Siddharth Garg
-
Institute Associate Professor
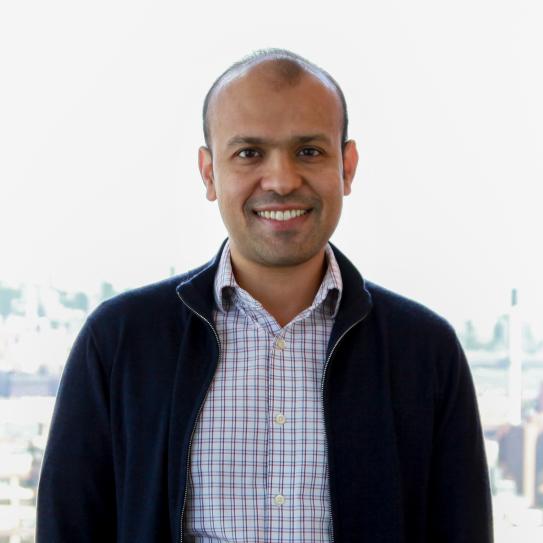
Siddharth Garg is currently the Institute Associate Professor of ECE at NYU Tandon, where he leads the EnSuRe Research group (https://wp.nyu.edu/ensure_group/). Prior to that he was in Assistant Professor also in ECE from 2014-2020, and an Assistant Professor of ECE at the Unversity of Waterloo from 2010-2014. His research interests are in machine learning, cyber-security and computer hardware design.
He received his Ph.D. degree in Electrical and Computer Engineering from Carnegie Mellon University in 2009, and a B.Tech. degree in Electrical Enginerring from the Indian Institute of Technology Madras. In 2016, Siddharth was listed in Popular Science Magazine's annual list of "Brilliant 10" researchers. Siddharth has received the NSF CAREER Award (2015), and paper awards at the IEEE Symposium on Security and Privacy (S&P) 2016, USENIX Security Symposium 2013, at the Semiconductor Research Consortium TECHCON in 2010, and the International Symposium on Quality in Electronic Design (ISQED) in 2009. Siddharth also received the Angel G. Jordan Award from ECE department of Carnegie Mellon University for outstanding thesis contributions and service to the community. He serves on the technical program committee of several top conferences in the area of computer engineering and computer hardware, and has served as a reviewer for several IEEE and ACM journals.
His research is supported in part by NYU WIRELESS.
Education
Stanford University, 2005
M.S., Electrical Engineering
Indian Institute of Technology Madras, 2004
B.Tech., Electrical Engineering
Carnegie Mellon University, 2009
Ph.D., Electrical and Computer Engineering
Experience
Carnegie Mellon University
Post-doctoral Fellow
From: October 2009 to August 2010
University of Waterloo
Assistant Professor of Electrical and Computer Engineering
From: September 2010 to August 2014
Publications
Journal Articles
Awards
- Popular Science Magazine's "Brilliant 10" of 2016
- Distinguighed Student Paper Award, IEEE Symp. on Security & Privacy (Oakland) 2016
- NSF CAREER Award 2015
- Best Student Paper Award, USENIX Security Symposium 2013
- Angel G. Jordan Award for thesis contributions, CMU 2010
- Best Paper Award, International Symposium on Quality in Electronic Design (ISQED) 2009
Research News
Studying the online deepfake community
In the evolving landscape of digital manipulation and misinformation, deepfake technology has emerged as a dual-use technology. While the technology has diverse applications in art, science, and industry, its potential for malicious use in areas such as disinformation, identity fraud, and harassment has raised concerns about its dangerous implications. Consequently, a number of deepfake creation communities, including the pioneering r/deepfakes on Reddit, have faced deplatforming measures to mitigate risks.
A noteworthy development unfolded in February 2018, just over a week after the removal of r/deepfakes, as MrDeepFakes (MDF) made its entrance into the online realm. Functioning as a privately owned platform, MDF positioned itself as a community hub, boasting to be the largest online space dedicated to deepfake creation and discussion. Notably, this purported communal role sharply contrasts with the platform's primary function — serving as a host for nonconsensual deepfake pornography.
Researchers at NYU Tandon led by Rachel Greenstadt, Professor of Computer Science and Engineering and a member of the NYU Center for Cybersecurity, undertook an exploration of these two key deepfake communities utilizing a mixed methods approach, combining quantitative and qualitative analysis. The study aimed to uncover patterns of utilization by community members, the prevailing opinions of deepfake creators regarding the technology and its societal perception, and attitudes toward deepfakes as potential vectors of disinformation.
