Fall 2019 Seminars
A complete listing
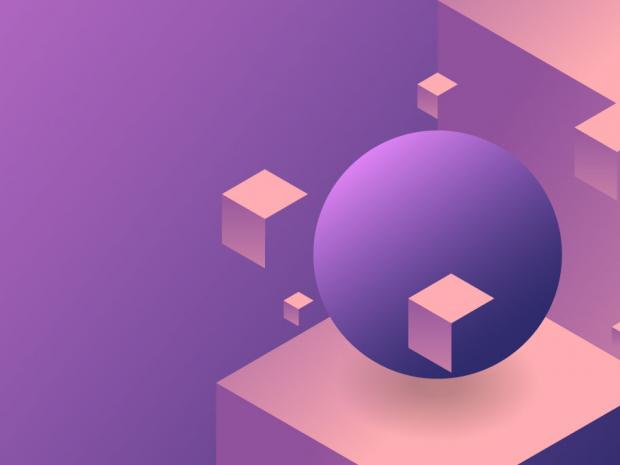
Date | Time | Speaker | From | Title | Location |
---|---|---|---|---|---|
Sep 11 | 3pm - 4pm | Seisuke Kyochi | The University of Kitakyushu, Japan | Sparsity/Low-rankness-aware proximal methods and their applications to signal processing | 370 Jay, Room: 824 |
Sep 12 | 11am - 12pm | Jie Wu | Temple University | Collaborative Mobile Charging: From Abstraction to Solution | 370 Jay, Room: 1013 |
Sep 19 | 11am - 12pm | Nitin Vaidya | McDevitt Chair, Department of Computer Science, Georgetown University | Security and and Privacy for Distributed Optimization and Learning | 370 Jay, Room: 824 |
Sep 20 | 11am - 12pm | Vladimir V. Vantsevich | The University of Alabama at Birmingham | Manned and Autonomous Vehicle Dynamics and Design: Towards Convergence and Transdisciplinary Technologies | 370 Jay, Room: 824 |
Sep 26 | 11am - 12pm | Guru Prasadh Venkataramani | George Washington University | Unmasking and Defeating Cache Timing Channels | 370 Jay, Room: 1013 |
Oct 7 | 11am - 12pm | Leon Bottou | Facebook AI Research |
AI Seminar Series: Learning Representations Using Causal Invariance |
370 Jay, 1201 Seminar Room |
Oct 8 | 11am - 12pm | José Moura | Carnegie Mellon University | Jack Keil Wolf Lecture Series: Understanding Behaviors in Instrumented Cities: Challenges and Opportunities | 370 Jay, Room: 825 |
Oct 10 (Cancelled) | 11am - 12pm | Serge Leef | DARPA | Toward Simulation and Optimization of Distributed Real-time Intelligent Vehicle Electronics | 370 Jay, Room: TBD |
Oct 17 | 11am - 12pm | Slawomir Stanczak | Heinrich Hertz Institute, Germany | Towards Robust Machine Learning in 5G Radio Access Networks | 370 Jay, Room: 913 |
Oct 24 | 11am - 12pm | Flavio du Pin Calmon | Harvard University | On representations and fairness: information-theoretic tools for machine learning | 370 Jay, Room: 913 |
Oct 31 | 11am - 12pm | Kurt Becker | New York University | Intellectual Property, Patents, and Entrepreneurship | 370 Jay, Room: 825 |
Nov 4 | 11am - 12pm | William Rosenbluth, Sophia Y. Wang; Peter J. Sullivan | Automotive Systems Analysis, Inc; Advanced Analysis Associates, Inc. | A Multi-Purpose Vehicle Test Instrument | 370 Jay, Room: 825 |
Nov 7 | 11am - 12pm | Luca Sanguinetti | University of Pisa | Cell-Free Massive MIMO: Make it Competitive! | 370 Jay, Room: 913 |
Nov 14 | 11am - 12pm | Francis Bach | INRIA, Paris France | AI Seminar Series: Distributed Machine Learning over Networks | 370 Jay, 1201 Seminar Room |
Nov 15 | 11am - 12pm | Elisabeth de Carvalho | Aalborg University, Denmark | Two trends in post massive MIMO: extra-large scale MIMO and holographic MIMO | 370 Jay, Room: 913 |
Nov 19 | 11am - 12pm | Farah Shamout | NYU Abu Dhabi | Machine Learning for the Detection of Clinical Deterioration | 370 Jay, Room: 1013 |
Nov 20 | 11am - 12pm | Hideaki Ishii | Tokyo Institute of Technology, Japan | Resilient Synchronization with Pulse-Based Communications under Robust Networks | 370 Jay, Room: 1013 |
Dec 6 | 11am - 12pm | Raiai Hadsell | DeepMind | AI Seminar Series: Challenges for Deep Reinforcement Learning in Complex Environments | 6MTC/Rogers Hall MakerEvent Space |
Dec 9 | 2pm - 3pm | Sandeep Chinchali | Stanford University | Distributed Perception and Learning Between Robots and the Cloud | 370 Jay, Room: 825 |
Sparsity/Low-rankness-aware proximal methods and their applications to signal processing
Speaker: Seisuke Kyochi, The University of Kitakyushu, Japan
Date: Sep 11
Abstract: Optimization with proximal method has shown to be effective and widely applied in many signal and image processing tasks. In particular, proximal operators for sparse and low-rank modeling have been proposed conventionally, since those characteristics often appear in signal processing. For example, in image recovery problem, the sparsity of gradient is often considered to estimate a latent image, since natural images are basically smooth. For more accurate signal and image processing, more sophisticated structured proximal operators are discussed recently. In this talk, some examples of sparsity/low-rankness-aware proximal methods from the author's group are introduced and shown their effectiveness in experiments.

About the Speaker: Seisuke Kyochi (S’08–M’10) received the B.S. degree in mathematics from Rikkyo University, Japan, in 2005, and the M.E. and Ph.D. degrees from Keio University, Japan, in 2007 and 2010, respectively. He was a Researcher with NTT Cyberspace Laboratories, from 2010 to 2012. In 2012, he joined The University of Kitakyushu and, since 2015, he has been an Associate Professor with the Faculty of Environmental Engineering. His research interests include the theory and design of wavelets/filter banks for efficient image processing applications, convex optimization in signal processing.
Collaborative Mobile Charging: From Abstraction to Solution
Date: Sep 12
Speaker: Jie Wu, Temple University
Abstract: Wireless energy charging using mobile vehicles has been a viable research topic recently in the area of wireless networks and mobile computing. This talk gives a short survey of recent research conducted in our research group in the area of collaborative mobile charging. In collaborative mobile charging, multiple mobile chargers work together to accomplish a given set of objectives. These objectives include charging sensors at different frequencies with a minimum number of mobile chargers and reaching the farthest sensor for a given set of mobile chargers, subject to various constraints, including speed and energy limits of mobile chargers. Testbed results are also given on the proposed mobile charging solutions. Through the process of problem formulation, solution construction, and future work extension for problems related to collaborative mobile charging and coverage, we present three principles for good practice in conducting research, that is, select a simple problem, find an elegant solution, and use imagination for extension.
