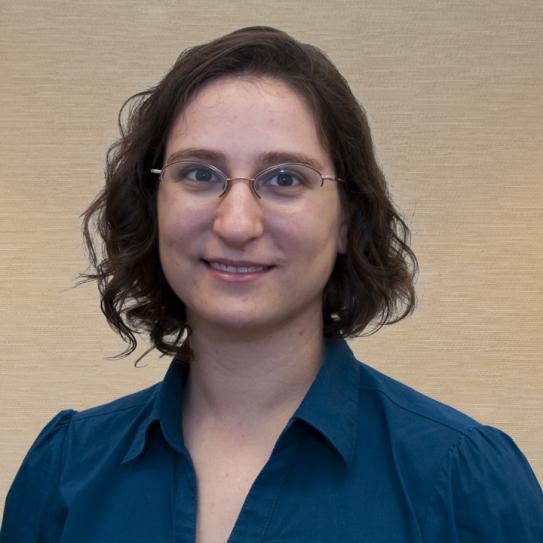
Rachel Greenstadt holds a bachelor’s degree in Computer Science (2001) and master’s degrees in Electrical Engineering and Computer Science (2002) from MIT, as well as a Ph.D. (2007) in Computer Science from Harvard. Her honors have included membership in the DARPA Computer Science Study Group, a U.S. Department of Homeland Security Fellowship, a PET Award for Outstanding Research in Privacy Enhancing Technologies, and a National Science Foundation CAREER Award.
Greenstadt's research has focused on designing more trustworthy intelligent systems — systems that act not only autonomously, but also with integrity, so that they can be trusted with important data and decisions. She takes a highly interdisciplinary approach to this research, incorporating ideas from artificial intelligence, psychology, economics, data privacy, and system security.
Prior to joining NYU, Greenstadt was an Associate Professor of Computer Science at Drexel University, where she ran the highly regarded Privacy, Security, and Automation Laboratory (PSAL) and served as an advisor to the Drexel Women in Computing Society. Before that, she was a Postdoctoral Fellow at Harvard’s School of Engineering and Applied Sciences, a Visiting Scholar with the University of Southern California TEAMCORE group, and a Research Intern at Lawrence Livermore National Laboratory. Throughout her career, she has edited multiple volumes of the journal Proceedings on Privacy Enhancing Technologies (PoPETs) and has been in demand as a peer reviewer. Greenstadt has chaired the ACM Workshop on Artificial Intelligence and Security multiple times and has regularly participated in, spoken at, and served on program committees for several other workshops building ties between the security, AI, and usability communities. She has long been an active speaker and participant in the international hacking community, and her work has been presented at Hacking at Random, Vierhouten, NL, ShmooCon, DefCon, and the Chaos Communication Congress.
Research News
Large Language Models fall short in detecting propaganda
In an era of rampant misinformation, detecting propaganda in news articles is more crucial than ever. A new study, however, suggests that even the most advanced artificial intelligence systems struggle with this task, with some propaganda techniques proving particularly elusive.
In a paper presented at the 5th International Workshop on Cyber Social Threats, part of the 18th International AAAI Conference on Web and Social Media in June 2024, Rachel Greenstadt — professor in the Computer Science and Engineering Department and a member of NYU Center for Cybersecurity — and her Ph.D. advisee Julia Jose evaluated several large language models (LLMs), including OpenAI's GPT-3.5 and GPT-4, and Anthropic's Claude 3 Opus, on their ability to identify six common propaganda techniques in online news articles:
- Name-calling: Labeling a person or idea negatively to discredit it without evidence.
- Loaded language: Using words with strong emotional implications to influence an audience.
- Doubt: Questioning the credibility of someone or something without justification.
- Appeal to fear: Instilling anxiety or panic to promote a specific idea or action.
- Flag-waving: Exploiting strong patriotic feelings to justify or promote an action or idea.
- Exaggeration or minimization: Representing something as excessively better or worse than it really is.
The study found that while these AI models showed some promise, they consistently underperformed compared to more specialized systems designed for propaganda detection.
“LLMs tend to perform relatively well on some of the more common techniques such as name-calling and loaded language,” said Greenstadt. “Their accuracy declines as the complexity increases, particularly with ‘appeal to fear’ and ‘flag-waving’ techniques.”
