NYU Tandon robotics researchers showcase groundbreaking innovations at ICRA 2023
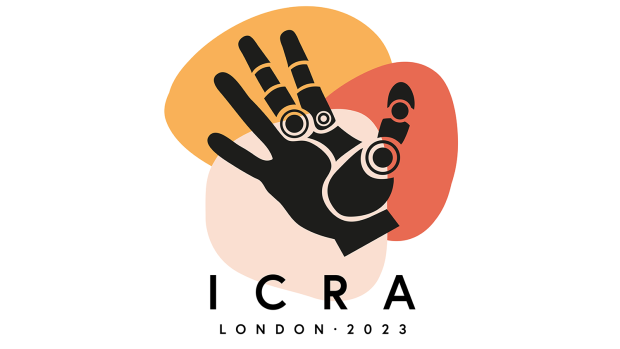
Story updated on August 1, 2023
In a remarkable display of cutting-edge advancements in robotics, a team of researchers from the NYU Tandon School of Engineering will present their groundbreaking work at this year's International Conference on Robotics and Automation (ICRA) held in London.
ICRA is the flagship conference in robotics, and attracts top scientists, engineers, and researchers from around the world. NYU Tandon's researchers, representing the departments of electrical and computer engineering, mechanical and aerospace engineering and civil and urban engineering, are presenting a record 16 papers for the institution, further solidifying its reputation as a leading institution in the field of robotics.
The NYU Tandon team will present groundbreaking research projects, which encompass various aspects of robotics and artificial intelligence including autonomy, locomotion, physical human-robot interaction, and perception.
New research to be presented includes:
- “GaPT: Gaussian Process Toolkit for Online Regression with Application to Learning Quadrotor Dynamics” from the lab of Giuseppe Loianno. Gaussian Processes (GPs) are expressive models for capturing signal statistics and expressing prediction uncertainty. As a result, the robotics community has gathered interest in leveraging these methods for inference, planning, and control. Unfortunately, GPs don’t scale very efficiently, making it difficult to use on Size, Weight, and Power (SWaP) constrained aerial robots.
The researchers propose GaPT, a novel toolkit that converts GPs to their state space form and performs regression in linear time. GaPT is designed to be highly compatible with several optimizers popular in robotics, and accurately captures the system behavior in multiple flight regimes and operating conditions.
- “MPC with Sensor-Based Online Cost Adaptation” from the Machines in Motion laboratory, led by Ludovic Righetti. Model predictive control is a powerful tool to generate complex motions for robots. However, it often requires solving very complicated optimization problems to produce rich behaviors, which is typically not feasible in real-time. Additionally, direct integration of high dimensional sensor data — such as vision or tactile sensing — in the feedback loop is challenging with current state-space methods.
This new paper aims to address both issues. It introduces a model predictive control scheme, where a neural network constantly updates the cost function of a quadratic program based on sensory inputs. By updating the cost, the robot is able to adapt to changes in the environment directly from sensor measurement without requiring a new cost design. And since the quadratic program can be solved efficiently with hard constraints, a safe deployment on the robot is ensured.
- "Upper-limb Geometric MyoPassivity Map for Physical Human-Robot Interaction” from the lab of S. Farokh Atashzar. This paper explores the intrinsic biomechanical characteristic of the human upper limb that plays a central role in absorbing the interactive energy during physical human-robot interaction (pHRI). The lab recently decoded such energetic behavior for both upper and lower limbs. That knowledge can be used in the design of controllers for optimizing the transparency and fidelity of force fields in human-robot interaction and in haptic systems.
In this paper, researchers investigated for the first time the frequency behavior of the passivity map for the upper limb when the muscle co-activation was controlled in real-time through visual electromyographic feedback. Results showed a correlation between Electromyography and EoP, which was further altered by increasing the frequency. The proposed energetic behavior is named the Geometric MyoPassivity (GMP) map.
- "Toward Zero-Shot Sim-to-Real Transfer Learning for Pneumatic Soft Robot 3D Proprioceptive Sensing" from the AI4CE lab of Chen Feng. Pneumatic soft robots present many advantages in manipulation tasks. Notably, their inherent compliance makes them safe and reliable in unstructured and fragile environments. However, full-body shape sensing for pneumatic soft robots is challenging because of their high degrees of freedom and complex deformation behaviors.