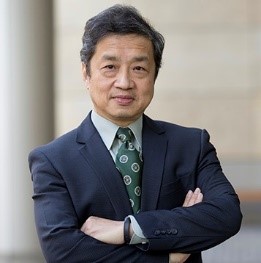
About the Speaker: Jie Wu is the Director of the Center for Networked Computing and Laura H. Carnell professor at Temple University. He also serves as the Director of International Affairs at College of Science and Technology. He served as Chair of Department of Computer and Information Sciences from the summer of 2009 to the summer of 2016 and Associate Vice Provost for International Affairs from the fall of 2015 to the summer of 2017. Prior to joining Temple University, he was a program director at the National Science Foundation and was a distinguished professor at Florida Atlantic University. His current research interests include mobile computing and wireless networks, routing protocols, cloud and green computing, network trust and security, and social network applications. Dr. Wu regularly publishes in scholarly journals, conference proceedings, and books. He serves on several editorial boards, including IEEE Transactions on Mobile Computing, IEEE Transactions on Service Computing, the Journal of Parallel and Distributed Computing, and the Journal of Computer Science and Technology. Dr. Wu was general co-chair for IEEE MASS 2006, IEEE IPDPS 2008, IEEE ICDCS 2013, ACM MobiHoc 2014, ICPP 2016, and IEEE CNS 2016, as well as program co-chair for IEEE INFOCOM 2011 and CCF CNCC 2013. He was an IEEE Computer Society Distinguished Visitor, ACM Distinguished Speaker, and chair for the IEEE Technical Committee on Distributed Processing (TCDP). Dr. Wu is a Fellow of the AAAS and a Fellow of the IEEE.
Security and and Privacy for Distributed Optimization and Learning
Speaker: Nitin Vaidya, McDevitt Chair, Department of Computer Science, Georgetown University
Date: Sep 19
Abstract: Consider a network of agents wherein each agent has a private cost function. In the context of distributed machine learning, the private cost function of an agent may represent the “loss function” corresponding to the agent’s local data. The objective here is to identify parameters that minimize the total cost over all the agents. In machine learning for classification, the cost function is designed such that minimizing the cost function should result model parameters that achieve higher accuracy of classification. Similar problems arise in the context of other applications as well, including swarm robotics.
Our work addresses privacy and security of distributed optimization with applications to machine learning. In privacy-preserving machine learning, the goal is to optimize the model parameters correctly while preserving the privacy of each agent’s local data. Privacy-preserving machine learning is becoming important due to the increasing reliance on user-generated data for machine learning. In security, the goal is to identify the model parameters correctly while tolerating adversarial agents that may be supplying incorrect information. When a large number of agents participate in distributed optimization, security compromise of some of the agents becomes increasingly likely. We constructively show that such privacy-preserving and secure algorithms for distributed optimization exist. The talk will provide intuition behind the design and correctness of the algorithms.

About the Speaker: Nitin Vaidya is the Robert L. McDevitt, K.S.G., K.C.H.S. and Catherine H. McDevitt L.C.H.S. Chair of Computer Science at Georgetown University. He received Ph.D. from the University of Massachusetts at Amherst. He previously served as a Professor and Associate Head in Electrical and Computer Engineering at the University of Illiniois at Urbana-Champaign. He has co-authored papers that received awards at several conferences, including 2015 SSS, 2007 ACM MobiHoc and 1998 ACM MobiCom. He is a fellow of the IEEE. He has served as the Chair of the Steering Committee for the ACM PODC conference, as the Editor-in-Chief for the IEEE Transactions on Mobile Computing, and as the Editor-in-Chief for ACM SIGMOBILE publication MC2R.
Manned and Autonomous Vehicle Dynamics and Design: Towards Convergence and Transdisciplinary Technologies
Speaker: Vladimir Vantsevich, University of Alabama at Birmingham
Date: Sep 20
Abstract: This seminar discusses four emerging research directions in manned and autonomous ground vehicle dynamics and vehicle design that provide necessary conditions for creating vehicle transformative technologies:
1. Vehicle operational properties and vehicle system design
2. Specific features of autonomous vehicle dynamics and design
3. Coupled and interactive dynamics of vehicle systems
4. Vehicle mechatronics and intelligent physical systems
5. Open architecture system design
6. Agile tire and vehicle dynamics
Wheel-power-distribution optimization and control ensured by mechanical and mechatronic driveline systems and by hybrid and electrical virtual/distributed driveline systems is presented as a key foundation of the above-listed research directions.
Several research and engineering projects accomplished for industry and government within the four research avenues are presented to illustrate the effectiveness of the proposed analytical methods and engineered systems, including a new hybrid-electric power transmitting unit, a new wheel rotational kinematics sensor, various limited slip and controllable differentials, and wheel power management control algorithms for fully electric vehicles. The seminar presents and discusses results on fundamental improvements of terrain mobility, vehicle energy efficiency, maneuver, stability of motion and safety of terrain vehicles.
About the Speaker: Dr. Vladimir V. Vantsevich joined the UAB Department of Mechanical Engineering as a Professor and the founding Director of Vehicle and Robotics Engineering Laboratory in 2012 (Secondary appointment is in the ECE Dept.). Prior to UAB he was a Professor and the founding Director of the M.S. in Mechatronic Systems Engineering Program and the Laboratory of Mechatronic Systems at Lawrence Technological University, Michigan. He was also a co-founder and served as Associate Director/Director of the LTU Automotive Engineering Institute. Before Lawrence Tech, Dr. Vantsevich was a Professor and the Head of Research and Design Group on Multi-Wheel Drive Vehicles that designed and developed mechatronic and mechanical driveline systems for various purpose vehicles in Belarus. He earned his Ph.D. and Sc.D. degrees from Belarusian National Technical University.
Prof. Vantsevich’s research area is mechanical and intelligent mechatronic multi-physics systems, system modeling, design and control. Applications include conventional and autonomous multi-wheel ground vehicles, and vehicle driveline systems. He developed a new research avenue – inverse ground vehicle dynamics, which is the basis of his optimization of power distribution among the drive wheels and control of vehicle mobility, energy/fuel consumption, traction performance, maneuver and stability of motion. Prof. Vantsevich’s recent research work is on coupled and interactive dynamics of vehicle systems, agile tire dynamics, virtual drivelines for electric and hybrid vehicles, and protection of vehicle sensors from cyber-threats.
He is author of 6 technical books and more than 170 research articles. Prof. Vantsevich delivered more than 160 science and engineering seminars, invited lectures and technical presentations to industry, academic institutions and professional societies across 17 countries. He is a registered inventor of the U.S.S.R. with 30 certified inventions and holds US invention disclosures. Prof. Vantsevich is the Founder and Editor of two book series: (i) Ground Vehicle Engineering at Taylor and Francis Group/CRC Press and (ii) Robotics Engineering at ASME Press. Dr. Vantsevich is Editor-in-Chief of the Journal of Terramechanics and Associate Editor of the SAE International Journal of Commercial Vehicles; he is a member of the Editorial Board of the International Journal of Vehicle Autonomous Systems.
