Rumi Chunara
-
Associate Professor, Computer Science and Engineering and Biostatistics (Global Public Health)
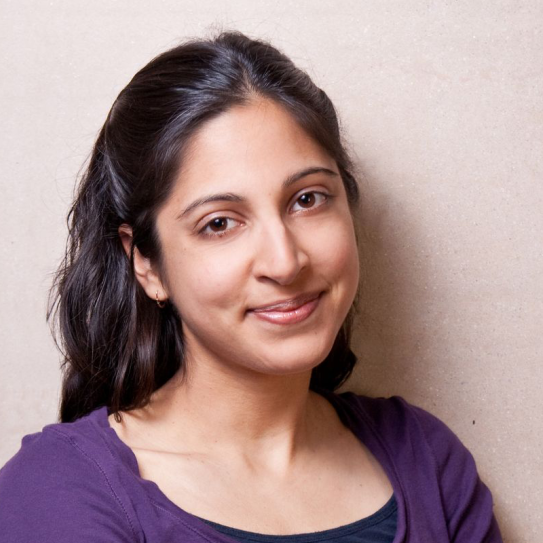
Dr. Chunara is Director of the Center for Health Data Science, and jointly appointed in Computer Science (Engineering) and Biostatistics (Public Health).
Her work and innovations in computer science and biostatistics are driven by themes of public health and equity. These themes have led to a focus on 1) Design and development of data mining and machine learning methods to address challenges related to data and goals of public health and 2) Fairness and ethics in the design and use of data and algorithms embedded in social systems.
She is an NSF CAREER, Facebook Research, Gates Foundation Grand Challenges, MIT Technology Review Top 35 Innovators Under 35 and Max Planck Sabbatical award winner.
MIT
Ph.D., Harvard-MIT Division of Health Sciences and Technology
MIT
S.M., Electrical Engineering and Computer Science
Caltech
B.S. (Honors), Electrical Engineering
About Me: At Tandon since 2015, jointly appointed at Tandon CSE and the School of Global Public Health in Biostatistics.
My Science: data science, machine learning, statistics, health
Research Briefs
Data science can be a valuable tool for analyzing social determinants of health and help solve root causes of health inequities
Data science methods can help overcome challenges in measuring and analyzing social determinants of health (SDoH), according to a paper published in Lancet Digital Health, helping mitigate the root causes of health inequities that are not fully addressed through health care spending or lifestyle choices.
The paper came out of the NYU-Moi Data Science Social Determinants Training Program (DSSD), a collaboration between New York University, the NYU Grossman School of Medicine, Moi University, and Brown University that is funded by the National Institutes of Health (NIH). Through interdisciplinary training at NYU, DSSD aims to build a cohort of data science trainees from Kenya.
Rumi Chunara, associate professor at both NYU Tandon School of Engineering and NYU School of Global Public Health, is a DSSD Program Principal Investigator and wrote the paper with colleagues from DSSD’s collaborating institutions and the NIH.
SDoH are the diverse conditions in people's environments that affect their health, such as racism and climate. These conditions can negatively impact quality of life and health outcomes by shaping economic policies, social norms, and other environmental factors that consequently influence individual behaviors.
According to the researchers, the three main challenges — and potential solutions — in studying SDoH are:
- SDoH data is hard to measure, especially at multiple levels like individual, community, and national, with racism being one notable example. Data science methods can help capture social determinants of health not easily quantified, like racism or climate impacts, from unstructured data sources including social media, notes, or imagery. For example, natural language processing can extract housing insecurity from medical notes, and deep learning can parse environmental factors from satellite imagery. These unstructured sources provide diverse insights compared to tabular, structured data, but also may contain biases requiring careful inspection. Incorporating social determinants from flexible, unstructured sources into analyses can better capture the heterogeneity of health effects across different populations.
- SDoH impact health through complex, nonlinear pathways over time. Social factors like income or education are farther removed from health outcomes than medical factors. They affect health through complicated chains of intermediate factors that can also flow back to influence the social factors. For instance, income provides resources for healthy behaviors that improve health, while poor health hinders income. Advanced modeling techniques like machine learning can handle these tangled relationships between many variables better than simpler statistical models. Models that simulate individuals' behaviors and interactions allow studying how health patterns emerge from social factors. This captures the real-world complexity traditional models may miss between broad social conditions and individual health.
- It takes a long time, sometimes decades, to observe how SDoH ultimately affect health outcomes. For example, lack of fresh produce and recreation options leads to poor nutrition, but chronic diseases take decades to develop. Longitudinal data over such time spans is rare, especially globally. Collecting representative surveys is resource-intensive. But novel digital data like mobile usage, purchases, or satellite imagery can provide longitudinal views at granular place and time scales. With proper privacy protections and population considerations, these new data managed with data science methods can help model social determinants' long-term health impacts.
Fully leveraging data science for SDoH research requires diverse experts working collaboratively across disciplines, according to the researchers. Training more data scientists, especially from underrepresented backgrounds, in SDoH is pivotal. Developing local data science skills grounded in community knowledge and values is also vital.
