Explainability and Regulation
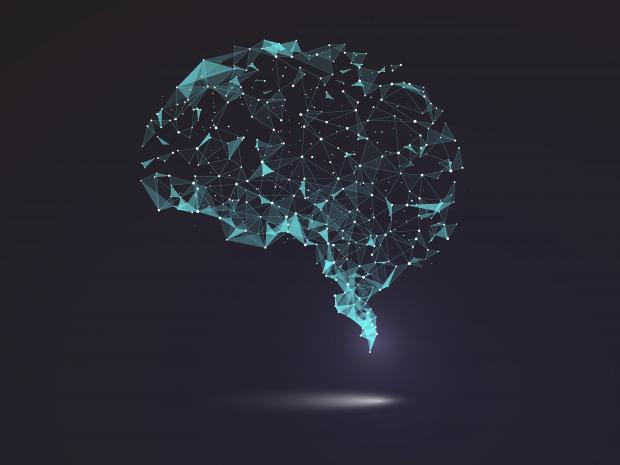
Part of the Special ECE Seminar Series
Modern Artificial Intelligence
Title:
Explainability and regulation
Speaker:
Ulrike von Luxbur, University of Tuebingen, Germany
Abstract:
Explainability is one of the concepts that dominate debates about the regulation of machine learning algorithms. In my presentation I will argue that in its current form, post-hoc explanation algorithms are unsuitable to achieve the law's objectives, for rather fundamental reasons. In particular, most situations where explanations are requested are adversarial, meaning that the explanation provider and receiver have opposing interests and incentives, so that the provider might manipulate the explanation for her own ends. I then discuss a theoretical analysis of Shapley Value based explanation algorithms that open the door to more formal guarantees for posthoc explanations.
Bio:
Ulrike von Luxburg is a full professor for the Theory of Machine Learning at the University of Tuebingen, Germany. Her research analyzes machine learning algorithms from a theoretical point of view, tries to understand their implicit mechanisms, and to give formal statistical guarantees for their performance. In this way, she reveals fundamental assumptions, biases and strenghts and weaknesses of widely used machine learning algorithms, for example in the field of explainable machine learning. Next to her own research group, she is coordinating a large research consortium on Machine Learning in Science. She is an active participant in local debates about ethics and responsibility in machine learning.