Data science can be a valuable tool for analyzing social determinants of health and help solve root causes of health inequities
Paper comes from NYU-Moi Data Science Social Determinants Training Program
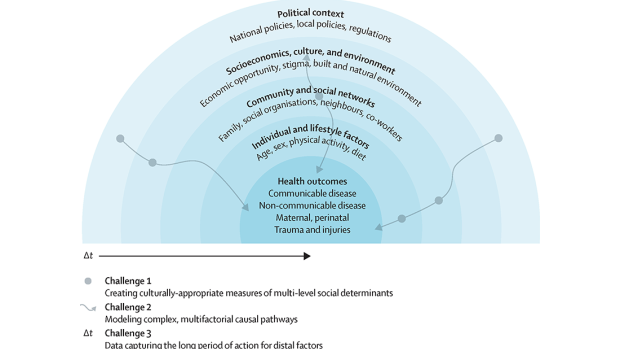
The concentric blue circles depict social determinants influencing health outcomes, guiding the application of data science methods. Three challenges are highlighted: capturing the exposure of interest at multiple levels (eg, individual, neighbourhood, and national) in a culturally appropriate manner, capturing complex relationships between variables and enabling flexibility between model components, and considerations of the time required to observe the impact of an exposure on a health outcome endpoint. Individuals with substantive knowledge of Social Determinants of Health (SDoH) and their effect on health outcomes in the appropriate contexts need to be equipped with data science skills to address each of these challenges. The complex pathways and exposures at different levels are illustrated by grey wavy arrows and circles, respectively, reflecting the diversity involved in various analyses.
Data science methods can help overcome challenges in measuring and analyzing social determinants of health (SDoH), according to a paper published in Lancet Digital Health, helping mitigate the root causes of health inequities that are not fully addressed through health care spending or lifestyle choices.
The paper came out of the NYU-Moi Data Science Social Determinants Training Program (DSSD), a collaboration between New York University, the NYU Grossman School of Medicine, Moi University, and Brown University that is funded by the National Institutes of Health (NIH). Through interdisciplinary training at NYU, DSSD aims to build a cohort of data science trainees from Kenya.
Rumi Chunara, associate professor at both NYU Tandon School of Engineering and NYU School of Global Public Health, is a DSSD Program Principal Investigator and wrote the paper with colleagues from DSSD’s collaborating institutions and the NIH.
SDoH are the diverse conditions in people's environments that affect their health, such as racism and climate. These conditions can negatively impact quality of life and health outcomes by shaping economic policies, social norms, and other environmental factors that consequently influence individual behaviors.
According to the researchers, the three main challenges — and potential solutions — in studying SDoH are:
- SDoH data is hard to measure, especially at multiple levels like individual, community, and national, with racism being one notable example. Data science methods can help capture social determinants of health not easily quantified, like racism or climate impacts, from unstructured data sources including social media, notes, or imagery. For example, natural language processing can extract housing insecurity from medical notes, and deep learning can parse environmental factors from satellite imagery. These unstructured sources provide diverse insights compared to tabular, structured data, but also may contain biases requiring careful inspection. Incorporating social determinants from flexible, unstructured sources into analyses can better capture the heterogeneity of health effects across different populations.
- SDoH impact health through complex, nonlinear pathways over time. Social factors like income or education are farther removed from health outcomes than medical factors. They affect health through complicated chains of intermediate factors that can also flow back to influence the social factors. For instance, income provides resources for healthy behaviors that improve health, while poor health hinders income. Advanced modeling techniques like machine learning can handle these tangled relationships between many variables better than simpler statistical models. Models that simulate individuals' behaviors and interactions allow studying how health patterns emerge from social factors. This captures the real-world complexity traditional models may miss between broad social conditions and individual health.
- It takes a long time, sometimes decades, to observe how SDoH ultimately affect health outcomes. For example, lack of fresh produce and recreation options leads to poor nutrition, but chronic diseases take decades to develop. Longitudinal data over such time spans is rare, especially globally. Collecting representative surveys is resource-intensive. But novel digital data like mobile usage, purchases, or satellite imagery can provide longitudinal views at granular place and time scales. With proper privacy protections and population considerations, these new data managed with data science methods can help model social determinants' long-term health impacts.
Fully leveraging data science for SDoH research requires diverse experts working collaboratively across disciplines, according to the researchers. Training more data scientists, especially from underrepresented backgrounds, in SDoH is pivotal. Developing local data science skills grounded in community knowledge and values is also vital.
Along with Chunara, the paper’s authors are: Jessica Gjonaj from NYU School of Global Public Health and NYU Grossman; Rajesh Vedanthan from NYU Grossman; Eileen Immaculate, Iris Wanga, Judith Mangeni and Ann Mwangi from the College of Health Sciences at Moi University (Eldoret, Kenya); James Alaro and Lori A. J. Scott-Sheldon from the National Institutes of Health; and Joseph Hogan from Brown University.
Chunara, R., Gjonaj, J., Immaculate, E. et al. Social determinants of health: the need for data science methods and capacity. The Lancet Digital Health, 6(4), e235–e237 (2024). https://doi.org/10.1016/S2589-7500(24)00022-0