Tech Triumph: How Computer Imaging is Predicting Autism
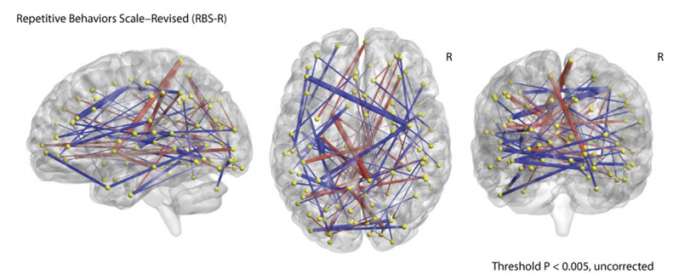
Differences in functional connectivity in ASD infants versus non-ASD infants. Robert W. Emerson et al., Sci Transl Med 2017
When clinical researchers wondered if it would be possible to detect autism before they could spot behavioral clues, they added to their team one of the world’s foremost authorities on medical image analysis: Guido Gerig, chair and professor in NYU's Tandon School of Engineering’s Department of Computer Science and Engineering. Gerig’s research team is part of NYU Tandon’s Visualization and Data Analytics (ViDA) Laboratory.
The psychiatrists, radiologists, and computer scientists published a landmark study earlier this year that predicted autism by looking not at how kids act but how their brains grew. Current work, which builds on earlier studies, suggests that neurological signs of autism might be detectable in infants as young as six months old.
The IBIS (Infant Brain Imaging Study) was sponsored by National Institutes of Health’s NICHD (National Institute of Child Health and Human Development) under the Autism Centers of Excellence Program. In this study, published in Science Translational Medicine, 59 six-month-old babies with an autistic older sibling had their brains scanned using functional magnetic resonance imaging (fMRI), a technology that can produce three-dimensional images of brain activity. Using these scans, the researchers then mapped 26,355 connections spanning 230 regions of the brain in each baby, then identified 974 connections related to the later development of autism.
— Guido Gerig
The image data was analyzed using a method known as a machine learning classifier. This program sorted through the differences in the way key brain regions — crucial for cognition, memory, and behavior — are synchronized and the change in such synchronization with age. Analyzing these maps resulted in a computer prediction modeling that correctly identified 81 percent of babies (9 of 11) who met the criteria for autism at two years of age. “These findings could pave the way for early diagnosis of autism with neuroimaging technologies, and offer possibilities for therapeutic intervention far before the current diagnosis at 24 months. Moreover, these key scientific findings propose origins of autism even earlier than six months, which is highly relevant particularly in the context of current discussions of the role of vaccines in causing autism,” Gerig said.
“This approach to image data has given us a new way of looking at the time of origin and developmental trajectories of many neurological conditions,” he added. The ACE-IBIS imaging study motivated the development of a number of new image processing methods and tools to study changes in anatomical structures as a function of aging, neurodevelopment, disease, or therapies. In another high-risk autism study, the NYU Tandon medical image analysis team collaborated with an ACE-IBIS partner at the University of Pennsylvania on developing ways to gain insight into brain maturation of individuals rather than whole populations. He is also a research partner in a clinical study to explore the effect of drug-addiction on early brain development, using the same neuroimaging methodologies.
Gerig's team remains part of the ACE-IBIS study group which has applied for new funding to continue imaging of infants into school age (7 to 10 years). His team has also started collaborations with NYU’s Department of Child and Adolescence Psychiatry and the Child Mind Institute, which are partners in the Autism Brain Imaging Data Exchange (ABIDE) project.
Gerig’s tools and methods are developed as open source software and made available to the public, including teaching materials and training workshops.
Gerig’s team at NYU Tandon continues to collaborate with NYU clinical partners in medicine, engineering, and statistics to support existing and novel imaging studies with state-of-the-art processing, analysis, and visualization tools.