Yao Wang
,
Ph.D.
-
Vice Dean for Faculty Affairs
-
Professor
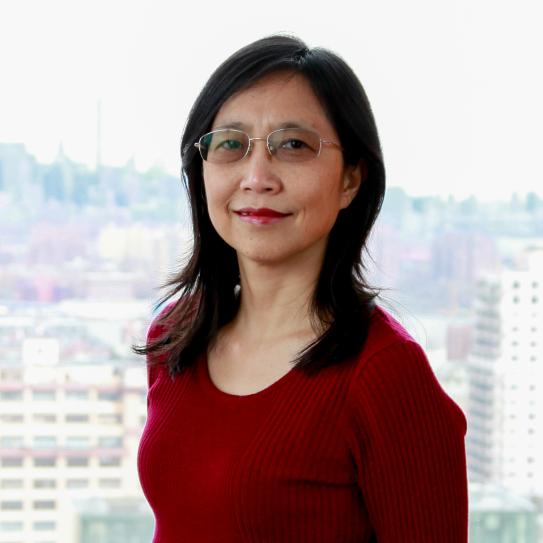
Yao Wang is a recognized expert in video coding, networked video applications, medical imaging, and computer vision.
She joined the faculty of Polytechnic Institute of New York University in 1990 as an assistant professor and became an associate professor in 1996 and a professor in 2000. She holds a joint appointment in Dept. Electrical and Computer Engineering and Dept. Biomedical Engineering, both in NYU Tandon School of Engineering, and has an Affiliated Appointment in the Radiology Department of NYU School of Medicine. She was the Speaker for the NYU Tandon Faculty from Sept. 2017 – Aug. 2019. She has been the Associate Dean for Faculty Affairs at NYU Tandon since June 2019.
Wang received her Bachelor of Science and Master of Science in Electronic Engineering from Tsinghua University in Beijing, China in 1983 and 1985, respectively, and a PhD in Electrical and Computer Engineering from the University of California at Santa Barbara in 1990.
She authored the well-known textbook Video Processing and Communications in addition to writing numerous book chapters and journal articles. She has also served as the associate editor of Institute of Electrical and Electronics Engineers’ (IEEE) Transactions on Multimedia and Transactions on Circuits and Systems for Video Technology.
Wang was elected a Fellow of the IEEE for her contributions to video processing and communications in 2004. She received the New York City Mayor’s Award for Excellence in Science and Technology, Young Investigator Category, in 2000; the IEEE Communications Society Leonard G. Abraham Prize Paper Award in the Field of Communications Systems in 2004; the IEEE Communications Society Multimedia Communication Technical Committee Best Paper Award in 2011. She received the Overseas Outstanding Young Investigator Award from the Natural Science Foundation of China in 2005; and was named the Yangtze River Lecture Scholar by the Ministry of Education in China in 2007. She was a keynote speaker at the 2010 International Packet Video Workshop, and a keynote speaker at the 2018 Picture Coding Symposium. She received the Distinguished Teacher Award of NYU Tandon School of Engineering in 2016. She has many past and on-going research projects funded by National Science Foundation and National Institutes of Health.
Her research is supported in part by NYU WIRELESS.
Tsinghua University, Beijing, P.R. China, 1983
Bachelor of Science, Electrical Engineering
Tsinghua University, Beijing, P.R. China, 1985
Master of Science, Electrical Engineering
University of California at Santa Barbara, 1990
Doctor of Philosophy, Electrical Engineering
AT&T Labs - Research, NJ
Part-time Consultant
From: January 1992 to December 2000
New York University (formerly Polytechnic Institute of NYU, Polytechnic University, Brooklyn Polytechnic Institute)
Professor
From: June 1990 to present
Authored/Edited Books
Yao Wang, Jörn Ostermann, and Ya-Qin Zhang , Video Processing and Communications , Prentice Hall, 2002 (Published September 2001) ISBN 0-13-017547-1.
Other Publications
Research News
Resource constrained mobile data analytics assisted by the wireless edge
The National Science Foundation grant for this research was obtained by Siddharth Garg and Elza Erkip, professors of electrical and computer engineering, and Yao Wang, professor of computer science and engineering and biomedical engineering. Wang and Erkip are also members of the NYU WIRELESS research center.
Increasing amounts of data are being collected on mobile and internet-of-things (IoT) devices. Users are interested in analyzing this data to extract actionable information for such purposes as identifying objects of interest from high-resolution mobile phone pictures. The state-of-the-art technique for such data analysis employs deep learning, which makes use of sophisticated software algorithms modeled on the functioning of the human brain. Deep learning algorithms are, however, too complex to run on small, battery constrained mobile devices. The alternative, i.e., transmitting data to the mobile base station where the deep learning algorithm can be executed on a powerful server, consumes too much bandwidth.
This project that this NSF funding will support seeks to devise new methods to compress data before transmission, thus reducing bandwidth costs while still allowing for the data to be analyzed at the base station. Departing from existing data compression methods optimized for reproducing the original images, the team will develop a means of using deep learning itself to compress the data in a fashion that only keeps the critical parts of data necessary for subsequent analysis. The resulting deep learning based compression algorithms will be simple enough to run on mobile devices while drastically reducing the amount of data that needs to be transmitted to mobile base stations for analysis, without significantly compromising the analysis performance.
The proposed research will provide greater capability and functionality to mobile device users, enable extended battery lifetimes and more efficient sharing of the wireless spectrum for analytics tasks. The project also envisions a multi-pronged effort aimed at outreach to communities of interest, educating and training the next generation of machine learning and wireless professionals at the K-12, undergraduate and graduate levels, and broadening participation of under-represented minority groups.
The project seeks to learn "analytics-aware" compression schemes from data, by training low-complexity deep neural networks (DNNs) for data compression that execute on mobile devices and achieve a range of transmission rate and analytics accuracy targets. As a first step, efficient DNN pruning techniques will be developed to minimize the DNN complexity, while maintaining the rate-accuracy efficiency for one or a collection of analytics tasks.
Next, to efficiently adapt to varying wireless channel conditions, the project will seek to design adaptive DNN architectures that can operate at variable transmission rates and computational complexities. For instance, when the wireless channel quality drops, the proposed compression scheme will be able to quickly reduce transmission rate in response while ensuring the same analytics accuracy, but at the cost of greater computational power on the mobile device.
Further, wireless channel allocation and scheduling policies that leverage the proposed adaptive DNN architectures will be developed to optimize the overall analytics accuracy at the server. The benefits of the proposed approach in terms of total battery life savings for the mobile device will be demonstrated using detailed simulation studies of various wireless protocols including those used for LTE (Long Term Evolution) and mmWave channels.
This award reflects NSF's statutory mission and has been deemed worthy of support through evaluation using the Foundation's intellectual merit and broader impacts review criteria.