Rose Faghih
-
Associate Professor of Biomedical Engineering
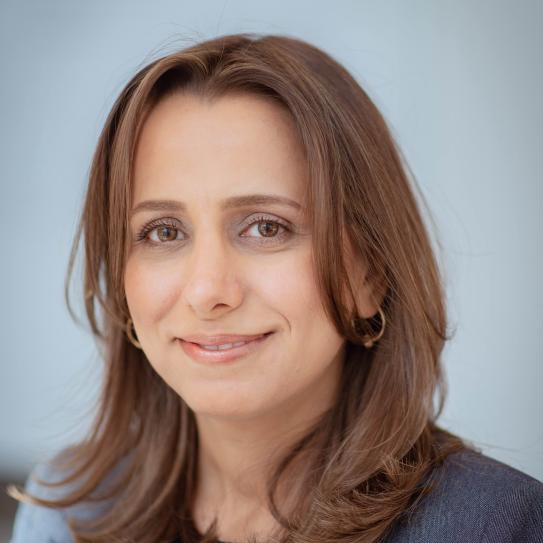
Education
Massachusetts Institute of Technology
Postdoctoral, Brain and Cognitive Sciences
Massachusetts Institute of Technology
Ph.D., Electrical Engineering and Computer Science
Massachusetts Institute of Technology
S.M., Electrical Engineering and Computer Science
University of Maryland
B.S. (Summa cum Laude), Electrical Engineering (Honors Program)
Awards & Distinctions
- MIT Technology Review Innovator Under 35, 2020
- National Science Foundation CAREER Award, 2020
- Junior Faculty Research Excellence Award, Cullen College of Engineering, University of Houston, 2020
- Teaching Excellence Award, Cullen College of Engineering, University of Houston, 2020
- Featured in IEEE Women in Engineering Magazine as a 'Woman To Watch', 2020
- Selected for the National Academy of Engineering's Frontiers of Engineering Symposium, 2019
- IEEE-USA’s New Face of Engineering, 2016
- National Science Foundation Graduate Research Fellowship, on tenure 2009-2012
- Massachusetts Institute of Technology Graduate Fellowship in Control, 2008
- Department of Electrical and Computer Engineering Chair’s Award, University of Maryland, 2008
- Phi Kappa Phi Honor Society, Inducted in 2008
- Tau Beta Pi, The Engineering Honor Society, Inducted in 2008
- Eta Kappa Nu, The Honor Society of IEEE, Inducted in 2008
- University of Maryland President's Scholarship, 2006-2008
Research News
New study tracks leptin pulse patterns, a potential clue to understanding obesity
Obesity has become a global epidemic, and the need for treatment and monitoring for people with obesity is growing. Researchers aiming to understand relevant biomarkers for the condition have fixed their gaze on leptin, a hormone that regulates energy intake and induces feelings of fullness, to eventually help improve treatments for obesity. Understanding the patterns of leptin secretion from fat cells throughout the body could help scientists identify hidden health issues in patients with obesity, monitor health development after treatment, and test drug effectiveness.
Now, in a new study in the Journal of the Endocrine Society, NYU Tandon Associate Professor of Biomedical Engineering Rose Faghih and her PhD students Qing Xiang and Revanth Reddy have utilized a probabilistic physiological modeling approach to investigate the pulse events underlying leptin secretion.
“Instead of only relying on visual inspection of leptin by statistical modeling of leptin secretion events, we quantify the underlying pulsatile physiological signaling to enable extending investigations of leptin signaling in health and disease and in response to medications,” said Faghih.
Leptin, produced primarily by fat cells, typically signals the brain when enough food has been consumed, helping to regulate energy and appetite. But in some individuals with obesity, this signaling system seems to malfunction — a condition known as leptin resistance, where high levels of leptin fail to curb hunger. Why this resistance develops remains unclear, but recent findings on leptin’s high-frequency, wave-like release patterns offer intriguing possibilities for future research.
Hormones like cortisol and insulin, which manage stress and blood sugar, are known to follow rhythmic release patterns, adapting to daily cycles. Leptin follows a similar — but unknown
— rhythm. Faghih’s lab set out to analyze leptin’s pulses, examining how often these leptin pulses occur and their relative strength. By breaking down the pulses into their timings and amplitudes, researchers could better understand these patterns, which could lead to breakthroughs in treating leptin resistance and, by extension, obesity.
The researchers applied statistical models with different complexities to fit the data and compared the performance of these distribution models for each subject using pre-established metrics. They aimed to find the best model for these distributions which would be important in monitoring leptin secretion and detecting deviations from a normal secretion pattern.
The results show that each of these models can capture the general shape of the distribution alone for each subject despite the complex process of leptin secretion which can be affected by various factors. These distribution models suggest several possible features of leptin secretion such as the rarity of extremely small or large pulses.
