Spring 2022 Seminars
Implicit and Recurrent Neural Networks via Contraction Theory
Speaker: Francesco Bullo, University of California, Santa Barbara
Date: Feb 10
Abstract: Basic questions in dynamical neuroscience and machine learning motivate the study of the stability, robustness, entrainment, and computational efficiency properties of neural network models. I will present some elements of a comprehensive contraction theory for neural networks. Using non-Euclidean norms I will review recent advances in analyzing and training a class of recurrent/implicit models.
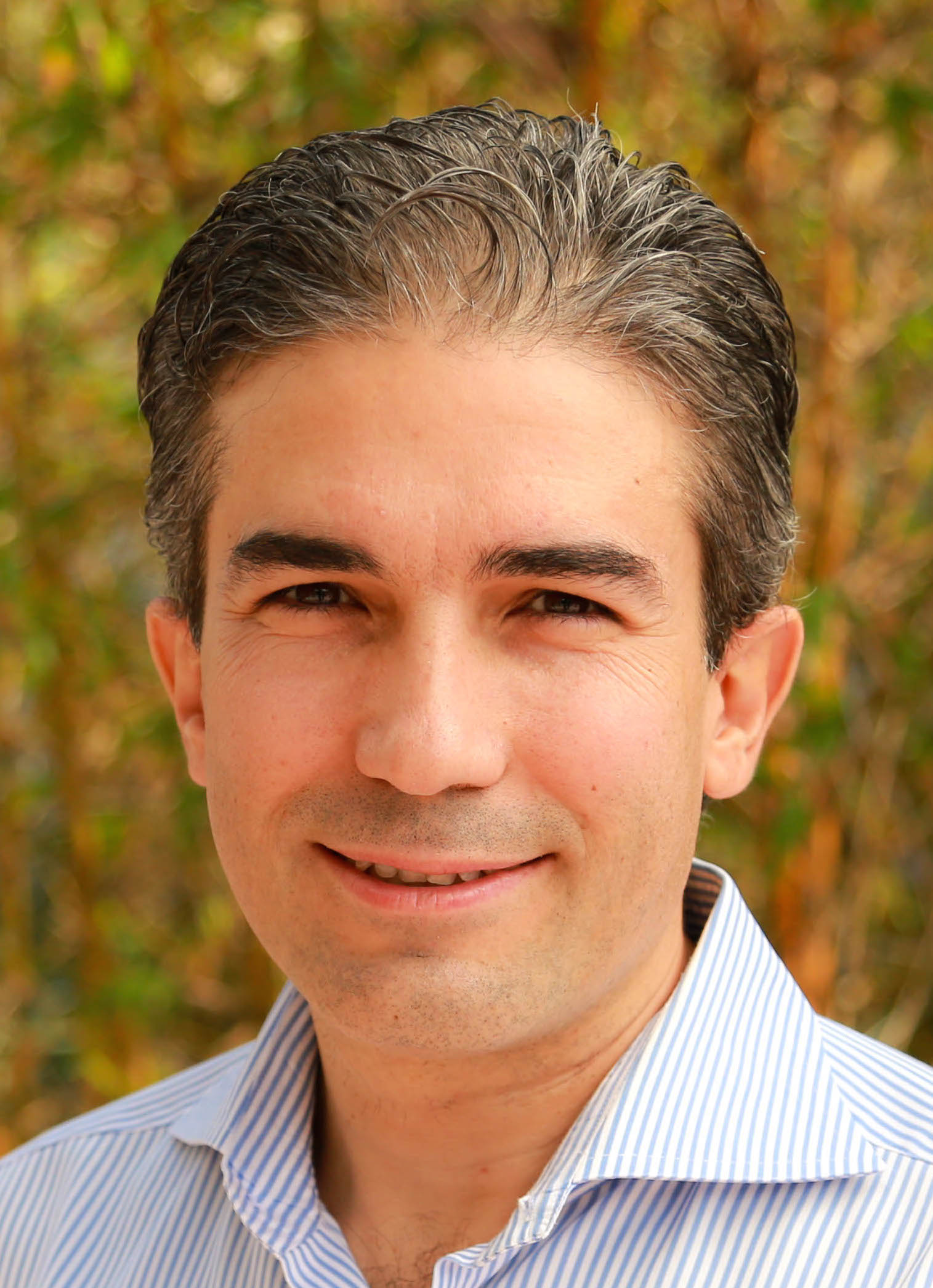
About the Speaker: Francesco Bullo is a Professor with the Mechanical Engineering Department and the Center for Control, Dynamical Systems and Computation at the University of California, Santa Barbara. He was previously associated with the University of Padova (Laurea degree in Electrical Engineering, 1994), the California Institute of Technology (Ph.D. degree in Control and Dynamical Systems, 1999), and the University of Illinois. He served on the editorial boards of IEEE, SIAM, and ESAIM journals and as IEEE CSS President and SIAM SIAG CST Chair. His research interests focus on network systems and distributed control with application to robotic coordination, power grids and social networks. He is the coauthor of “Geometric Control of Mechanical Systems” (Springer, 2004), “Distributed Control of Robotic Networks” (Princeton, 2009), and "Lectures on Network Systems" (Kindle Direct Publishing, 2021, v1.5). He received best paper awards for his work in IEEE Control Systems, Automatica, SIAM Journal on Control and Optimization, IEEE Transactions on Circuits and Systems, and IEEE Transactions on Control of Network Systems. He is a Fellow of IEEE, IFAC, and SIAM.