The baseline model, built on a technology called RoBERTa-CRF, significantly outperformed the LLMs across all six propaganda techniques examined. The researchers noted, however, that GPT-4 did show improvements over its predecessor, GPT-3.5, and outperformed a simpler baseline model in detecting certain techniques like name-calling, appeal to fear, and flag-waving.
These findings highlight the ongoing challenges in developing AI systems capable of nuanced language understanding, particularly when it comes to detecting subtle forms of manipulation in text.
"Propaganda often relies on emotional appeals and logical fallacies that can be difficult even for humans to consistently identify," Greenstadt said. "Our results suggest that we still have a long way to go before AI can reliably assist in this critical task, especially with more nuanced techniques. They also serve as a reminder that, for now, human discernment remains crucial in identifying and countering propaganda in news media.”
The study, which was supported by the National Science Foundation under grant number 1940713, adds to Greenstadt's body of work centering on developing intelligent systems that are not only autonomous but also reliable and ethical. Her research aims to create AI that can be entrusted with crucial information and decision-making processes.
Privacy-enhancing browser extensions fail to meet user needs, new study finds
Popular web browser extensions designed to protect user privacy and block online ads are falling short, according to NYU Tandon School of Engineering researchers, who are proposing new measurement methodologies to better uncover and quantify these shortcomings.
Led by Rachel Greenstadt, professor in the NYU Tandon Computer Science and Engineering (CSE) Department, the team will present its study at the 19th ACM ASIA Conference on Computer and Communications Security, taking place July 1–5, 2024 in Singapore.
Through an analysis of over 40,000 user reviews of seven of the most most popular privacy-preserving Chrome extensions, the researchers identified five key concerns among users: Performance, referring to the extent the extensions slowed down the system; Web compatibility, indicating how much they disrupted websites or caused substantial rendering delays; Data and Privacy Policy, pertaining to how the extensions handled user data; Effectiveness, evaluating how well they fulfilled their advertised purpose; and Default Configurations, assessing users' trust in the default settings.
"Our study found that there's a disconnect between what users want and what these extensions are actually providing," said Ritik Roongta, CSE PhD student who is the lead author of the study. "Developers need to do a much better job of understanding and addressing the real-world pain points."
The researchers analyzed extensions that fall into two main groups. The first category, dubbed “Ad-Blockers & Privacy Protection,” comprised extensions that block advertisements and third-party trackers. These include AdBlock Plus (ABP), uBlock Origin, Adguard, and Ghostery.
The second category, called “Privacy Protection,” encompasses extensions primarily focused on enhancing user privacy by blocking trackers and other privacy-invasive elements. This category includes Privacy Badger, Decentraleyes, and Disconnect.
The research team found that existing academic studies and benchmarking efforts had comprehensively explored just 4 out of the 14 key metrics underlying these five main user concerns. Crucial aspects like RAM usage overhead, ad-blocker detection likelihood, privacy policy soundness and adequacy of filtering rules were overlooked.
To bridge these research gaps, the researchers designed novel measurement methodologies and conducted extensive evaluation of the extensions against the unexplored metrics, providing a new benchmarking framework for evaluating the strengths and shortcomings of these privacy tools.
Their experiments involved smart crawlers visiting over 1,500 websites to analyze performance hits, compatibility issues, privacy policy strengths, ad-blocking capabilities and filter list configurations.
"The goal of this study is not to compare extensions specifically but to come up with a standardized benchmarking framework that addresses all user concerns so that the user can make informed decisions,” said Roongta. “As extensions evolve with every update, they might over- or underperform in different metrics at different times.”
The new measurement methodologies the researchers applied painted a mixed picture of the extensions they studied. While extensions like uBlock Origin optimized performance overheads well, most others like ABP exhibited significant CPU and memory overheads. Privacy Badger blocked ads and third-party trackers effectively while Ghostery struggled with them.
“Most of our analysis shows ABP needs to improve on metrics,” said Roongta. “That’s because it whitelists certain ads to show to the users. While this new dimension is often perceived critically by the users, it is important to sustain a free Internet. It will be interesting to see how user preferences change as these standards evolve with the advertiser policies over time and the system gets better so that the overhead caused by the extensions is negligible."
