New Faculty Help Keep Tandon's Finance and Risk Engineering Department on the Cutting Edge
The wide variety of expertise our new Finance and Risk Engineering (FRE) adjuncts bring with them is enabling students to stay on the leading edge and preparing them for jobs in the rapidly evolving financial industry. Students can now, for example, take a course that focuses on blockchain technology and the consensus protocols behind Bitcoin and the other cryptocurrencies making headlines on a regular basis. Machine learning, which has been steadily changing the nature of the industry in fundamental ways in recent years, also features broadly into the new curriculum, with several of the new instructors acknowledged as leaders in the field.
New FRE Faculty:
- Jerome Benveniste
- Maggie and Tom Copeland
- Sebastien Donadio
- Monty Essid
- Roza Galeeva
- Vikram Kuriyan
- Tore Opsahl
- Gordon Ritter
- Louis Scott
- Tyler Ward
- Kenneth Zhang
Jerome Benveniste

Jerome Benveniste’s students get the benefit of a dual perspective when taking his active portfolio management course: before joining Tandon’s Department of Finance and Risk Management, he was both a professor of pure mathematics and a working quant. “My approach to teaching encompasses both of those worlds,” he explains. “I want my students to understand the mathematical concepts underpinning what they’ll be doing as analysts in the financial industry, because if they understand the theory, they’ll know in what situations it will apply and when it will need to be modified.”
Beneviste, who earned his bachelor’s degree in mathematics from Harvard in 1990 followed by a doctoral degree from the University of Chicago in 1996, remained in academia during the first stage of his career, teaching at Stanford and Case Western Reserve. In 2002 he joined Highbridge Capital Management, a well-regarded investment firm headquartered in New York, and there he moved up the corporate ladder from associate to senior vice president, and, ultimately, managing director.
He retired from Highbridge in 2014 and has been relishing his return to the classroom. “I enjoy giving my students a look at what’s involved in working day-to-day in the industry, and I know firsthand what skills they will need to bring with them,” he says. “I want them to complete my course comfortable with the idea of approximation and uncertainty. Unlike the problems that are addressed in pure mathematics, the problems they will face as quants might not be solved in a rigid, linear way. Real-life problems can be messy.”
Maggie and Tom Copeland

Look at Maggie and Tom Copeland's CVs, and you are likely to be staggeringly impressed. The co-founders of an eponymous trading group, they have decades of collective experience to their credit.
The first woman ever to earn a doctoral degree in finance from UCLA, Maggie Copeland has published her research in such periodicals as the Financial Analyst Journal, the Journal of Portfolio Management, and Financial Management and has worked as a risk manager at Fidelity Investments, a senior portfolio manager and hedge fund manager at Citigroup, the vice president of proprietary trading at NatWest Securities, a proprietary trader at Bear Stearns, and a partner and portfolio manager at Roll and Ross, to list just some of the entries on her resume.

Tom Copeland has been teaching since the early 1970s at such institutions as UCLA, MIT, and Harvard and is the author of Finance Theory and Corporate Policy, a graduate-level textbook that has been in print for almost four decades, as well as six other highly regarded books and dozens of articles that have garnered more than 24,000 citations in the Google author index.
In addition to making his mark in academia and in print, he served for several years as a partner and co-leader of the corporate finance practice at McKinsey & Co., and when journalist Duff McDonald wrote about the iconic company in his 2013 book, The Firm, he referred to Copeland as the “valuation guru.” (Maggie Copeland has joked that her role in their work partnership is to “computerize the guru’s brain.”) And that’s all before mentioning that he has appeared twice as an expert witness before the World Court, testifying on corporate valuation.
They each possesses a fierce intellect and deep understanding of the financial industry, and students would undoubtedly count themselves lucky to be taught by either one. At NYU Tandon, however, FRE students will get the benefit of both, since Department Chair Peter Carr (who once served as Tom Copeland’s research assistant at UCLA during his own student years) has tapped them to co-teach a course.
In the classroom and in the financial industry, the Copelands prove the old adage that two heads are better than one.
Sebastien Donadio

