Learning to act in noisy contexts using deep proxy learning
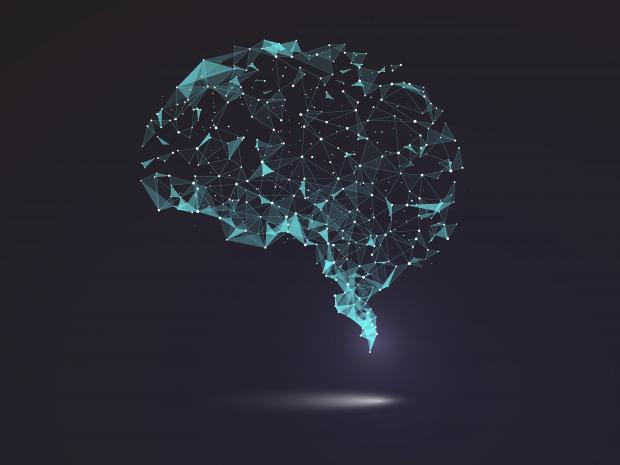
Part of the Special ECE Seminar Series
Modern Artificial Intelligence
Title:
Learning to act in noisy contexts using deep proxy learning
Speaker:
Arthur Gretton, Gatsby Computational Neuroscience Unit, Director of the Centre for Computational Statistics and Machine Learning (CSML) at UCL
Abstract:
We consider problem of evaluating the expected outcome of an action or policy, using off-policy observations of user actions, where the relevant context is noisy/anonymized. This scenario might arise due to privacy constraints, data bandwidth restrictions, or intrinsic properties of the setting.
We will employ the recently developed tool of proxy causal learning to address this problem. In brief, two noisy views of the context are used: one prior to the user action, and one subsequent to it, and influenced by the action. This pair of views will allow us to recover the average causal effect of an action under reasonable assumptions. As a key benefit of the proxy approach, we need never explicitly model or recover the hidden context. Our implementation employs learned neural net representations for both the action and context, allowing each to be complex and high dimensional (images, text). We demonstrate the deep proxy learning method in a setting where the action is an image, and show that we outperform an autoencoder-based alternative.
Bio:
Arthur Gretton is a Professor with the Gatsby Computational Neuroscience Unit, and director of the Centre for Computational Statistics and Machine Learning (CSML) at UCL; and a research scientist at Google Deepmind. His recent research interests include causal inference and representation learning, design and training of generative models, and nonparametric hypothesis testing.
Arthur has been an associate editor at IEEE Transactions on Pattern Analysis and Machine Intelligence, an Action Editor for JMLR, a Senior Area Chair for NeurIPS (2018,2021) and ICML (2022), a member of the COLT Program Committee in 2013, and a member of Royal Statistical Society Research Section Committee since January 2020. Arthur was program co-chair for AISTATS in 2016, tutorials co-chair for ICML 2018, workshops co-chair for ICML 2019, program co-chair for the Dali workshop in 2019, and co-organsier of the Machine Learning Summer School 2019 in London.