Their analysis, presented in a paper written by lead author and Ph.D. candidate Brian Timmerman, revealed a nuanced understanding of the community dynamics on these boards. Within both MDF and r/deepfakes, the predominant discussions lean towards technical intricacies, with many members expressing a commitment to lawful and ethical practices. However, the primary content produced or requested within these forums were nonconsensual and pornographic deepfakes. Adding to the complexity are facesets that raise concerns, hinting at potential mis- and disinformation implications with politicians, business leaders, religious figures, and news anchors comprising 22.3% of all faceset listings.
In addition to Greenstadt and Timmerman, the research team includes Pulak Mehta, Progga Deb, Kevin Gallagher, Brendan Dolan-Gavitt, and Siddharth Garg.
Timmerman, B., Mehta, P., Deb, P., Gallagher, K., Dolan-Gavitt, B., Garg, S., Greenstadt, R. (2023). Studying the online Deepfake Community. Journal of Online Trust and Safety, 2(1). https://doi.org/10.54501/jots.v2i1.126
DeepReDuce: ReLU Reduction for Fast Private Inference
This research was led by Brandon Reagen, assistant professor of computer science and electrical and computer engineering, with Nandan Kumar Jha, a Ph.D. student under Reagen, and Zahra Ghodsi, who obtained her Ph.D. at NYU Tandon under Siddharth Garg, Institute associate professor of electrical and computer engineering.
Concerns surrounding data privacy are having an influence on how companies are changing the way they use and store users’ data. Additionally, lawmakers are passing legislation to improve users’ privacy rights. Deep learning is the core driver of many applications impacted by privacy concerns. It provides high utility in classifying, recommending, and interpreting user data to build user experiences and requires large amounts of private user data to do so. Private inference (PI) is a solution that simultaneously provides strong privacy guarantees while preserving the utility of neural networks to power applications.
Homomorphic data encryption, which allows inferences to be made directly on encrypted data, is a solution that addresses the rise of privacy concerns for personal, medical, military, government and other sensitive information. However, the primary challenge facing private inference is that computing on encrypted data levies an impractically high penalty on latency, stemming mostly from non-linear operators like ReLU (rectified linear activation function).
Solving this challenge requires new optimization methods that minimize network ReLU counts while preserving accuracy. One approach is minimizing the use of ReLU by eliminating uses of this function that do little to contribute to the accuracy of inferences.
“What we are to trying to do there is rethink how neural nets are designed in the first place,” said Reagen. “You can skip a lot of these time and computationally-expensive ReLU operations and still get high performing networks at 2 to 4 times faster run time.”
The team proposed DeepReDuce, a set of optimizations for the judicious removal of ReLUs to reduce private inference latency. The researchers tested this by dropping ReLUs from classic networks to significantly reduce inference latency while maintaining high accuracy.
The team found that, compared to the state-of-the-art for private inference DeepReDuce improved accuracy and reduced ReLU count by up to 3.5% (iso-ReLU count) and 3.5× (iso-accuracy), respectively.
The work extends an innovation, called CryptoNAS. Described in an earlier paper whose authors include Ghodsi and a third Ph.D. student, Akshaj Veldanda, CryptoNAS optimizes the use of ReLUs as one might rearrange how rocks are arranged in a stream to optimize the flow of water: it rebalances the distribution of ReLUS in the network and removes redundant ReLUs.
The investigators will present their work on DeepReDuce at the 2021 International Conference on Machine Learning (ICML) from July 18-24, 2021.
Subverting Privacy-Preserving GANs: Hiding Secrets in Sanitized Images
This research was led by Siddharth Garg, Institute Associate Professor of electrical and computer engineering, and included Benjamin Tan, a research assistant professor of electrical and computer engineering, and Kang Liu, a Ph.D. student.
Machine learning (ML) systems are being proposed for use in domains that can affect our day-to-day lives, including face expression recognition systems. Because of the need for privacy, users will look to use privacy preservation tools, typical produced by a third party. To this end, generative adversarial neural networks (GANs) have been proposed for generating or manipulating images. Versions of these systems called “privacy-preserving GANs” (PP-GANs) are designed to sanitize sensitive data (e.g., images of human faces) so that only application-critical information is retained while private attributes, such as the identity of a subject, are removed — by, for example, preserving facial expressions while replacing other identifying information.
Such ML-based privacy tools have potential applications in other privacy sensitive domains such as to remove location-relevant information from vehicular camera data; obfuscate the identity of a person who produced a handwriting sample; or remove barcodes from images. In the case of GANs, the complexity involved in training such models suggests the outsourcing of GAN training in order to achieve PP-GANs functionality.
To measure the privacy-preserving performance of PP-GANs researchers typically use empirical metrics of information leakage to demonstrate the (in)ability of deep learning (DL)-based discriminators to identify secret information from sanitized images. Noting that empirical metrics are dependent on discriminators’ learning capacities and training budgets, Garg and his collaborators argue that such privacy checks lack the necessary rigor for guaranteeing privacy.