Vision-based proprioception sensing methods relying on embedded cameras and deep learning provide a good solution to proprioception sensing by extracting the full-body shape information from the high-dimensional sensing data. But the current training data collection process makes it difficult for many applications. To address this challenge, the researchers propose and demonstrate a robust sim-to-real pipeline that allows the collection of the soft robot's shape information in high-fidelity point cloud representation. They demonstrated the sim-to-real pipeline's potential for exploring different configurations of visual patterns to improve vision-based reconstruction results.
- “ERASE-Net: Efficient Segmentation Networks for Automotive Radar Signals” from the lab of Anna Choromanska. For autonomous vehicles, automotive radar has been considered as a robust and low-cost solution even in adverse weather or lighting conditions. With the recent development of radar technologies and open-sourced annotated data sets, semantic segmentation with radar signals has become very promising. However, existing methods are either computationally expensive or discard significant amounts of valuable information by converting images from raw 3D radar signals to 2D planes.
In this work, the researchers introduce ERASE-Net, an Efficient RAdar SEgmentation Network to segment the raw radar signals semantically. It first detects the center point of each object, then extracts a compact radar signal representation, and finally performs semantic segmentation. The method can achieve superior performance on radar semantic segmentation tasks compared to current techniques. It also requires up to 20x less computational resources. This work is a joint collaboration with NXP.
- “Self-Adaptive Driving in Nonstationary Environments through Conjectural Online Lookahead Adaptation” from the lab of Quanyan Zhu. Recent breakthroughs from machine learning have spurred wide interest and explorations in learning-based self driving. Deep reinforcement learning (RL) provides an end-to-end learning framework capable of solving self-driving tasks without manual designs, but time-varying nonstationary environments cause proficient but specialized RL policies to fail at execution time. (For example, an RL-based policy trained under sunny days does not generalize well to rainy weather.) Even though meta-learning enables the RL agent to adapt to new tasks/environments, its offline operation fails to equip the agent with online adaptation ability when facing nonstationary environments. Zhu and his co-authors propose an online meta-reinforcement learning algorithm based on the conjectural online lookahead adaptation (COLA), which determines the online adaptation at every step by maximizing the agent’s conjecture of the future performance in a lookahead horizon. Experimental results demonstrate that under dynamically changing weather and lighting conditions, the COLA-based self-adaptive driving outperforms the baseline policies regarding online adaptability.
In addition to the papers shown, Tandon researchers are presenting a large breadth of the research conducted at NYU as part of workshops. Loianno organized a workshop that will be devoted to the research and development related to the energy management in unmanned aerial systems and aerial robotics, increasing their ranges and efficiency. Feng is organizing two workshops. The first concerns collaborative perception, which may be able to mitigate some of the limitations of single-robot perception, including lack of real-world dataset, extra computational burden, high communication bandwidth, and subpar performance in adversarial scenarios. This workshop will provide a venue for academics and industry practitioners to create a vision for connected robots and vehicles to promote the safety and intelligence for humans. Feng is also organizing his second workshop titled “Future of Construction: Robot Perception, Mapping, Navigation, Control in Unstructured and Cluttered Environments,” which will facilitate discussion on technology that will enable advanced robotics for future construction workplaces with an emphasis on robust perception and navigation methods, learning-based task and motion planning, and safety-focused robot-worker interactions.
The work — both the papers and the presentations — emphasized the interdisciplinary nature of their work, highlighting the collaboration between robotics, artificial intelligence, computer vision, and other related fields. Such interdisciplinary approaches are vital for pushing the boundaries of robotics and unlocking its full potential for societal impact.