It would be no surprise to anyone casually perusing his CV that Sebastien Donadio is teaching at an engineering school. Currently head of software engineering at HC Technologies, where he manages software development, Donadio has a doctoral degree in computer engineering, with a specialty in high-performance computing and software design and development. But if you were to assume that he is a faculty member in the NYU Tandon Department of Computer Science and Engineering, you would be mistaken. Donadio has long worked in the financial world and is one of the newest members of Tandon’s Department of Finance and Risk Engineering, where his varied areas of expertise make him a valuable participant in Department Chair Peter Carr’s mission to incorporate more machine learning courses into the curriculum.
With artificial intelligence playing an increasing role in many aspects of the industry, from trading and portfolio management to mortgage lending and insurance underwriting, Donadio — who has been a quantitative trading strategy software developer, project lead for the Department of Defense, and IT credit risk manager, among other positions — has a wealth of experience to impart to FRE students on the technology and algorithmic finance track.
An avid programmer since he became entranced by an Amstrad 8-bit home computer as a child, Donadio has also been on the faculty of the University of Versailles, the University of Delaware, and the University of Chicago, and during his earliest forays in the classroom, he taught Java to economics students. “I am an engineer. I like building stuff,” he has said. “What other domains can you build something as fast as computer science? Teaching my students the power of building something they want in a very short period of time is exciting.”
He finds it especially gratifying to explain concepts that he knows will prove useful and practical to students as they enter the workforce. “Seeing my students’ progress and then watching them go on and get a job they love is more than I can ask for,” he has said. “Teaching, for me, is a way of closing the gap between two evolving worlds.”
Monty Essid

Adjunct Instructor Monty Essid remembers very well what it’s like to be a student: that’s because he is, in fact, still a student. Essid — who in 2013 earned his undergraduate degree from L’Ecole Centrale Paris in his native France and his master’s degree in Applied Math and Applied Physics from Columbia — is currently a doctoral candidate at NYU Courant, and he balances his own studies with teaching courses both there and at NYU Tandon.
Essid’s work focuses on the various aspects of Optimal Transport (a mathematical theory that allows for comparing two probability mesaures); generalizations of the original theory that enable it to be used as a natural mathematical framework for data-based applications in machine learning, data science, and finance; connections with stochastic optimal control (a subfield of control theory that deals with the existence of uncertainty); and mean field games (which involve strategic decision making in large populations of small interacting agents).
“In the financial industry, when we work with stocks and options, we must try to predict upswings and downturns by using models,” he explains, “and those models have to realistically allow for uncertainty and randomness.”
There is little uncertainty about Essid’s future path. He transitioned easily to life in New York, thanks to its diversity and cosmopolitan nature — as well as the ease of finding good French restaurants here — and he is engaged to be married to a fellow NYU graduate student. He intends to continue teaching after earning his Ph.D. and joining the world of professional finance. “I’ve been offered a few jobs in industry,” he says, “but I’ve made it plain in interviews that I need a schedule that will allow me to retain my adjunct posts, which I find very rewarding.”
Roza Galeeva
On occasion, the new Finance and Risk Engineering professor Roza Galeeva goes by the moniker Rhoza, because rho, the 17th letter of the Greek alphabet, is the mathematical symbol for correlation, the study of which has been a focus during her long career at Morgan Stanley (MS).
The credit for the creative twist goes to Department Chair Peter Carr, then a colleague at the company, who suggested using her name as a new “Greek” (derivative, that is), with respect to correlation. Alex Eydeland, a legendary figure in the commodities world who served for more than a decade as the head of commodity quants at MS, hit upon “Rhoza.” “Correlations — specifically correlations in commodity — is my passion, my life and my first name,” she says.
Here at NYU Tandon, she has several aims. First, she hopes to inspire in her students an appreciation of how exciting and challenging working with commodities can be. “You are not dealing with dry abstractions,” she explains. “You’re looking at real assets like pipelines, power plants, storage facilities, oil wells; you can’t create a universal model to value a power plant, for example, as every plant has unique features and physical characteristics.” She continues, “Every commodity is unique: a commodity delivered to a particular location, on a certain month, day or even hour, could be very different from the same commodity delivered at a different location or time.”
Another objective is to prepare her students for a seamless progression from the classroom into the real world: she wants to show them how the beauty and power of mathematics can be used in real applications (in particular, for modeling commodity derivatives) and to help them develop the skills for working with real problems and real data — looking for patterns and connections and delivering the results in a concise, clear fashion.
She hopes, as well, to attract more women to finance, a field in which they are still underrepresented. “I have been mentoring female students in my teaching posts, as well as at Morgan Stanley,” she says. “I want them to know they have a place at the table and can excel as long as they have the right knowledge, talent, confidence and attitude.”
While those are an ambitious set of goals, she is well-equipped to see them to fruition. She brings with her to NYU an impressive set of skills and capabilities — including almost 20 years of industry experience in modeling and risk management — and with advanced degrees in mathematical physics, she has extensive experience in academia also. She has taught advanced mathematical courses throughout the world, including the U.S., France, Mexico, Russia, and Bulgaria (doing so in five local languages, all of which she speaks fluently), and has done research in dynamical systems, collaborating and co-authoring papers with many renowned mathematicians.
It is clear why Chair Peter Carr, whom she first met at a financial conference in Mexico in 1999, recruited her to his department’s remarkable roster of instructors. “While my current job at Morgan Stanley is one I began dreaming of as soon as I decided to join the financial industry, I still often thought of returning to the classroom to teach and mentor,” she says. “I’m happy to get the chance to do that at NYU.”
Vikram Kuriyan

