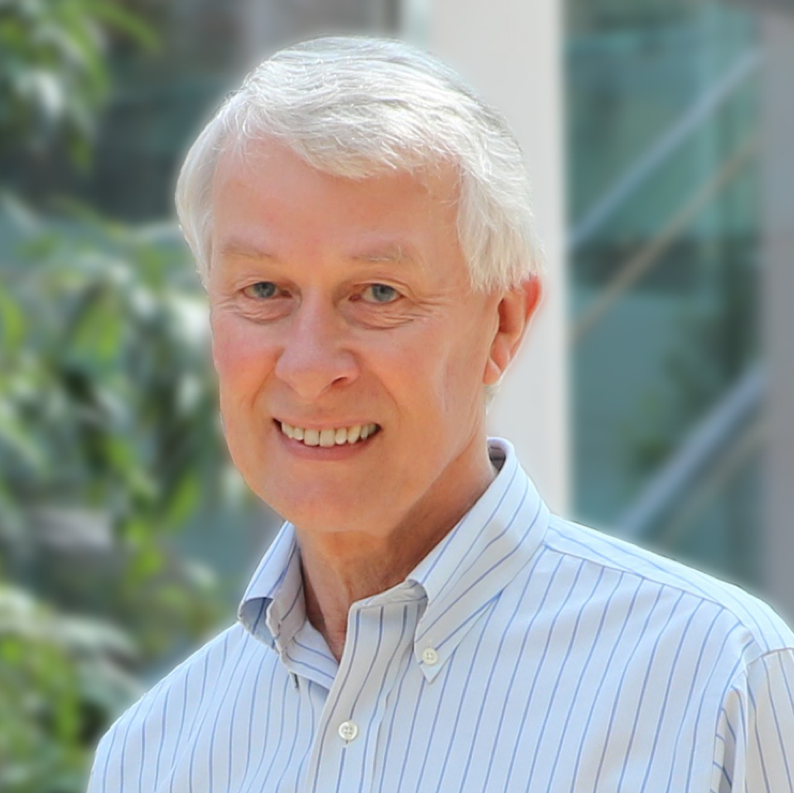
Richard J. Roberts, New England Biolabs, Inc.
"The Path to the Nobel Prize"
Fall 2017
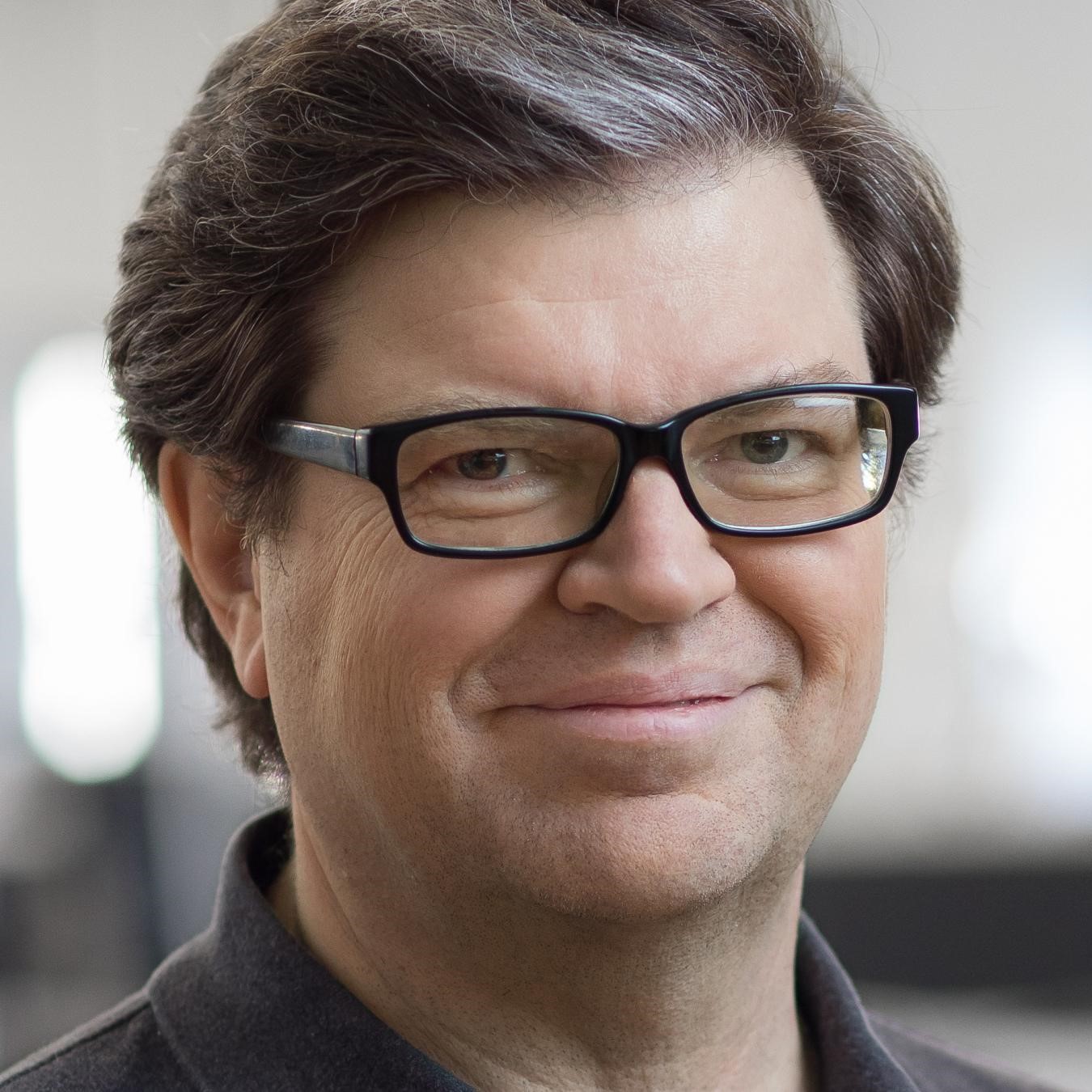
Yann LeCun, Facebook AI Research
