Convex Analysis at Infinity: An Introduction to Astral Space
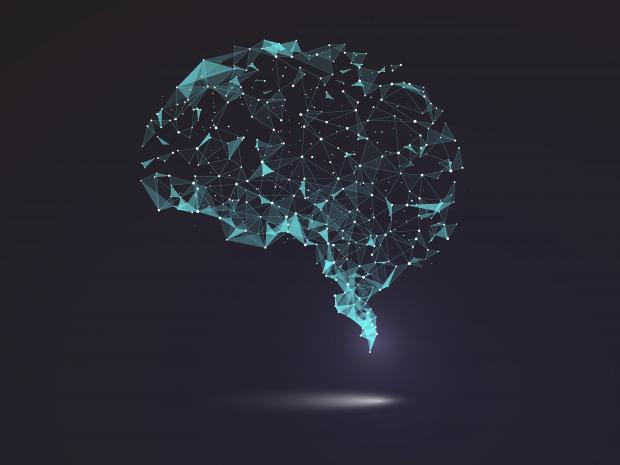
Part of the Special ECE Seminar Series
Modern Artificial Intelligence
Title:
Convex Analysis at Infinity: An Introduction to Astral Space
Speaker:
Robert Schapire, Microsoft Research
Abstract:
Not all convex functions have finite minimizers; some can only be minimized by a sequence as it heads to infinity. In this work, we aim to develop a theory for understanding such minimizers at infinity. We study astral space, a compact extension of Euclidean space to which such points at infinity have been added. Astral space is constructed to be as small as possible while still ensuring that all linear functions can be continuously extended to the new space. Although not a vector space, nor even a metric space, astral space is nevertheless so well-structured as to allow useful and meaningful extensions of such concepts as convexity, conjugacy, and subdifferentials. We develop these concepts and analyze various properties of convex functions on astral space, including the detailed structure of their minimizers, exact characterizations of continuity, and convergence of descent algorithms.
This is joint work with Miroslav Dudík, Ziwei Ji, and Matus Telgarsky.
Bio:
Robert Schapire is a Partner Researcher at Microsoft Research in New York City. He received his PhD from MIT in 1991. After a short postdoc at Harvard, he joined the technical staff at AT&T Labs (formerly AT&T Bell Laboratories) in 1991. In 2002, he became a Professor of Computer Science at Princeton University. He joined Microsoft Research in 2014. His awards include the 1991 ACM Doctoral Dissertation Award, the 2003 Gödel Prize, and the 2004 Kanelakkis Theory and Practice Award (both of the last two with Yoav Freund). He is a fellow of the AAAI, and a member of both the National Academy of Engineering and the National Academy of Sciences. His main research interest is in theoretical and applied machine learning.