Addressing regulatory challenges for AI in healthcare: Building a safe and effective machine learning life cycle
Speaker: Adarsh Subbaswamy, Johns Hopkins University
Date: Feb 28
Abstract: As machine learning (ML) is beginning to power technologies in high impact domains such as healthcare, the need for safe and reliable machine learning has been recognized at a national level. For example, the U.S. Food and Drug Administration (FDA) has recently had to rethink its regulatory framework for the ever growing number of machine learning-powered medical devices. The core challenge for these agencies is to determine whether machine learning models will be safe and effective for their intended use. In my research, I seek to develop safe and effective machine learning that can meet the needs of various stakeholders—including users, model developers, and regulators. Accomplishing this requires addressing technical challenges at all stages of a machine learning system’s life cycle, from new learning algorithms that allow users to specify desirable behavior, to stress-tests and verification of safety properties, to model monitoring and maintenance strategies. In this talk, I will overview my work addressing various parts of the machine learning life cycle with respect to the problem of dataset shift—differences between the model's training and deployment environments that can lead to failure to generalize. First, I will describe causally-inspired learning algorithms which allow model developers to specify potentially problematic dataset shifts ahead of time and then learn models which are guaranteed to be stable to these shifts. Then I will describe a new evaluation method for stress-testing a model's stability to dataset shift. This is generally a difficult task because it requires evaluating the model on a large number of independent datasets. Since the cost of collecting such datasets is often prohibitive, I will describe a distributionally robust framework for evaluating model robustness to user-specified shifts using only the available evaluation data.
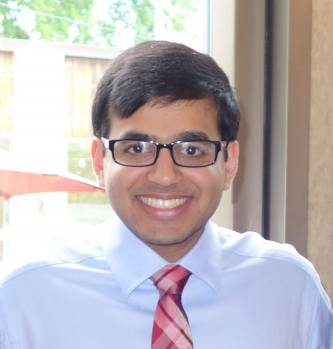
About the Speaker: Adarsh Subbaswamy is a PhD candidate in computer science at Johns Hopkins University advised by Suchi Saria, and a CERSI scholar affiliated with the Johns Hopkins Center of Excellence in Regulatory Science and Innovation. His research seeks to address challenges in developing safe and effective machine learning for safety-critical domains such as healthcare using techniques from machine learning, causal inference, and robust optimization. His work has appeared in machine learning conferences (e.g., AISTATS and UAI) as well as medical journals (Biostatistics and the New England Journal of Medicine). Prior to his PhD, Adarsh received his BS from Vanderbilt University.
Three challenges in Responsible ML and How to Overcome Them, Provably
Speaker: Yu-Xiang Wang, UCSB
Date: Mar 10
Abstract: The rise of machine learning (ML) and deep learning has revolutionized almost every aspect of our daily life. Learning-based methods are now widely used in financial, medical, and legal applications for tasks involving not only predictions, but also decision making, often in adversarial, non-stationary, and strategic environments, and sometimes relying on sensitive data. Classical statistical learning theory does not cover these new settings, which motivates us to develop new theories and algorithms for applying ML responsibly in these emerging applications.
In this talk, I will cover recent advances from that address these challenges with strong theoretical guarantees. Topics include new technical results in offline reinforcement learning, adaptive online learning and differential privacy as well as their promise in real-life applications.
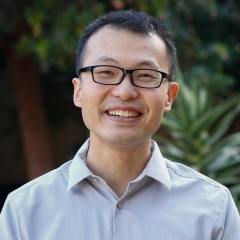
About the Speaker: Yu-Xiang Wang is the Eugene Aas Assistant Professor of Computer Science at UCSB. He runs the Statistical Machine Learning lab and co-founded the UCSB Center for Responsible Machine Learning. Prior to joining UCSB, he was a scientist with Amazon Web Services’s AI research lab in Palo Alto, CA. Yu-Xiang received his PhD in Statistics and Machine Learning in 2017 from Carnegie Mellon University (CMU). Yu-Xiang’s research interests include statistical theory and methodology, differential privacy, reinforcement learning, online learning and deep learning. His work had been supported by an NSF CAREER Award, Amazon ML Research Award, Google Research Scholar Award, Adobe Data Science Research Award and had received paper awards from KDD'15, WSDM'16, AISTATS'19 and COLT'21.
HOW TO HANDLE BIASED DATA AND MULTIPLE AGENTS IN MACHINE LEARNING?
Speaker: Manolis Zampetakis, University of California, Berkeley
Date: Mar 11
Abstract: Modern machine learning (ML) methods commonly postulate strong assumptions such as: (1) access to data that adequately captures the application environment, (2) the goal is to optimize the objective function of a single agent, assuming that the application environment is isolated and is not affected by the outcome chosen by the ML system. In this talk I will present methods with theoretical guarantees that are applicable in the absence of (1) and (2) as well as corresponding fundamental lower bounds. In the context of (1) I will focus on how to deal with truncation and self-selection bias and in the context of (2) I will present a foundational comparison between two-objective and single objective optimization.
About the Speaker: Manolis Zampetakis is currently a post-doctoral researcher at the EECS Department of UC Berkeley working with Michael Jordan. He received his PhD from the EECS Department at MIT where he was advised by Constantinos Daskalakis. He has been awarded the Google PhD Fellowship and the ACM SIGEcom Doctoral Dissertation Award. He works on the foundations of machine learning (ML), statistics, and data science, with focus on statistical analysis from systematically biased data, optimization methods for multi-agent environments, and convergence properties of popular heuristic methods.