"Obstacles to Progress in Deep Learning & AI"
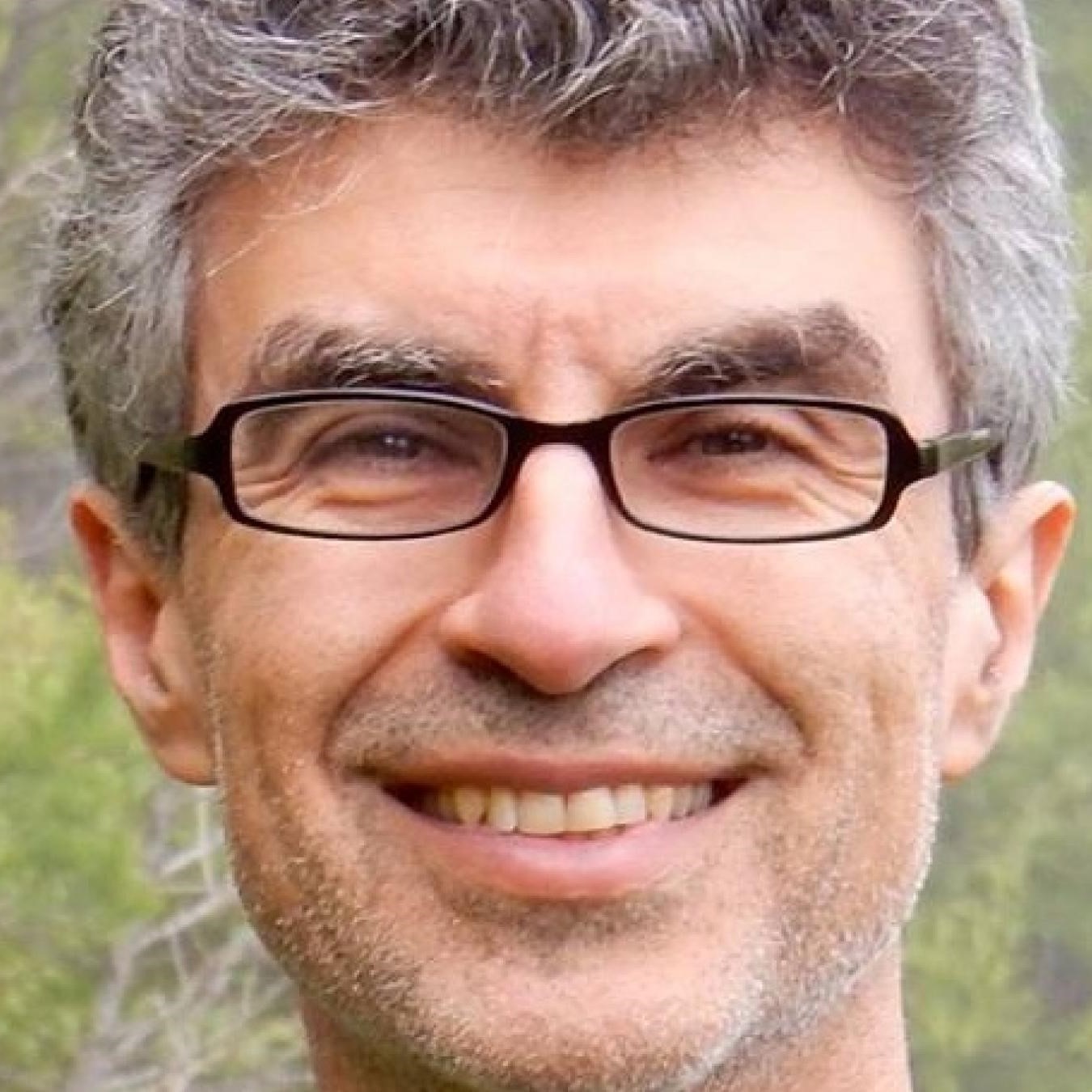
Yoshua Bengio, Montreal Institute for Learning Algorithms
"GANs and Unsupervised Representation Learning"
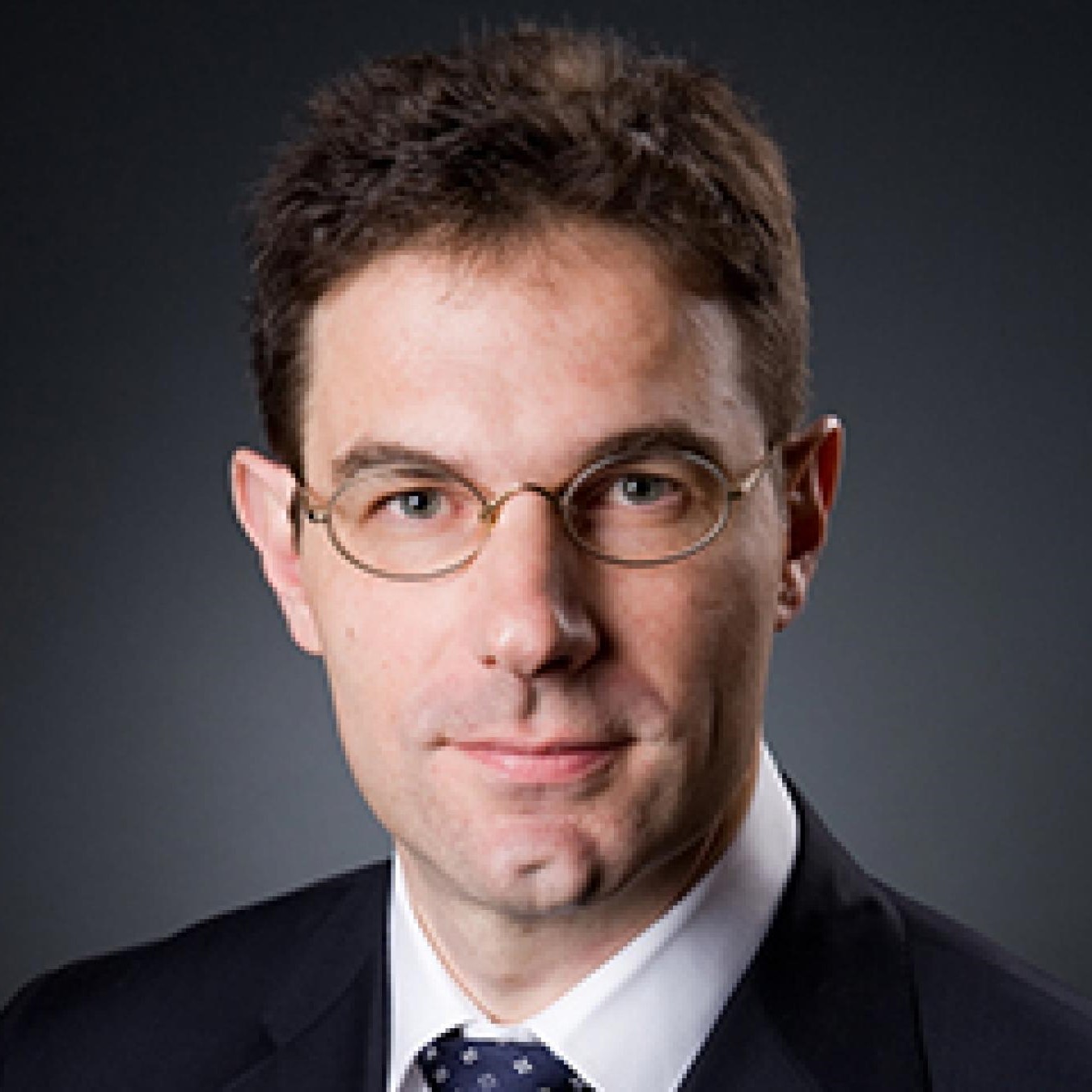
Stefano Soatto, UCLA Vision Lab
"The Information Knot Tying Sensing and Action"
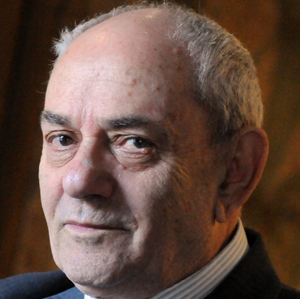
Vladimir Vapnik, Columbia University
"Rethinking Statistical Learning Theory"