Statistical physics, neural networks, and neuroscience: from then to now
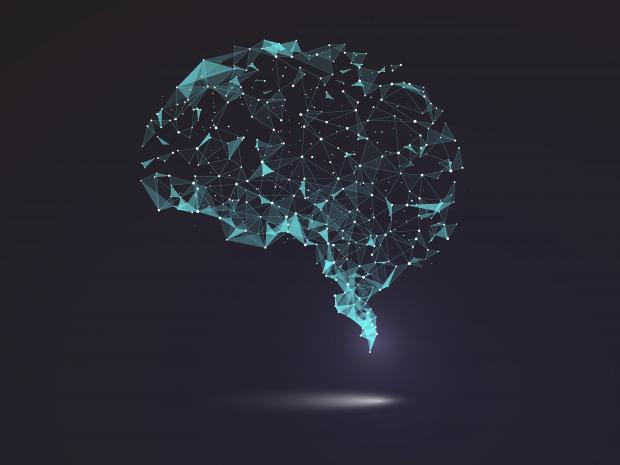
Part of the Special ECE Seminar Series
Modern Artificial Intelligence
Title:
Statistical physics, neural networks, and neuroscience: from then to now
Speaker:
Sara A. Solla, Department of Neuroscience, Department of Physics and Astronomy, Northwestern University
Abstract:
In early 80s, machine learning experienced a magnificent change. Work by John Hopfield and Geoffrey Hinton, published between 1982 and 1986, put forward several important concepts that are at the foundation of much current work: associative memories, recurrent neural networks, generative models, layered neural networks trained by gradient descent. The motivation and presentation style of this work attracted the attention of a generation of theoretical physicists trained in statistical physics who embraced this new area of research using their conceptual, mathematical, and computational tools. In addition to its contribution to current AI, this line of work opened a new field: theoretical and computational neuroscience. I will review the early work with an emphasis on its importance on laying the foundations for so much subsequent work, and provide some examples of current applications to neuroscience research.
Bio:
Sara A. Solla obtained a BSc in Mathematics and Physics and a MSc in Physics at the University of Buenos Aires in Argentina, and a Ph.D. in Physics at the University of Washington in Seattle. She is trained in statistical physics, critical phenomena, and renormalization group. While a postdoc at Cornell University in Ithaca, she heard John Hopfield talk about memory storage and retrieval through attractor dynamics, and life was never the same. She spent fruitful and exciting years as member of the legendary Neural Networks group at AT&T Bell Laboratories in Holmdel. She then joined the faculty at Northwestern University, where she is Professor of Neuroscience and Professor of Physics and Astronomy. Her work in theoretical and computational neuroscience uses conceptual, mathematical, and computational frameworks from statistical physics, statistical inference, and nonlinear dynamics to investigate information processing in the brain at the systems level.