Spring 2023 ECE Seminars
Automotive CAN Reverse Engineering Seminar: Applying Reverse Engineering Analysis to Resolve Key Operational Parameters in Undefined Data
Speaker: William Rosenbluth, Automotive Systems Analysis (ASA) : Peter J Sullivan, Advanced Analysis Associates, Inc
Date: Tue, Jan 31
About the Speakers: William Rosenbluth has been President and Principal Engineer for Automotive Systems Analysis (ASA), Reston, VA, for 33 years. He has 58 years of experience with complex electro-mechanical, electronic and computer components and systems. He was employed by the IBM Corporation for 21 years, until forming ASA. At ASA, he specializes in the analysis and diagnosis of computer-related vehicle control systems and in the retrieval and analysis of electronic crash-event data in accident vehicles (black box data). His has authored two books, 'Investigation and Interpretation of Black Box Data in Automobiles' (2001) and 'Black Box Data from Accident Vehicles' (2009). He holds a BEE (‘61) and an MSEE (‘65) from the Polytechnic Institute of Brooklyn.
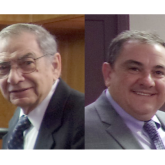
Peter J Sullivan has been President and Principal Engineer for Advanced Analysis Associates, Inc, for the past 26 years. His performs Forensic Expert Witness investigations for clients throughout the US, and he testifies in State and Federal Courts throughout the US. In his investigative capacity, he performs data downloads and imaging of Electronic Control Modules and ESI, including analysis and application to elements of accident reconstruction, validation, and electronic testing, on almost all makes and models of vehicles, equipment, and hand-held electronics. He holds a Bachelor of Science in Chemistry and Physics (‘84) from Texas State University.
Wireless Networks for Future Applications: from Networks of Drones to Adaptive Control of Integrated Circuits
Speaker: Igor Kadota, Massachusetts Institute of Technology
Date: Mon, Feb 6
Abstract: Emerging applications such as the Internet-of-Things and Smart-City Intersections have in common: (i) the potential to greatly benefit society, and (ii) the need for an underlying communication network that can satisfy stringent performance requirements in terms of data rates, latency, information freshness, scalability, and resiliency, which are unachievable by traditional networks, including current 5G deployments. Developing the next-generation communication networks is a challenging endeavor that requires interdisciplinary research combining rigorous theory, data-driven solutions, and experimentation with advanced wireless systems.
In this talk, I will discuss selected interdisciplinary projects, including: (i) A network control algorithm with provable performance guarantees in terms of information freshness and its implementation in a network of drones. (ii) A predictive weather-aware routing and admission control algorithm for a city- scale millimeter-wave backhaul network in Sweden. (iii) A system that adaptively reconfigures a highly complex state-of-the-art integrated circuit in order to enable full-duplex wireless communication. Finally, I will discuss my research vision on developing cross-layer networking solutions that can dynamically adapt the wireless systems and, at the same time, intelligently allocate the available communication and computation resources aiming to meet the stringent performance requirements of emerging and future applications.
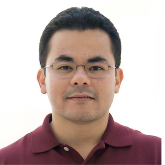
About the Speaker: Igor Kadota is a Postdoctoral Research Scientist at Columbia University. He received the Ph.D. degree from the Laboratory for Information and Decision Systems (LIDS) at MIT in 2020. His research is on modeling, analysis, optimization, and implementation of next-generation communication networks, with the emphasis on advanced wireless systems and time-sensitive applications. Igor was a recipient of several research, teaching, and mentoring awards, including the 2018 Best Paper Award at IEEE INFOCOM, the 2020 MIT School of Engineering Graduate Student Extraordinary Teaching and Mentoring Award, and he was selected as a 2022 LATinE Trailblazer in Engineering Fellow by Purdue’s College of Engineering. For additional information, please visit: http://www.igorkadota.com
Modern AI Series: AutoGluon: Empowering (Multimodal) AutoML for the Next 10 Million Users
Speaker: Alex Smola, Amazon Web Services
Date: Wed, Feb 8
Abstract: Automated machine learning (AutoML) offers the promise of translating raw data into accurate predictions without the need for significant human effort, expertise, and manual experimentation. AutoGluon is a state-of-the-art and easy-to-use toolkit that empowers multimodal AutoML. Different from most AutoML systems that focus on solving tabular tasks containing categorical and numerical features, we consider supervised learning tasks on various types of data including tabular features, text, image, time series, as well as their combinations.

About the Speaker: Alex Smola studied physics in Munich at the University of Technology, Munich and computer science at the University of Technology in Berlin. He received a PhD in 1998. After that, he joined the Australian National University and National ICT Australia (now part of Data61 at CSIRO) where he worked until 2008 as full professor and group leader. From 2008 to 2012 he worked at Yahoo! Research and moved to google in 2012. From 2013-17 he was full professor at Carnegie Mellon University's Machine Learning Department. Alex co-founded Marianas Labs in 2015. Since 2016 he works as VP/Distinguished Scientist at Amazon Web Services to help build AI and ML tools for everyone. His work includes Kernel Methods, Bayesian Nonparametrics, Distributed Optimization and Systems, and Deep Learning. He has published over 250 papers and five books.
Next-Generation Wireless Solutions for the Real World: Theory, Practice, and Opportunities Open configuration options
Speaker: Ian P. Roberts, University of Texas at Austin
Date: Thu, Feb 9
Abstract: Noteworthy challenges and ripe opportunities are unfolding as 5G cellular systems rollout and future 6G systems are imagined. Such systems make use of high carrier frequencies, wide bandwidths, and dense antenna arrays to meet the high-rate, low-latency demands of modern applications. In this talk, I highlight how next-generation millimeter wave (mmWave) transceivers can be upgraded with full-duplex capability: the long-sought ability to simultaneously transmit and receive over the same frequency spectrum. By combining theory and practice, I introduce two novel enablers of full-duplex mmWave systems that rely solely on beamforming to cancel self-interference to levels near or even below the noise floor, all while maintaining backward compatibility with beam alignment protocols in 5G. I demonstrate the effectiveness of my proposed solutions using 28 GHz and 60 GHz phased arrays, the first real-world evaluations of their kind. I conclude this talk by forecasting future research directions that will transform the next decade of wireless connectivity.

About the Speaker: Ian P. Roberts is a Ph.D. candidate at the University of Texas at Austin, where he is part of the 6G@UT Research Center within the Wireless Networking and Communications Group. He has been a visiting student at Arizona State University and Yonsei University. He has industry experience developing and prototyping wireless technologies at AT&T Labs, Amazon, GenXComm (startup), and Sandia National Labs. His research interests are in the theory and implementation of millimeter wave systems, full-duplex, and other next-generation technologies for wireless communication and sensing. He is a National Science Foundation Graduate Research Fellow.
Designing Computing Systems for Robotics and Physically Embodied Deployments
Speaker: Dr. Yuzhang Lin, University of Massachusetts, Lowell
Date: Mon, Feb 13
Abstract: The operation of modern power grids is increasingly challenged by the massive integration of volatile renewable energy as well as high-impact events such as natural disasters and cyber-attacks. The conventional rule-based operational paradigm is no longer a viable solution, and real-time situational awareness must be obtained from massive and heterogeneous sensor data streams to support intelligent decision-making and control. This talk will address two main pillars of the situational awareness required by a resilient and renewable power grid of the future. 1) Physics-informed adaptive data fusion for reliable interpretation of heterogeneous and imperfect data. Key methodologies allowing for the integration of grid physics with sensor data will be presented, including adaptive state estimation under unknown measurement error statistics, cyber-physically discriminative anomaly detection, and deep-learning-based forecasting of distributed renewable energy and load. 2) Resilient cyber-physical infrastructure for timely, economical, and uninterrupted data collection and transfer against disasters and attacks. Novel concepts for enhancing resilient data delivery will be introduced, including cross-domain sensor network planning for pre-disturbance hardening, observability-aware network routing for peri-disturbance adaptation, and observability-oriented network restoration for post-disturbance recovery. Future research plans with interdisciplinary collaboration opportunities will be discussed at the end of the talk.
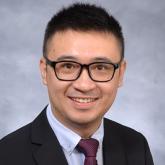
About the Speaker: Dr. Yuzhang Lin is currently an Assistant Professor in the Department of Electrical and Computer Engineering at the University of Massachusetts, Lowell. He obtained his Bachelor and Master’s degrees from Tsinghua University, Beijing, China in 2012 and 2014, respectively, and his Ph.D. degree from Northeastern University, Boston, MA in 2018. Since then, he has been a tenure-track Assistant Professor at the University of Massachusetts Lowell. His research interests focus on smart power grid and renewable energy systems, especially in the aspects of data-driven modeling, situational awareness, cyber-physical resilience, and machine learning applications. He has published 5 book chapters and 38 journal papers, and his research has been widely supported by federally funding agencies including NSF, DOE, and ONR. He currently serves as the Co-Chair of the IEEE Power & Energy Society (PES) Task Force on Standard Test Cases for Power Systems State Estimation, and the Secretary of the IEEE PES Distribution System Operation and Planning Subcommittee. He is a recipient of the NSF CAREER Award.
Towards Situation-Aware Resilient Power Grids for Massive Renewable Energy Integration
Speaker: Dr. Sabrina M. Neuman, Harvard University
Date: Tue, Feb 14
Abstract: Emerging applications that interact heavily with the physical world (e.g., robotics, medical devices, the internet of things, augmented and virtual reality, and machine learning on edge devices) present critical challenges for modern computer architecture, including hard real-time constraints, strict power budgets, diverse deployment scenarios, and a critical need for safety, security, and reliability. Hardware acceleration can provide high-performance and energy-efficient computation, but design requirements are shaped by the physical characteristics of the target electrical, biological, or mechanical deployment; external operating conditions; application performance demands; and the constraints of the size, weight, area, and power allocated to onboard computing-- leading to a combinatorial explosion of the computing system design space. To address this challenge, I identify common computational patterns shaped by the physical characteristics of the deployment scenario (e.g., geometric constraints, timescales, physics, biometrics), and distill this real-world information into systematic design flows that span the software-hardware system stack, from applications down to circuits. An example of this approach is robomorphic computing: a systematic design methodology that transforms robot morphology into customized accelerator hardware morphology by leveraging physical robot features such as limb topology and joint type to determine parallelism and matrix sparsity patterns in streamlined linear algebra functional units in the accelerator. Using robomorphic computing, we designed an accelerator for a critical bottleneck in robot motion planning and implemented the design on an FPGA for a manipulator arm, demonstrating significant speedups over state-of-the-art CPU and GPU solutions. Taking a broader view, in order to design generalized computing systems for robotics and other physically embodied applications, the traditional computing system stack must be expanded to enable co-design with physical real-world information, and new methodologies are needed to implement designs with minimal user intervention. In this talk, I will discuss my recent work in designing computing systems for robotics, and outline a future of systematic co-design of computing systems with the real world.

