AI Seminar Series: Stefano Soatto - The Information Knot Tying Sensing and Action
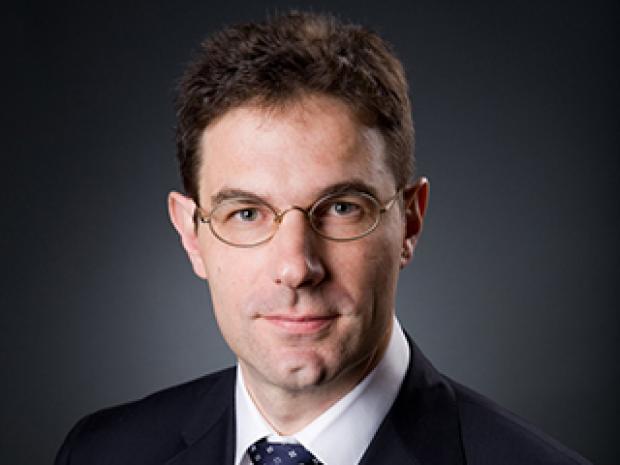
ECE Seminar Series on Modern Artificial Intelligence presents:
The Information Knot Tying Sensing and Action; Emergence Theory of Representation Learning
Representations are functions of past data useful to accomplish future decision or control tasks. Ideally, they should be as informative as the data (sufficient), unaffected by nuisance factors in future data (invariant), as simple as possible (minimal), and easy to work with (disentangled). Such ideal representations are what one should store in memory in lieu of past data. But do they exist? If so, can they be computed? or learned? Minimality and sufficiency can be achieved by optimizing the Information Bottleneck Lagrangian, but how to do so? And what about invariance and disentanglement?
At face value, these classical principles from statistical decision and information theory have little to do with Deep Learning, where an empirical decision criterion is optimized with respect to a biologically-inspired (parametric) family of functions using stochastic gradient descent (SGD). Despite its simplicity, however, SGD has some surprising properties: First, it does not converge in the classical sense, but instead exhibits limit cycles that can be far from the critical points of the empirical loss. Second, it induces a bias - or regularization - in the learning process that is reminiscent of the Information Bottleneck Lagrangian, but not the usual one: This new one measures the information the parameters of the network (weights) contain about past data. The ideal properties we want, however, pertain to future data. What is the relation between these two information bottlenecks, past and future?
The Emergence Theory shows that minimizing the information the weights of a deep neural network contain about past data bounds minimality, invariance and disentanglement of the resulting representation of future data (activations). The resulting bound can be derived equivalently using Information Theory, or from PAC-Bayes theory. So, (explicit or implicit) regularization of the empirical loss used in Deep Learning provably induces the emergence of desirable properties of the representation implemented. I willi discuss examples in visual recognition and control.
REFERENCES:
https://arxiv.org/pdf/1706.01350.pdf (to appear at JMLR) and https://arxiv.org/abs/1710.11029 (to appear at ICLR). Joint work with Alessandro Achille and Pratik Chaudhari.
Stefano Soatto Biography
Stefano Soatto is Professor of Computer Science and Electrical Engineering, and Director of the UCLA Vision Lab, in the Henry Samueli School of Engineering and Applied Sciences at UCLA. He is also Director of Applied Science at Amazon AI - AWS. He received his Ph.D. in Control and Dynamical Systems from the California Institute of Technology in 1996; he joined UCLA in 2000 after being Assistant and then Associate Professor of Electrical and Biomedical Engineering at Washington University, and Research Associate in Applied Sciences at Harvard University. Between 1995 and 1998 he was also Ricercatore in the Department of Mathematics and Computer Science at the University of Udine - Italy. He received his D.Ing. degree (highest honors) from the University of Padova- Italy in 1992. Dr. Soatto is the recipient of the David Marr Prize for work on Euclidean reconstruction and reprojection up to subgroups. He also received the Siemens Prize with the Outstanding Paper Award from the IEEE Computer Society for his work on optimal structure from motion. He received the National Science Foundation Career Award and the Okawa Foundation Grant. He was Associate Editor of the IEEE Transactions on Pattern Analysis and Machine Intelligence (PAMI) and a Member of the Editorial Board of the International Journal of Computer Vision (IJCV) and Foundations and Trends in Computer Graphics and Vision, Journal of Mathematical Imaging and Vision, SIAM Imaging. He is a Fellow of the IEEE.
Free and open to the public
This event will be live-streamed on engineering.nyu.edu/live
Upcoming Speakers:
- May 4, 2018: Vladimir Vapnik, Columbia University and Facebook AI Research