They also allow researchers to see the effects of drugs by identifying changes in the model parameters before and after treatment. They compared leptin behavior before and after treatment with bromocriptine, a medication that affects neuroendocrine signaling. After treatment, subtle shifts in one model of leptin’s pulse timings (the diffusion model) suggested that it might be possible to influence these patterns with medication. Such findings open the door to exploring hormonal treatments for obesity that work by reestablishing leptin’s natural rhythms.
The research is a key first step in reliable long term leptin monitoring that could help doctors and patients fight obesity.
This work was supported by the National Institutes of Health (NIH) under grant R35GM151353: MESH: Multimodal Estimators for Sensing Health.
Qing Xiang, Revanth Reddy, Rose T Faghih, Marked Point Process Secretory Events Statistically Characterize Leptin Pulsatile Dynamics, Journal of the Endocrine Society, Volume 8, Issue 10, October 2024, bvae149, https://doi.org/10.1210/jendso/bvae149
Harnessing the power of eye tracking in brain-machine interfaces
In recent years, eye tracking technology has advanced rapidly, suggesting that our eyes deserve greater attention within the evolving brain-machine interface (BMI) landscape. One particularly intriguing area is the connection between eye movements and internal brain states—a link that is becoming increasingly difficult to ignore. Eye tracking systems can function in a completely contactless manner, integrated into devices like screens, laptops, tablets, and smartphones. In contrast, wearable-based systems utilize wearable technology to monitor and even influence brain states, presenting a more hands-on approach to BMI development.
However, a promising alternative lies in the development of a framework that decodes hidden brain states, such as interoceptive awareness, directly from eye tracking data. This advance could help create safer, more efficient closed-loop systems that monitor and modulate the brain-body connection. That is the findings of a new study from the lab of Rose Faghih, Associate Professor of Biomedical Engineering at NYU Tandon.
Decoding the Brain’s Hidden Signals
Interoceptive awareness represents the brain’s ability to interpret bodily sensations—signals that arise in response to internal or external stimuli. However, these states are difficult to observe and must be decoded through physiological indicators. Tracking and understanding these internal brain states is critical for optimizing the brain-body connection, yet the challenge lies in how to access them.
One potential solution is to study interoception in the context of fear conditioning, a process where heightened arousal correlates with heightened interoception. In Pavlovian fear conditioning, subjects learn to anticipate aversive events—such as a mild electric shock—creating an ideal model for observing interoceptive signals. In a recent experiment, participants underwent fear conditioning and extinction, with mild electric shocks used as the aversive stimulus. Given the strong association between arousal and interoceptive awareness, researchers anticipated synchronized responses between these two states.
In this study, the research team decoded interoceptive awareness by analyzing neural activity linked to eye tracking data—specifically, measurements from pupillometry and eye gaze patterns. In parallel, they decoded arousal states from skin conductance data. While it was expected that the two states would show similar responses to the electric shock, the interoceptive awareness state, as inferred from eye tracking data, showed greater sensitivity to the mild shocks than the arousal state decoded from skin conductance.
This finding underscores the potential of eye tracking technology as a powerful psychophysiological tool for decoding interoceptive awareness, a signal that could offer significant insight into brain-body interactions.
Towards Future Closed-Loop Systems: The Dawn of ‘MINDCAM’
The discovery that eye tracking signals can serve as sensitive indicators of interoceptive awareness opens up exciting possibilities. These findings could pave the way for new therapeutic approaches to treating neuropsychiatric and neurodegenerative disorders. By decoding interoceptive awareness, future closed-loop systems may be able to restore and enhance the brain-body connection, offering safer, more personalized interventions.
One particularly promising application is the development of ‘MINDCAM’—a system that integrates eye-tracking-enabled cameras into devices like smartphones, tablets, and monitors. This technology could potentially monitor a user’s interoceptive awareness in real time, helping individuals regulate their mood and cognitive performance. However, while this research represents an exciting first step, much more work is needed to develop safe and effective closed-loop systems that can reliably decode and modulate interoceptive states.
Faghih’s previous research on wearables includes the development of ‘MINDWATCH,’ which uses information collected from electrical charges in skin to assess brain states. MINDCAM could be used to complement that technology to provide even better data on how the brain reacts to stress.
The integration of eye tracking technology into brain-machine interfaces may hold the key to unlocking deeper insights into the mind, offering new hope for improving mental health and cognitive function in the years to come.
This research was supported in part by National Institutes of Health (NIH) grant R35GM151353 - Maximizing Investigators' Research Award (MIRA) for Early Stage Investigators (ESI): MESH: Multimodal Estimators for Sensing Health and in part by National Science Foundation (NSF) under Grant 2226123 - Faculty Early Career Development Program (CAREER): MINDWATCH: Multimodal Intelligent Noninvasive brain state Decoder for Wearable AdapTive Closed-loop arcHitectures.