About the Speaker: Sabrina M. Neuman is a postdoctoral NSF Computing Innovation Fellow at Harvard University. Her research interests are in computer architecture design informed by explicit application-level and domain-specific insights. She is particularly focused on robotics applications because of their heavy computational demands and potential to improve the well-being of individuals in society. She received her S.B., M.Eng., and Ph.D. from MIT. She is a 2021 EECS Rising Star, and her work on robotics acceleration has received Honorable Mention in IEEE Micro Top Picks 2022 and IEEE Micro Top Picks 2023.
Designing, Prototyping, and Automating Next-Generation Hardware
Speaker: Austin Rovinski, Cornell University
Date: Thu, Feb 16
Abstract: For nearly five decades, Moore’s Law offered the promise of exponentially increasing computer performance. In Moore’s own words, however, “no exponential is forever”. The past decade has witnessed the stagnation of general-purpose computer performance and the consequential rise of specialized processors to succeed them. While specialized processors offer order-of-magnitude performance and energy efficiency improvements over their general-purpose counterparts, incorporating these processors into a modern system-on-chip (SoC) incurs a dramatic increase in the expertise, time, and effort to implement the system. In this talk, I will present my work on addressing these barriers to modern SoC design and implementation. Specifically, I will present my work on prototyping next-generation SoCs, creating an open-source design automation platform, and accelerating design automation algorithms.

About the Speaker: Dr. Austin Rovinski is a Postdoc at Cornell University and advised by Prof. Christopher Batten. Before Cornell, he obtained his Ph.D. from the University of Michigan in 2022. Austin works at the intersection of computer architecture, VLSI design, and electronic design automation (EDA). His research focuses on designing architectures, chips, and platforms for large scale, post-Moore systems-on-chip (SoCs). He is a founding member of the open-source EDA project OpenROAD and has published at top conferences including ASPLOS, VLSI, and ICCAD. Austin is a recipient of the Dwight F. Benton Fellowship and a Michigan EECS Outstanding Research Award.
Assured Machine Learning for Power Systems
Speaker: Dr. Yang Weng, Arizona State University
Date: Mon, Feb 20
Abstract: Deep penetration of distributed energy resources (DERs) calls for improved monitoring of power systems against reliability and security issues. For example, the low observability in some distribution grids makes monitoring DERs hard due to limited investment and vast coverage of distribution grids. Past methods proposed machine learning models with limited explainability. However, critical energy infrastructure needs assurance. For such a need, this talk shows how to design assured machine learning for power system monitoring via a twin structure of two learning agents, namely the AI-based and physics-guided models. The twins will collaborate adaptively to minimize the learning error while maximizing physical consistency. The structure ensures good generalization properties of the learned models. We then illustrate how twin models can be used to design cyber-attacks that can bypass chi-squared test on bad data without the system information, and discuss how to monitor and mitigate such attacks. Finally, we will demonstrate how the proposed methods are validated using our utility-connected hardware-in-the-loop microgrid.
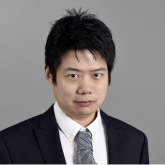
About the Speaker: Yang Weng received Ph.D. in Electrical and Computer Engineering from Carnegie Mellon University (CMU). Upon graduation, he joined Stanford University as a Postdoctoral Fellow at the Precourt Institute for Energy. He is currently an Assistant Professor in the School of Electrical, Computer, and Energy Engineering at Arizona State University (ASU). He is the consortium chair for Energy Cyber, a joint center established by the US Department of Energy and the Israel Ministry of Energy. Yang's research interests are power systems, data science, and cybersecurity. Yang received the NSF CAREER Award, AFOSR YIP Finalist Award, Amazon Research Award, Outstanding IEEE Young Professional Award, Outstanding Faculty Mentor Award, and Centennial Award for Teaching. Yang also received 9 Best Paper Awards, the Winner Award for International Competition on Innovation and Entrepreneurship, and the 2nd Place in Accuracy/1st Place in Speed for the RTE International Competition on "Learning to Run a Power Network" in 2019. His work is also recognized by ABB Fellowship, Stanford Tomcat Fellowship, and CMU Dean's Fellowship.
Intelligent Control and Optimization Techniques for Next-Generation Complex Dynamic Interconnected Systems
Speaker: Shirantha Welikala, University of Notre Dame
Date: Wed, Feb 22
Abstract: Complex dynamic interconnected systems arise in many emerging applications in areas like surveillance, patrolling, smart grid, supply chain networks, multi-robot systems, biochemical reactions, interacting populations, and epidemics. Therefore, developing intelligent control and optimization techniques targeting such interconnected systems is of prime importance to advance the state of the art across many application domains.
However, several persistent challenges in this endeavor include handling concerns related to complexity, scalability, uncertainty, resiliency, security, and optimality. To address these challenges, it is paramount that we: (1) Identify and exploit unique structural properties behind each interested class of problems, (2) Develop and use rigorous theoretical concepts, and (3) Specialize and deploy cutting-edge techniques in control, optimization, artificial intelligence, and interconnected systems.
To showcase this approach, in this talk, I will discuss a selected set of control and optimization techniques developed for several interesting interconnected systems applications. They include: (1) Off-line and on-line control techniques developed for a generic class of multi-agent persistent monitoring problems with applications in surveillance, patrolling, distributed sensing, etc., and (2) Decentralized and compositional analysis, distributed controller synthesis and interconnection topology synthesis techniques developed for generic linear and non-linear networked systems with applications in smart grid, supply chains, multi-robotic systems, etc. I will conclude this talk by outlining several future research plans toward addressing fundamental challenges and exploring emerging applications of complex dynamic interconnected systems.

About the Speaker: Shirantha Welikala is currently a Postdoctoral Research Fellow in the Department of Electrical Engineering, University of Notre Dame, South Bend, IN, USA. He received a B.Sc. degree in Electrical and Electronic Engineering from the University of Peradeniya, Peradeniya, Sri Lanka, in 2015. From 2015 to 2017, he was with the Department of Electrical and Electronic Engineering, University of Peradeniya, where he worked as a Temporary Instructor and subsequently as a Research Assistant. He received his M.Sc. and Ph.D. in Systems Engineering from Boston University, Brookline, MA, USA, in 2019 and 2021, respectively. His main research interests include control and optimization of cooperative multi-agent systems, analysis, controller synthesis and topology synthesis in large-scale networked systems, passivity-based control, symbolic control, robotics, data-driven control, machine learning, and smart-grid applications. He is a recipient of several awards and fellowships, including the 2015 Ceylon Electricity Board Gold Medal from the University of Peradeniya, a 2017 Dean’s Fellowship from Boston University, the 2019 and the 2022 President’s Award for Scientific Research in Sri Lanka, the 2021 Outstanding Ph.D. Dissertation Award in Systems Engineering from Boston University and the 2022 Best Paper Award at the 30th Mediterranean Conference on Control and Automation. For more information, please visit http://www.shiranthawelikala.com.
Autonomous Decision-Making: Adapt Fast, Counter Adversaries, and Resolve Conflicts
Speaker: Yue Yu, University of Texas at Austin
Date: Mon, Feb 27
Abstract: Can autonomous systems adapt to sudden and unexpected changes in the environment? Can they survive cyberattacks from adversaries? Can they resolve the conflicts among different decision-makers? To answer these questions, my research develops 1) trajectory optimization methods with computation speed that improves the state-of-the-art by orders of magnitude, 2) data poisoning attacks that expose the vulnerabilities of learning-based control methods, and 3) incentive mechanisms that mitigate the malicious competition in multiagent systems. These results contribute to the research in different areas—including optimization, control, learning, and game theory—and pave the way toward intelligent decision-making in robotics, transportation, and aerospace.
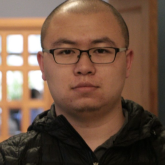
About the Speaker: Yue Yu is a postdoctoral research scholar with the Oden Institute for Computational Engineering and Sciences at The University of Texas at Austin. In 2021, Yue obtained his Ph.D. in Aeronautics and Astronautics from the University of Washington. Yue's research develops decision-making capabilities for autonomous systems. It contributes to multiple research areas, including optimization, learning, control, game theory, and transportation.
Architecting High Performance Silicon Systems for Accurate and Efficient On-Chip Deep Learning
Speaker: Thierry Tambe, Harvard University
Date: Tue, Feb 28
Abstract: The unabated pursuit for omniscient and omnipotent AI is levying hefty latency, memory, and energy taxes at all computing scales. At the same time, the end of Dennard scaling is sunsetting traditional performance gains commonly attained with reduction in transistor feature size. Faced with these challenges, my research is building a heterogeneity of solutions co-optimized across the algorithm, memory subsystem, hardware architecture, and silicon stack to generate breakthrough advances in arithmetic performance, compute density and flexibility, and energy efficiency for on-chip machine learning, and natural language processing (NLP) in particular. I will start, in the algorithm front, by discussing award-winning work on developing a novel floating-point based data type, AdaptivFloat, which enables resilient quantized AI computations; and is particularly suitable for NLP networks with very large parameter distribution. Then, I will describe a 16nm chip prototype that adopts AdaptivFloat in the acceleration of noise-robust AI speech and machine translation tasks – and whose fidelity to the front-end application is verified via a formal hardware/software compiler interface. Towards the goal of lowering the prohibitive energy cost of inferencing large language models on TinyML devices, I will describe a principled algorithm-hardware co-design solution, validated in a 12nm chip tapeout, that accelerates Transformer workloads by tailoring the accelerator's latency and energy expenditures according to the complexity of the input query it processes. Finally, I will conclude with some of my current and future research efforts on further pushing the on-chip energy-efficiency frontiers by leveraging specialized non-conventional dynamic memory structures for on-device training -- and recently prototyped in a 16nm tapeout.

