Computer Vision Expert Martial Herbert Kicks of Second Year Of Seminars on Modern Artificial Intelligence
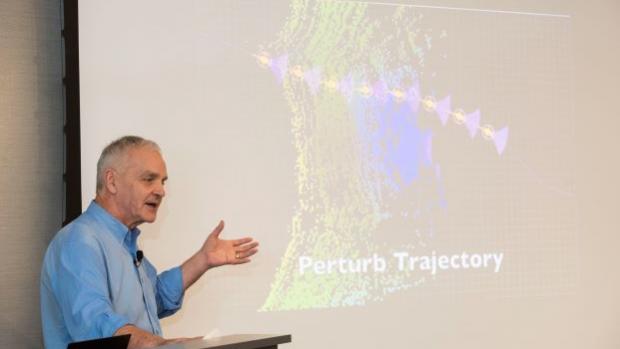
Martial Hebert, Professor of Robotics at Carnegie-Mellon University and Director of the Robotics Institute
Whether fast-moving autonomous vehicles on roads and highways, airborne drones zipping between buildings or anthropomorphic machines moving about on legs, mobile robots need artificial intelligence (AI) models that interpret data from cameras, LiDAR, GPS and more to know when the object in its path is a pedestrian, plastic bag, brick, puddle, or shadow. In many real-world autonomous-machine problems, a high level of accuracy is imperative; failure costs can be high, not just monetarily, but ethically and socially as well.
Martial Hebert, a Professor of Robotics at Carnegie-Mellon University and Director of the Robotics Institute, whose work in Machine Learning and Computer Vision has established him as one the foremost authorities on autonomous robotics and perception explained the challenges involved in creating computer vision systems that knows when to trust its own eyes, so to speak.
Hebert spoke to a packed house at NYU Tandon’s Maker Space, kicking off the second year of NYU Tandon’s popular AI lectures, Modern Artificial Intelligence, organized by Anna Choromanska and hosted by NYU Tandon’s Department of Electrical and Computer Engineering. He used all-too-human expressions to describe what machine learning systems must evince when using an operational vision system: introspection and even self-awareness.
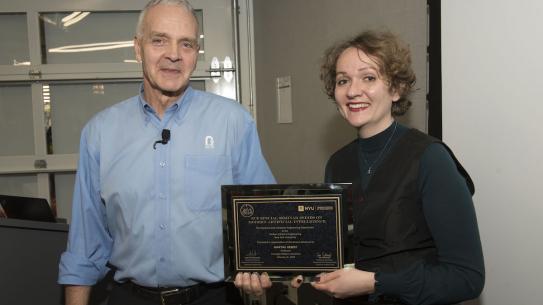
At the interface of data and interpretation, the AI must be capable of saying the cybernetic version of “I’m not sure.” If a person walks through a forest at dusk he or she will almost certainly slow their pace to the extent that his or her vision is compromised by shadows and darkness. Is that dark spot a hole in the ground or leaves?
Similarly, a robotic system must be able to pause rather than assign a label to an image that may not be what it appears to be.
“Should a perception system always give an answer; can we anticipate when it shouldn’t or when it will be unreliable,” said Hebert.
Hebert, whose interests include computer vision, recognition in images and video data, object recognition from 3D data, and perception for mobile robots and for intelligent vehicles raised 3 major points where he believes most challenges lie:
- The assumption that the system will always produce an output
- Minimizing training data in visual models
- Making time-budgeted decisions with varying degrees of accuracy
While integrating computer vision into real-time systems, a common assumption is that everything the camera sees or sensor senses is interpreted, parsed or otherwise handled by the system — a model for every situation. Hebert argues that there are scenarios where this does not hold true and can cause a system to fail early. He recommends addressing this by introducing a so-called “introspection engine” that maps directly from input to accuracy, to identify whether a given input can (or should) produce an output at all. This allows the system to anticipate failures and change approach — allowing a drone in mid-flight to pause and reassess, for example, instead of risking slamming into a tree that might or might not be a shadow.
He also articulated a method for reducing supervision by using as little training data as possible to learn a model. This involves incorporating prior experience into the model and using minimal training examples.
And, in situations where the output is constrained by time or memory or other external limitations, ensuring that a system always delivers an output is a difficult task. The idea is to produce an output of varying quality, based on how limiting the constraints are. Hebert discusses the use of a layered neural network to produce finer data the more the resources available, but capable of delivering coarse data when constraints are very high.
Hebert’s work at CMU continues to drive AI innovation and has helped transform Pittsburgh into a thriving automation hub today with over 60 robotics startups.
“This series, which we launched last year, aims to bring together faculty, students, and researchers to discuss the most important trends in the world of AI, and this year’s speakers, like last year’s, are world-renowned experts whose research is making an immense impact on the development of new machine learning techniques and technologies,” said Choromanska. “In the process, they’re helping to build a better, smarter, more-connected world, and we’re proud to have them here.”
Subsequent seminars will feature Tony Jebara, Director of Machine Learning at Netflix and associate professor (on leave) of computer science at Columbia University; Manuela Velosa, Head of Machine Learning at JP Morgan Chase; and Eric Kandel, Nobel Prize Winner.