Along with Chunara, the paper’s authors are: Jessica Gjonaj from NYU School of Global Public Health and NYU Grossman; Rajesh Vedanthan from NYU Grossman; Eileen Immaculate, Iris Wanga, Judith Mangeni and Ann Mwangi from the College of Health Sciences at Moi University (Eldoret, Kenya); James Alaro and Lori A. J. Scott-Sheldon from the National Institutes of Health; and Joseph Hogan from Brown University.
Chunara, R., Gjonaj, J., Immaculate, E. et al. Social determinants of health: the need for data science methods and capacity. The Lancet Digital Health, 6(4), e235–e237 (2024). https://doi.org/10.1016/S2589-7500(24)00022-0
Authors
- Rumi Chunara
RAPID: Visualizing Epidemical Uncertainty for Personal Risk Assessment
The National Science Foundation RAPID grant for this research was obtained by Rumi Chunara and Enrico Bertini, professors in the Department of Computer Science and Engineering.
COVID-19 is one of the most deadly and fastest transmitting viruses in modern history. In response to this pandemic, news agencies, government organizations, citizen scientists, and many others have released hundreds of visualizations of pandemic forecast data. While providing people with accurate information is essential, it is unclear how the average person understands the widely distributed depictions of pandemic data. Prior research on uncertainty communication shows that even common visualizations can be confusing. One possible source of inappropriate responses to COVID-19 is the lack of knowledge about personal risk and the nature of pandemic uncertainty.
The goal of this research is to test how people understand currently available COVID-19 data visualizations and create communication guidelines based on these findings. Further, the researchers will develop an application to help people understand the factors that contribute to their risk. Users are able to interact with the application to learn about the impact of their actions on their risk. This research provides immediate solutions for teaching people about their personal risk associated with COVID-19 and how their actions influence the risks of others, which could improve the public's response and decrease fatalities. Additionally, this work supports decision making for future pandemics and any subsequent outbreaks of COVID-19 or other viruses.
Specifically, the research team, in an effort to understand how people respond to uncertainty about the nature of the pandemic, is testing the effects of currently available visualizations on personal risk judgments and behavior. By studying how changes in factors influence risk perceptions, the research can contribute to understanding how people conceptualize compound uncertainties from different sources (e.g., uncertainties associated with location, time, demographics and risk behaviors). The researchers are using this information to produce a visualization application that allows people to change the parameters of a simulation to see how the resulting changes affect their risk judgments. For example, users in one city are able to see the pandemic risk to individuals of their age in their zip code and then see how that risk would change if the infection rate increased or decreased.
The aim is to promote intuitive understanding of the epidemiological uncertainty in the forecast through participants? experimentation with the application. While in line with current recommendations for intrinsic uncertainty visualization, this work is the first of its kind to test the effect of user interaction to convey uncertainty through visualization.
This award reflects NSF's statutory mission and has been deemed worthy of support through evaluation using the Foundation's intellectual merit and broader impacts review criteria.
Authors
- Rumi Chunara
Telemedicine and Healthcare Disparities: A cohort study in a large healthcare system in New York City during COVID-19
Rumi Chunara, an assistant professor in the Department of Computer Science and Engineering at NYU Tandon, and in the Department of Biostatistics at NYU School of Global Public Health, was corresponding author.
Through the COVID-19 pandemic, telemedicine has become a necessary entry point into the process of diagnosis, triage and treatment. Racial and ethnic disparities in health care have been well documented in COVID-19 with respect to risk of infection and in-hospital outcomes once admitted. The researchers assessed disparities in those who access healthcare via telemedicine for COVID-19.
The researchers used electronic health record data of patients at New York University Langone Health between March 19th and April 30, 2020 to conduct descriptive and multilevel regression analyses with respect to visit type (telemedicine or in-person), suspected COVID diagnosis and COVID test results.
The collaborators included Yuan Zhao of the NYU School of Global Public Health; Ji Chen of the NYU Grossman School of Medicine; Katharine Lawrence, Paul A. Testa and Devin M. Mann of NYU Langone Health; and Oded Nov, professor in the Department of Technology Management and Innovation at NYU Tandon.
Controlling for individual and community-level attributes, the researchers found that Black patients had 0.6 times the adjusted odds of accessing care through telemedicine compared to white patients, though they are increasingly accessing telemedicine for urgent care, driven by a younger and female population. COVID diagnoses were significantly more likely for Black versus white telemedicine patients (while they were more likely for white patients when considering in-person and telemedicine visits).
While the study reports that Black patients are not accessing care through telemedicine (versus by in-person visits to emergency department and physician’s offices) at the same rate as white patients, it notes increased uptake by young, female Black patients. Mean income and decreased mean household size of patients' home zip code were also significantly related to telemedicine use.
The team reports that telemedicine access disparities reflect those in in-person healthcare access. Roots of disparate use are complex and reflect individual, community, and structural factors, including their intersection; many of which are due to systemic racism. Evidence regarding disparities that manifest through telemedicine can be used to inform tool design and systemic efforts to promote digital health equity.
The research, which was supported by a generous grant from the National Science Foundation,
Authors
- Rumi Chunara