Millimeter-Wave Massive MIMO for Communication and Sensing: Developing a Wireless Backbone for Smart Cities
Speaker: Maryam Eslami Rasekh, UCSB
Date: Mar 21
Abstract: The rise of millimeter wave frequencies – sparked by unlicensing of large swaths of spectrum two decades ago – is posed to significantly reshape the wireless landscape. For mobile networks, a throughput boost of >1000x is promised through “picocellular” architectures and vigorous spatial reuse, while physically small yet electronically large antenna arrays raise the bar for what is possible in terms of sensing and environmental awareness. Incorporating these capabilities in the next-generation of urban wireless infrastructure entails large scale deployment of massive MIMO frontends for backhaul relaying, multi-user multiplexing, localization, and sensing. It is therefore imperative to develop highly scalable, low-power, and cost-efficient frontend designs tailored for each application, as well as scalable signal processing solutions that can handle the high dimensionality with low overhead and delay.
In the first half of my talk, I discuss how compressive signal processing techniques may be used to exploit the inherent sparsity of MIMO channels for scalable channel tracking and radar sensing, and how these techniques can be adapted to simplified frontends and limited synchronization. In the second half, I will cover our collaborative efforts toward developing scalable MIMO frontends. I will discuss how adopting a “modular” architecture can simplify hardware scaling for all-digital frontends and ease requirements such as oscillator phase noise. In RF-beamformed phased arrays, we show that per-channel power consumption can be slashed by an order of magnitude by adopting a highly simplified on-off architecture, and quantify the inherent power-utilization tradeoffs that arise in this and other low-resolution beamforming architectures.
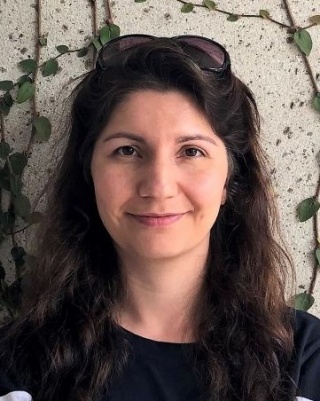
About the Speaker: Maryam received her BS and MS from Isfahan University of Technology and Sharif University of Technology, Iran. She completed her PhD at University of California Santa Barbara in 2020 and is currently a postdoctoral researcher in the Wireless Communications and Sensornets Laboratory at UCSB, working with Prof. Upamanyu Madhow. Her research is mainly focused on the development of scalable massive MIMO frontends and signal processing techniques, as well as networking and cross-layer design tools for the next generation of wireless communication and sensing applications, primarily at millimeter-wave and THz frequencies.
Enabling Intelligent Services at the Network Edge: A Cross-Layer Approach
Speaker: Konstantinos Poularakis, Yale University
Date: Mar 23
Abstract: The proliferation of novel mobile applications and the associated machine learning services in the 5G/6G era necessitates a fresh view on the architecture and algorithms at the network edge in order to meet stringent performance requirements. I will present a cross-layer approach to address these challenges. Starting with intricate service placement, chaining, and computation offloading problems at the application layer, I will gradually move to lower layers of the protocol stack and propose methods that are cognizant of the operations at these layers. Algorithms with provably near optimal performance will be designed leveraging techniques from discrete and stochastic optimization. Evaluations will demonstrate significant benefits in terms of latency, energy and resource consumption for a range of services with diverse requirements.
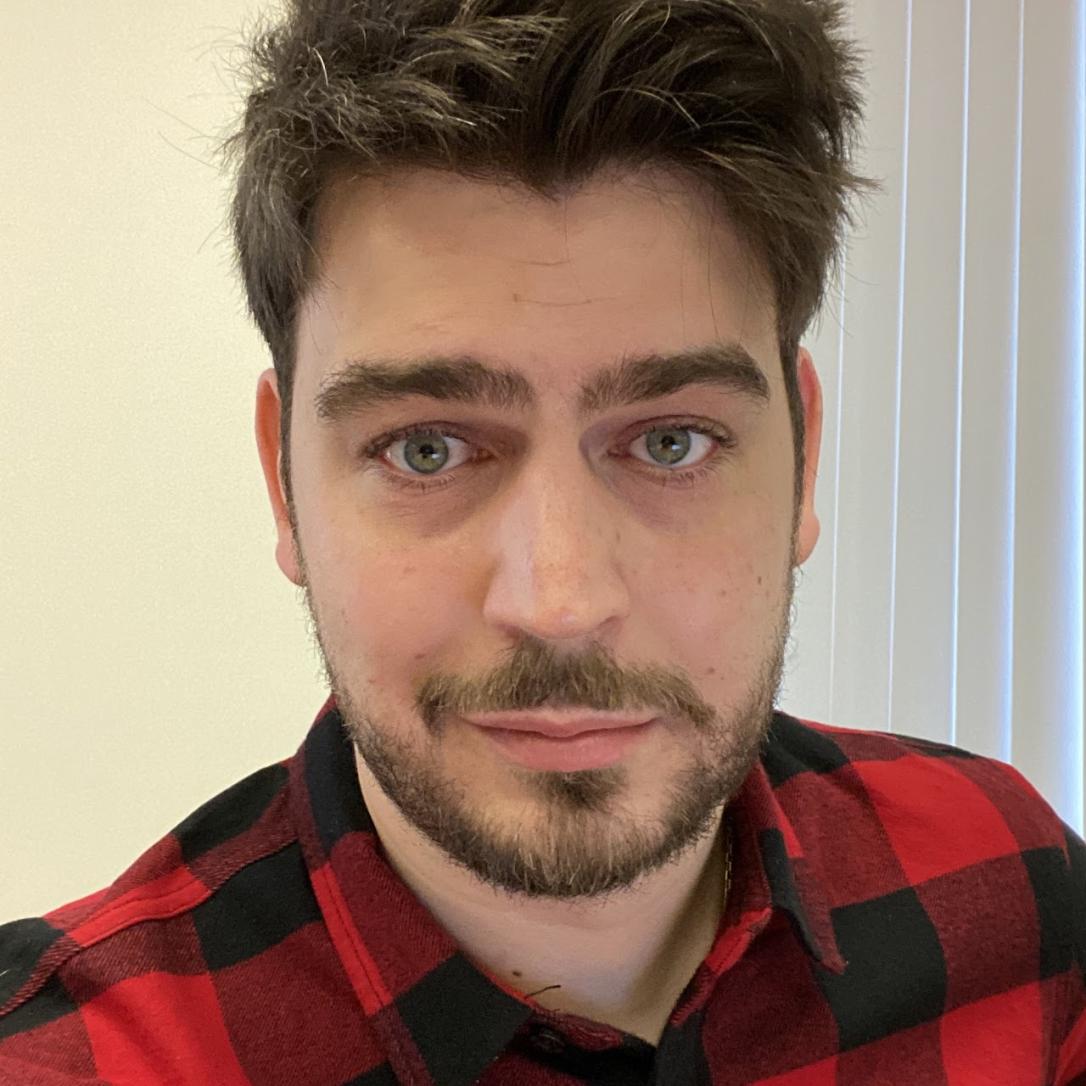
About the Speaker: Konstantinos Poularakis is an Associate Research Scientist at the Electrical Engineering Department of Yale University. His research interests lie on the nexus of network optimization and machine learning with emphasis on emerging network architectures such as mobile edge computing, software-defined and wireless caching networks. Konstantinos received his Ph.D. from University of Thessaly in Greece where he was advised by Professor Leandros Tassiulas. His work has been supported by fellowships from the Alexander S. Onassis and Bodossaki foundations, as well as by several research grants including a recently-awarded NSF grant on learning architectures applied to 5G/6G networks for which he serves as a co-PI. Konstantinos also received the best paper awards at the IEEE Infocom 2017 and IEEE ICC 2019 conferences.