In his capacities as chairman of Global Wealth Allocation (GWA) Ltd., the founder and director of the Indian School of Business’s Investment Laboratory, and trustee of the nonprofit CFA Institute Research Foundation, Vikram Kuriyan very literally circles the globe multiple times a year. It’s a set of circumstances that has shaped his outlook on the financial industry — and on life itself. “As the pace of globalization accelerates, the world shrinks,” he says. “Talent and human capital are more vital to a company’s success than geographic location.”
Educated at MIT and Harvard, Kuriyan has a background as varied and wide-ranging as his travels. “I was exposed as a student to soft management skills, but I was more interested in hard, math-oriented finance,” he recalls. “A successful financial professional needs a good combination: a solid understanding of the mathematical theory along with a healthy skepticism about models, which are useful but have undeniable weaknesses.”
Kuriyan spent several years of his career at Bank of America’s asset management division, where as global head of quantitative strategies and chairman of the asset allocation committee, he saw every side of the industry, from equities to fixed income securities to hedge funds, and more. “It was a wonderful experience, because I was engaged in a virtuous cycle in which client interests and business incentives were nicely aligned.”
He sees teaching not just as a way of giving back to the industry that has helped him forge such a rewarding life but as a method of keeping himself sharp and improving his own thought processes. “When working in industry, I deal every day with very fine minds,” he says. “Being with students exposes me to fresh minds, and that’s a valuable thing in its own right.” He hopes that he can be an even better instructor than the admittedly brilliant figures he studied with in his own youth — one of whom was the Nobel Prize-winning economist Robert C. Merton. “In addition to having studied the academic theories underpinning the financial industry, I have the advantage of many years of real-world experience, so I think the students will benefit from that broad perspective,” he says. “The course may be called ‘Advanced Portfolio Management,’ but I want them to think of it as a look at the art and science of investing.”
Tore Opsahl

“It’s not enough for students interested in financial technology to know how to write computer code or run an on-line tool,” Tore Opsahl, one of the newest faculty members in the Department of Finance and Risk Engineering, explains. “They need to have an understanding of the entire model development pipeline because selecting the appropriate tool is only a tenth of the job. If they want to effectively deliver a completed project independently, which many will be called upon to do in the work world, they have to also know how to get it implemented.”
Opsahl aims, in his words, to better prepare students to become “not only experts in machine learning, but also experts in executing machine-learning projects,” thereby increasing their value to employers and allowing them to hit the ground running when they join the workforce.
Opsahl, an industry professional with varied experience, knows well the importance of delivering in the workplace. Now a Senior Trading Strategist within the Global Equities at Bank of America Merrill Lynch, he has also served as Chief Scientist at DeepMile Networks, a government-contracted data-analytics firm, where he developed and prototyped changes to large-scale big-data products, led a technical team in implementing them, and set the strategic direction as part of the senior executive team. He later joined with a group of DeepMile developers to form a spinout start-up, which provided information on companies' website traffic and social media presence.
Opsahl comes to NYU Tandon with not only broad industry experience, but a global outlook and background. Born in Norway, he studied and lived for a year during high school in Malaysia (where he was grateful for the year-round beach weather after Norway’s cold, dark winters). He subsequently spent many years in Great Britain (at the University of London and Imperial College London), California (at UC Irvine’s Paul Merage School of Business), and Washington, D.C. (where DeepMile was based). Since joining Bank of America in 2013, he has enjoyed living in New York and appreciates its diversity and vibrant culture. The only thing lacking, he quips, is Malaysia’s tropical climate.
Gordon Ritter