Prof. Vantsevich was honored with Fellowship of the American Society of Mechanical Engineers. He is the Technical Committee Chair on Transportation Machinery at the International Federation for the Promotion of Mechanism and Machine Science. He served as the Chair of the ASME Vehicle Design Committee (VDC). Prof. Vantsevich is the founder of the ASME VDC William Milliken Invited Lecture Award. He is also a member of Association for Unmanned Vehicle Systems International, International Society for Terrain-Vehicle Systems, Society of Automotive Engineers, and International Association for Vehicle System Dynamics. His major awards include the ASME Design Engineering Division Thar Energy Design Award for significant contributions to the design research, innovation and product design in the areas related to energy engineering; Hyundai Distinguished Lecturer; Laureate of the National Academy of Sciences of Belarus for the Best Mechanical Engineering Book of the Year; Biographical article in the Encyclopedia of the Republic of Belarus; Certificate of an Honorary Citizen of Dallas, TX, and Medal of the U.S.S.R. Government for exemplary efficiency in work.
Unmasking and Defeating Cache Timing Channels
Speaker: Guru Prasadh Venkataramani, George Washington University
Date: Sep 26
Abstract: Caches present a large attack surface for adversaries to exploit and realize their timing channels. With advances in cache monitoring and protection techniques within individual caches, adversaries may resort to more sophisticated methods such as taking undue advantage of inter-cache hardware mechanisms in order to meet their malicious objectives. It becomes necessary to understand the hardware vulnerabilities and devise mitigation mechanisms to prevent adversaries from exploiting them.
In this talk, I will first briefly describe CC-Hunter our early work that detects cache timing channels using correlation patterns between cache misses. I will then go over some of our recent research that highlights how performance monitoring hardware can be leveraged for better security. Next, we will see how more sophisticated adversaries may target inter-cache hardware mechanisms such as cache coherence protocols.
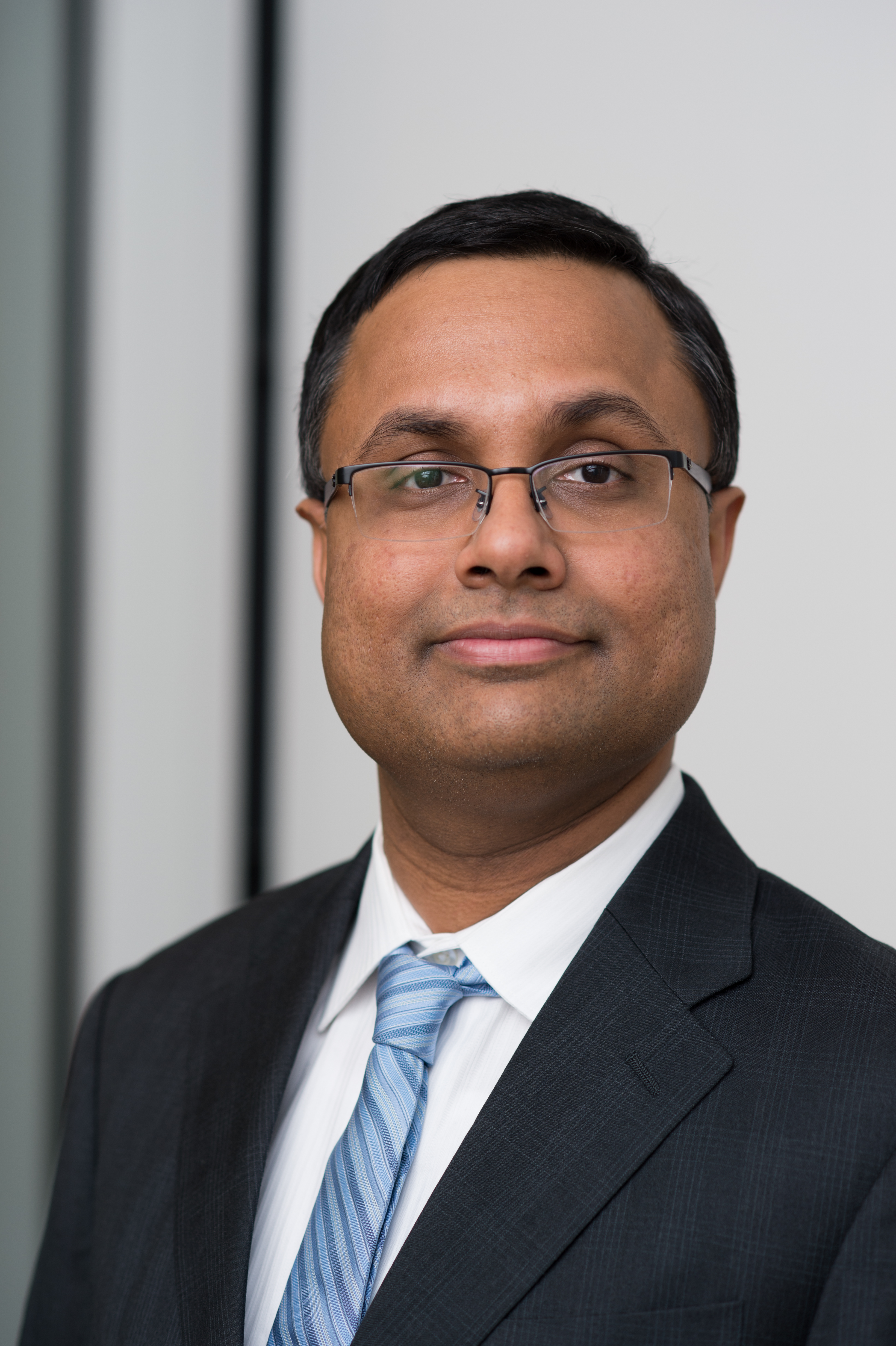
About the Speaker: Guru Prasadh Venkataramani is an associate professor of electrical and computer engineering at George Washington university in Washington, DC. He received his PhD from Georgia Tech, and his current research interests are in computer architecture and security. He is a recipient of NSF Career Award, ORAU Ralph E. Powe junior faculty enhancement award, best poster award in PACT'11, GWU Hegarty Award for faculty in innovation. His research has been funded by NSF, ONR and SRC. He served as a General Chair for IEEE HPCA 2019, and is a senior member of both IEEE and ACM.