Strengthening and Enriching Machine Learning for Cybersecurity
Speaker: Wenbo Guo, Penn State
Date: Apr 4
Abstract: Nowadays, security researchers are increasingly using AI to automate and facilitate security analysis. Although making some meaningful progress, AI has not maximized its capability in security yet, mainly due to two challenges. First, existing ML techniques have not reached security professionals' requirements in critical properties, such as interpretability and adversary-resistancy. Second, Security data imposes many new technical challenges, and these challenges break the assumptions of existing ML models and thus jeopardize their efficacy.
In this talk, I will describe my research efforts to address the above challenges, with a primary focus on strengthening the interpretability of ML-based security systems and enriching ML to handle low-quality labels in security data. I will describe our technique to robustify existing explanation methods against attacks and a novel explanation method for deep learning-based security systems. I will also demonstrate how security analysts could benefit from explanations to discover new knowledge and patch ML model vulnerabilities. Then, I will introduce a novel ML system to enable high accurate categorizations of low-quality attack data and demonstrate its utility in a real-world industrial-level application. Finally, I will conclude by highlighting my plan towards maximizing the capability of advanced ML in cybersecurity.

About the Speaker: Wenbo Guo is a Ph.D. Candidate at Penn State and a visiting student at Northwestern. His research interests are machine learning and cybersecurity. His work includes strengthening the fundamental properties of machine learning models and designing customized machine learning models to handle security-unique challenges. He is a recipient of the IBM Ph.D. Fellowship (2020-2022), Facebook/Baidu Ph.D. Fellowship Finalist (2020), and ACM CCS Outstanding Paper Award (2018). His research has been featured by multiple mainstream media and has appeared in a diverse set of top-tier venues in security and machine learning. Going beyond academic research, he also actively participates in many world-class cybersecurity competitions and has won the 2018 DEFCON/GeekPwn AI challenge finalist award..
Integrated Circuit and System for Next Generation Communications, Sensing and Imaging
Speaker: Aoyang Zhang, Harvard University
Date: Mar 28
Abstract: Today’s CMOS technology scaling allows circuits to operate above 100 GHz, opening revolutionary applications in communications, imaging, and sensing. Future communication and sensing systems will open up more and more applications that can vary from 100 Kbps to 100 Gbps. These heterogeneous networks enable many distinct applications. The vast number of wireless communications and sensing nodes are largely convenient for our lives. However, we are seeing the total energy consumption increase dramatically with conventional technology. To reduce the carbon emissions and finally achieve carbon neutrality, we should not only come up with new hardware architectures for power efficient wireless communications and sensing systems, but also leverage the emerging devices to further reduce computation complexity and power consumption. On the other hand, the conventional sensing technologies, including magnetic resonance imaging (MRI) with limited sensing resolution are bulky, heavy, costly, and thus available only at dedicated facilities. Therefore, architecture innovations with communication efficiency enhancement are critical for enabling future IoT, 5G, and beyond 5G communications and sensing systems.