Even the most skilled mathematician or quantitative analyst can’t change the immutable fact that there are just 24 hours in a day. Gordon Ritter, however, seems to somehow fit much more work than should be possible into each 24-hour period.
In addition to designing and teaching a graduate course in advanced econometric modeling at NYU Courant, instructing Baruch College master’s students in portfolio management and algorithmic trading, and lecturing in Rutgers University’s Financial Statistics and Risk Management program, he recently joined the faculty of NYU Tandon, where he co-teaches (along with longtime friend and colleague Jerome Benveniste) a class entitled Topics in Active Portfolio Management. And as if that weren’t enough, Ritter is, in fact, an active portfolio manager: since 2015 he has been a senior manager with GSA Capital, a global firm that has garnered multiple EuroHedge Awards, widely regarded as being among the most prestigious honors in the finance industry.
“There are great teachers who’ve worked only in academia or who have retired from the trading floor and entered the classroom,” he says. “But students really appreciate being taught by someone still active in the industry. They know that what I’ve done during the day at GSA has a direct bearing on what I’m teaching them at night.” (The company has a small, selective internship program, so a few students might get the chance to experience a GSA workday themselves at some point.)
Ritter began his career as a quant shortly after earning his doctoral degree in physics from Harvard in 2007. While still a student, he began publishing numerous scholarly papers in such journals as Communications in Mathematical Physics, Physical Review, Journal of Mathematical Physics, and Experimental Mathematics, and he has continued to attract attention for his thought-provoking writing. A recent Newsweek article, for example, cited him as the author of “the first conclusive study that shows success in having a machine learning-based trading strategy” and explained that the 2017 paper, “Machine Learning for Trading,” was an unusual undertaking. “It's not uncommon to hear sophisticated quant shops espousing the opportunities of machine learning,” the reporter asserted, “but the fact remains that these firms don't really publish anything about how well this works.”
In the study, Ritter successfully employed a mathematical method called Q-learning, which involves reinforcement techniques, to teach a machine learning agent to find and exploit a profitable trading strategy. “Laypeople often think that the industry consists of people shouting across a crowded trading floor, as they see in movies, but that’s not the way things are done today,” Ritter says. “Thanks to machine learning, computers are doing the trading, and financial professionals now need a solid understanding of portfolio theory and statistical learning. My aim is to impart that.”
And if any of his students think that becoming successful in the industry requires staying inside yoked to a desk 24 hours a day, Ritter stands as an example that they are mistaken: he has completed full-distance ironman triathlons — and that’s in addition to skydiving, motorcycling, and other outdoor pursuits.
Louis Scott
Louis Scott has lived all over the country: from Louisiana and Georgia to Oregon, Illinois, and beyond, including a few years in London. Variety has also been a hallmark of his career, which has encompassed academia, the financial industry, and public service; before joining Tandon’s Department of Finance and Risk Engineering, Scott taught at such schools as the University of Illinois at Urbana-Champaign and University of Georgia, worked at iconic firms like Morgan Stanley and UBS, and now serves as an officer of the Federal Reserve Bank of New York, which had been founded by Congress near the turn of the 20th century to provide the country with a safe and stable monetary system and which many consider to be among the most powerful financial institutions in the world.
Scott will be focused on a different type of power here at Tandon: the course he has developed focuses on building financial models using graphics processing units (GPUs), the powerful processors designed to perform the complex mathematical and geometric calculations needed to render graphics. While GPUs were used early on mainly in graphics and gaming, they are now helping forge new frontiers in machine learning and analytics, and Scott explains that becoming proficient in this new technology will open up a wider array of job opportunities for graduates.
He cautions, however, that the new course is not for those who do not know their way around a computer lab: he and his students will have the privilege of working with NYU’s state-of-the-art High Performance Computing (HPC) clusters. “We’ll be building on their knowledge of C++, and they’ll need a basic understanding of financial modeling and mathematical techniques,” Scott explains. “We will use the new computing technology to develop and run models that would have been impossible 10 years ago.”
It is, he asserts, an especially exciting time to be involved in technology for the financial industry. “Look at all the advances that computer technology is making possible,” he says. “Soon our homes will be totally connected, and our cars will be driving themselves. Similarly big things are happening in finance thanks to high performance computers, and our FRE students will be in a position to be part of it all.”
Tyler Ward
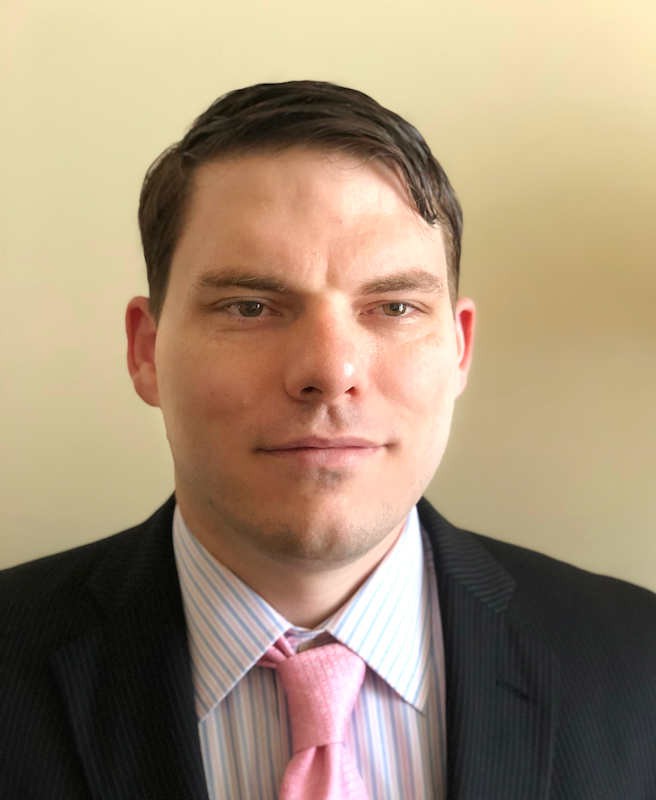
“In a classroom environment, a financial model may reflect things going exactly right, but that’s not real life; as a result some standard models and techniques never work out quite as well in real life as they do in the classroom,” new FRE faculty member Tyler Ward explains. “The fundamental human condition is that data comes from the past, but predictions are about the future. Often, these environments are not the same, so we need to take extra care when designing classroom exercises to make sure that they reflect this reality.”
Citing the financial crisis of 2008 as a good illustration of what happens when a model results in a horrible mistake, he continues, “The models used in that situation were made with data that simply didn’t go back far enough: no recession had occurred in the years the data covered. The danger was recognized even at the time, but it takes great care to build a model that can safely navigate an environment that doesn’t exist in the historical record.”
Ward, now the head of local search at Google, was working at Morgan Stanley at the time of the crisis, studying the solvency of Fannie Mae and Freddie Mac, organizations created by Congress to try to provide stability and affordability in the U.S. mortgage market. “My brief brush with pop-culture fame came at that time,” he quips, explaining that he worked only a few floors away from Howie Hubler, the infamous bond trader who played a major role in the single biggest trading loss ($9 billion) in Wall Street history. “Michael Lewis, the author of The Big Short, which was later made into a movie, dramatized the events at Morgan Stanley but got many things wrong,” he says. “In the few pages of the book that describe our work with Fannie Mae and Freddie Mac, he describes us making paper copies of our data and shipping them to India in cargo boxes, to be pored over by an army of temp workers. Obviously, we had analysts working in other time zones because it was a massive, around-the-clock undertaking, but by the turn of the 21st century, we were not making paper copies of anything, and certainly only software, not people, was individually analyzing actual loans.”
The students in the data science-focused course he teaches with colleague Kenneth Yu Zhang will be learning to load and analyze similarly massive data sets. “A key will be refining the questions they hope their data analysis will answer,” Ward says, “and, of course, trying to account for the unexpected circumstances that come up in the real world.”
Kenneth Zhang