Learning Representations Using Causal Invariance
Speaker: Léon Bottou, Facebook AI Research
Date: Oct 7
Abstract:Learning algorithms often capture spurious correlations present in the training data distribution instead of addressing the task of interest. Such spurious correlations occur because the data collection process is subject to uncontrolled confounding biases. Suppose however that we have access to multiple datasets exemplifying the same concept but whose distributions exhibit different biases. Can we learn something that is common across all these distributions, while ignoring the spurious ways in which they differ? This can be achieved by projecting the data into a representation space that satisfy a causal invariance criterion. This idea differs in important ways from previous work on statistical robustness or adversarial objectives. Similar to recent work on invariant feature selection, this is about discovering the actual mechanism underlying the data instead of modeling its superficial statistics.

About the Speaker: Léon Bottou received the Diplôme d’Ingénieur de l’École Polytechnique (X84) in 1987, the Magistère de Mathématiques Fondamentales et Appliquées et d’Informatique from École Normale Supérieure in 1988, and a Ph.D. in Computer Science from Université de Paris-Sud in 1991. His research career took him to AT&T Bell Laboratories, AT&T Labs Research, NEC Labs America and Microsoft. He joined Facebook AI Research in 2015. The long-term goal of Léon Bottou’s research is to understand and replicate human-level intelligence. Because this goal requires conceptual advances that cannot be anticipated, Leon’s research has followed many practical and theoretical turns: neural networks applications in the late 1980s, stochastic gradient learning algorithms and statistical properties of learning systems in the early 1990s, computer vision applications with structured outputs in the late 1990s, theory of large scale learning in the 2000s. During the last few years, Léon Bottou’s research aims to clarify the relation between learning and reasoning, with more and more focus on the many aspects of causation (inference, invariance, reasoning, affordance, and intuition.)
Understanding Behaviors in Instrumented Cities: Challenges and Opportunities
Speaker: José M F Moura, Carnegie Mellon University
Date: Oct 8
Abstract: Cities are increasingly instrumented and many of their activities progressively digitized. How and what can be done and can be gained from the datasets that are and will continue to be gathered. In this talk, we consider the challenges and opportunities to extract relevant behaviors from real time NYC webcam videos and offline pick-up and drop-off taxi records.
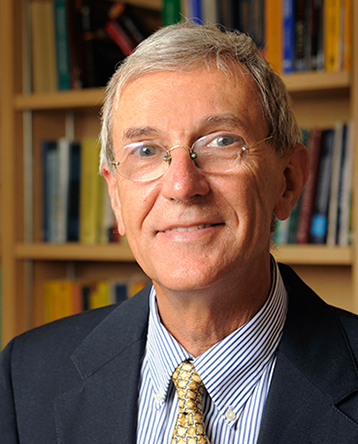
About the Speaker: José M. F. Moura is the Philip L. and Marsha Dowd University Professor at CMU. His areas of expertise are signal and image processing, graph signal processing, data science, and learning. Two of his patents (co-inventor Alek Kavcic) are used in over three billion hard disk drives in 60% of all computers sold in the last 13 years, and they were the subject of a $750million settlement between CMU and a chip semiconductor company. He serves as IEEE President and CEO and is a member of the US NAE.
Toward Simulation and Optimization of Distributed Real-time Intelligent Vehicle Electronics
Speaker: Serge Leef, DARPA
Date: Oct 10
Abstract: Distributed compute and control systems make numerous modern world application including automobiles and airplanes possible. These are complex, networked systems with hundreds to thousands computers collaborating to interact with human operators and the physical world. Design and verification of these systems is expensive, cumbersome and suboptimal as current approaches only cover a small number of operational scenarios. Problems identified in late stages of a project are difficult and very expensive to fix since the system is already built. The process and the outcome would be profoundly improved if the system could be simulated and optimized while still under development.

About the Speaker: Mr. Serge Leef joined DARPA in August 2018 as a program manager in the Microsystems Technology Office (MTO). His research interests include computer architecture, simulation, synthesis, semiconductor intellectual property (IP), cyber-physical modeling, distributed systems, secure design flows, and supply chain management. He is also interested in the facilitation of startup ecosystems and business aspects of technology.
Leef came to DARPA from Mentor, a Siemens Business where from 2010 until 2018 he was a Vice President of New Ventures, responsible for identifying and developing technology and business opportunities in systems-oriented markets. Additionally, from 1999 to 2018, he served as a division General Manager, responsible for defining strategies and building successful businesses around design automation products in the areas of hardware/software co-design, multi-physics simulation, IP integration, SoC optimization, design data management, automotive/aerospace networking, cloud-based electronic design, Internet of Things (IoT) infrastructure, and hardware cybersecurity.
Prior to joining Mentor, he was responsible for design automation at Silicon Graphics, where he and his team created revolutionary, high-speed simulation tools to enable the design of high-speed 3D graphics chips, which defined the state-of-the-art in visualization, imaging, gaming, and special effects for a decade. Prior to that, he managed a CAE/CAD organization at Microchip and developed functional and physical design and verification tools for major 8- and 16-bit microcontroller and microprocessor programs at Intel.
Leef received his Bachelor of Science degree in electrical engineering and Master of Science degree in computer science from Arizona State University. He has served on corporate, state, and academic advisory boards, delivered numerous public speeches, and holds two patents.
Towards Robust Machine Learning in 5G Radio Access Networks
Speaker: Slawomir Stanczak, Heinrich Hertz Institute, Germany
Date: Oct 17
Abstract:Wireless communications poses some fundamental challenges to machine learning (ML). Due to high mobility, wireless links exhibit ephemeral and highly dynamic nature; moreover, the links are corrupted by noise and are in general exposed to interference, while wireless resources (spectrum and energy) are scarce. All this may greatly limit the capacity of wireless networks. Since data (including measurement data) is not available at a single point but distributed among different locations, there is a strong need for distributed ML algorithms that efficiently use the scarce wireless resources in 5G networks. Moreover, the new ML methods need to provide robust results based on small data sets and under latency constraints.
In this talk, we will discuss why ML has the potential for substantially enhancing current radio access networks (RAN) solutions, and we will give an overview of promising techniques and algorithmic approaches for overcoming the above mentioned challenges in 5G networks. We argue in favor of hybrid-driven methods that combine purely data-driven ML approaches with classical model-based methods, while making use of the available expert knowledge. In particular, it is in general necessary to exploit structures in the wireless channel, transmission signals and various functions such as load maps. We will consider both kernel-based methods and deep neural networks. Meeting the latency requirements of 5G networks requires massive parallelization. We'll discuss how to parallelize certain radio access network (RAN) functions to GPU architectures to achieve orders-of-magnitude acceleration.

About the Speaker: Slawomir Stanczak studied electrical engineering with specialization in control theory at the Wroclaw University of Technology and at the Technical University of Berlin (TU Berlin). He received the Dipl.-Ing. degree in 1998 and the Dr.-Ing. degree (summa cum laude) in electrical engineering in 2003, both from TU Berlin; the Habilitation degree (venialegendi) followed in 2006. Since 2015, he has been a Full Professor for network information theory with TU Berlin and the head of the Wireless Communications and Networks department. Prof. Stanczak is a co-author of two books and more than 200 peer-reviewed journal articles and conference papers in the area of information theory, wireless communications, signal processing and machine learning.He was an Associate Editor of the IEEE Transactions on Signal Processing between 2012 and 2015. Since February 2018 Prof. Stanczak has been the chairman of the ITU-T focus group on machine learning for future networks including 5G.
