AI: The Promises and the Pitfalls of the Biggest Story in Tech
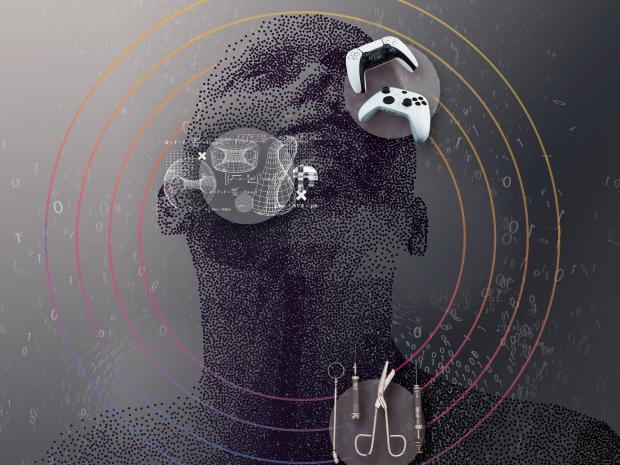
If you’ve read the news this year, you know the topic on everyone’s mind: AI. With the launch of publicly-available large language models (LLMs) and image generation software, many people were able to experience the power — and the limitations — of machine learning algorithms.
Here at NYU Tandon, AI has long been a focal point of our researchers’ work, from examining how it will change hardware and software design, to how it can be used in the future of medical care, to how it can be used (and misused) in some of the most important processes in your life.
We asked a few of our researchers how they saw AI changing their research, and how its potential may change their field in the future.
Creating responsible AI that works for everyone
As AI begins to infiltrate many of the key parts of our lives — including law enforcement, job hiring, housing, and much more — there is no more pressing moment than now to ensure that the use of AI is ethical and equitable.
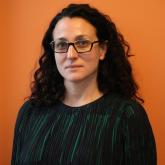
This year, Julia Stoyanovich — Associate Professor of Computer Science and Engineering and Director of the Center for Responsible AI — testified before the New York City Department of Consumer and Worker Protection regarding a first-of-its-kind law mandating transparency and bias protections for automated hiring tools.
Stoyanovich, whose research focuses on making AI responsible and equitable, and her colleagues had previously actively participated in guiding NYC on the development of Local Law 144 — which passed in 2021, and went into effect this year. The law will force employers who use automated employment decision tools to commission independent bias audits, publish a summary of the results, notify applicants and employees of the tool’s use and functioning, and let affected individuals know they may request an accommodation or alternative selection process.
Now, she’s looking to help ensure AI is used ethically in any number of cases. This year, the Center for Responsible AI partnered with New York City’s libraries on a “We Are AI” curriculum that teaches the fundamental of AI and ethics, just one example of how academics can teach the public about AI and its potential abuses. These educational opportunities — free of charge and available in public spaces — are aimed at creating a populace informed about AI and how it affects each of their lives, and giving them the tools to ask the right questions and fight for a more equitable society.
My prediction for the hopefully not-too-distant future is that we will find a way to make the use of AI socially sustainable. That we will collectively find a way to use this amazing technology for good — for the benefit of many people, and of many different kinds of people — while controlling the risks. To reach social sustainability, we will have to change how we think about AI innovation. It’s no longer technology first, society second. Technology is the easy part. The hard part is: ethics and values, laws and regulations, and training and education for all, so we can use AI productively and control it together!"
The AI Powering Medical Tools
Stroke is the leading cause of age-related motor disabilities and is becoming more prevalent in younger populations as well. But while there is a burgeoning marketplace for rehabilitation devices that claim to accelerate recovery, including robotic rehabilitation systems, recommendations for how and when to use them are based mostly on subjective evaluation.
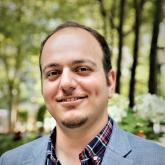
S. Farokh Atashzar, Assistant Professor of Electrical and Computer Engineering and Mechanical and Aerospace Engineering, as well as a member of NYU WIRELESS and the Center for Urban Science and Progress, is collaborating to design a regulatory science tool based on data from biomarkers in order to improve the review processes for such devices and how best to use them; another project, called EyeScore, tackles the issue of strokes through a different lens, by developing a technology that uses non-invasive scans of the retina to predict the recurrence of stroke in patients. Both processes utilize AI to analyze the data and draw out inferences and connections that may be beyond human observers.
Atashzar’s latest project uses AI to analyze data from COVID-19 patients to predict whether their condition will worsen in the next 24 hours. Thanks to his AI model, he needs only a few inputs — heart rate, body temperature, and oxygen saturation — that can easily be collected by a wearable device. This would give patients and their doctors advanced warning about whether they can be discharged from the hospital, or if they should be seeking further medical attention.