In this talk, I will first discuss a new “transmitter family”, the subharmonic switching (SHS) digital transmitter architecture that largely enhances PA/transmitter efficiency from RF to mm-wave frequencies, enabling high efficiency wireless communications. Based on the proposed architecture, I will walk the audience through a few benchmarking designs with silicon prototypes and measurement results. Secondly, I will introduce the new 5G receiver architecture and high-speed low-power ADCs by leveraging digital signal processing and mixed-signal techniques. Then I will discuss the next-generation of on-chip sensing systems, which involve both MRI sensing and quantum sensing and imaging systems. The proposed sensing and imaging systems can largely minimize the MRI size and beef up the sensing resolution capable of spectroscopy and imaging at the single-cell level. Finally, I will conclude this talk with future research directions for developing next-generation wireless communication systems, new circuit opportunities with two-dimensional devices and chip-scale sensing systems for quantum sensing, biotechnology, and subsurface imaging.

About the Speaker: Aoyang Zhang is currently a postdoctoral fellow at Harvard University. He received his B.S. degree from Zhejiang University, Hangzhou, China, in 2014, and the Ph.D. degree from University of Southern California, Los Angeles, in 2020, all in electrical engineering.
His current research interests include threefold. First, Analog/Mixed-Signal/RF/Mm-wave integrated circuits (IC) design including highly efficient RF/millimeter wave power amplifiers and transceiver architectures for 5G/beyond 5G/IoT/WiFi-7 wireless communications. Second, scalable nuclear magnetic resonance (NMR) and electron spin resonance (ESR) based silicon and GaN quantum sensing and wireless sensing integrated systems for biological sensing, chemical sensing, and subsurface imaging. Third, new circuit architecture with two-dimensional (2D) memristive devices to overcome technological limitations in computational complexity and power consumption.
Dr. Zhang was the recipient of the 2021 USC Best Dissertation Award in Electrical Engineering, 2020-2021 IEEE Solid-State Circuits Society (SSCS) Predoctoral achievement award, Ming Hsieh Institute Scholar in 2020, IEEE SSCS Student Travel Grant Award (STGA) in 2018, Best Bachelor Thesis Award in 2014, and the first prize of Chinese National Mathematical Competition in 2010. From 2015 to present, he has served as a reviewer for IEEE Transactions on Circuits and Systems I/II (TCAS), IEEE Solid-State Circuits Letters (SSC-L), IEEE Transactions on Microwave Theory and Techniques (TMTT), and IEEE Journal of Solid-State Circuits (JSSC).
Learning 3D representations with minimal supervision
Speaker: Yue Wang, MIT
Date: Mar 29
Abstract: Deep learning has demonstrated considerable success embedding images and more general 2D representations into compact feature spaces for downstream tasks like recognition, registration, and generation. Learning from 3D data, however, is the missing piece needed for embodied agents to perceive their surrounding environments. To bridge the gap between 3D perception and robotic intelligence, my present efforts focus on learning 3D representations with minimal supervision from a geometry perspective.
In this talk, I will discuss two key aspects to reduce the amount of human supervision in current 3D deep learning algorithms. First, I will talk about how to leverage geometry of point clouds and incorporate such inductive bias into point cloud learning pipelines. These learning models can be used to tackle object recognition problems and point cloud registration problems. Second, I will present our works on leveraging natural supervision in point clouds to perform self-supervised learning. In addition, I will discuss how these 3D learning algorithms enable human-level perception for robotic applications such as self-driving cars. Finally, the talk will conclude with a discussion about future inquiries to design complete and active 3D learning systems.

About the Speaker: Yue Wang is a final year PhD student with Prof. Justin Solomon at MIT. His research interests lie in the intersection of computer vision, computer graphics, and machine learning. His major field is learning from point clouds. His paper "Dynamic Graph CNN" has been widely adopted in 3D visual computing and other fields. He is a recipient of the Nvidia Fellowship and is named the first place recipient of the William A. Martin Master’s Thesis Award for 2021. Yue received his BEng from Zhejiang University and MS from University of California, San Diego. He has spent time at Nvidia Research, Google Research and Salesforce Research.
Towards Scalable Representation Learning for Visual Recognition
Speaker: Saining Xie, Facebook AI Research
Date: Mar 29
Abstract: A powerful biological and cognitive representation is essential for humans' remarkable visual recognition abilities. Deep learning has achieved unprecedented success in a variety of domains over the last decade. One major driving force is representation learning, which is concerned with learning efficient, accurate, and robust representations from raw data that are useful for a downstream classifier or predictor.
A modern deep learning system is composed of two core and often intertwined components: 1) neural network architectures and 2) representation learning algorithms. In this talk, we will present several studies in both directions. On the neural network modeling side, we will examine modern network design principles and how they affect the scaling behavior of ConvNets and recent Vision Transformers. Additionally, we will demonstrate how we can acquire a better understanding of neural network connectivity patterns through the lens of random graphs. In terms of representation learning algorithms, we will discuss our recent efforts to move beyond the traditional supervised learning paradigm and demonstrate how self-supervised visual representation learning, which does not require human annotated labels, can outperform its supervised learning counterpart across a variety of visual recognition tasks. The talk will encompass a variety of vision application domains and modalities (e.g. 2D images, 3D scenes and languages). The goal is to show existing connections between the techniques specialized for different input modalities and provide some insights about diverse challenges that each modality presents. Finally, we will discuss several pressing challenges and opportunities that the ``big model era’’ raises for computer vision research.
