Modern artificial intelligence — causality and fairness in recommendation systems
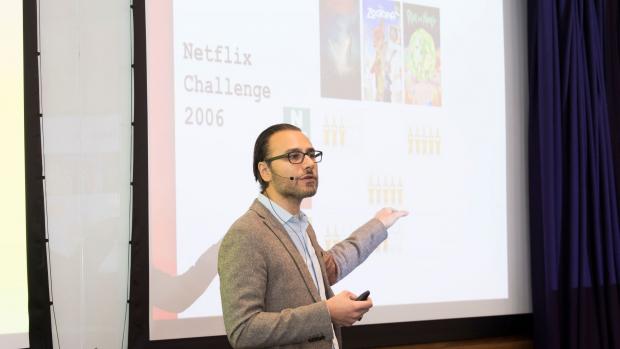
Tony Jebara, Director of Machine Learning at Netflix
Video is taking over the internet, a fact that can be corroborated by identifying who’s hogging the web. Netflix alone is responsible for 15 percent of global internet traffic, according to a report published by bandwidth management company Sandvine. A key player in the staggering oligopoly that Netflix and its competitors have created is Tony Jebara, Director of Machine Learning at Netflix and a professor on leave from Columbia University.
With a passion for machine learning even before it was a proven technology, Jebara particularly enjoys working with human-based use cases, noting that it is difficult to build predictive models on the basis of human behavior and its vagaries. “The non-conformity of humans to patterns and the inability to perfectly predict what they do is what makes the challenge interesting.”
Jebara spoke before a full house at NYU’s Center for Urban Science and Progress (CUSP), the second speaker of this year’s edition of the popular AI lectures, Modern Artificial Intelligence, hosted by NYU Tandon’s Department of Electrical and Computer Engineering and organized by Anna Choromanska, professor of computer science and engineering. He discussed how Netflix’s recommendation and personalization algorithms — responsible for a revenue increase of as much as $2 billion — drive how the video channel delivers content to individual tastes.
“Netflix isn’t really offering one product. It is offering hundreds of millions of products, because each person gets a unique flavor of the product. We personalize everything about the experience,” said Jebara, “[Each homepage] is really tuned for [a user] in many different ways, and all those ways are powered by Machine Learning.”
Jebara said Netflix takes three approaches to delivering personalized content: collaborative ranking, causal learning for messaging, and image personalization through real-time online learning.
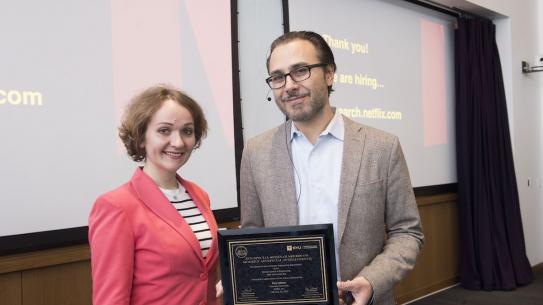
For example, Jebara oversaw the team designing the algorithm for the company’s thumbs-up, thumbs-down ranking system, which Netflix began using in 2017 instead of its now-defunct star rating system. He said his team deployed complex machine learning formulas to help predict what a user might be interested in watching based on past clicks.
While devising ways to apply machine learning to such problems as how to attract users to a show they might like in a non-intrusive way, and how to communicate with users, Jebara’s team examined causal factors that affect results, such as confounders, hidden factors that affect output and action, ultimately recommending a two-stage process using variables independent of confounders.
He went on to discuss the most significant change in Netflix’s personalization strategy, the use of user-targeting image art: Netflix uses an online machine learning model that resembles the widely famed Monte Carlo tree search method used by DeepMind’s AlphaGo Zero, in which user response over time hones the system’s sense of what images users prefer over time. By incorporating user data and context, they can then predict the kind of artwork a user will prefer based on his or her view history.
Jebara’s talk at NYU puts into context just how effective machine learning is at understanding and adapting to human behavior. The exponential rise in Netflix use over the past few years only reaffirms that idea.
The first series of Modern Artificial Intelligence seminars in 2018 attracted over a thousand students, faculty, and researchers to hear presentations by various stalwarts of AI and Machine Learning research. The talks, which are available to view online, have drawn the attention of more than 4,200 people worldwide.
Subsequent seminars will feature Manuela Velosa, Head of Machine Learning at JP Morgan Chase; and Eric Kandel, winner of the 2000 Nobel Prize in medicine.
Videet Rajeev Parekh
NYU Tandon School of Engineering
MS Computer Science, 2020