Saif Eddin Jabari
,
Ph.D.
-
Associate Professor, NYUAD
-
Global Network Associate Professor NYU
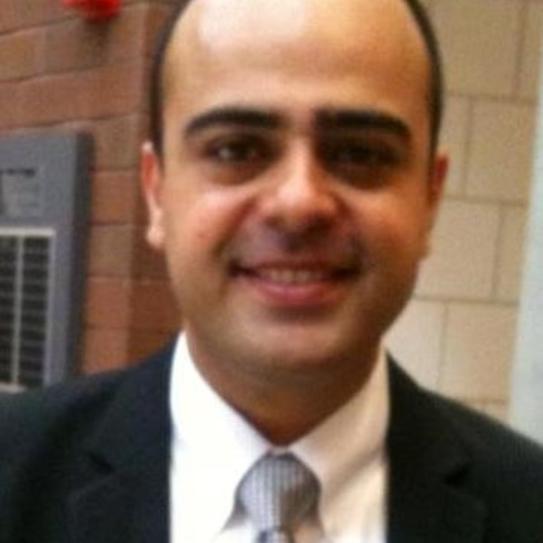
Saif Jabari is a Global Network Associate Professor of Civil and Urban Engineering at New York University. His research interests center on theoretical aspects of traffic flow, specifically topics related to modeling uncertainty and emergent phenomena. The applications focus on traffic operations problems, including traffic state estimation and prediction, distributed traffic control, and cybersecurity.
Prior to joining NYUAD, Jabari was a Post-Doctoral Researcher in the Mathematical Sciences and Analytics Department at the IBM T.J. Watson Research Center in Yorktown Heights, NY. Jabari received his Ph.D. in Civil Engineering from the University of Minnesota, Twin Cities in 2012 and his B.Sc. degree in Civil Engineering from in the University of Jordan in 2001.
Education
University of Minnesota, Twin Cities 2012
Doctor of Philosophy, Civil Engineering
University of Minnesota, Twin Cities 2009
Master of Science, Civil Engineering
University of Jordan 2001
Bachelor of Science, Civil Engineering
Experience
University of Minnesota, Twin Cities
Research Assistant
From: June 2006 to September 2012
New York University, Abu Dhabi
Assistant Professor
From: September 2014 to present
IBM T.J. Watson Research Center
Post-doctoral Researcher
From: November 2012 to August 2014
Arabtech Jardaneh, Engineers and Architects
Highway Engineer
From: May 2002 to June 2005
Awards
- Award for Innovation and Outstanding Teaching at NYUAD Engineering
- Milton Pikarsky Award. 2012 Best Doctoral Dissertation in Science and Technology. Awarded by the Council of University Transportation Centers (CUTC).
- Student of the Year. 2010 Student of the Year in ITS. Awarded by the ITS Institute of the University of Minnesota.
- Best MS Thesis in Civil Engineering, 2009. Awarded by the Department of Civil Engineering at the University of Minnesota.
Patents
Systems and methods for sparse travel time estimation (US20180232650A1)
Systems and methods for travel time density estimation based on sparse approximation. The density estimation problem is reformulated as a kernel-based regression problem and sparsity is the driving principle underlying model selection. This is particularly useful when the objective is to promote the predictive capabilities of the model and avoid overfitting; in the context of travel time density estimation, the objective is to determine the optimal number of kernels that can faithfully capture the multiple modes resulting from the state of traffic in the network.
Traffic Network Sensor Placement (US9466209 B2)
Locations for traffic sensors can be determined by a computer system that identifies a particular segment of a travel path. Traffic flow data from other segments of travel path are accessed based on traffic flow characteristics of the particular path. Using the traffic flow data, parameters for a traffic incident symptom propagation model are generated, and a location of a traffic incident along the segment of the path is determined. Using time-to-detection limits and the incident model, upstream and downstream distances are determined, and the locations of two sensors are identified based on the distances.
Traffic Incident Location Identification (US9286797 B1)
A location for a traffic incident can be determined by a computer system, using data from a first and second sensor along a travel path. A receiving and sending symptom of the traffic incident are detected from a first and second sensor, using traffic flow data from the sensors. The locations of the first and second sensors are determined. The location and traffic flow data from each sensor are used to create a sending and receiving profiles. From the profiles, a convergence formula is build. Using the convergence formula and by determining a convergence point for the sending and receiving symptoms, a time and location of the traffic incident is identified.