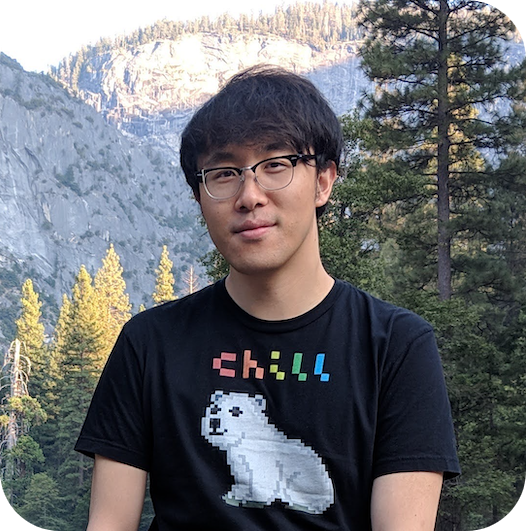
About the Speaker: Saining Xie is a research scientist at Facebook AI Research (FAIR). He received his Ph.D. and M.S. degrees in computer science from the University of California San Diego, advised by Zhuowen Tu. Prior to that, he received his Bachelor's degree from Shanghai Jiao Tong University. He has broad research interests in deep learning and computer vision, with a focus on developing deep representation learning techniques to push the boundaries of core visual recognition. His research has been extensively cited (more than 16,000 times) by other researchers and adopted in several industrial-scale applications. He is also a recipient of the Marr Prize Honorable Mention at ICCV 2015.
Perceiving the World in 2D and 3D
Speaker: Georgia Gkioxari, Meta AI
Date: Mar 30
Abstract: Images are powerful storytellers as they capture events, memorable or mundane, from our everyday lives. Humans have the ability to perceive images effortlessly but for machines to do the same, they need to build an understanding of the world, a world composed of complex objects, humans and their rich interactions. In this talk, I will present my work towards enabling machines to recognize and localize objects and their interactions from images, work that is powering products in industry used by millions of people, such as Portal. The advances in 2D visual understanding are unprecedented but the world is 3D and objects have 3D properties which modern recognition models ignore. Toward 3D perception, I will present my work on inferring 3D object shapes from real-world images and understanding 3D scenes via multi-view 2D supervision. To this end, I will present PyTorch3D, our efficient and modular 3D deep learning library which efficiently fuses advances in deep learning with geometry and is widely adopted within the academic and industry research community.
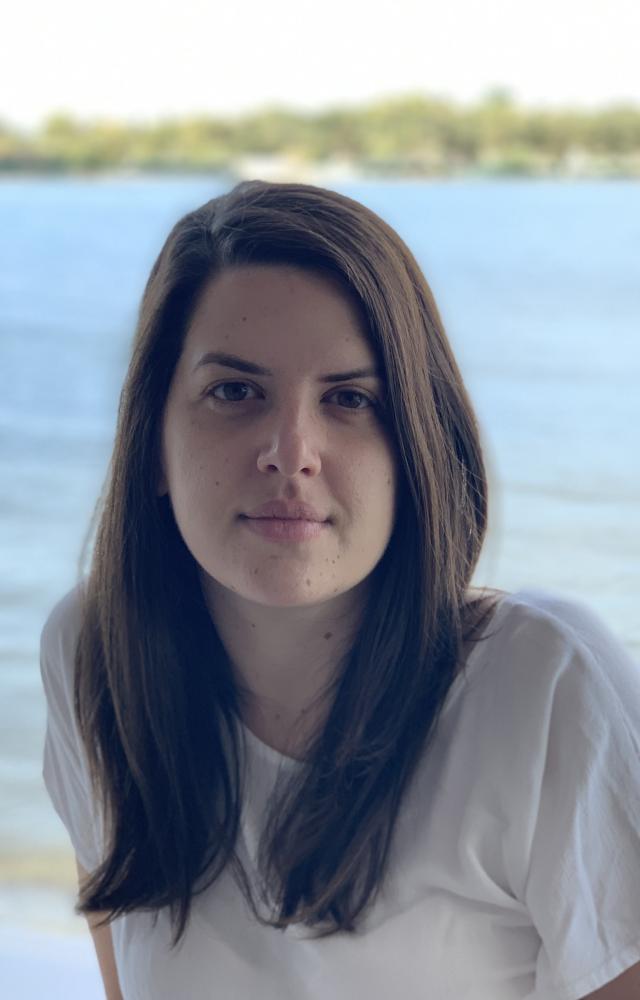
About the Speaker: Georgia Gkioxari is a research scientist at Meta AI. She received her PhD in computer science and electrical engineering from the University of California at Berkeley under the supervision of Jitendra Malik in 2016. Her research interests lie in computer vision, with a focus on object recognition from images and videos. In 2017, Georgia received the Marr Prize at ICCV for "Mask R-CNN". In 2019, she was named one of the 30 Influential Women Advancing AI by ReWork and was nominated for the Women in AI awards by VentureBeat. In 2021, Georgia received the PAMI Young Researcher Award and the Mark Everingham prize for Detectron.
Wireless Hardware Design in the mmWave and THz Spectrum: From Circuit Innovation to Holistic Integration
Speaker: Sensen Li, Samsung Research America
Date: Mar 31
Abstract: There has been an exponential growth of data-rate demand for modern wireless communications over the past decades, driven by the rapid growth of mobile devices. This trend continues with the beyond-5G and 6G wireless systems that will leverage mmWave and sub-THz spectrum with large available bandwidth and the resulting proportionate increase in channel capacity. These new spectra will serve as the backbone for next-generation of wireless networks allowing high- speed and low-latency connectivity to enable emerging wireless applications such as immersive extended reality (XR). To support the ever-evolving wireless services and applications, stringent system requirements are therefore imposed on the hardware design. Silicon-based integrated electronics are one of the most powerful, reliable, and cost-effective platforms for building complex systems. However, operating at high mmWave/sub-THz has been pushing the silicon-based device to its limits, which necessitates technological innovations in devices, circuits, antennas, packaging, and many other relevant areas.
