NYU Tandon robotics teams present the future of robotics work at IROS 2022
The robotics labs at NYU Tandon are improving teamwork among autonomous devices, developing new advanced neurorobotics, and more
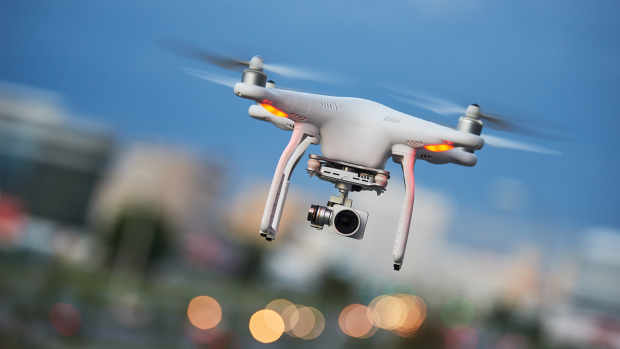
NYU Tandon Robotics researchers will present a wide-ranging array of work at the upcoming 2022 IEEE/RSJ International Conference on Intelligent Robots and Systems (IROS 2022). The conference, which runs from October 23rd to October 27th, is one of the most important and prominent conferences on robotics, and represents a wide swath of the academic and research community. The conference will be held in-person in Kyoto after two years away due to the COVID-19 pandemic.
Tandon’s robotics researchers in NYU Tandon’s departments of electrical and computer engineering, biomedical engineering, mechanical and aerospace engineering and civil and urban engineering, have seven accepted papers and several workshops at the conference, which brings together experts to discuss the most cutting-edge research in robotics and autonomous systems.
The research presented builds on a number of advances presented at previous conferences. NYU Tandon’s varied robotics labs are developing key technology behind autonomous vehicles, biomedical devices, and fine-tuned control. This continues their innovation in robotics research and teaching at all levels — along with unprecedented collaboration across disciplines, schools, and geographies — leading to significant advances in healthcare, transportation, logistics, and more.
Hand Gesture Recognition via Transient sEMG Using Transfer Learning of Dilated Efficient CapsNet: Towards Generalization for Neurorobotics
From the lab of S. Farokh Atashzar
There is a new focus on using deep neural network to decode central and peripheral activations of the human nervous system boost the spatiotemporal resolution of neural interfaces used in human-centered robotic systems, such as prosthetics, and exoskeletons. In this letter, Atashzar is suggesting “Dilated Efficient CapsNet” to improve the predictive performance of algorithms when there isn't enough data to use traditional neural networks, potentially improving the may that medical robotics are trained and operated.
Introducing Force Feedback in Model Predictive Control
From the lab of Ludovic Righetti
Much like humans, robots need a sense of ‘touch’ in order to best accomplish many tasks. Model predictive control (MPC) is a powerful technique to generate robust and adaptable movements, yet it rarely makes use of explicit force sensing, hence limiting its applicability.
In this paper, the researchers propose a novel paradigm to incorporate effort measurements into a predictive controller, hence allowing to control them by direct measurement feedback. They demonstrate why the classical optimal control formulation, based on position and velocity state feedback, cannot handle direct feedback on force information.They also propose to augment the classical formulations with a model of the robot actuation, which naturally allows to generate online trajectories that adapt to sensed position, velocity and torques.
Deep Augmentation for Electrode Shift Compensation in Transient High-density sEMG: Towards Application in Neurorobotics
From the lab of S. Farokh Atashzar
High-density surface electromyography (HD-sEMG) has been shown significant potential for decoding upper-limb motor intention, a necessary prerequisite for control of bionic limbs and neurorobots. For the first time, the researchers implemented gesture prediction on the transient phase of HD-sEMG data while robustifying the human-machine interface decoder to electrode shift. They were able to create a resilient algorithmic solution to recover the performance which would be significantly degraded without the proposed system by small electrode misplacement and displacement.