The study highlighted instances of potential permission abuse and non-compliance with data protection regulations by some of the evaluated extensions. It provided recommendations for extension developers to enhance transparency around data practices.
The research underscores the pressing need for more rigorous analysis and systematic benchmarking of privacy-preserving browser additions that millions entrust with their online data and browsing experience daily. It contributes to Greenstadt’s body of research that explores what happens when people try to use privacy-enhancing technologies and how the Internet responds.
The following chart shows the new and re-assessed metrics the NYU Tandon researchers introduced to evaluate browser extensions, as presented in From User Insights to Actionable Metrics: A User-Focused Evaluation of Privacy-Preserving Browser Extensions.
User Concern | Measurements |
---|---|
Performance | RAM usage: measure of the RAM used by the extensions during website loading. |
CPU usage: Measure of the CPU cycles used by the extensions during website loading (studied before but researchers re-assessed with enhanced measurement methods) | |
Data Usage: Measure of the disk space used by the extensions during website loading (studied before but researchers re-assessed with enhanced measurement methods). | |
Web compatibility | Ad-Blocker Detection Prompt: the number of websites that either employ javascript to detect the presence of an ad-blocker extension, or display a prompt asking the user to disable their ad-blocker. |
Unable to Load: websites taking longer than 60 seconds to load when the extensions are present. | |
Data and Privacy Policy | Permissions: evaluation of the extra permissions requested for the actual functioning of the extension. |
Privacy Policy: evaluation of the privacy policies of the extensions. | |
Extension Effectiveness | Ads: the extension's ability to block third-party trackers. |
Default Configurations | Default Filter Lists: set of rules to identify and block various web content like advertisements, trackers, and other unwanted elements from being loaded or displayed. |
Acceptable Ads: ABP filterlist that allows certain advertisements to appear that adhere to acceptable ad standards. Acceptable Ad Standards. |
Studying the online deepfake community
In the evolving landscape of digital manipulation and misinformation, deepfake technology has emerged as a dual-use technology. While the technology has diverse applications in art, science, and industry, its potential for malicious use in areas such as disinformation, identity fraud, and harassment has raised concerns about its dangerous implications. Consequently, a number of deepfake creation communities, including the pioneering r/deepfakes on Reddit, have faced deplatforming measures to mitigate risks.
A noteworthy development unfolded in February 2018, just over a week after the removal of r/deepfakes, as MrDeepFakes (MDF) made its entrance into the online realm. Functioning as a privately owned platform, MDF positioned itself as a community hub, boasting to be the largest online space dedicated to deepfake creation and discussion. Notably, this purported communal role sharply contrasts with the platform's primary function — serving as a host for nonconsensual deepfake pornography.
Researchers at NYU Tandon led by Rachel Greenstadt, Professor of Computer Science and Engineering and a member of the NYU Center for Cybersecurity, undertook an exploration of these two key deepfake communities utilizing a mixed methods approach, combining quantitative and qualitative analysis. The study aimed to uncover patterns of utilization by community members, the prevailing opinions of deepfake creators regarding the technology and its societal perception, and attitudes toward deepfakes as potential vectors of disinformation.
Their analysis, presented in a paper written by lead author and Ph.D. candidate Brian Timmerman, revealed a nuanced understanding of the community dynamics on these boards. Within both MDF and r/deepfakes, the predominant discussions lean towards technical intricacies, with many members expressing a commitment to lawful and ethical practices. However, the primary content produced or requested within these forums were nonconsensual and pornographic deepfakes. Adding to the complexity are facesets that raise concerns, hinting at potential mis- and disinformation implications with politicians, business leaders, religious figures, and news anchors comprising 22.3% of all faceset listings.
In addition to Greenstadt and Timmerman, the research team includes Pulak Mehta, Progga Deb, Kevin Gallagher, Brendan Dolan-Gavitt, and Siddharth Garg.
Timmerman, B., Mehta, P., Deb, P., Gallagher, K., Dolan-Gavitt, B., Garg, S., Greenstadt, R. (2023). Studying the online Deepfake Community. Journal of Online Trust and Safety, 2(1). https://doi.org/10.54501/jots.v2i1.126