About the Speaker: Thierry Tambe is a final year Electrical Engineering PhD candidate at Harvard University advised by Prof. Gu-Yeon Wei and Prof. David Brooks. His current research interests focus on designing energy-efficient and high-performance algorithms, hardware accelerators and systems for machine learning and natural language processing in particular. He also bears a keen interest in agile SoC design methodologies. Prior to debuting his doctoral studies, Thierry was an engineer at Intel in Hillsboro, Oregon, USA designing various mixed-signal architectures for high-bandwidth memory and peripheral interfaces on Xeon and Xeon-Phi HPC SoCs. He received a B.S. (2010) and M.Eng. (2012) in Electrical Engineering from Texas A&M University. Thierry Tambe is a recipient of the Best Paper Award at the 2020 ACM/IEEE Design Automation Conference, a 2021 NVIDIA Graduate PhD Fellowship, and a 2022 IEEE SSCS Predoctoral Achievement Award.
Active Sensing and Safe Stabilizing Control for ODE and PDE Systems
Speaker: Shumon Koga, University of California, San Diego
Date: Wed, Mar 1
Abstract: This talk will discuss control techniques for ODE and PDE systems with applications to robotics and energy systems. The first part of the talk will focus on active sensing, a problem in which sensor data collection needs to be controlled to maximize sensing performance. Planning the sensing trajectory of a mobile robot to explore and map an unknown environment is an example application in robotics. I will present an open-loop planning method for active mapping, a closed-loop control policy for active Simultaneous Localization and Mapping (SLAM), and a model-free reinforcement learning for active object localization, all of which are developed over continuous control space under a limited field of view in sensing. The second part of the talk will consider control synthesis for ODE and PDE systems with stability and safety guarantees. Quadratic programming with control Lyapunov function (CLF) and control barrier function (CBF) constraints has become a widely adopted technique for enforcing stability and safety constraints. However, CLF-CBF-QP methods introduce undesired equilibria on the boundary of the safe set. I propose a new formulation based on a differential complementarity problem to design controllers which avoid many of the undesired equilibria introduced by the CLF-CBF-QP approach. Finally, I will discuss control and estimation techniques for PDE models of thermal and electrical energy storage systems that exhibit phase change, known as the Stefan Problem. I propose a novel infinite-dimensional backstepping control approach to exactly store the desired amount of energy, which achieves provable stability, robustness, and safety.

About the Speaker: Shumon Koga is a Postdoctoral Scholar in Electrical and Computer Engineering at the University of California, San Diego. He works with Professor Nikolay Atanasov in the Existential Robotics Laboratory. He received the Ph.D. degree in Mechanical and Aerospace Engineering from the University of California, San Diego in 2020, under the supervision of Professor Miroslav Krstic. He was an intern at NASA Jet Propulsion Laboratory and Mitsubishi Electric Research Laboratories. He received the Robert E. Skelton Systems and Control Dissertation Award in 2020, the O. Hugo Schuck Best Paper Award in 2019, and the Outstanding Graduate Student Award in 2018.
Energy Autonomous Integrated SoCs: Towards Next-Generation Ubiquitous Connectivity and Sensing
Speaker: Hamed Rahmani, IBM T.J. Research Center
Date: Thu, Mar 2
Abstract: System-on-chip (SoC) solutions have the potential to enable low-cost, eco-friendly, and scalable communication/sensing platforms for the next generation of wireless networks. The evolution of wireless technology to 5G and beyond will increase the number of connected devices at an unprecedented rate. On the other hand, the existing electronics/optics do not meet the performance requirements of future technologies, e.g., energy efficiency, power handling, bandwidth, and batteryless operation. In this talk, I highlight how custom silicon Integrated Circuits (ICs) can build the foundation for energy-autonomous, reconfigurable, and scalable SoC platforms from microwave to millimeter-wave (mm-Wave)/sub-terahertz (THz) frequencies. To this end, I present integrated solutions for 1- Low-power ubiquitous medical and IoT sensing. 2- High-power signal generation at sub-6GHz and mmWave frequency range, and 3- High-speed and energy-efficient wireline connectivity. First, I introduce batteryless wireless SoCs capable of harvesting energy from ambient and dedicated energy sources. I adopt an antenna-to-system integration approach and address the challenges of next-generation wirelessly powered integrated systems. For the first time, I present a fully integrated high-performance data transceiver with a power harvesting platform under severe power constraints and small form factors offering a broad scope of applications, including medical implants, point-of-care diagnostic, ubiquitous sensing, and localization. I provide my vision for the future of integrated electronics/optics beyond the limits of SoCs that will transform the next decade of connectivity and high-performance computing and conclude this talk.

About the Speaker: Hamed Rahmani received a Ph.D. degree from the University of California Los Angeles (UCLA) in 2020 and an M.Sc. degree from Rice University in 2017, both in electrical and computer engineering. He completed his B.Sc. degree at the Sharif University of Technology in Tehran, Iran in Electrical Engineering in 2014.
He has been with IBM T. J. Research Center in Yorktown Heights, NY since 2022 and is currently a Research Staff Member working on research projects investigating Mixed-Signal CMOS circuits for high-speed electrical and optical data communication. He is also an Adjunct Professor at Columbia University in New York, NY, where he has taught graduate-level courses in analog and RF circuit design. He was also a visiting lecturer at the ECE department of Princeton University in the Fall of 2022 where he taught a graduate-level course on RFIC design. From 2020 to 2022, He was a senior RFIC design engineer at Qualcomm Inc., Boxborough, MA where he focused on advanced 5G transmitters for cellular applications and RF front-end designs. His Ph.D. thesis was focused on wirelessly powered solutions based on low-power integrated systems and circuits for biomedical applications and IoT sensors. His research focus includes high-speed mm-wave wireline and wireless integrated circuits and low-power integrated system-on-chip solutions for biomedical implants and IoT sensors.
Dr. Rahmani received several awards and fellowships including the IEEE MTT-S Graduate Fellowship for medical applications and the Texas Instruments Distinguished fellowship. He serves on the technical committee for the International Microwave Symposium (IMS) 2022. Also, he is a member of "MTT-26: RFID, wireless sensors and IoT" and an affiliate member of " MTT-25: wireless power transfer and energy conversion" technical committees of the IEEE Microwave Theory and Techniques Society.
Enabling Self-Sufficient Robot Learning
Speaker: Rika Antonova, Stanford University
Date: Thu, Mar 2
Abstract: Autonomous exploration and data-efficient learning are important ingredients for handling the complexity and variety of real-world interactions. In this talk, I will describe methods that provide these ingredients and serve as building blocks for enabling self-sufficient robot learning.
First, I will outline a family of methods that facilitate active global exploration. Specifically, they enable ultra data-efficient Bayesian optimization in reality by leveraging experience from simulation to shape the space of decisions. In robotics, these methods enable success with a budget of only 10-20 real robot trials for a range of domains: bipedal and hexapod walking, task-oriented grasping, and nonprehensile manipulation.
Next, I will describe how to bring simulations closer to reality. This is especially important for scenarios with highly deformable objects, where simulation parameters influence the dynamics in unintuitive ways. Here, adaptive distribution embeddings allow incorporating noisy state observations into modern Bayesian tools for simulation parameter inference. This novel representation ensures success in estimating posterior distributions over simulation parameters, such as elasticity, friction, and scale, even for highly deformable objects and using only a small set of real-world trajectories.
Lastly, I will share a vision of using distribution embeddings to make the space of stochastic policies in reinforcement learning suitable for global optimization. This direction involves formalizing and learning novel distance metrics and will support principled ways of seeking diverse behaviors. This can unlock truly autonomous learning, where learning agents have incentives to explore, build useful internal representations, and discover a variety of effective ways of interacting with the world.