“You do not have to be working directly in the field of data science to benefit from the course I am co-teaching with Tyler Ward of Google,” Kenneth Yu Zhang, one of the Department of Finance and Risk Engineering’s newest faculty members, explains. “You might one day be working on a team or collaborating with data scientists, and it would be very useful for you to know the basics and understand the lingo.”
Zhang comes to NYU Tandon with well over a decade of experience in the area, having served, among other posts, as a senior data scientist at Dell EMC, a lead data scientist at Ernst & Young, a VP and head of data science at Freedom Mortgage’s Business Innovation Group, and VP and lead data scientist at Morgan Stanley, where he now designs and builds machine learning and natural language processing models in the quantitative, marketing, fixed income, and equity realms. His affiliation with FRE does not mark his first experience in academia, however; early on, he taught at the Beijing University of Technology, in his native China, and after earning his doctoral degree in applied mathematics from Rutgers University and the New Jersey Institute of Technology (NJIT) in 2008, he joined the faculty of the School of Medicine at Mount Sinai, where he focused on mathematical and statistical modeling, data mining, machine learning, bioinformatics and computational neuroscience, among other such topics. “There really is no limit to where you can apply a knowledge of machine learning, natural language processing, and data analytics,” he says of that foray into the medical field, “and use in the financial industry has been exploding in recent years.”
Zhang remembers earlier days, during his time as a computer systems engineering master’s student at the University of Southern Denmark, when everyone studying AI tools was most excited about their applicability to robotics. “Once I discovered that working in the financial industry would allow me to use my knowledge to solve real-world problems with major economic impact, I found my career path,” he says. “I’m looking forward to helping my students do the same by assigning practical assignments involving large data sets in a realistic work environment, just as they’ll encounter after graduation.”