In the paper “Subverting Privacy-Preserving GANs: Hiding Secrets in Sanitized Images,” the team formulated an adversarial setting to "stress-test" whether empirical privacy checks are sufficient to guarantee protection against private data recovery from data that has been “sanitized” by a PP-GAN. In doing so, they showed that PP-GAN designs can, in fact, be subverted to pass privacy checks, while still allowing secret information to be extracted from sanitized images.
While the team’s adversarial PP-GAN passed all existing privacy checks, it actually hid secret data pertaining to the sensitive attributes, even allowing for reconstruction of the original private image. They showed that the results have both foundational and practical implications, and that stronger privacy checks are needed before PP-GANs can be deployed in the real-world.
“From a practical stand-point, our results sound a note of caution against the use of data sanitization tools, and specifically PP-GANs, designed by third-parties,” explained Garg.
The study, which will be presented at the virtual 35thAAAI Conference on Artificial Intelligence, provides background on PP-GANs and associated empirical privacy checks; formulates an attack scenario to ask if empirical privacy checks can be subverted, and outlines an approach for circumventing empirical privacy checks.
- The team provides the first comprehensive security analysis of privacy-preserving GANs and demonstrate that existing privacy checks are inadequate to detect leakage of sensitive information.
- Using a novel steganographic approach, they adversarially modify a state-of-the-art PP-GAN to hide a secret (the user ID), from purportedly sanitized face images.
- They show that their proposed adversarial PP-GAN can successfully hide sensitive attributes in “sanitized” output images that pass privacy checks, with 100% secret recovery rate.
“Our experimental results highlighted the insufficiency of existing DL-based privacy checks, and potential risks of using untrusted third-party PP-GAN tools,” said Garg, in the study.
Resource constrained mobile data analytics assisted by the wireless edge
The National Science Foundation grant for this research was obtained by Siddharth Garg and Elza Erkip, professors of electrical and computer engineering, and Yao Wang, professor of computer science and engineering and biomedical engineering. Wang and Erkip are also members of the NYU WIRELESS research center.
Increasing amounts of data are being collected on mobile and internet-of-things (IoT) devices. Users are interested in analyzing this data to extract actionable information for such purposes as identifying objects of interest from high-resolution mobile phone pictures. The state-of-the-art technique for such data analysis employs deep learning, which makes use of sophisticated software algorithms modeled on the functioning of the human brain. Deep learning algorithms are, however, too complex to run on small, battery constrained mobile devices. The alternative, i.e., transmitting data to the mobile base station where the deep learning algorithm can be executed on a powerful server, consumes too much bandwidth.
This project that this NSF funding will support seeks to devise new methods to compress data before transmission, thus reducing bandwidth costs while still allowing for the data to be analyzed at the base station. Departing from existing data compression methods optimized for reproducing the original images, the team will develop a means of using deep learning itself to compress the data in a fashion that only keeps the critical parts of data necessary for subsequent analysis. The resulting deep learning based compression algorithms will be simple enough to run on mobile devices while drastically reducing the amount of data that needs to be transmitted to mobile base stations for analysis, without significantly compromising the analysis performance.
The proposed research will provide greater capability and functionality to mobile device users, enable extended battery lifetimes and more efficient sharing of the wireless spectrum for analytics tasks. The project also envisions a multi-pronged effort aimed at outreach to communities of interest, educating and training the next generation of machine learning and wireless professionals at the K-12, undergraduate and graduate levels, and broadening participation of under-represented minority groups.
The project seeks to learn "analytics-aware" compression schemes from data, by training low-complexity deep neural networks (DNNs) for data compression that execute on mobile devices and achieve a range of transmission rate and analytics accuracy targets. As a first step, efficient DNN pruning techniques will be developed to minimize the DNN complexity, while maintaining the rate-accuracy efficiency for one or a collection of analytics tasks.
Next, to efficiently adapt to varying wireless channel conditions, the project will seek to design adaptive DNN architectures that can operate at variable transmission rates and computational complexities. For instance, when the wireless channel quality drops, the proposed compression scheme will be able to quickly reduce transmission rate in response while ensuring the same analytics accuracy, but at the cost of greater computational power on the mobile device.
Further, wireless channel allocation and scheduling policies that leverage the proposed adaptive DNN architectures will be developed to optimize the overall analytics accuracy at the server. The benefits of the proposed approach in terms of total battery life savings for the mobile device will be demonstrated using detailed simulation studies of various wireless protocols including those used for LTE (Long Term Evolution) and mmWave channels.
This award reflects NSF's statutory mission and has been deemed worthy of support through evaluation using the Foundation's intellectual merit and broader impacts review criteria.