Building Reproducible, Reusable, and Robust Deep Reinforcement Learning Systems
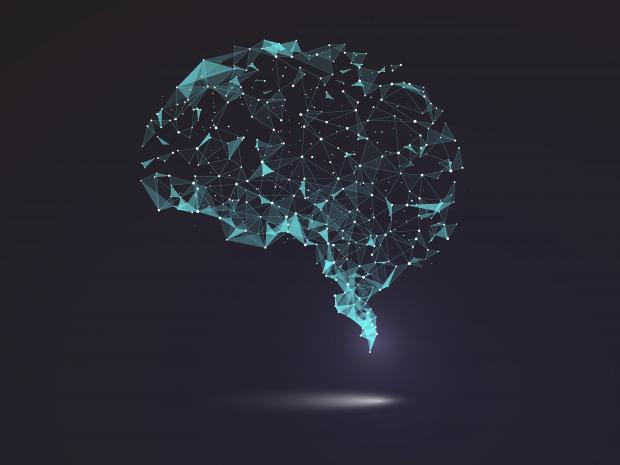
Part of the Special ECE Seminar Series
Modern Artificial Intelligence
Title:
Building Reproducible, Reusable, and Robust Deep Reinforcement Learning Systems
Speaker:
Joelle Pineau, co-Managing Director of Facebook AI Research and Associate Professor at McGill University
Abstract:
We have seen amazing achievements with machine learning in recent years. Yet reproducing results for state-of-the-art deep learning methods is seldom straightforward. Results can vary significantly given minor perturbations in the task specification, data or experimental procedure. This is of major concern for anyone interested in using machine learning in real-world applications. In this talk, I will review challenges that arise in experimental techniques and reporting procedures in deep learning, with a particular focus on reinforcement learning and applications to healthcare. I will also describe several recent results and guidelines designed to make future results more reproducible, reusable and robust.
Bio:
Joelle Pineau is the co-Managing Director of Facebook AI Research, where she oversees the Montreal, Seattle, Pittsburgh, and Menlo Park labs. She is also a faculty member at Mila and an Associate Professor and William Dawson Scholar at the School of Computer Science at McGill University, where she co-directs the Reasoning and Learning Lab. She holds a BASc in Engineering from the University of Waterloo, and an MSc and PhD in Robotics from Carnegie Mellon University. Dr. Pineau's research focuses on developing new models and algorithms for planning and learning in complex partially-observable domains.