About the Speaker: Rika Antonova is a postdoctoral scholar at Stanford University at the Interactive Perception and Robot Learning lab. I received NSF Computing Innovation Fellowship for research on active learning of transferable priors, kernels, and latent representations for robotics. I completed my Ph.D. work on data-efficient simulation-to-reality transfer at KTH. Earlier, I completed my research Master's degree at the Robotics Institute at Carnegie Mellon University, where I developed Bayesian optimization approaches for robotics and personalized tutoring systems. Prior to that, I was a software engineer at Google, first in the Search Personalization group and then in the Character Recognition team (developing open-source OCR engine Tesseract).
Aligning Robot and Human Representations
Speaker: Andreea Bobu, University of California Berkeley
Date: Wed, Mar 8
Abstract: To perform tasks that humans want in the world, robots rely on a representation of salient task features; for example, to hand me a cup of coffee, the robot considers features like efficiency and cup orientation in its behavior. Prior methods try to learn both a representation and a downstream task jointly from data sets of human behavior, but this unfortunately picks up on spurious correlations and results in behaviors that do not generalize. In my view, what’s holding us back from successful human-robot interaction is that human and robot representations are often misaligned: for example, our lab’s assistive robot moved a cup inches away from my face -- which is technically collision-free behavior -- because it lacked an understanding of personal space. Instead of treating people as static data sources, my key insight is that robots must engage with humans in an interactive process for finding a shared representation for more efficient, transparent, and seamless downstream learning. In this talk, I focus on a divide and conquer approach: explicitly focus human input on teaching robots good representations before using them for learning downstream tasks. This means that instead of relying on inputs designed to teach the representation implicitly, we have the opportunity to design human input that is explicitly targeted at teaching the representation and can do so efficiently. I introduce a new type of representation-specific input that lets the human teach new features, I enable robots to reason about the uncertainty in their current representation and automatically detect misalignment, and I propose a novel human behavior model to learn robust behaviors on top of human-aligned representations. By explicitly tackling representation alignment, I believe we can ultimately achieve seamless interaction with humans where each agent truly grasps why the other behaves the way they do.
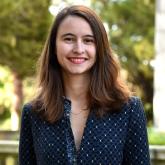
About the Speaker: Andreea Bobu is a Ph.D. candidate at the University of California Berkeley in the Electrical Engineering and Computer Science Department advised by Professor Anca Dragan. Her work is at the intersection of robotics, machine learning, and mathematical human modeling. Specifically, Andreea studies algorithmic human-robot interaction, with a focus on how autonomous agents and humans can efficiently and interactively arrive at shared representations of their tasks for more seamless and reliable interaction. Prior to her Ph.D. she earned her Bachelor’s degree in Computer Science and Engineering from MIT in 2017. She is the recipient of the Apple AI/ML Ph.D. fellowship, is a Rising Star in EECS and an R:SS and HRI Pioneer, has won best paper award at HRI 2020, and has interned at NVIDIA Research.
Light in Artificial Intelligence: Hardware/Software Co-Design for Photonic Machine Learning Computing
Speaker: Jiaqi Gu, University of Texas at Austin
Date: Wed, Mar 15
Abstract: The proliferation of big data and artificial intelligence (AI) has motivated the investigation of next-generation AI computing hardware to support massively parallel and energy-hungry machine learning (ML) workloads. Photonic computing, or computing using light, is a disruptive technology that can bring orders-of-magnitude performance and efficiency improvement to AI/ML with its ultra-fast speed, high parallelism, and low energy consumption. There has been growing interest in using nanophotonic processors for performing optical neural network (ONN) inference operations, which can make transformative impacts in future datacenters, automotive, smart sensing, and intelligent edge. However, the substantial potential in photonic computing also brings significant design challenges, which necessitates a cross-layer co-design stack where the circuit, architecture, and algorithm are designed and optimized in synergy.
In this talk, I will present my exploration to address the fundamental challenges faced by optical AI and to pioneer a hardware/software co-design methodology toward scalable, reliable, and adaptive photonic neural accelerator designs. First, I will delve into the critical area scalability issue of integrated photonic tensor units and present specialized photonic neural engine designs with domain-specific customization that significantly “compresses” the circuit footprint while realizing comparable inference accuracy. Next, I will present efficient on-chip training frameworks to show how to build a self-learnable photonic accelerator and overcome the robustness and adaptability bottlenecks by directly training the photonic circuits in situ. Then, I will introduce how to close the virtuous cycle between photonics and AI by applying AI/ML to photonic device simulation. In the end, I will conclude the talk with future research directions of emerging domain-specific photonic AI hardware with an intelligent end-to-end co-design & automation stack and deploying it to support real-world applications.

About the Speaker: Jiaqi Gu is a final-year Ph.D. candidate in the Department of Electrical and Computer Engineering at The University of Texas at Austin, advised by Prof. David Z. Pan and co-advised by Prof. Ray T. Chen. Prior to UT Austin, he received his B.Eng. from Fudan University, Shanghai, China, in 2018. His research interests include emerging post-Moore hardware design for efficient computing, hardware/software co-design, photonic machine learning, and AI/ML algorithms.
He has received the Best Paper Award at the ACM/IEEE Asian and South Pacific Design Automation Conference(ASP-DAC) in 2020, the Best Paper Finalist at the ACM/IEEE Design Automation Conference (DAC) in 2020, the Best Poster Award at the NSF Workshop for Machine Learning Hardware Breakthroughs Towards Green AI and Ubiquitous On-Device Intelligence in 2020, the Best Paper Award at the IEEE Transaction on Computer-Aided Design of Integrated Circuits and Systems (TCAD) in 2021, the ACM Student Research Competition Grand Finals First Place in 2021, and Winner of the Robert S. Hilbert Memorial Optical Design Competition in 2022.
Co-optimize DNN Arithmetics and Hardware System for Efficient Inference and Training
Speaker: Sai Qian Zhang, Meta Reality Labs
Date: Mon, Mar 20
Abstract: In recent years, we have seen a proliferation of sophisticated Deep Neural Network (DNN) architectures that have achieved state-of-the-art performances across a variety of domains. However, the algorithmic superiority of DNNs levies high latency and energy taxes at all computing scales, which further poses significant challenges to the hardware platforms executing them. Given the fact that the DNN architectures and the hardware platform executing them are tightly coupled and tangled, my research lies in building a full-stack solution to co-optimize DNN across the architecture, datatype and supporting hardware system to achieve efficient inferences and training operations.
In this talk, I will first describe Column-Combining, an innovative pruning strategy that packs sparse filter matrices into a denser format for efficient deployment in a novel systolic architecture with nearly perfect utilization rate. Following that, I will then describe a bit-level quantization method named Term Quantization (TQ). Unlike the conventional quantization methods that operate on individual values, Term Quantization is a group- based method that keeps a fixed number of largest terms (nonzero bits in the binary representations) within a group of values, and this in turn leads to a significantly smaller amount of quantization error compared to other quantization approaches under the same bitwidth. Next, I will introduce the work I have done to facilitate the DNN training process. In particular, I will describe the Fast First, Accurate Second Training (FAST) system that adaptively adjusts the precision of the DNN operands for efficient DNN training. Last but not least, I will conclude with some of my recent research efforts and future research plans on further extending the frontiers of the DNN training hardware efficiency by leveraging the underlying reversibility of the DNN architecture.