Physics-Inspired Temporal Learning of Quadrotor Dynamics for Accurate Model Predictive Trajectory Tracking
From the lab of Giuseppe Loianno
Accurately modeling quadrotor's system dynamics is critical for guaranteeing agile, safe, and stable navigation. The model needs to capture the system behavior in multiple flight regimes and operating conditions, including those producing highly nonlinear effects such as aerodynamic forces and torques, rotor interactions, or possible system configuration modifications.
In this paper, the researchers present a novel Physics-Inspired Temporal Convolutional Network (PI-TCN) approach to learning quadrotor's system dynamics purely from robot experience. Experimental results demonstrate that their approach accurately extracts the structure of the quadrotor's dynamics from data, capturing effects that would remain hidden to classical approaches.
Continuous Safety Control of Mobile Robots in Cluttered Environments
From the lab of Zhong-Ping Jiang
The lab’s letter studies the safety control problem for mobile robots working in cluttered environments. A compact set is employed to represent the obstacles, and a direction-distance function is used to describe the obstacle-measurement model.
The researchers modified the quadratic programming (QP) approach for continuous safety control of integrator-modeled mobile robots. In particular, they propose a refinement of the Moreau-Yosida method to regularize the measurement model while retaining feasibility and safety. The second contribution is the development of a new feasible set shaping technique with a positive basis for a QP-based continuous safety controller.
Vision-based Relative Detection and Tracking for Teams of Micro Aerial Vehicles
From the lab of Giuseppe Loianno
In this paper, the researchers address the vision-based detection and tracking problems of multiple aerial vehicles using a single camera and Inertial Measurement Unit (IMU) as well as the corresponding perception consensus problem (i.e., uniqueness and identical IDs across all observing agents). Through the analysis of several methods to address this issue, the researchers provide useful insights about the most appropriate design choice for any given task.
Adaptive Wave Reconstruction Through Regulated-BMFLC for Transparency-Enhanced Telerobotics Over Delayed Networks
From the lab of S. Farokh Atashzar
Bilateral telerobotic systems have attracted a great deal of interest during the last two decades. The major challenges in this field are the transparency and stability of remote force rendering, which are affected by network delays causing asynchrony between the actions and the corresponding reactions. In this article, the researchers propose a real-time frequency-based delay compensation approach maximize transparency while reducing the activation of the stabilization layer. The proposed technique will reduce the force-tracking error by 40% and the activation of the stabilizer by 79%.
In addition to the papers, the researchers are organizing two all-day workshops. The first is “Horizons of an Extended Robotics Reality (XR2) – a Converging Future of XR and Robotics,” organized by Atashzar. Through talks, interactions, and poster presentations, the hybrid workshop seeks to bring together researchers from the worlds of XR and Robotics, from Mechanisms and Control, AI/ML, HCI, Ergonomics, Human Factors, with the twin goals of presenting the advances in XR technologies and discussing how XR can help address challenges in robotics. The workshop will address the aspects of XR-mediated interaction in robotics, e.g., XR in telesurgery, XR for robot learning, tele-health, rehabilitation, industry, teleoperation, and more.
The second is “Agile Robotics: Perception, Learning, Planning, and Control,” organized by Loianno. The workshop brings together researchers from several heterogeneous robotics communities such as aerial, legged, ground, space robotics and autonomous vehicles to study and discuss scientific approaches for agile autonomous robots’ navigation across air, space, ground, and off-terrain domains. It will consist of 14 different talks by experts and panel discussions.
In addition, Righetti has been invited to speak at “Cloud and Fog Robotics In The Age of Deep Learning.” As roboticists move towards computationally expensive models — such as deep neural networks — for perception and planning, resource-constrained robots like low-power drones often cannot run the most accurate, power-hungry compute models. In such cases, cloud and fog robotics allows robots to utilize both on-robot and cloud resources for compute and storage. The workshop will convene researchers and industry experts from computer systems and robotics to jointly discuss the challenges and promises of cloud-based robotics.
Atashzar is also invited to speak at “A Panacea or an Alchemy? —— Benefits and Risks of Robot Learning in Medical Applications.” This workshop will specifically focus on advancements in machine learning techniques (including deep learning, reinforcement learning) for medical robots’ perception, modeling, control, and navigation.