In this talk, I will present my exploration to address the fundamental challenges faced by silicon circuits at mmWave/THz frequencies, and more importantly, to pioneer an interdisciplinary design methodology that breaks boundaries between circuits and other disciplines, opening up new design spaces in wireless hardware. First, I will delve into the design of mmWave power amplifiers and other building blocks in transmitter systems that govern both system energy efficiency and spectral efficiency. I will present two design examples on how to overcome silicon device limitations by circuit architecture/topology innovations. Next, I will present several mmWave circuits and systems by fusing complex electronics with antennas and signal processing. In particular, novel multi-feed antennas and their co-integration with electronics can actively synthesize desired radiation characteristics with unprecedented on-antenna functionalities and reconfigurability far beyond electronics-only designs, including high- efficiency power combining, impedance scaling, active load modulation, noise cancellation, and gain boosting. In the end, I will conclude the talk by looking into future challenges and opportunities enabled by these co-design innovations in circuits, electromagnetics, and packaging.

About the Speaker: Sensen Li is a research engineer at Samsung Research America, leading the RFIC R&D effort for the development of next-generation wireless communication systems. He received his B.Eng. with highest honors and B.A. from Zhejiang University, Zhejiang, China, in 2013, and the Ph.D. degree from the Georgia Institute of Technology, Atlanta, GA, USA, in 2020. His research interests include RF, mmWave, and THz integrated antenna, circuit, and system designs for wireless communication and sensing applications.
Dr. Li was a recipient of the IEEE Microwave Theory and Techniques Society (MTT-S) Graduate Fellowship in 2019, the Best Student Paper Award at the 2018 IEEE Radio Frequency Integrated Circuits Symposium (RFIC), the IEEE Antennas and Propagation Society (AP-S) Doctoral Research Grant in 2018, and the Analog Devices, Inc. Outstanding Student Designer Award in 2018. He was also a co-recipient of multiple best paper awards, including the IEEE Radio Frequency Integrated Circuits Symposium (RFIC) Best Student Paper Award in 2021, the IEEE International Microwave Symposium (IMS) Best Student Paper Award in 2021, and the IEEE Custom Integrated Circuits Conference (CICC) Best Paper Award in 2019.
A roadmap for incorporating physical layer security in 6G: why it is needed and how we will do it
Speaker: Arsenia (Ersi) Chorti, ENSEA, France
Date: Apr 6
Abstract: Quality of security (QoSec) is envisioned as a flexible framework for future networks with highly diverse non-functional requirements (delays, energy conusmption, massive connectivity / scalability, computational power, etc.). In parallel, the integration of communications and sensing along with embedded artificial intelligence can provide the foundations for building autonomous and adaptive security protocols. In this talk, we will discuss how physical layer security (PLS), being naturally adaptive, fits in the QoSec framework; we will further propose a comprehensive roadmap for its incorporation in 6G, by leveraging adaptation of the transmission parameters to underlying security assumptions. In this framework, we will present smart pre-processing schemes for RF fingerprinting and secret key generation (SKG), focusing on disentangling deterministic from random components in both synthetic and real channel state information (CSI) vectors. Finally, we will present a novel approach for distributed anomaly detection in large scale Internet of Things (IoT) systems, leveraging self-monitoring of a device’s hardware (e.g., memory usage, time to Tx-Rx). Through experiments in software defined wireless sensor networks, we will discuss the potential advantages of such distributed, PHY based, anomaly detection solutions.

About the Speaker: Arsenia (Ersi) Chorti is a Professor at the École Nationale Supérieure de l'Électronique et de ses Applications (ENSEA), Joint Head of the Information, Communications and Imaging (ICI) Group of the ETIS Lab UMR 8051 and a Visiting Scholar at Princeton and Essex Universities. Her research spans the areas of wireless communications and wireless systems security for 5G and 6G, with a particular focus on physical layer security. Current research topics include : context aware security, multi-factor authentication protocols, 5G / 6G and IoT, anomaly detection, machine learning for communications, new multiple access techniques and scheduling. She is a Senior IEEE Member, member of the IEEE INGR on Security and of the IEEE P1951.1 standardization workgroup (Smart Cities), member of the Competitive Pole Systematic and of the PhD Thesis GdR ISIS Award Committee in France. Since October 2021 she is chairing the IEEE Focus Group on Physical Layer Security.
Converse and Achievability Bounds for Finite Length Quantum Codes in Quantum Erasure Channel
Speaker: Alexei Ashikhmin, Nokia Bell Labs
Date: Apr 19
Abstract: In recent years quantum computers moved much closer to reality. There are a number of companies that are making rapid progress toward building a large-scale quantum computer. In particular, IBM T. J. Watson Research Center in Yorktown, NY, made quantum computing available via the cloud to anyone interested in access to an IBM 20-qubit quantum processor. Thus, anyone can run quantum algorithms and experiments that involve up to 20 physically instantiated qubits. The quantum technology used is scalable and, therefore, it is expected that a larger quantum computer will be built within several years. It is important to remember that a quantum computer of only 50 logical qubits would be able to solve certain tasks that are not feasible for any of today’s supercomputers.