About the Speaker: Sai Qian Zhang is a research scientist at Meta Reality Labs. He also holds an appointment as research associate at Harvard University hosted by Prof. David Brooks and Prof. Gu-Yeon Wei. Sai received his Ph.D. degree from Harvard University under the supervision of Prof. H.T. Kung in 2021, and obtained both of his M.A.Sc and B.A.Sc degrees from University of Toronto.
Sai’s research interest lies in algorithm and hardware codesign for the efficient deep neural network implementation. He is also interested in multi-agent reinforcement learning and its applications on hardware system design. His works have been published in multiple top-tier conferences such as ASPLOS, NeurIPS, HPCA, and AAAI. He has won the best paper award at IEEE international conference on Communication.
Foundations First: Improving C’s Viability in Introductory Programming Courses with the Debugging C Compiler
Speaker: Jake Renzella, University of New South Wales, Sydney : Sasha Vassar, University of New South Wales, Sydney
Date: Tue, Mar 21
Abstract: We present The Debugging C Compiler (DCC), a system that composes a suite of compilers with static and dynamic analysis tools to support introductory C programming students. Using C in our introductory computing courses exposes students to low-level mechanics of the operating system, such as pointers and manual memory management — concepts critical in establishing a solid foundation of computing. Unlike typical C implementations, DCC provides programmers with enhanced, approachable run- and compile-time checking and messages. DCC clarifies C’s cryptic operating system errors such as segmentation faults and alleviates the need for students to analyse memory dumps and tackle undefined behaviours. This paper describes DCC’s implementation and features and measures the tool’s efficacy in aiding novice C programmers. We further present our deep reflections on how DCC has successfully allowed us to use C in our large introductory programming courses, with an estimated five million compilations to date. Our research also outlines avenues for future work, which we hope will support others in delivering a foundations-first approach to introductory programming.
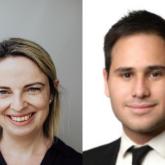
About the Speaker: Jake Renzella:
Jake is a Lecturer (Asst. Professor) and Co-Head of the Computing and Education research group in the School of Computer Science Engineering at the University of New South Wales, Sydney.
Jake’s research is at the intersection of software and artificial intelligence-based systems to support computing education. Jake’s work has been published in premier conferences and journals such as SIGCSE-TS, and the International Conference on Software Engineering. More importantly, it is embedded in open-source education projects such as DCC, SplashKit, and notably, Formatif, used at several Australian and New Zealand universities with over 230,000 users.
Jake is an Associate Fellow of the Higher Education Academy, an Early Career Academic member of the Australasian Association for Engineering Education, and was a recipient of a 2022 UNSW Teaching Excellence award.
Sasha Vassar:
Sasha's background spans multiple disciplines, including Computer Engineering, Biomedical Engineering, and a Ph.D. in Education from UNSW. Prior to joining the School of Computer Science and Engineering as a Lecturer (Asst. Prof), she worked in the engineering industry, focusing on improving problem-solving and design processes. Sasha's passion for education and teaching drew her back to the university, where she now specializes in CS1 education, the role of design thinking in engineering problem-solving, and the application of cognitive load theory concepts to improve pedagogy across the degree.
Sasha is an Associate Fellow of the Higher Education Academy, and her dedication to teaching excellence was recognized with the 2022 UNSW Teaching Excellence Award.
Investigations of materials and metasurfaces for solar cells efficiency optimization
Speaker: Ana Barar, Polytechnic University of Bucharest
Date: Thu, Mar 23
Abstract: Photovoltaic technology has established itself as one of the main contenders to replace fossil fuels as the principal source of energy, due to worldwide accessibility of sunlight, and the minimal impact this technology has on the environment. Extensive research has been conducted in the fields of materials science, metasurfaces engineering, and optics, which has led to an increase in the efficiency of solar cells from 14% to 40%. This enhancement has been made possible by means of doping, device structure optimization, or addition of light-trapping surfaces. This talk explores a series of studies and simulations carried out on material properties, metasurface designs, and device structures, with the purpose of solar cells efficiency optimization.

About the Speaker: Ana Barar (she/her) is a tenured Lecturer of Materials Science for Electronics, at the Polytechnic University of Bucharest, Romania. She has a PhD in Electronics Engineering, from the same university. Her research interests include design and simulation of metasurfaces for RF and optical applications, organic/inorganic materials for solar cells, simulation and theoretical analysis of excitonic solar cells.
Protecting Privacy Through Metadata Analysis
Speaker: Sandra Siby, Imperial College, London
Date: Thu, Mar 23
Abstract: Metadata, or 'data about data' can be a rich source of details and context, revealing information about a user even in the presence of encryption. In this talk, I will discuss ways in which we can use metadata analysis to protect privacy at the network and web level. First, I will demonstrate how metadata analysis can be used by adversaries to perform website-fingerprinting attacks against newly-standardized networking protocols, and how we can develop defenses against such attacks. Second, I will describe how we can use the difficulty in hiding metadata to build robust systems for detecting online advertising and tracking services. Finally, I will outline my vision to build privacy frameworks for understudied scenarios.
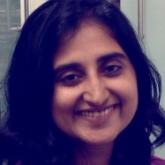
About the Speaker: Sandra Siby is a Research Associate at Imperial College London, working with Prof. Hamed Haddadi. She recently completed her PhD at EPFL, advised by Prof. Carmela Troncoso. She is interested in the areas of network and web privacy. Prior to her PhD, she worked in IoT network security, sensor networks, and delay-tolerant networks. She obtained her masters from ETH Zurich, and her bachelors from the National University of Singapore.
Engineering Intelligent Physical Human-Robot Interactions
Speaker: Keya Rajesh Ghonasgi, University of Texas at Austin
Date: Thu, Mar 27
Abstract: Technology for human use capitalizes on our ability to learn from interactions with the environment. Robotics technology has advanced significantly in the past few decades making physical human-robot interaction (HRI) a safe and promising new mode through which humans can learn and act upon their environment. At the same time, advances in artificial intelligence (AI) have provided us with frameworks for how robotic devices can control their behavior at a high level. As a result, we can now harness both human learning and robot learning abilities to engineer meaningful physical interactions that go beyond conventional technological solutions. In this talk, I will explore how physical HRI can be interpreted through the lens of neuroscience and translated into engineering solutions that can intelligently affect the human-robot system's behavior. I begin with a deep dive into human-exoskeleton interaction for motor training protocols using a curriculum learning-based approach. In particular, I will address the challenges in human data interpretation, exoskeleton control, and curriculum design. Additionally, I will examine how the fields of engineering design, AI, and neuroscience can be simultaneously leveraged to engineer effective physical interactions across a variety of potential HRI applications.

About the Speaker: Keya Ghonasgi is a doctoral candidate in the Mechanical Engineering department at the University of Texas at Austin (UT). Her research vision is to harness human and robot learning abilities to engineer intelligent human-robot interactions with applications including assistance, training, and augmentation. At UT Austin, she is a member of the Rehabilitation and Neuromuscular Robotics lab directed by Dr. Ashish Deshpande and collaborates with the Learning Agents Research Group directed by Dr. Peter Stone. In 2018, she earned her M.S. in Mechanical Engineering from Columbia University under Dr. Sunil Agrawal's guidance. Keya has been recognized as a 2022 Rising Star in ME and a 2023 CalTech Young Investigator. Her research has been supported through a graduate fellowship awarded by UT Austin (2022-23), an NSF M3X grant, and research collaborations with Meta Reality Labs and Google Brain.
Renewable Energies for Power Systems
Speaker: Edris Pouresmaeil, Aalto University, Finland
Date: Thu, Mar 27
About the Speak: My name is Edris Pouresmaeil, and I am a professor of Renewable Energies at Aalto University, located in Finland. In my presentation at NYU, I will delve into my extensive research endeavors and ongoing projects related to the integration of renewable energy sources (RESs) and energy storage systems (ESSs) within power grids.
My primary research focus is centered on the development and implementation of innovative techniques to facilitate the large-scale deployment of renewable energies in power grids, thereby increasing their efficiency, reliability, resilience, and stability. Such efforts align with the global initiative to transition towards green energy and carbon neutrality.

My research findings have been published in reputable journals and conferences, and I have secured funding from distinguished entities such as the European Commission, Business Finland, the Academy of Finland, and numerous national and international industrial sectors.
Furthermore, during the presentation, I will discuss my teaching philosophy and experience, as well as my efforts towards student supervision and mentoring. I will also explore the societal impacts of my research, potential funding avenues, and collaborators. Lastly, I will outline my future plans for teaching and research.
Modern AI Series: Online Learning, Bandits, and Digital markets
Speaker: Nicolò Cesa-Bianchi, University of Milan and Polytechnic University of Milan
Date: Tue, Mar 28
Abstract: Online learning is concerned with the study of algorithms that learn sequentially through repeated interactions with an unknown environment. The goal is to understand how fast an agent can learn depending on the information received from the environment. Digital markets, with their complex ecosystems of algorithmic agents, provide countless examples of sequential decision-making problems with different utility functions and types of learning feedback. In the talk, after tracing the roots and the main algorithmic ideas behind online learning, we will show how solving problems arising from digital markets has improved our understanding of what machine learning algorithms can do.

About the Speaker: Nicolò Cesa-Bianchi is professor of Computer Science at the University of Milan (Italy), where he leads the laboratory of artificial intelligence and learning algorithms. He also holds a joint appointment at Polytechnic University of Milan (Italy). His main research interests are the design and analysis of machine learning algorithms for online learning, sequential decision-making, and graph analytics. He is co-author of the monographs "Prediction, Learning, and Games" and "Regret Analysis of Stochastic and Nonstochastic Multi-armed Bandit Problems". He served as President of the Association for Computational Learning and co-chaired the program committees of some of the most important machine learning conferences. He is the recipient of a Google Research Award, a Xerox Foundation Award, a Criteo Faculty Award, a Google Focused Award, and an IBM Research Award. He is ELLIS fellow, member of the ELLIS board, and co-director of the Milan ELLIS unit.
Neural Mechanisms of attention and speech perception in complex, spatial acoustic environment
Speaker: Prachi Patel, Columbia University
Date: Wed, Mar 29
Abstract: We can hold conversations with people in environments where typically there are additional simultaneous talkers in background acoustic space or noise like vehicles on the street or music playing at a café on the sidewalk. This seemingly trivial everyday task is difficult for people with hearing deficits and is extremely hard to model in machines. This talk focuses on exploring the neural mechanisms of how the human brain encodes such complex acoustic environments and how cognitive processes like attention shapes processing of the attended speech.
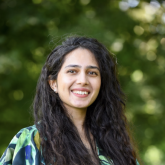
About the Speaker: Prachi Patel is a recent PhD graduate from Columbia University. Her research focuses on how the human brain makes sense of speech in complex multi-talker settings. She uses her background in Electrical Engineering and works with invasive and non-invasive neural recordings to uncover this scientific question. Her work has been published in current biology, cell reports and jNeuro.
Architectures, Compilers, and Hardware Security for Neuromorphic Computing-in-Memory Systems
Speaker: Jan Moritz Joseph, RWTH Aachen University
Date: Tue, Apr 4
Abstract: Recently, memory technologies emerged that enable data storage and computation within the same hardware block. This Computing-In-Memory (CIM) technology enables fundamentally novel architectures not limited by data movements. There are different technology contenders, e.g., Resistive Random Access Memory (RRAM) that can be used for efficient matrix-vector multiplications in memory, accelerating the dominant operations in ML inference and training. Therefore, CIM technology is one cornerstone of novel computing concepts to achieve more efficient and pervasive AI, disrupting its application and bringing complex models to the end user. This talk will summarize the current state-of-the-art for CIM edge-AI accelerators to motivate their key advantage. We will also discuss this technology's challenges before mass-market adoption is possible. Then, the talk will focus on three challenges for these systems: compute-in-memory architectures, AI compilers and hardware security. In the final part of the talk, we will introduce our proposed solution for an integrated system design. We are convinced that efficient, widely adopted CIM systems will only be possible if they are integrated into existing edge-AI software stacks. Otherwise, seamless and risk-free migration from existing CMOS technology will not be attractive or realistic for companies, even though emerging memories offer substantial power savings. Therefore, it is imperative to provide an ecosystem of software and hardware development kits that work with existing edge-ML tools.

