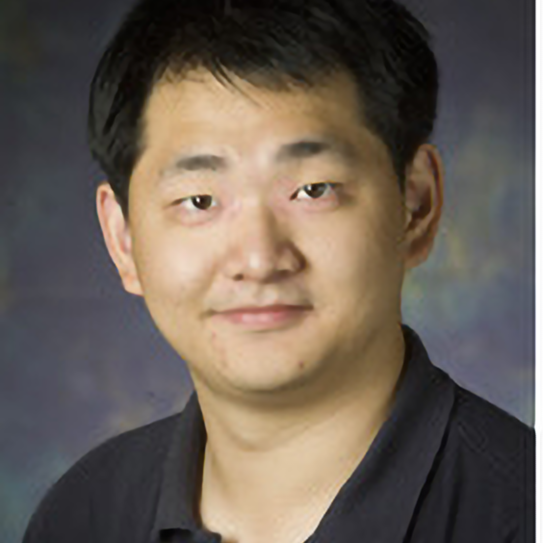
Game Theory | Control Theory | Cyber-physical System | Risk Management | Smart Grid | Wireless Network
University of Illinois at Urbana-Champaign, 2013
PhD, Electrical and Computer Engineering
University of Toronto, 2008
Master of Applied Science, Electrical and Computer Engineering
McGill University, 2006
B. ENG in Honours Electrical Engineering, Electrical and Computer Engineering
- J. Chen and Q. Zhu, Game and Decision Theoretic Approach to Resilient Interdependent Network Analysis and Design, SpringerBrief, 2020.
- S. Rass, S. Schauer, S. Konig, and Q. Zhu, Cyber-Security in Critical Infrastructures: A Game-Theoretic Approach, Advanced Sciences and Technologies for Security Applications, Springer, 2020.
- Q. Zhu and Z. Xu, Cyber-Physical Co-Design for Secure and Resilient Control Systems, Advances in Information Security, Springer, 2020.
- C. Rieger, I. Ray, Q. Zhu, and M. Haney (Eds.), Industrial Control Systems Security and Resiliency: Practice and Theory, Advances in Information Security, Series Volume 75, 2019, Springer.
- C. A. Kamhoua, C. Kiekintveld, F. Fang and Q. Zhu (Eds.), Game Theory and Machine Learning for Cyber Security, Wiley-IEEE Press, 2021. (to appear)
- J. Pawlick and Q. Zhu, Game Theory for Cyber Deception, Static & Dynamic Game Theory: Foundations & Applications Series, Birkhauser, 2020. (to appear).
- Q. Zhu, J. S. Baras, R. Poovendran, J. Chen (Eds.), Proceedings of 11th International Conference on Decision and Game Theory for Security (GameSec) 2020, College Park, MD, USA, October 28–30, 2020.
- ECE-UY 3064 Feedback Control Systems
- ECE-GY 9213 Game Theory
- ECE-GY 9223 Reinforcement Learning
- ECE-GY 9233 Cyber-Physical Human Networks
- ECE-GY 9243 Convex Optimization and Optimal Control
- ECE-GY 9253 Cyber Physical System Security
- ECE-GY 9263 Stochastic Processes and Control
- ECE-GY 5213 Introduction to System Engineering
- ECE-GY 6233 System Optimization Methods
Research Positions
Postdoctoral Fellowships
We are looking for PhD graduates who are interested in multidisciplinary research related to cyber-physical security and privacy, system and control theory, machine learning and game theory. Interested candidates should email their CV/Resume to qz494@nyu.edu
Research Assistantships/Graduate Assistantships
We are actively looking for motivated undergraduate and graduate students in research projects on the Internet of Things, cyber-physical systems, machine learning, smart cities and next-generation systems and control. Interested students should email their CV/Resume to qz494@nyu.edu
Project Courses
NYU undergraduate and graduate students can also get involved in research projects through the following courses:
- ECE-UY 4223 Electrical Engineering Design Project II
- ECE-UY 397 Senior Thesis
- ECE-GY9941 Advanced Projects III
- ECE-GY9953 Advanced Projects I
- ECE-GY9963 Advanced Projects II
- ECE-GY997x MS Thesis in Electrical & Computer Engineering
Undergraduate Summer Research Program:
Interested undergraduate students can apply using the following link
or send their CV/Resume to qz494@nyu.edu
Vertically Integrated Projects (VIP) Program
The Laboratory for Agile and Resilient Complex Systems (LARX) invites undergraduate students who are interested in the VIP on Smart Internet of Controlled Things.
Description: The Internet of Things foresees a highly networked future, where every object is integrated to interact with each other, allowing for communications between objects, as well as between humans and objects, which enables the control of intelligent systems in our daily lives. This project aims to design and prototype smart, resilient and secure IoTs that can adapt to changing environment, uncertainties, and adversarial behaviors. This project will allow students to leverage knowledge from communications, signal processing, and systems and control to build applications including smart homes, smart healthcare, smart grids and smart building.
Interested students should contact VIP Director at voltz@nyu.edu. For more information on the program, please see http://engineering.nyu.edu/research/student/vip
-
Workshop on Learning and Security for Multi-agent Systems, Virtual Conference, 59th IEEE Conference on Decision and Control, Dec 13th, 2020
- IEEE International Workshop on Information Forensics and Security, December 6-11, 2020
Research Briefs
NYU Tandon School of Engineering researchers develop algorithm for safer self-driving cars
In a promising development for self-driving car technology, a research team at NYU Tandon School of Engineering has unveiled an algorithm — known as Neurosymbolic Meta-Reinforcement Lookahead Learning (NUMERLA) — that could address the long-standing challenge of adapting to unpredictable real-world scenarios while maintaining safety.
The research was conducted by Quanyan Zhu, NYU Tandon associate professor of electrical and computer engineering, and his Ph.D. candidate Haozhe Lei.
Artificial intelligence and machine learning have helped self-driving cars operate in increasingly intricate scenarios, allowing them to process vast amounts of data from sensors, make sense of complex environments, and navigate city streets while adhering to traffic rules.
As they venture beyond controlled environments into the chaos of real-world traffic, however, such vehicles’ performance can falter, potentially leading to accidents.
NUMERLA aims to bridge the gap between safety and adaptability. The algorithm achieves this by continuously updating safety constraints in real-time, ensuring that self-driving cars can navigate unfamiliar scenarios while maintaining safety as the top priority.
The NUMERLA framework operates as follows: When a self-driving car encounters an evolving environment, it uses observations to adjust its “belief” about the current situation. Based on this belief, it makes predictions about its future performance within a specified timeframe. It then searches for appropriate safety constraints and updates its knowledge base accordingly.
The car's policy is adjusted using lookahead optimization with safety constraints, resulting in a suboptimal but empirically safe online control strategy.
One of the key innovations of NUMERLA lies in its lookahead symbolic constraints. By making conjectures about its future mode and incorporating symbolic safety constraints, the self-driving car can adapt to new situations on the fly while still prioritizing safety.
The researchers tested NUMERLA in a computer platform that simulates urban environments – specifically to ascertain its ability to accommodate jaywalkers — and it outperformed other algorithms in those scenarios.
Lei, Haozhe & Zhu, Quanyan. (2023). Neurosymbolic Meta-Reinforcement Lookahead Learning Achieves Safe Self-Driving in Non-Stationary Environments.
Authors
- Quanyan Zhu