One of the main difficulties in building a real-life quantum computer is that any real physical system cannot be completely isolated from its environment. This causes unavoidable environmental and control errors in quantum circuits and quantum memory. An efficient way of fighting this problem is using methods of Quantum Information Theory for quantum error correction and quantum storage. Quantum Information Theory is significantly more diverse and complex than its classical counterpart. It is enough to say that the capacities of some basic quantum channels, like the quantum counterpart of the classical binary symmetric channel, are still not known. Even less is known about the performance of finite length quantum codes.
In this talk I will present my recent results on the converse and achievability bounds for finite length codes in the quantum erasure channel. The obtained bounds significantly improve on the previously known bounds, and in some scenarios are very tight. I will also share some counter intuitive and surprising effects of quantum mechanics, for example, Elitzur–Vaidman quantum tester and information transmission with data rates exceeding the classical channel capacity.
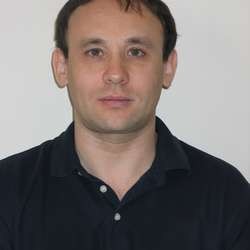
About the Speaker: Dr. Alexei Ashikhmin is Distinguished Member of Technical Staff in the Communications and Statistical Sciences Research Department of Nokia Bell Labs. He is also an adjunct professor at Columbia University, where he teaches courses on Digital Communications, Quantum Computing and Communications, and Error Correcting Codes. His research interests include communications theory, massive MIMO systems, quantum information theory and quantum error correction, theory of error correcting codes and its modern applications, such as Blockchain.
Dr. Ashikhmin received his PhD in Electrical Engineering from the Institute of Information Transmission Problems, Russian Academy of Science. Prior to joining Bell Labs, he was associated with Computer Science Department of Delft University of Technology (the Netherlands), and with Computer, Information, and Communication Group of Los Alamos National Laboratory, New Mexico.
Alexei is an IEEE Fellow and currently serves on the IEEE Information Theory Society Fellows Evaluation Committee. Previously, he served two terms as an Associate Editor of the IEEE Transactions on Information Theory. He is a recipient of multiple awards, including the 2017 SPS Donald G. Fink Overview Paper Award, the 2004 Best Paper IEEE Communications Society Stephen O. Rice Prize, the 2014 Thomas Edison Patent Award, and the 2019 Top Ten Nokia Inventors Award with the All-Time Highest Number of Granted Patents.
How To Break Step or Reducing Oscillations
Speaker: D.V. & G.V. Chudnovsky, New York University
Date: May 9
Abstract: We present analysis of transient’s minimization problems in large coupled systems. These problems occur in mechanical and electronic systems. The main emphasis here is on minimization of power transients in large computer systems near resonances. The main tool is the method of extremal functions in studies of trigonometric sums interference, related to famous Erdos-Turan discrepancy bound.

About the Speaker: The Chudnovsky brothers have held records, at different times, for computing π to the largest number of places, including two billion digits in the early 1990s on a supercomputer they built (dubbed 'm-zero') in their apartment in Manhattan. In 1987, the Chudnovsky brothers developed the algorithm (now called the Chudnovsky algorithm) that they used to break several π computation records. Today, this algorithm is used by Mathematica to calculate π, and has continued to be used by others who have achieved world records in pi calculation.
The brothers also assisted the Metropolitan Museum of Art around 2003 in the merging of a series of digital photographs taken of 'The Hunt of the Unicorn' tapestries during their cleaning. PBS aired a program on its science show NOVA, hosted by Robert Krulwich, that described the difficulties in photographing the tapestries and the math used to fix them.
The brothers are currently Distinguished Industry Professors at the New York University Tandon School of Engineering. Gregory was awarded the MacArthur Fellowship (also known as the 'Genius Grant') in 1981.
Generating HPC memory architectures with HLS: The two sides of the medal
Speaker: Christian Pilato, Politecnico di Milano, Italy
Date: May 18
Abstract: Many HPC applications are massively parallel and can benefit from the spatial parallelism offered by reconfigurable logic. While modern memory technologies can offer high bandwidth, designers must craft advanced communication and memory architectures for efficient data movement and on-chip storage. Addressing these challenges requires to combine compiler optimizations, high-level synthesis, and hardware design. In this talk, I will present challenges and trends for generating massively parallel accelerators on FPGA for high-performance computing and how the H2020 EVEREST project is addressing them.
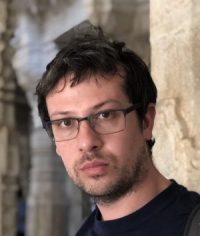
About the Speaker: Christian Pilato is a Tenure-Track Assistant Professor at Politecnico di Milano. He was a Post-doc Research Scientist at Columbia University (2013-2016) and at the ALaRI Institute of the Università della Svizzera italiana (2016-2018). He was also a Visiting Researcher at New York University, Delft University of Technology, and Chalmers University of Technology. He has a Ph.D. in Information Technology from Politecnico di Milano (2011). His research interests focus on the design, optimization, and prototyping of heterogeneous system-on-chip architectures and reconfigurable systems, with emphasis on memory and security aspects. Starting from October 2020, he is the Scientific Coordinator of the H2020 EVEREST project. He served as program chair of EUC 2014 and he will be program chair of ICCD 2022. He is currently serving in the program and organizing committees of many conferences on EDA, CAD, embedded systems, and reconfigurable architectures (DAC, ICCAD, DATE, ASP-DAC, CASES, FPL, FPT, ICCD, etc.) He is a Senior Member of IEEE and ACM, and a Member of HiPEAC.