About the Speaker: Dr. Joseph holds a tenured position as senior researcher at the RWTH Aachen University. He leads a team researching compilers, architectures, and security for computing-in-memory-based edge AI accelerators. He and his team won the RWTH innovation award for the universities best transfer-to-industry project in 2022. From 2020 to 2022, he was a postdoctoral research fellow in Aachen and led an industry project on parallel simulation with gem5. From 2019 to 2020 Dr. Joseph was a visiting researcher at Dr. Krishna’s Synergy Lab at Georgia Institute of Technology, Atlanta, GA. He worked on architectures for AI accelerators. For his PhD on "Networks-on-Chip for heterogeneous 3D Systems-on-Chip" he received the award for the best PhD thesis from the Faculty of Electrical Engineering and Information Technology at Otto-von-Guericke Universität Magdeburg, Germany in 2020.
When Laser meets Surgery: An update to MIRACLE project
Speaker: Azhar Zam, NYU Abu Dhabi
Date: Fri, Apr 7
Abstract: The need for non-invasive, affordable, and label-free sensing and imaging techniques for both diagnosis and treatment monitoring has sparked a renewed interest in the potential of light-based sensing and imaging. These smart technologies, which combine advanced optical methods and AI, can detect, measure, and quantify what is otherwise invisible, addressing unmet needs in biology and medicine. In this presentation, Dr. Zam will provide an overview of the development of smart devices that utilize novel optical technologies, from early diagnosis to providing real-time feedback for laser surgery. He will also share the latest updates on the MIRACLE project and offer his insights on developing light-based smart technologies at NYUAD. By leveraging the power of light-based sensing and imaging, we can unlock new possibilities in healthcare, enabling earlier and more accurate diagnoses, more effective treatments, and better patient outcomes.
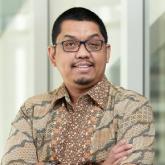
About the Speaker: Dr. Zam joined NYU Abu Dhabi in the fall of 2022 as an Associate Professor of Bioengineering, where he leads the cutting-edge Laboratory for Advanced Bio-Photonics and Imaging (LAB-π).
He is also an Associated Faculty with the Department of Biomedical Engineering at NYU Tandon School of Engineering. Dr. Zam's impressive academic background includes a B.S. from the University of Indonesia, an M.Sc. from the University of Luebeck, Germany, and a Ph.D. from Friedrich-Alexander-University Erlangen-Nuremberg, Germany. Before joining NYUAD, Dr. Zam was an Assistant Professor at the Department of Biomedical Engineering, University of Basel, Switzerland, where he founded the Biomedical Laser and Optics Group (BLOG) and co-established the MIRACLE II flagship project. He has also held research positions at prestigious institutions such as the University of Waterloo, the National University of Ireland Galway, the Toronto Metropolitan University (formerly known as Ryerson University), and the University of California at Davis. Dr. Zam's contributions include over 90 peer-reviewed articles and book chapters to his name, as well as several patents. Overall, Dr. Zam's academic background and extensive research experience make him a valuable addition to the NYU Abu Dhabi community, and his leadership of LAB-π is sure to bring exciting new developments to the field of Biophotonics and Imaging.
Insights and new questions for machine and natural learning of spatial intelligence in robots and animals
Speaker: Michael Milford, Queensland University of Technology
Date: Fri, Apr7
Abstract: Our lab has spent the past two decades bridging the divide between our understanding of the neuroscience and behaviour underlying animal mapping, localization and perception systems, and creating their high performance technological equivalents for robots and autonomous vehicles. In this talk I will cover some of the key insights we’ve discovered from these very different research endeavours, in particular in going all the way from theoretical models of neural systems to high performance, deployable technology. Our quest to create reliable, introspective mapping and positioning systems for robots has also cast a light on the limited utility of the performance metrics so strongly favoured in current computer science research, that both challenge our concepts of how we conduct research, and reframe how we might think about analysing the performance of natural animal systems. I’ll also introduce the robot and autonomous system technologies our QUT Centre for Robotics creates, that fly through the skies, move through and under the sea, and drive across the land: on-road, off-road, and underground.
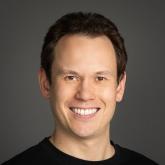
About the Speaker: Professor Milford conducts interdisciplinary research at the boundary between robotics, neuroscience, computer vision and machine learning, and is a multi-award winning educational entrepreneur. His research models the neural mechanisms in the brain underlying tasks like navigation and perception to develop new technologies in challenging application domains such as all-weather, anytime positioning for autonomous vehicles. From 2022 – 2027 he is leading a large research team combining bio-inspired and computer science-based approaches to provide a ubiquitous alternative to GPS that does not rely on satellites. He is also one of Australia’s most in demand experts in technologies including self-driving cars, robotics and artificial intelligence, and is a passionate science communicator. He currently holds the position of Joint Director of the QUT Centre for Robotics, Australian Research Council Laureate Fellow, Professor at the Queensland University of Technology, and is a Microsoft Research Faculty Fellow and Fellow of the Australian Academy of Technology and Engineering.
His research has helped attract more than 48 million dollars in research and industry funding for fellowships and team projects. Michael’s papers have won (6) or been finalists (9) for 15 best paper awards including the 2012 ICRA Best Vision paper. His citation h-index is 48, with 11,836 citations as of March 2023. Michael has dual Australian-US citizenship and has lived and worked in locations including Boston, Edinburgh and London. He has led or co-led projects and research collaborating with leading global organizations including Amazon, Google, Intel, Ford, Rheinmetall, Air Force Office of Scientific Research, NASA, Harvard, Oxford and MIT.
Michael has given more than 250 keynotes, plenaries and invited presentations at major industrial corporations (Google, Amazon, Microsoft, Toyota, OpenAI, Uber), top universities (including Harvard University, MIT, Oxford University, CMU, Imperial College London, Cambridge), international conferences, workshops and scientific meetings across thirteen countries to audiences of up to 2000 people. His work has been recognized by many international and national awards including the 2019 Batterham Medal for Engineering Excellence, the 2015 Queensland Young Tall Poppy Scientist of the Year award and a Microsoft Research Faculty Fellowship. He was recently awarded a $2.7M Australian Research Council Laureate Fellowship, the premier Australian fellowship scheme, and is one of the youngest recipients in the program’s history.
As a lifelong educational entrepreneur, Michael has written innovative textbooks, novels and storybooks (20 titles to date) for early childhood, primary and high school audiences, and has collaborated with the major movie studio representatives to write a regular “science in the movies” review series. His company Math Thrills combines mass market entertainment and STEM education, is funded from Kickstarter, QUTBluebox and the AMP Foundation, and has been recognized through honours including being a Reimagine Education Awards finalist, a TedXQUT talk and World Science Festival event. His company has sold in 35 countries to date with recent titles including the Complete Guide to Autonomous Vehicles for Kids… and Everyone Else, STEM Storybook, The Complete Guide to Artificial Intelligence for Kids, Robot Revolution and Rachel Rocketeer.
We're (finally) getting Dexterous Robotic Manipulation. Now what?
Speaker: Matei Ciocarlie, Columbia University
Date: Wed, Apr 19
Abstract: At long last, robot hands are becoming truly dexterous. It took advances in sensor design, mechanisms, and computational motor learning all working together, but we’re finally starting to see true dexterity, in our lab as well as others. This talk will focus on the path our lab took to get here, and questions for the future. From a mechanism design perspective, I will present our work on optimizing an underactuated hand transmission mechanism jointly the grasping policy that uses it, an approach we refer to as “Hardware as Policy”. From a sensing perspective, I will present our optics-based tactile finger, providing accurate touch information over a multi-curved three-dimensional surface with no blind spots. From a motor learning perspective, I will talk about learning tactile-based policies for dexterous in-hand manipulation and object recognition. Finally, we can discuss implications for the future: how do we consolidate these gains by making dexterity more robust, versatile, and general, and what new applications can it enable?
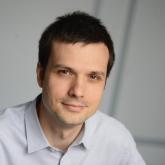
About the Speaker: Matei Ciocarlie is an Associate Professor in the Mechanical Engineering Department at Columbia University, with affiliated appointments in Computer Science and the Data Science Institute. His work focuses on robot motor control, mechanism and sensor design, planning and learning, all aiming to demonstrate complex motor skills such as dexterous manipulation. Matei completed his Ph.D. at Columbia University in New York; before joining the faculty at Columbia, Matei was a Research Scientist and then Group Manager at Willow Garage, Inc., and then a Senior Research Scientist at Google, Inc. In these positions, Matei contributed to the development of the open-source Robot Operating System (ROS), and led research projects in areas such as hand design, manipulation under uncertainty, and assistive robotics. In recognition of his work, Matei was awarded the Early Career Award by the IEEE Robotics and Automation Society, a Young Investigator Award by the Office of Naval Research, a CAREER Award by the National Science Foundation, and a Sloan Research Fellowship by the Alfred P. Sloan Foundation.
Distributed Fault Diagnosis of Interconnected Cyber-Physical Systems
Speaker: Marios M. Polycarpou, KIOS Research and Innovation Center of Excellence
Date: Wed, Apr 19
Abstract: The emergence of interconnected cyber-physical systems and sensor/actuator networks has given rise to advanced automation applications, where a large amount of sensor data is collected and processed in order to make suitable real-time decisions and to achieve the desired control objectives. However, in situations where some components behave abnormally or become faulty, this may lead to serious degradation in performance or even to catastrophic system failures, especially due to cascaded effects of the interconnected subsystems. Distributed fault diagnosis refers to monitoring architectures where the overall system is viewed as an interconnection of various subsystems, each of which is monitored by a dedicated fault diagnosis agent that communicates and exchanges information with other “neighboring” agents. The goal of this presentation is to provide insight into various aspects of the design and analysis of intelligent monitoring and control schemes and to discuss directions for future research.