On representations and fairness: information-theoretic tools for machine learning
Speaker: Flavio du Pin Calmon, Harvard
Date: Oct 24
Abstract: Information theory can shed light on the algorithm-independent limits of learning from data and serve as a design driver for new machine learning algorithms. In this talk, we discuss a set of information-theoretic tools that can be used to (i) help understand fairness and discrimination in machine learning and (ii) characterize data representations learned by complex learning models. On the fairness side, we explore how local perturbations of distributions can help both identify proxy features for discrimination, and how a formulation inspired by information projection can be applied to repair models for bias. On the representation learning side, we explore a theoretical tool called principal inertia components (PICs), which enjoy a long history in the statistics and information theory literature. We use the PICs to scale-up a multivariate statistical tool called correspondence analysis (CA) using neural networks, enabling data dependencies to be visualized and interpreted at a large scale. We illustrate these techniques in both synthetic and real-world datasets, and discuss future research directions.
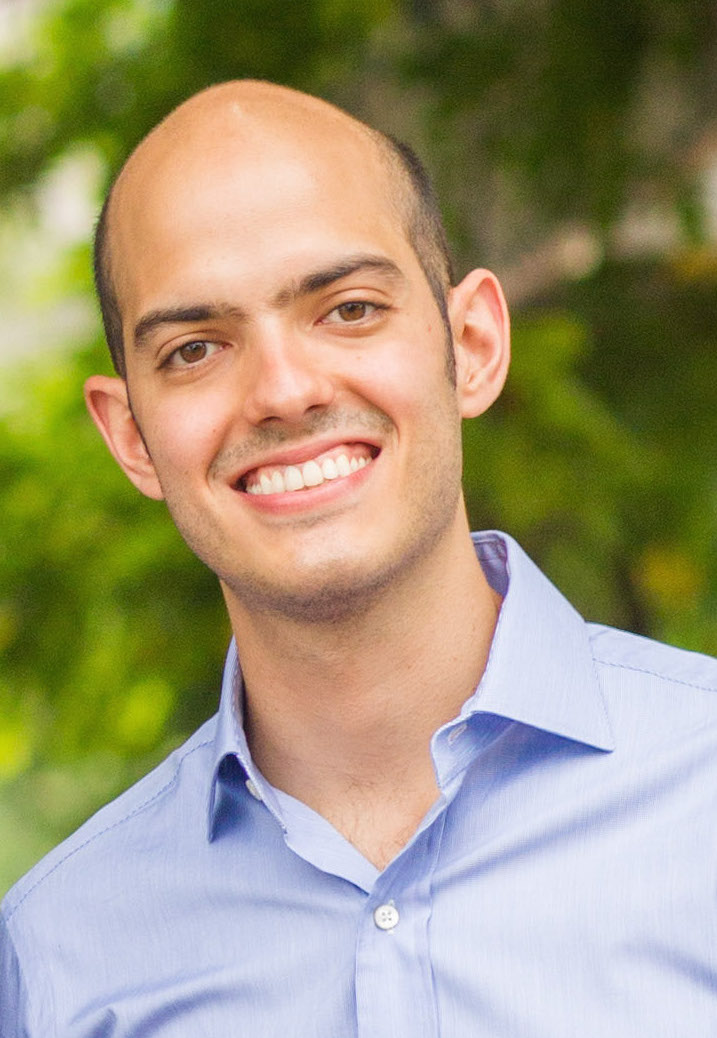
About the Speaker: Flavio P. Calmon is an Assistant Professor of Electrical Engineering at Harvard's John A. Paulson School of Engineering and Applied Sciences. Before joining Harvard, he was the inaugural data science for social good post-doctoral fellow at IBM Research in Yorktown Heights, New York. He received his Ph.D. in Electrical Engineering and Computer Science at MIT. His main research interests are information theory, inference, and statistics, with applications to fairness, privacy, machine learning, and communications engineering. Prof. Calmon has received the NSF CAREER award, the Google Research Faculty Award, the IBM Open Collaborative Research Award, and Harvard's Lemann Brazil Research Fund Award.
Intellectual Property, Patents, and Entrepreneurship
Speaker: Kurt H Becker, New York University
Date: Oct 31
Abstract: The talk will address issues about generating and protecting intellectual property, patents, their commercialization and give an overview of entrepreneurial resources available to faculty and students at NYU Tandon and NYU.
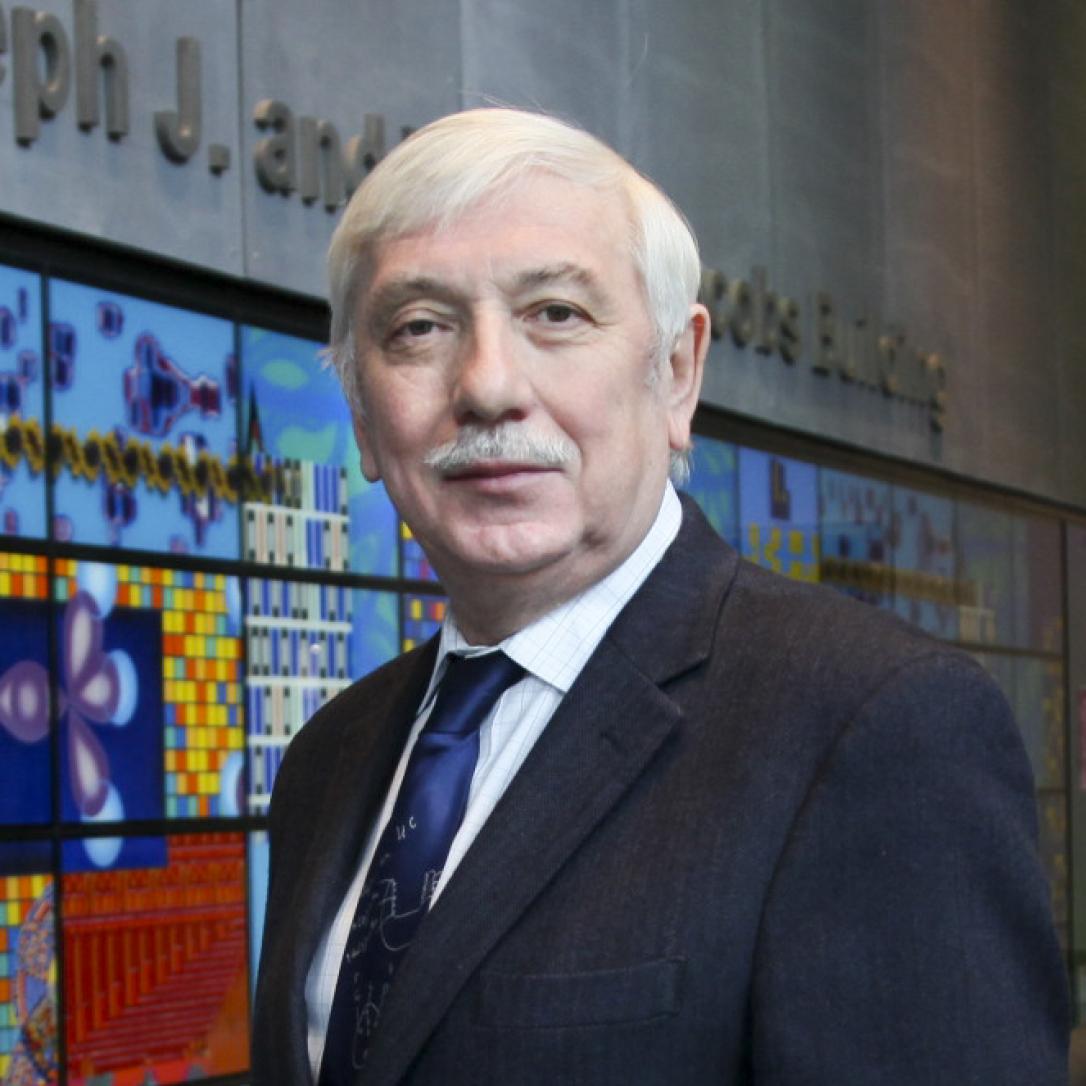
About the Speaker: Kurt Becker is an international expert in the areas of the experimental and theoretical study of electron-driven processes in plasmas. He was part of a group of pioneering scientists who determined the ionization cross sections for atoms and molecules, which are critical to understanding the charge carrier formation in plasmas.