The full list of publications, workshops, and forums includes:
Publications
- F. Crocetti, J. Mao, S. Saviolo, G. Costante, and G. Loianno, "GaPT: Gaussian Process Toolkit for Online Regression with Application to Learning Quadrotor Dynamics", IEEE International Conference on Robotics and Automation (ICRA), 2023
- S. Saviolo, J. Mao, R. Balu TMB, V. Radakrishnan, and G. Loianno, "AutoCharge: Autonomous Charging for Perpetual Quadrotor Missions", IEEE International Conference on Robotics and Automation (ICRA), 2023
- S. Khorshidi, A. Gazar, N. Rotella, M. Naveau, L. Righetti, M. Bennewitz and M. Khadiv, "On the Use of Torque Measurement in Centroidal State Estimation", IEEE International Conference on Robotics and Automation (ICRA), 2023
- V. Dhedin, H. Li, S. Khorshidi, L. Mack, AKC Ravi, A. Meduri, P. Shah, F. Grimminger, L. Righetti, M. Khadiv, and J. Stueckler, "Visual-Inertial and Leg Odometry Fusion for Dynamic Locomotion", IEEE International Conference on Robotics and Automation (ICRA), 2023
- A. Meduri, H. Zhu, A. Jordana, and L. Righetti "MPC with Sensor-Based Online Cost Adaptation", IEEE International Conference on Robotics and Automation (ICRA), 2023
- K. Pfeiffer, Y. Jia, M. Yin, A K Veldanda, Y Hu, A Trivedi, JJ Zhang, S Garg, E Erkip, S Rangan, and L Righetti, “Path Planning under Uncertainty to Localize mmWave Sources”, IEEE International Conference on Robotics and Automation (ICRA), 2023
- A. Meduri, P. Shah, J. Viereck, M. Khadiv, I. Havoutis, amd L. Righetti, "Biconmp: A nonlinear model predictive control framework for whole body motion planning”, IEEE Transactions on Robotics (with ICRA presentation)
- P. Paik, S. Thudi, and S. F. Atashzar, "Power-Based Velocity-Domain Variable Structure Passivity Signature Control for Physical Human-(Tele)Robot Interaction", in IEEE Transactions on Robotics (with ICRA presentation), 2022, DOI: 10.1109/TRO.2022.3197932.
- X. Zhou, P. Paik, and S. F. Atashzar, "Upper-limb Geometric MyoPassivity Map for Physical Human-Robot Interaction”, IEEE International Conference on Robotics and Automation (ICRA), 2023
- S. Kumar, D. Hu Liu, F. S. Racz, M. Retana, S. Sharma, F. Iwane, B. Murphy, R. O'Keeffe, S. F. Atashzar, F. Alambeigi, J. del R. Millán, "CogniDaVinci: Towards Estimating Mental Workload Modulated by Visual Delays During Telerobotic Surgery -- An EEG-based Analysis”, IEEE International Conference on Robotics and Automation (ICRA), 2023
- Navid Feizi, Zahra Bahrami, S. Farokh Atashzar, Mehrdad R. Kermani, “Design Optimization and Data-driven Shallow Learning for Dynamic Modeling of a Smart Segmented Electroadhesive Clutch,” ICRA 2023
- Yoo, U., Zhao, H., Altamirano, A., Yuan, W. and Feng, C., "Toward Zero-Shot Sim-to-Real Transfer Learning for Pneumatic Soft Robot 3D Proprioceptive Sensing", IEEE International Conference on Robotics and Automation (ICRA), 2023
- Lu, Y., Li, Q., Liu, B., Dianat, M., Feng, C., Chen, S. and Wang, Y., "Robust Collaborative 3D Object Detection in Presence of Pose Errors", IEEE International Conference on Robotics and Automation (ICRA), 202
- Su, S., Li, Y., He, S., Han, S., Feng, C., Ding, C. and Miao, F, "Uncertainty quantification of collaborative detection for self-driving", IEEE International Conference on Robotics and Automation (ICRA), 2023
- S. Fang, H. Zhu, D. Bisla, A. Choromanska, S. Ravindran, D. Ren, R. Wu, "ERASE-Net: Efficient Segmentation Networks for Automotive Radar Signals", in the IEEE International Conference on Robotics and Automation (ICRA), 2023
- Tao Li, Haozhe Le, and Quanyan Zhu. “Self-Adaptive Driving in Nonstationary Environments through Conjectural Online Lookahead Adaptation,” in the IEEE International Conference on Robotics and Automation (ICRA), 2023
Workshops organization
- G. Loianno, Energy Efficient Aerial Robotic Systems Workshop
- C. Feng, et. al., CoPerception: Collaborative Perception and Learning Workshop
- C. Feng, et. al., 2nd Workshop on Future of Construction: Robot Perception, Mapping, Navigation, Control in Unstructured and Cluttered Environments
Invited talks at workshop
RAS Cluster Forum Organization:
- S. F. Atashzar, Cecilia Laschi, Ann Majewicz Fey, RAS Technical Committee (TC) Cluster Forums - Human-centred Life-like Robotics Cluster Forum,
- G. Loianno, M. Ang, J. J. Cheung, “Robotics Foundation Cluster Forum”, RAS Technical Committee (TC) Cluster Forums