About the Speaker: Marios Polycarpou is a Professor of Electrical and Computer Engineering and the Director of the KIOS Research and Innovation Center of Excellence at the University of Cyprus. He is also a Founding Member of the Cyprus Academy of Sciences, Letters, and Arts, an Honorary Professor of Imperial College London, and a Member of Academia Europaea (The Academy of Europe). He received the B.A degree in Computer Science and the B.Sc. in Electrical Engineering, both from Rice University, USA in 1987, and the M.S. and Ph.D. degrees in Electrical Engineering from the University of Southern California, in 1989 and 1992 respectively. His teaching and research interests are in intelligent systems and networks, adaptive and learning control systems, fault diagnosis, machine learning, and critical infrastructure systems.
Prof. Polycarpou is the recipient of the 2023 IEEE Frank Rosenblatt Technical Field Award and the 2016 IEEE Neural Networks Pioneer Award. He is a Fellow of IEEE and IFAC. He served as the President of the IEEE Computational Intelligence Society (2012-2013), as the President of the European Control Association (2017-2019), and as the Editor-in-Chief of the IEEE Transactions on Neural Networks and Learning Systems (2004-2010). Prof. Polycarpou currently serves on the Editorial Boards of the Proceedings of the IEEE and the Annual Reviews in Control. His research work has been funded by several agencies and industry in Europe and the United States, including the prestigious European Research Council (ERC) Advanced Grant, the ERC Synergy Grant and the EU-Widening Teaming program.
Statistical Graph Signal Processing with Applications to Smart Grids
Speaker: Tirza Routtenberg Ben-Gurion, University of the Negev, Israel
Date: Thu, Apr 20
Abstract: Graphs are fundamental mathematical structures that are widely used in various fields for network data analysis to model complex relationships within and between data, signals, and processes. In particular, graph signals arise in many modern applications, leading to the emergence of the area of graph signal processing (GSP) in the last decade. GSP theory extends concepts and techniques from traditional digital signal processing (DSP) to data indexed by generic graphs, including the graph Fourier transform (GFT), graph filter design, and sampling and recovery of graph signals. However, most of the research effort in this field has been devoted to the purely deterministic setting. In this study, we consider the extension of statistical signal processing (SSP) theory by developing graph SSP (GSSP) methods and bounds. Special attention will be given to the development of GSP methods for monitoring the power systems, which has significant practical importance, in addition to its contribution to the enrichment of theoretical GSSP tools. In particular, we will discuss the following problems (as time permits): 1) Bayesian estimation of graph signals in non-linear models; 2) the identification of edge disconnections in networks based on graph filter representation; 3) the development of performance bounds, such as the well-known Cramér-Rao bound (CRB), on the performance in various estimation problems that are related to the graph structure; 4) the detection of false data injected (FDI) attacks on the power systems by GSP tools; 5) Laplacian learning with applications to admittance matrix estimation. The methods developed in these works use GSP concepts, such as graph spectrum, GSP, graph filters, and sampling over graphs.
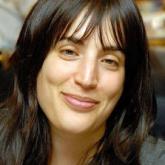
About the Speaker: Tirza Routtenberg is an Associate Professor in the School of Electrical and Computer Engineering at Ben-Gurion University of the Negev, Israel. In addition, she is a William R. Kenan, Jr., Visiting Professor for Distinguished Teaching at the Electrical and Computer Engineering Department at Princeton University for 2022-2023. She was the recipient of four Best Student Paper Awards at international conferences. She is currently an Associate Editor of IEEE Transactions on Signal and Information Processing Over Networks and of IEEE Signal Processing Letters. In addition, she is part of the SPS Technical Directions Board Representative on the Education Board. Her research interests include statistical signal processing, graph signal processing, and optimization and signal processing for smart grids.
Wireless Communication Using Reconfigurable Intelligent Surfaces: Fundamentals, Challenges and Use-Cases
Speaker: Dr. Qurrat-Ul-Ain Nadeem , University of British Columbia, Canada
Date: Fri, Apr 21
Abstract: Reconfigurable Intelligent Surfaces (RISs) assisted wireless communication has emerged as a promising paradigm for 6G networks that leverages a large number of low-cost passive reflecting elements with independently controllable reflection amplitudes and/or phases to smartly reconfigure the wireless channels. By dynamically adapting the reflection coefficients of all elements, we can realize desired communication objectives without generating additional signals and therefore without consuming any significant additional power. In this talk, we highlight the need for RISs in existing 5G communication frameworks, discuss how RISs are related to conventional relaying technologies, and present the signal model and channel estimation protocols for RIS-assisted systems by taking into account the hardware constraints of the RISs. We then illustrate the main functions and applications of RISs, providing recent insights into the best use cases of this technology. We also present our results on a recently developed RIS prototype for a wide-band communication system, before concluding with discussion on the future of this technology.
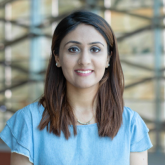
About the Speaker: Qurrat-Ul-Ain Nadeem received her M.S. and Ph.D. degrees in electrical engineering from King Abdullah University of Science and Technology (KAUST), Saudi Arabia in 2015 and 2018 respectively. She is currently a Postdoctoral Research Fellow in the Electrical Engineering Department at the University of British Columbia (UBC), Canada. Concurrently, she holds the Postdoctoral Teaching Fellow position at UBC, and teaches both undergraduate and graduate level engineering courses. Her research focus is on the modeling, design and performance analysis of next-generation wireless communication systems, and her expertise lies in the areas of communication theory, random matrix theory, optimization theory, signal processing, and electromagnetics and antenna theory. Dr. Nadeem was the recipient of the Natural Sciences and Engineering Research Council of Canada (NSERC)’s Postdoctoral Fellowship Award in 2021. She received the Paul Baron Young Scholar Award from The Marconi Society in 2018 for her work on full-dimension massive multiple-input multiple-output (MIMO) systems. Dr Nadeem is a member of IEEE since 2019, and serves as the General Chair of the workshop on Reconfigurable Intelligent and Holographic surfaces in IEEE PIMRC 2023. She was an exemplary reviewer for the IEEE Transactions on Communications and the IEEE Transactions on Wireless Communications several times, and has served as a technical program committee member of multiple conferences.
Additive Manufacturing Security: 10+ Reasons to be Concerned
Speaker: Mark Yampolskiy, Auburn University
Date: Wed, May 3
Abstract: Additive Manufacturing (AM), often referred to as 3D printing, is a rapidly growing multibillion-dollar industry. AM community was very successful in improving AM processes and developing new materials, enabling 3D-printed parts to be used in a wide range of applications, including aerospace and medical fields. However, the success comes at a price of being an increasingly attractive target for attacks.
As often the case, securing a new technology is not a simple “plug-and-play” task for already established cyber-security approaches. While cyber-security is a necessary component of AM Security, it is not even remotely sufficient to fully secure against the recognized Security Threats. This talk will provide an introduction in AM Security, a highly inter-disciplinary field of research that both poses novel challenges and provides unexpected opportunities. Focusing predominantly on attacks, this talk will outline an often-surprising cyber-physical “toolkit” that malicious actors can employ to achieve their nefarious goals. The presenter will also point out the common myths and misconceptions that – in his opinion – have been plagued this field so far. A summary of discrepancies between the current state of the field and the emerging needs will provide an invitation to the experienced and aspiring researchers in the field to address the gaps.

