Researchers study how to unlock clinical risk-prediction models so they can be applied to multiple clinical settings
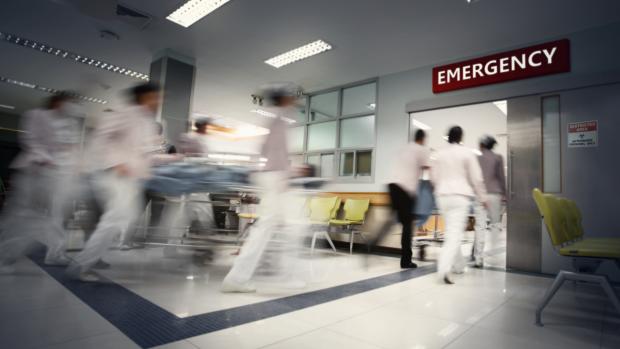
BROOKLYN, New York, Monday, April 11, 2022 – Modern predictive models require large amounts of data for training and evaluation, the absence of which may result in models that are specific to certain locations, their populations and the clinical practices there. Currently, best practices for clinical risk prediction models lack a level of “generalizability” that could vastly increase their usefulness for other clinical settings in other locations.
A team of NYU Tandon researchers led by Rumi Chunara, professor of computer science and engineering with affiliations with the NYU School of Global Public Health, investigated whether mortality prediction models vary significantly when applied to hospitals or geographies different from the ones in which they are developed. They also queried the data to determine specific characteristics of the datasets — involving analysis of electronic health records from 179 hospitals across the U.S. with 70,126 hospitalizations from 2014 to 2015 — that could explain variations in clinical performance based on factors like race.
In a new paper in PlOS Digital Health, the investigators, including Harvineet Singh, a Ph.D. student at the NYU Center for Data Science, and Vishwali Mhasawade, a Ph.D. candidate, both under Chunara’s direction, found that mortality risk prediction models that included clinical (vitals, labs and surgery) variables developed in one hospital or geographic region exhibited a lack of generalizability to different hospitals or regions. Based on a causal discovery analysis, they postulated that this lack of generalizability results from dataset shifts in race and clinical variables across hospitals or regions. In short, the race variable is intimately connected to clinical variables.
“It is clear from this research that data models — in terms of factors like mortality risk prediction at a hospital to hospital and regional hospital group level — are not immediately generalizable, and that has implications for hospitals that can’t generate these models for themselves,” said Chunara.
Findings also demonstrate evidence that predictive models can exhibit disparities in performance across racial groups even while performing well in terms of average population-wide metrics.
“While it is well documented that clinical factors and outcomes can vary significantly by race, it is critical that we understand why those differences exist, and thus examination of data and models must be done in a larger context alongside diverse influences, from geographic and socioeconomic to clinical,” she said.
Specifically, the study suggests that beyond algorithmic fairness metrics, an understanding of data generating processes for sub-groups is needed to identify and mitigate sources of variation, and to decide whether to use a risk prediction model in new environments.
The paper, “Generalizability challenges of mortality risk prediction models: A retrospective analysis on a multi-center,” can be found at journals.plos.org/digitalhealth
About the New York University Tandon School of Engineering
The NYU Tandon School of Engineering dates to 1854, the founding date for both the New York University School of Civil Engineering and Architecture and the Brooklyn Collegiate and Polytechnic Institute. A January 2014 merger created a comprehensive school of education and research in engineering and applied sciences as part of a global university, with close connections to engineering programs at NYU Abu Dhabi and NYU Shanghai. NYU Tandon is rooted in a vibrant tradition of entrepreneurship, intellectual curiosity, and innovative solutions to humanity’s most pressing global challenges. Research at Tandon focuses on vital intersections between communications/IT, cybersecurity, and data science/AI/robotics systems and tools and critical areas of society that they influence, including emerging media, health, sustainability, and urban living. We believe diversity is integral to excellence, and are creating a vibrant, inclusive, and equitable environment for all of our students, faculty and staff. For more information, visit engineering.nyu.edu.