He is also well known for microplasmas or plasmas generated and sustained at atmospheric pressure. These plasmas are very unstable and prone to destructive arching. By confining these plasmas to less than 1mm, they become more stable. The plasmas provide a fertile environment for basic plasma physics and for developing new plasma technologies. Additional areas of interest include atomic, molecular and chemical physics, the development of new experimental techniques and processes, technology transfer, and IP commercialization.
Becker joined the Polytechnic Institute of NYU faculty in his current capacity in 2007. Prior to joining NYU-Poly, he was associate director of the Center for Environmental Systems at Stevens Institute of Technology from 2003-2007 and department head from 2000 to 2007.
He earned a Diplom in Physik (MS) and Dr. rer. Nat. Universitat des Saarlandes in 1978 and 1981, respectively. He is the recipient of the Dr. Eduard-Martin Prize for Excellence in Research, American Physical Society Fellow, Thomas Alva Edison Patent Award (with Erich Kunhardt), and an honorary professorship from the Leopold Franzens Universitat.
A prolific author, Becker has written over 200 articles in refereed journals and books, 375 conference presentations and abstracts and seven patents on the stabilization of atmospheric pressure plasmas and their applications. In addition, he has served on the editorial boards of numerous journals and was the invited presenter at national and international conferences.eived the NSF CAREER award, the Google Research Faculty Award, the IBM Open Collaborative Research Award, and Harvard's Lemann Brazil Research Fund Award.
A Multi-Purpose Vehicle Test Instrument
Speaker: William Rosenbluth, Sophia Y. Wang, Automotive Systems Analysis, Inc.; Peter J. Sullivan, Advanced Analysis Associates, Inc.
Date: Nov 4
Abstract: In the course of many vehicle road and reconstruction tests, the authors found that no single diagnostic or test suite combined their needs for high intuitive graphic visibility while presenting simultaneous data from both CAN networks and analog sensors. This presentation includes five examples of forensic investigation cases requiring such an instrument, and describes how they achieved that new test instrument by using two custom Python scripts to combine the desired parts of existing and cost effective test interfaces to create a new test instrument. The resulting new test instrument implements a test data acquisition and recording package that, in operational road tests, accident reconstructions, and laboratory component tests, acquires and records CAN network and analog sensor data while simultaneously presenting a highly readable and scaled graphical interface to the test operator. The new test instrument was designed to utilize any of several very cost effective USB or PCI CAN and analog interfaces and handles both 11 and 29 bit ID CAN data. It also, in addition to logging raw CAN and scaled parameter data, incorporates a playback feature to review in real time, prior recorded CAN logs from several source formats including Bosch Busmaster®, Intrepid Control Systems®, and Kvaser® as well as its own native format.

About the Speakers: William Rosenbluth has been President and Principal Engineer for Automotive Systems Analysis (ASA), Reston, VA, for 33 years. He has 58 years of experience with complex electro-mechanical, electronic and computer components and systems. He was employed by the IBM Corporation for 21 years, until forming ASA. At ASA, he specializes in the analysis and diagnosis of computer-related vehicle control systems and in the retrieval and analysis of electronic crash-event data in accident vehicles (black box data). His has authored two books, 'Investigation and Interpretation of Black Box Data in Automobiles' (2001) and 'Black Box Data from Accident Vehicles' (2009). He holds a BEE (‘61) and an MSEE (‘65) from the Polytechnic Institute of Brooklyn.

Peter J Sullivan has been President and Principal Engineer for Advanced Analysis Associates, Inc, for the past 26years. His performs Forensic Expert Witness investigations for clients throughout the US, and he testifies in State and Federal Courts throughout the US. In his investigative capacity, he performs data downloads and imaging of Electronic Control Modules and ESI, including analysis and application to elements of accident reconstruction, validation, and electronic testing, on almost all makes and models of vehicles, equipment, and hand-held electronics. He holds a Bachelor of Science in Chemistry and Physics (‘84) from Texas State University.

Sophia Wang is a Senior at Thomas Jefferson High School for Science and Technology. She is proficient in Python, Java, Android Studio, JavaScript, HTML, CSS, SQL and Arduino languages. During the Summer of 2018 she assisted with Computer Science instruction of rising sophomores and juniors. During the Summer of 2019 she worked with Bill Rosenbluth at Automotive Systems Analysis, Inc, to develop the new Multi-Purpose Vehicle Test Instrument. In the course of this assignment, she was exposed to real world electrical and mechanical engineering methods and fabrication activities, in which she eagerly participated.
Cell-Free Massive MIMO: Make it Competitive!
Speaker: Luca Sanguinetti, University of Pisa
Date: Nov 7
Abstract: Cell-free Massive MIMO is considered as a promising technology for satisfying the increasing number of users and high rate expectations in beyond-5G networks. The key idea is to let many distributed access points (APs) communicate with all users in the network, possibly by using joint coherent signal processing. The aim of this talk is to provide a comprehensive analysis of this technology under different degrees of cooperation among the APs. Particularly, the uplink spectral efficiencies of four different cell-free implementations are analyzed, with spatially correlated fading and arbitrary linear processing. It turns out that it is possible to outperform conventional Cellular Massive MIMO and small cell networks by a wide margin, but only using global or local minimum mean-square error (MMSE) combining. This is in sharp contrast to the existing literature, which advocates for maximum-ratio combining. Also, we show that a centralized implementation with optimal MMSE processing not only maximizes the SE but largely reduces the fronthaul signaling compared to the standard distributed approach. This makes it the preferred way to operate Cell-free Massive MIMO networks. Non-linear decoding is also investigated and shown to bring negligible improvements.