About the Speaker: Dr. Mark Yampolskiy is an Associate Professor at Auburn University, department of Computer Science and Software Engineering (CSSE). He is also an Affiliated Faculty with Auburn Cyber Research Center (ACRC) and National Center for Additive Manufacturing Excellence (NCAME). He was among the pioneers and is one of the leading experts in the field of Additive Manufacturing Security. His research interests include the cyber-physical means of attack and defense in AM.
In addition to his research activities, Dr. Yampolskiy is chairing Additive Manufacturing (3D Printing) Security workshop co-located with ACM Conference on Computer and Communications Security (CCS) and co-chairs Industry 4.0 symposium at International Conference on Advanced Manufacturing (ICAM). He is also leading the standardization effort on AM Security at ASTM International, under the F.42 committee.
Modern AI Series: Explainability and Regulation
Speaker: Ulrike von Luxburg, University of Tuebingen, Germany
Date: Tue, May 9
Abstract: Explainability is one of the concepts that dominate debates about the regulation of machine learning algorithms. In my presentation I will argue that in its current form, post-hoc explanation algorithms are unsuitable to achieve the law's objectives, for rather fundamental reasons. In particular, most situations where explanations are requested are adversarial, meaning that the explanation provider and receiver have opposing interests and incentives, so that the provider might manipulate the explanation for her own ends. I then discuss a theoretical analysis of Shapley Value based explanation algorithms that open the door to more formal guarantees for posthoc explanations.

About the Speaker: Ulrike von Luxburg is a full professor for the Theory of Machine Learning at the University of Tuebingen, Germany. Her research analyzes machine learning algorithms from a theoretical point of view, tries to understand their implicit mechanisms, and to give formal statistical guarantees for their performance. In this way, she reveals fundamental assumptions, biases and strenghts and weaknesses of widely used machine learning algorithms, for example in the field of explainable machine learning. Next to her own research group, she is coordinating a large research consortium on Machine Learning in Science. She is an active participant in local debates about ethics and responsibility in machine learning.
Learning for Pricing and Rate Control in Serverless Edge Computing
Speaker: György Dán, KTH Royal Institute of Technology, Stockholm, Sweden
Date: Thu, May 18
Abstract: Edge computing could enable low latency access to computing resources at the network edge. Nonetheless, in order for it to be able to cater for the needs of latency sensitive applications, there is a need for resource management algorithms that span communication, computing and storage resources, as well as pricing schemes that provide incentives for operators and users. Focusing on a serverless edge with a set of wireless devices that aim at offloading computational tasks, in this talk we consider two aspects of resource management and pricing. We first consider the problem of distributed rate adaptation of wireless devices as they interact with a serverless edge that performs load balancing. We provide a generalized Nash equilibrium formulation of the problem and use variational inequality theory to prove that the game admits an equilibrium. For the case of imperfect information we propose an online learning algorithm for the devices to maximize their utility through rate adaptation and resource reservation. We show that the proposed algorithm can converge to equilibria and achieves zero regret asymptotically, outperforming the state of the art in online convex optimization. We then consider the problem of optimal pricing for joint resource management and caching under incomplete information, and provide a single-leader multiple-follower Stackelberg game formulation. Based on results obtained for the complete information case, we propose a Bayesian Gaussian process bandit algorithm for joint price and cache optimization and we provide a bound on its asymptotic regret. Our results show that the proposed algorithm outperforms state of the art algorithms by up to 50% at little computational overhead.
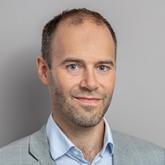
About the Speaker: György Dán is professor of teletraffic systems at KTH Royal Institute of Technology, Stockholm, Sweden. He received the M.Sc. in computer engineering from the Budapest University of Technology and Economics, Hungary in 1999, the M.Sc. in business administration from the Corvinus University of Budapest, Hungary in 2003, and the Ph.D. in Telecommunications from KTH in 2006. He worked as a consultant in the field of access networks, streaming media and videoconferencing 1999-2001. He was a visiting researcher at the Swedish Institute of Computer Science in 2008, a Fulbright research scholar at University of Illinois at Urbana-Champaign in 2012-2013, and an invited professor at EPFL in 2014-2015. He served as area editor of Computer Communications 2014-2021, as editor of IEEE Transactions on Mobile Computing 2019-2023, and serves as TPC member of conferences like IEEE Infocom and ACM e-Energy. He has received several best paper awards from IFIP and IEEE in recent years. His research interests include the design and analysis of content management and computing systems, game theoretical models of networked systems, and cyber-physical system security and resilience.
Taking Power Transformer FRA Interpretation to the Next Level
Speaker: L. Satish, Indian Institute of Science, Bangalore, INDIA
Date: Thu, May 25
Abstract: Irrespective of the type of winding damage FRA shows a left or right shift of natural frequencies. Interpretation of FRA has so far been confined to capturing these frequency shifts by statistical indices, followed by establishing a mapping between fault-type and a range for each index. Establishing this forward mapping seems to work on lab-scale experimental setups but is hard to generalize in practice. This is because it is a many-to-one mapping, and so a unique diagnosis becomes difficult. Thus, FRA remains a monitoring tool. Literature analysis reveals that this predicament of FRA is perhaps due to the lack of a suitable mathematical basis. Taking this cue, author’s research group developed a unified mathematical basis – which relates the harmonic sum of squares of winding natural frequencies to winding’s inductances and capacitances. These formulae can be manipulated to indirectly measure high-frequency inductance (L eff ) of an iron-core winding, and thereafter, the same can be reworked in conjunction with measured total shunt capacitance (C G ) to identify which winding has suffered an axial displacement (AD) or radial displacement (RD).
In this talk, the author will discuss much more challenging fault scenarios – viz., where more than one winding (in Y or Δ configuration) suffers more than one AD or RD or both, each of them occurring at different positions and in different windings. The goal is to find faulted windings and identify if it has suffered an AD or RD or both. Only quantities measurable at winding terminals are used. Experiments conducted on a hand-assembled 33kV, 3.5MVA, HV winding (Y or Δ) containing 22 double-disks/phase are presented.

About the Speaker: L. Satish (1964) received his B.E. in Electrical Engineering in 1987 from U.V.C.E, Bangalore University. Thereafter, he completed M.E (1989) and Ph.D. (1993) from Department of High Voltage Engineering, Indian Institute of Science (IISc),
Bangalore. He was in Swiss Federal Institute of Technology (ETH), Zurich, Switzerland, from 93-95 pursuing post-doctoral research. He joined Dept. of High Voltage Engineering, IISc in May 1995 as an Assistant Professor, and became a Professor in May 2007, in the Dept of Electrical Engineering. During summer of 1998 he was a visiting professor for four months at HV Institute, Helsinki University of Technology, Finland. His current research interests are – studies on transformer windings, condition monitoring and diagnostics,FRA, testing of high-speed high-resolution ADCs, and PD measurements. He was conferred “Young Engineer Award 1999,” by Indian National Academy of Engineering. He is an Associate Editor of IEEE Trans on Power Delivery since Feb. 2020 and served as Associate Editor of IET High Voltage from 2017-2023.
Machine Learning as a Service: From Trust to Efficiency
Speaker: Souvik Kundu, Intel Labs, USA
Date: Thu, Aug 10
Abstract: With the proliferation of various AI driven applications the use of machine learning as a service (MLaaS) is on the rise. Simultaneously, trustworthy MLaaS has become essential for various safety-critical and user personal applications, requiring to address both privacy and robustness concerns of the deep learning models. Towards that goal, this talk will first discuss on the requirement of privacy preserving inference (PI) service along with their limitations in terms of prohibitive interference latency and cost. We will then discuss our recent research on improving PI efficiency via novel algorithmic and model architecture optimization for both convolution and attention layer driven neural networks. We will then discuss on the robustness concerns associated with the efficient deep neural networks (DNNs). This talk will finally end with the discussion on the future research scope for trustworthy MLaaS in the era of foundation AI models. The talk has been outlined based on the following papers of the speaker: S. Kundu et al., Analyzing the confidentiality of Undistillable Teacher in Knowledge Distillation, NeurIPS 2021 S. Kundu et al., Learning to Linearize Deep Neural Networks for Secure and Efficient Private Inference, ICLR 2023 S. Kundu et al., FLOAT: Fast Learnable Once for All Adversarial Training for Tunable Trade-off between Accuracy and Robustness, WACV 2023 S. Kundu et al., Making Models Shallow Again: Jointly Learning to Reduce Non-Linearity and Depth for Latency-Efficient Private Inference, CVPRW 2023 (ORAL presentation) S. Kundu et al., SAL-ViT: Towards Latency Efficient Private Inference on ViT using Selective Attention Search with a Learnable Softmax Approximation, ICCV 2023.

About the Speaker: Souvik Kundu (Member, IEEE and ACM) received his Ph.D. degree in 2022 from the department of Electrical and Computer engineering at the University of Southern California, Los Angeles, California. He is currently a Research Scientist at Intel Labs, USA. His research interest includes scalable and efficient AI algorithm-architecture co-design, optimization, for secure and robust machine learning. Souvik has co-authored more than forty-five peer-reviewed publications and patents. He is one of the youngest recipient of the prestigious SRC Outstanding Liaison award 2023 for his contribution towards driving fruitful industrial and academic joint research. He serves as the founding area chair of the Conference on Parsimony and Learning (CPAL) 2024. Souvik served as TPC member at DATE’23 and received outstanding reviewer recognition at NeurIPS’22 and EMNLP’20.