Saman Khazaei, Rose T Faghih, Eye tracking is more sensitive than skin conductance response in detecting mild environmental stimuli, PNAS Nexus, Volume 3, Issue 9, September 2024, page 370
New research develops algorithm to track cognitive arousal for optimizing remote work
In the ever-evolving landscape of workplace dynamics, the intricate dance between stress and productivity takes center stage. A recent study, spanning various disciplines and delving into the depths of neuroscience, sheds light on this complex relationship, challenging conventional wisdom and opening new pathways for understanding how to improve productivity.
At the heart of this exploration lies the Yerkes-Dodson law, a theory proposing an optimal level of stress conducive to peak productivity. Yet, as researchers unveil, the universality of this law remains under scrutiny, prompting deeper dives into the nuances of stress-response across different contexts and populations.
Drawing from neuroscience, researchers from NYU Tandon led by Rose Faghih, Associate Professor of Biomedical Engineering, have published a study illuminating the role of autonomic nervous system, which is directly influenced by key brain regions — like the amygdala, prefrontal cortex, and hippocampus — in shaping our responses to stress and influencing cognitive functions. These insights not only deepen our comprehension of stress but also offer pathways to enhance cognitive performance.
The researchers’ approach is innovative, in that it concurrently tracks cognitive arousal and expressive typing states, employing sophisticated multi-state Bayesian filtering techniques. This allows them to paint a picture of how physiological responses and cognitive states interplay to influence productivity.
One particularly innovative aspect of the study involves typing dynamics as a measure of cognitive engagement and emotional expression. By examining typing patterns and brain autonomic nervous system activation, researchers gain insights into individuals' cognitive states, especially relevant in remote work environments. The integration of typing dynamics into the analysis provides a tangible link between internal cognitive processes and externalized behaviors.
“With the rise of remote work, understanding how stress impacts productivity takes on newfound significance,” Faghih says. “By uncovering the mechanisms at play, we’re paving the way for developing tools and strategies to eventually optimize performance and well-being in remote settings.”
Moreover, the study's methodology, grounded in sophisticated Bayesian models, promises not only to validate existing theories but also to unveil new patterns and insights. As the discussion turns to practical applications, the potential for integrating these findings into ergonomic workspaces and mental health support systems becomes apparent.
This research offers a glimpse into the intricate web of stress, productivity, and cognition. As we navigate the evolving landscape of work, understanding these dynamics becomes paramount, paving the way for a more productive, resilient workforce.
Alam, S., Khazaei, S., & Faghih, R. T. (2024). Unveiling productivity: The interplay of cognitive arousal and expressive typing in remote work. PLOS ONE, 19(5). https://doi.org/10.1371/journal.pone.0300786
How can music choices affect productivity?
Human brain states are unobserved states that can constantly change due to internal and external factors, including cognitive arousal, a.k.a. intensity of emotion, and cognitive performance states. Maintaining a proper level of cognitive arousal may result in being more productive throughout daily cognitive activities. Therefore, monitoring and regulating one’s arousal state based on cognitive performance via simple everyday interventions such as music is a critical topic to be investigated.
Researchers from NYU Tandon led by Rose Faghih — inspired by the Yerkes-Dodson law in psychology, known as the inverted-U law — investigated the arousal-performance link throughout a cognitive task in the presence of personalized music. The Yerkes-Dodson law states that performance is a function of arousal and has an inverted-U shaped relationship with cognitive arousal, i.e., a moderate level of arousal results in optimal performance, on the other hand, an excessively high level of arousal may result in anxiety, while a deficient level of arousal may be followed by boredom.
In this study, participants selected music with calming and exciting music components to mimic the low and high-arousing environment. To decode the underlying arousal and performance with respect to everyday life settings, they used peripheral physiological data as well as behavioral signals within the Bayesian Decoders. In particular, electrodermal activity (EDA) has been widely used as a quantitative arousal index. In parallel, behavioral data such as a sequence of correct/incorrect responses and reaction time are common cognitive performance observations.
The decoded arousal and performance data points in the arousal-performance frame depict an inverted U shape, which conforms with the Yerkes-Dodson law. Also, findings present the overall better performance of participants within the exciting background music. Considering the Yerkes-Dodson law, we develop a performance-based arousal decoder that can preserve and account for the cognitive performance dynamic. Such a decoder can provide a profound insight into how physiological responses and cognitive states interplay to influence productivity.
Although several factors, such as the nature of the cognitive task, the participant’s baseline, and the type of applied music, can impact the outcome, it might be feasible to enhance cognitive performance and shift one’s arousal from either the left or right side of the curve using music. In particular, the baseline of arousal level varies among humans, and the music may be selected to set the arousal within the desired range. The outcome of this research can advance researchers closer to developing a practical and personalized closed-loop brain-computer interface for regulating internal brain states within everyday life activities.
S. Khazaei, M. R. Amin, M. Tahir and R. T. Faghih, "Bayesian Inference of Hidden Cognitive Performance and Arousal States in Presence of Music," in IEEE Open Journal of Engineering in Medicine and Biology, doi: 10.1109/OJEMB.2024.3377923.