About the Speakers: Luca Sanguinetti is an Associate Professor at the University of Pisa, Italy. He received the Laurea degree (cum laude) in telecommunications engineering and his Ph.D. degree in information engineering from the University of Pisa in 2002 and 2005, respectively. Since 2005, he has been with the Department of Information Engineering . In 2004, he was a visiting Ph.D. Student with the German Aerospace Center, Oberpfaffenhofen, Germany. From 2007 to 2008, he was a Post-Doctoral Associate with the Department Electrical Engineering, Princeton. In 2010, he was selected for a Research Assistantship with the Technische Universitat Munchen. From 2013 to 2017, he was with the Large Systems and Networks Group, CentraleSupélec, Gif-sur-Yvette, France.
Professor Sanguinetti served as the Exhibit Chair of the 2014 IEEE International Conference on Acoustics, Speech, and Signal Processing and as the General Co-Chair of the 2016 Tyrrhenian Workshop on 5G & Beyond. He also served as a Technical Co-Chair of European Wireless 2018, and as Special Session Chair of ISWCS18. He is the general chair of SPAWC 2021 (Lucca, June 2021), and also the executive co-chair of ICC 2023 (Rome, May 2023).
He received the 2018 Marconi Prize Paper Award in Wireless Communications and co-authored a paper that received the young best paper award from the ComSoc/VTS Italy Section. Professor Sanguinetti was a co-recipient of two best paper awards: the IEEE Wireless Communications and Networking Conference (WCNC) 2013 and the IEEE WCNC 2014. He was also a recipient of the FP7Marie Curie IEF 2013 DENSE4GREEN (Dense deployments for green cellular networks).
AI Seminar Series: Distributed Machine Learning over Networks
Speaker: Francis Bach, INRIA, Paris France
Date: Nov 14
Abstract: The past decade has seen a remarkable increase in the level of performance of computer vision techniques, including with the introduction of effective deep learning techniques. Much of this progress is in the form of rapidly increasing performance on standard, curated datasets. However, translating these results into operational vision systems for robotics applications remains a formidable challenge. This talk with explore some of the fundamental questions at the boundary between computer vision and robotics that need to be addressed. This includes introspection/self-awareness of performance, anytime algorithms for computer vision, multi-hypothesis generation, rapid learning and adaptation. The discussion will be illustrated by examples from autonomous air and ground robots.
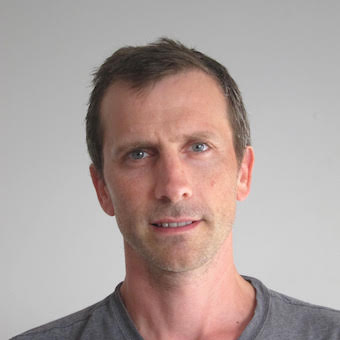
About the Speakers: Francis Bach is a researcher at Inria, leading since 2011 the machine learning team which is part of the Computer Science department at Ecole Normale Superieure. He graduated from Ecole Polytechnique in 1997 and completed his Ph.D. in Computer Science at U.C. Berkeley in 2005, working with Professor Michael Jordan. He spent two years in the Mathematical Morphology group at Ecole des Mines de Paris, then he joined the computer vision project-team at Inria/Ecole Normale Superieure from 2007 to 2010. Francis Bach is primarily interested in machine learning, and especially in sparse methods, kernel-based learning, large-scale optimization, computer vision and signal processing. He obtained in 2009 a Starting Grant and in 2016 a Consolidator Grant from the European Research Council, and received the Inria young researcher prize in 2012, the ICML test-of-time award in 2014, as well as the Lagrange prize in continuous optimization in 2018, and the Jean-Jacques Moreau prize in 2019. In 2015, he was program co-chair of the International Conference in Machine learning (ICML), and general chair in 2018; he is now co-editor-in-chief of the Journal of Machine Learning Research.
Two trends in post massive MIMO: extra-large scale MIMO and holographic MIMO
Speaker: Elisabeth de Carvalho, Aalborg University, Denmark
Date: Nov 15
Abstract: We describe two current trends in the post massive MIMO research: extra-large scale MIMO and holographic MIMO. Both are extensions of massive MIMO and call for a new generation of base stations equipped with an even larger number of antennas. In extra large scale MIMO, the antenna array at the access point has a very large dimension. In holographic MIMO, the arrays are not necessarily large and are a collection of tiny closely-spaced antenna elements. The prime focus of this presentation is to describe a communication model for holographic MIMO. The proximity of the antenna elements results in mutual coupling. Therefore, it is important to account for the mutual coupling in the communication model but also in the transceiver design. For a single user communication, the optimal transmitter consists of the inversion of the mutual coupling matrix followed by a filter matched to the channel vector. We show that the potential gains of holographic MIMO are superior to conventional arrays with antenna spacing of half a wavelength.

About the Speakers: Elisabeth de Carvalho received the Ph.D. degree in electrical engineering from Telecom ParisTech, France. After her Ph.D. degree, she was a Post-Doctoral Fellow at Stanford University, Stanford, CA, USA, and then worked in industry in the field of DSL and wireless LAN. She is currently a Professor with Aalborg University, where she has led many research projects in wireless communications. She has co-authored the book ``A Practical Guide to MIMO Radio Channel''. Her main expertise is in the field of signal processing with emphasis on MIMO communications. She is a member of IEEE signal processing society technical committee ``Signal Processing for Communications and Networking'' (SPCOM) and vice chair of the IEEE COMSOC Emerging Technology Initiative on Machine Learning for Communications. She is the coordinator of the H2020 Innovative Training Network WindMill, a European network of early stage researchers focusing on the application of machine learning for wireless communications.
Machine Learning for the Detection of Clinical Deterioration
Speaker: Farah Shamout, NYU Abu Dhabi
Date: Nov 19
Abstract: Clinical deterioration often goes undetected on hospital wards. Assessment of clinical deterioration preceding specific adverse outcomes, such as unplanned ICU admission, cardiac arrest, or mortality, is traditionally done using Early Warning Score (EWS) systems. Those scores consider a limited set of physiological variables, do not account for temporal changes leading to adverse events, are heuristic in nature, or do not generalize well for unseen patient cohorts. Increased access to Electronic Health Records (EHR) motivates the development of more robust and flexible machine learning techniques to improve predictions of clinical deterioration. This talk will focus on the opportunities and challenges of modelling EHR data and the results of the work in which we develop EWS systems using deep learning. It will also present ongoing projects at the MLHR Lab.