Imagine a world where AI seamlessly combines with medical robotics, opening up a universe of untapped potential. This blending amplifies the power of cognitive intelligence and self-directed action, presenting solutions to the complex healthcare challenges that often leave conventional methods struggling in areas with limited resources and particularly in catering to the needs of our aging society. Over the last decade, the ascent of AI has reshaped innovation across a multitude of sectors, with healthcare standing out prominently.”
Video games, design, and AI
If one were to draw a Venn diagram with a domain for games and a domain for computer science/ AI, the overlap would be huge. The Game Innovation Lab at NYU Tandon is mining that fertile ground by exploring the symbiotic relationship between video and digital games and AI. The implications go far beyond avatars and joysticks: the work done at the Lab could lead to profound innovations in automated systems able to run processes for everything from building HVAC systems to global shipping.
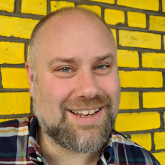
The Lab, under the leadership of Julian Togelius — Associate Professor of Computer Science and Engineering — is a hotbed of research into how games can help deep neural networks learn and how AI can help game developers automate expensive and time-consuming aspects of game development — and make new types of games possible.
The researchers’ work on how to use automatic generation of gameplay and levels to create more general game playing has put the Lab at the vortex of a virtuous cycle: smarter, more flexible machine learning systems that can play games can also help design them level by level, as well as personalize the playing experience for each player in real-time and automatically generate tutorial content.
AI is transforming gaming in a number of ways. The first is testing, which is a huge bottleneck for games in order to optimize performance, and we’ll likely see AI be able to make huge advances in testing games in the future. Another possibility is introducing AI non-player characters that can react to your inputs organically — though it may take a while to create games that can respond in that way. And one of the biggest goals is to design games that can react to your own inputs — your own playstyles and personality — and redesign themselves around the user’s preferences. That’s something I’ve been working towards for a long time.”
AI’s role in fundamental science
AI’s potential role for the public is obvious. With advancements in language and art applications, it’s clear how individuals can harness AI for personal use. But AI is already being utilized in the scientific field itself. Take Miguel Modestino, the Donald F. Othmer Associate Professor of Chemical Engineering in the Chemical and Biomolecular Engineering Department as well as the Director of the Sustainable Engineering Initiative at NYU Tandon.
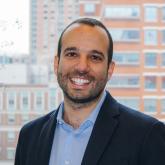
His research lies at the interface of multifunctional material development and electrochemical engineering. Electrochemical devices are ubiquitous to a broad range of energy conversion technologies and chemical processes. Their core components rely on complex materials that provide the required electrocatalytic activity and mass transport functionality.
His group has expertise in composite materials development, processing and characterization, and this expertise is used to improve and redefine electrochemical reactors with direct industrial applications. They are also using machine learning to enhance their own experiments, using data they collect to feed into their models and extrapolate findings that would otherwise require significant time and energy to manually acquire.
It is exciting to see so much enthusiasm about AI, but it is even more exciting to see how AI is expanding across all science and engineering disciplines. It was uncommon to find AI techniques in Chemical Engineering until the late 2010s, and it is remarkable to see how these tools have started to percolate throughout our field.
In our research, we have used machine learning tools to identify efficient sustainable chemical processes, to find optimal trade-offs between energy efficiency and productivity in hydrogen production systems, to substantially improve CO2 upconversion technologies, and even our student entrepreneurs have created commercial tools to make AI tools mainstream in the chemical industry. This is just a sample of AI applications that can help us accelerate the creation of sustainable engineering technologies, and step-by-step help us reach our decarbonization goals by 2050.”
Chip Chat: Designing microchips with the help of LLMs
Designing microchips can be a laborious process, requiring specialized skill in manufacturing language.
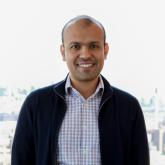
This year, researchers at NYU Tandon including Siddharth Garg, Institute Associate Professor of Electrical and Computer Engineering and a member of NYU WIRELESS, as well the Center for Cybersecurity have fabricated a microprocessing chip using plain English “conversations” with an AI model, a first-of-its-kind achievement that could lead to more democratized and faster chip development and allow individuals without specialized technical skills to design chips.
The team showed how hardware engineers “talked” in standard English with ChatGPT-4 — an LLM built to understand and generate human-like text type — to design a new type of microprocessor architecture. The LLM was able to turn that into Hardware Description Languages (HDLs), Verilog being one example, to create the actual circuit elements that allow the hardware to perform its tasks. The researchers then sent the designs to manufacture.
The process they developed could also eliminate the need for HDL fluency among chip designers, a relatively rare skill that represents a significant hurdle to people seeking those kinds of jobs.
Right now, I’m most excited about the use of machine learning to improve the efficiency of chip design to allow people to design better chips faster. There’s a huge opportunity to use large language models and reinforcement learning to enable this. I would like to see, in 10 years, tools that would allow even a biologist who has no prior expertise in chip design to be able to bring to life their new idea, in silicon.”