NYU Tandon team demonstrates the potential dangers of incorporating deep neural networks into automotive systems
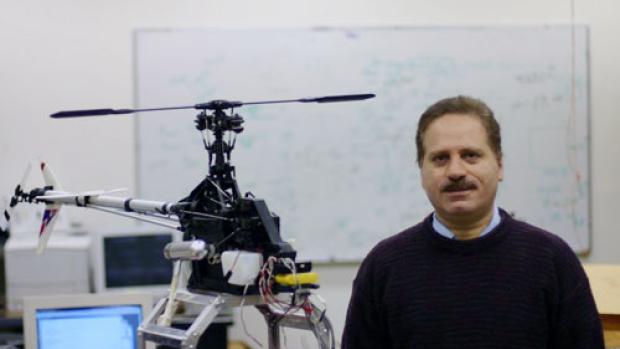
Professor of Electrical and Computer Engineering Farshad Khorrami
Digital billboards seem to be everywhere nowadays, flashing their brightly colored messages to anyone who drives by.
So, what’s the worst that can happen? Sure, they can be a little distracting, and there was that one time last year when pranksters broke into a shed housing the ad company’s computers and streamed X-rated content for 15 minutes over a Michigan highway.
A team from NYU Tandon recently erected a digital billboard on the side of a highway to prove a point: that the deep neural networks (DNNs) being incorporated into various autonomous systems like self-driving cars are open to adversarial attack by hackers using a car simulator.
Their billboard, which had an integrated camera estimating the pose of the on-coming vehicle in a high-fidelity simulator, displayed videos that caused the DNN controller in the car to generate potentially harmful steering commands, precipitating, for example, unintended lane changes or motion off the road.
The approach enables dynamic adversarial perturbation that adapts to the relative pose of the vehicle and uses its own dynamics to steer it along adversary-chosen trajectories while being robust to variations in view, lighting, and weather.
This stillshot of a video displays how a self-driving car will veer outside its lane when it encounters a digital billboard.
The team included Professor of Electrical and Computer Engineering Farshad Khorrami, research scientist Prashanth Krishnamurthy, and doctoral student Naman Patel of Tandon’s Controls/Robotics Research Laboratory, as well as Associate Professor of Electrical and Computer Engineering Siddharth Garg of the school’s Center for Cybersecurity. Their paper, Adaptive Adversarial Videos on Roadside Billboards: Dynamically Modifying Trajectories of Autonomous Vehicles,” was presented at the last IEEE Intelligent Robots and Systems (IROS), considered among the largest and most prestigious robotics conferences in the world. Additionally, the team has developed process-aware approaches for real-time monitoring of dynamical behaviors of unmanned vehicles and other cyber-physical systems to detect and mitigate anomalies, adversarial effects, sensor malfunctions, and other issues. (You can read the 2016 paper "Cybersecurity for Control Systems: A Process-Aware Perspective," published in IEEE’s Design and Test Magazine, for example, and "Learning-Based Real-Time Process-Aware Anomaly Monitoring for Assured Autonomy," which will be published in an upcoming issue of IEEE Transactions on Intelligent Vehicles).
The team has also developed robust approaches for real-time fusion of sensors on unmanned vehicles to enable accurate and resilient autonomous navigation under sensor failures (see "Sliding-Window Temporal Attention based Deep Learning System for Robust Sensor Modality Fusion for UGV Navigation," published last year in IEEE Robotics and Automation Letters). The team has also developed multi-modal sensor fusion algorithms for simultaneous localization and mapping (SLAM) in uncertain environments (see "Tightly Coupled Semantic RGB-D Inertial Odometry for Accurate Long-Term Localization and Mapping," presented at the 2019 International Conference on Advanced Robotics).
“There has been rising concern about the robustness of these automotive systems because of their susceptibility to adversarial attacks on DNNs,” Khorrami explained. “We wanted to show the effectiveness of an adversarial dynamic attack on an end-to-end trained DNN controlling an autonomous vehicle, but the proposed approach could also be applied to other systems driven by an end-to-end trained network.”