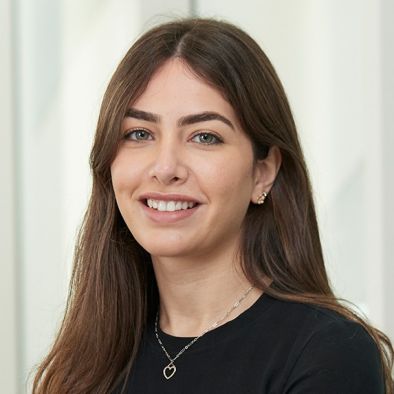
About the Speakers: Farah Shamout is an Assistant Professor & Emerging Scholar at NYU Abu Dhabi in Computer Engineering, who recently set up the Machine Learning in Healthcare Research (MLHR) Laboratory. Prior to joining NYU Abu Dhabi, Farah completed her doctoral studies in Engineering Science at the University of Oxford as a Rhodes Scholar. She received her Bachelor of Science cum laude in Computer Engineering from New York University Abu Dhabi. Her research focuses on designing robust, interpretable, and generalisable machine learning models to inform decision-making in healthcare.
Resilient Synchronization with Pulse-Based Communications under Robust Networks
Speaker: Hideaki Ishii, Tokyo Institute of Technology, Japan
Date: Nov 20
Abstract: In this talk, we consider a synchronization problem for wireless sensor networks using pulse-based communication in the presence of faulty or malicious nodes which try to disturb the normal nodes. In particular, we develop a resilient distributed algorithm for achieving synchronization among the normal nodes by assuming worst-case attacks. The algorithm functions even under a sparse network structure, which is important for scalability in this type of application. The approach is based on the socalled mean subsequence reduced type algorithms from the area of fault-tolerant distributed algorithms in computer science. We characterize a detection method for finding malicious nodes that transmit pulses frequently. Then, we show that as long as the malicious ones send pulses in a way they can stay undetected, the normal nodes can reach synchronization by ignoring suspicious pulses. We further discuss related problem where an interesting interplay between systems control and computer science has been recently taking place.
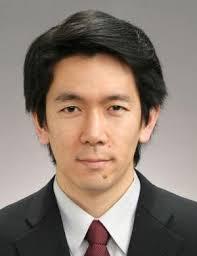
About the Speakers: Hideaki Ishii received the M.Eng. degree in applied systems science from Kyoto University in 1998, and the Ph.D. degree in electrical and computer engineering from the University of Toronto in 2002. He was a Postdoctoral Research Associate with the Coordinated Science Laboratory at the University of Illinois at Urbana-Champaign from 2001 to 2004, and a Research Associate with the Department of Information Physics and Computing, The University of Tokyo from 2004 to 2007. Currently, he is an Associate Professor in the Department of Computer Science, Tokyo Institute of Technology. He was a Humboldt Research Fellow at the University of Stuttgart in 2014-2015. He has also held visiting positions at the Politecnico di Torino and CNR-IEIIT, Technical University of Berlin, City University of Hong Kong, and Zhejiang University. His research interests are in networked control systems, multi-agent systems, hybrid systems, cyber security of
power systems, and probabilistic algorithms.
Dr. Ishii has served as an Associate Editor for the IEEE Control Systems Letters, and Mathematics of Control, Signals, and Systems, and previously for Automatica, the IEEE Transactions on Automatic Control, and the IEEE Transactions on Control of Network Systems. He is the Chair of the IFAC Coordinating Committee on Systems and Signals since 2017 and was the Chair of the IFAC Technical Committee on Networked Systems from 2011 to 2017. He received the IEEE Control Systems Magazine Outstanding Paper Award in 2015.
AI Seminar Series: Challenges for Deep Reinforcement Learning in Complex Environments
Speaker: Raia Hadsell, DeepMind
Date: Dec 6
Abstract: Deep reinforcement learning has rapidly grown as a research field with far-reaching potential for artificial intelligence. Games and simple physical simulations have been used as the main benchmark domains for many fundamental developments. As the field matures, it is important to develop more sophisticated learning systems with the aim of solving more complex real-world tasks, but problems like catastrophic forgetting remain critical, and important capabilities such as skill composition through curriculum learning remain unsolved. Continual learning is an important challenge for reinforcement learning, because RL agents are trained sequentially, in interactive environments, and are especially vulnerable to the phenomena of catastrophic forgetting and catastrophic interference. This talk will cover some of the challenges that exist for learning from interactions in more complex, constrained, and real-world settings, and some promising new approaches that have emerged.

About the Speakers: Raia Hadsell is the head of Robotics research at DeepMind. Her early research was in the use of Siamese networks for learning neural embeddings, an approach which now commonly used for representation learning. After completing a PhD which featured a self-supervised deep learning vision system for a mobile robot, her research continued at Carnegie Mellon’s Robotics Institute and SRI International, and in early 2014 she joined DeepMind in London to study artificial general intelligence. Dr. Hadsell’s current research focuses on the challenge of continual learning for AI agents and robots, and she has proposed neural approaches such as policy distillation, progressive nets, and elastic weight consolidation to solve the problem of catastrophic forgetting. Dr. Hadsell is on the executive boards of ICLR, WiML, and CoRL, and is a fellow of the European Lab on Learning Systems (ELLIS).
Distributed Perception and Learning Between Robots and the Cloud
Speaker: Sandeep Chinchali, Stanford University
Date: Dec 9
Abstract: Today’s robotic fleets are increasingly facing two coupled challenges.
First, they are measuring growing volumes of high-bitrate video and LIDAR sensory streams, which, second, often leads them to use increasingly compute-intensive models, such as deep neural networks
(DNNs), for downstream perception or control. To cope with these intertwined challenges, compute and storage-limited robots, such as low-power drones, can offload data to central servers (or “the cloud”), for more accurate real-time perception as well as offline model learning. However, cloud processing of large robotic sensory streams introduces acute systems bottlenecks ranging from network delay for real-time inference, to cloud storage, human annotation, and cloud-computing costs for offline model learning.
In this talk, I will present learning-based approaches for robots to improve model performance with cloud computing, but with minimal systems cost. For real-time inference, I will present a deep reinforcement learning based offloader that decides when a robot should exploit low-latency, on-board computation, or, when highly uncertain, query a more accurate cloud model. Then, for continual learning, I will present an intelligent, on-robot sampler that mines real-time sensory streams for valuable training examples to send to the cloud for model re-training and specialization. Using insights from months of field data and experiments on state-of-the-art embedded deep learning hardware, I
will show how simple learning algorithms can allow robots to significantly transcend their on-board sensing performance, but with limited cloud communication cost.

About the Speakers: Sandeep Chinchali is a final-year Computer Science PhD candidate at Stanford, advised by Marco Pavone and Sachin Katti. Previously, he was an early engineer at Uhana, a Stanford startup working on data-driven optimization of cellular networks, now acquired by VMWare. His research on cloud robotics and data-driven control of wireless systems has led to successful proof-of-concept trials with major cellular network operators, and was a finalist for best student paper at Robotics: Science and Systems 2019. Prior to Stanford, he graduated from Caltech, where he worked on applications of formal methods to robotics.