NYU Tandon Researcher Uses Machine Learning to Help Early Detection and Treatment of Lymphedema
Machine learning is driving advances in areas like commerce, gaming, navigation, cybersecurity, and voice recognition. However, Yao Wang, a professor of electrical and computer engineering, is using it to deliver strong medicine to health care research, diagnostics, self-therapy, and ultimately, prognosis.
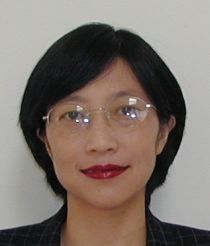
Yao Wang
Among the most promising of Wang’s projects, and one having immediate impact on patients’ lives, is a multi-year collaborative research program with Mei Fu, Associate Professor at NYU Rory Meyers College of Nursing. The project uses machine learning and video analysis to detect and manage a lymphatic-system malady common among breast cancer survivors
Every year more than 1.4 million women worldwide are diagnosed with breast cancer, and 90 percent of these patients are alive five years after diagnosis. But while major advances in surgical intervention, chemotherapy, and radiology have engendered higher survival rates, they can cause lymphedema, an incurable obstruction or disruption of the lymphatic system. Characterized by swelling of one or more limbs, with stiffness, pain, and other symptoms, lymphedema affects 40 percent of the 3.1 million breast cancer survivors in the U.S.
Partly because of the enigmatic nature of lymph circulation, whose movement more closely resembles the diffusion of water through a marsh than the flow of water down a river, lymphedema is hard to identify in early stages. Although it can manifest after a latency period of as many as 20 years, its sufferers often report symptoms long before practitioners can make a clear diagnosis — usually with pricey technology.
“There is no ‘gold standard’ for diagnosis. Even today we have difficulty diagnosing patients until a very late stage, when it can’t be reversed,” noted Fu.
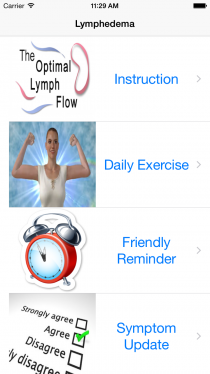
A screenshot of The Optimal Lymph-Flow (TOLF), a web-based, mobile health self-care system
To counteract a paucity of information about lymphedema symptoms and augment early diagnosis and interventional treatment, Fu, Wang and fellow researchers including Deborah M. Axelrod, M.D. and Amber Guth, M.D., professors of surgery at the NYU Langone Medical Center, developed The Optimal Lymph-Flow (TOLF) system. TOLF is a web-based, mobile health self-care system to assist breast cancer survivors in reducing the risk of lymphedema and optimizing in-home self-care. It combines clinical and diagnostic patient information for healthcare providers with self-care and symptom amelioration tools for the patient. With an emphasis on exercises that help improve lymph flow, TOLF comprises three major elements employed via smartphone, tablet or laptop:
- Collection of demographic and clinical information related to the survivor’s treatment history
- A tool allowing daily self-inventory of occurrence and level of distress of symptoms
- A collection of avatar-based lymphatic exercise videos that provide at-home symptom management for those who experience any of more than 20 lymphedema-related symptoms
The NYU research team conducted a test of TOLF tools with 355 breast cancer survivors and established TOLF’s overall usability with 30 other patient volunteers. Participants reported that TOLF self-care intervention relieved the pain and symptoms of lymphedema.
The National Cancer Institute (NCI) has recently awarded Fu and Wang a 3-year research award, as part of a joint program on Smart and Connected Health by National Science Foundation and National Institutes of Health. The grant will allow the researchers to develop a precise assessment of lymphedema risk from patient-reported symptoms through machine learning. Wang said the researchers will use both new patient data and the large amount of patient data already collected to develop and validate TOLF machine learning algorithms.
With the software to be developed by Wang’s team at NYU Tandon, patients using TOLF will soon be able to engage the mobile platform to learn with high accuracy whether they have, or are likely to contract, lymphedema based on their symptoms, body mass index, and other indicators.
With NCI support, the team is also developing a Microsoft Kinect-enhanced intervention training system that can track patients’ movements during their intervention exercises and provide instant audio-visual feedback about how certain movements should be improved to make the exercise more effective.
“Lymphedema exercise training has always been nurse-driven,” said Wang. “But with our system, the patient will be able to watch, practice, and receive instantaneous feedback.”
Wang says the system will be ready for usability testing by summer, 2018.
Axelrod said Fu and Wang’s work has changed perceptions about lymphedema, including among health professionals.
“Before [their] work, the belief was that lymphedema can only be confirmed if swelling can be detected by the naked eye, people are in terrible shape, and their life comes to a standstill. Now we understand that it is a continuum and can be controlled earlier, even for people with significant progression,” said Axelrod.
Professor Wang is also collaborating with:
- Yvonne W. Lui, M.D., a neuroradiologist at the NYU School of Medicine’s department of radiology and Center for Biomedical Imaging, to investigate the uses of machine learning for the detection and outcome prediction of mild traumatic brain injury (MTBI) using advanced MRI imaging features
- Jeffrey Ketterling from Riverside Research and Daniel Turnbull, a professor in the department of radiology and pathology at the NYU School of Medicine, in ultra-high frequency ultrasound imaging of mouse embryos for analyzing brain and body development and defects caused by mutations
- Professor Eric Brenner of the NYU Department of Biology, on using video analysis techniques to develop an educational app that students could use to visualize and quantify plant motion in time-lapse videos and compare motion patterns of normal vs mutant plants
- Professor Gabriel Rose of Mount Sinai St. Luke's Hospital and Mount Sinai Roosevelt Hospital, on using video analysis to detect and quantify B-lines in thorax ultrasound video, a marker of pulmonary congestion or edema
- Professor Jonathan Viventi of Duke University, on developing machine learning and video analysis algorithms to characterize high-resolution recordings of brain signals in patients with epilepsy