Spring 2021 Seminars
A complete listing
Date | Time | Speaker | From | Title | Recording |
---|---|---|---|---|---|
Thur, Feb 4 | 11am - 12pm | Hai (Helen) Li | Duke University | Advancing the Design of Adversarial Machine Learning Methods | Record |
Wed, Feb 10 | 10am - 11am | Joelle Pineau | Facebook AI Research | Building Reproducible, Reusable, and Robust Deep Reinforcement Learning Systems | Record |
Thur, Feb 11 | 11am - 12pm | Michel Kinsy | Texas A&M University | Establishing the Essential Hardware Primitives for Quantum-Proof Secure Computer Systems | Record |
Thur, Feb 18 | 11am - 12pm | Yatish Turakhia | UC Santa Cruz | Accelerating Biology and Medicine with Hardware Specialization | Record |
Thur, Feb 18 | 1pm - 2pm | Dr. Mads Almassalkhi | University of Vermont | Towards scalable integration of distributed energy resources with packetized energy management | Record |
Wed, Feb 24 | 12pm - 1pm | Pranav Rajpurkar | Stanford University | Computer Science Colloquium, Advancements and Challenges for Deep Learning in Medical Imaging | Unavailable |
Mon, Mar 1 | 9am - 10am | Mengye Ren | University of Toronto | STEPS TOWARDS MAKING MACHINE LEARNING MORE NATURAL | Unavailable |
Thur, Mar 4 | 11am - 12pm | Yu Qiaoyan | University of New Hampshire | Hardware Security in Three Dimensional (3D) Integrated Circuits and Systems | Record |
Monday, Mar 8 | 11am - 12pm | Sarah Dean | University of California, Berkeley | Reliable Machine Learning in Feedback Systems | Unavailable |
Wed, Mar 10 | 11am - 12pm | Urvashi Khandelwal | Stanford University | The Generalizability and Interpretability of Neural Language Models | Unavailable |
Wed, Mar 10 | 11am - 12pm | Danielle Belgrave, Niranjani Prasad | Microsoft Research Cambridge, UK | Machine Learning for Personalised Healthcare: Opportunities, Challenges and Insights | Record |
Thur, Mar 11 | 12pm - 1pm | Kyri Baker | University of Colorado | Data-powered smart grids and communities | Record |
Thur, Mar 11 | 12:30pm - 1:30pm | Manish Raghavan | Cornell University | The Societal Impacts of Algorithmic Decision-Making | Unavailable |
Mon, Mar 15 | 12pm - 1pm | Michelle Lee | Stanford University | - | Unavailable |
Thur, Mar 18 | 11am - 12pm | Kalesha Bullard | Georgia Institute of Technology | - | Unavailable |
Mon, Mar 22 | 11am - 12pm | Dani Yogatama | DeepMind | - | Unavailable |
Tue, Mar 23 | TBD | Alekh Agarwal | Microsoft Research | - | Unavailable |
Wed, Mar 24 | 11am - 12pm | Wilko Schwarting | MIT | - | Unavailable |
Thur, Mar 25 | 11am - 12pm | Enrique Mallada | Johns Hopkins University | Embracing Low Inertia in Power System Frequency Control: A Dynamic Droop Approach | Record |
Fri, Mar 26 | TBD | Ellen Vitercik | Carnegie Mellon University | - | Unavailable |
Wed, Mar 31 | TBD | Eric Mazumdar | University of California, Berkeley | - | Unavailable |
Thur, Apr 1 | 11am - 12pm | Benjamin K. Sovacool | University of Sussex Business School, UK | Decarbonisation and its discontents: A critical justice perspective on four low-carbon transitions | Record |
Thur, Apr 8 | 11am - 12pm | Jingjin Yu | Rutgers University | Decision Making for Many Mobile Objects | Record |
Tue, Apr 13 | 10:15am - 11:15am | Mutale Nkonde | AI for the People | Elections, Online Chatter and Content Moderation | Record |
Thur, Apr 22 | 11:00am - 12:00pm | Phillip Stanley-Marbell | University of Cambridge, UK | Newton: A Language for Describing the Physical World to Hardware Architectures and Programming Language Compilers | Record |
Thur, Apr 29 | 11am - 12pm | Inna Partin Vaisband | University of Illinois at Chicago | Deep Neural Networks that Route VLSI Systems | Record |
Tue, May 4 | 11am - 12pm | Sham Kakade | University of Washington/Microsoft Research | Towards a Theory of Generalization in Reinforcement Learning | Record |
Tue, May 11 | 12pm - 1pm | Laure Zanna | NYU | The future of climate modeling in the age of artificial intelligence | Record |
Advancing the Design of Adversarial Machine Learning Methods
Speaker: Hai (Helen) Li, Duke University
Date: Feb 4
Abstract: It has become clear that deep neural networks (DNNs) have an immense potential to learn and perform complex tasks. It is also evident that DNNs have many vulnerabilities with the potential to render them useless in complex and extended operating environments. The purpose of our research is to investigate ways in which DNN models are vulnerable to “adversarial attacks,” while also leveraging such adversarial techniques to construct more robust and reliable deep learning-based systems. We explore the potential weaknesses of DNN models by developing advanced feature space-based adversarial attacks, which create adversarial directions that are generally effective for a data distribution. The learned distributions can also be used to analyze layer-wise and model-wise transfer properties and gain insights into how feature distributions evolve with layer depth and architecture. Alternatively, we investigate the ensemble methods against transfer attacks. Our approach (namely, DVERGE) isolates the adversarial vulnerability in each sub-model by distilling non-robust features, and diversifies the adversarial vulnerability to induce diverse outputs against a transfer attack. The novel diversity metric and training procedure enables DVERGE to achieve higher robustness against transfer attacks, and enables the improved robustness when more sub-models are added to the ensemble.

About the Speaker: Hai “Helen” Li is Clare Boothe Luce Professor and Associate Chair of the Department of Electrical and Computer Engineering at Duke University. She received her B.S and M.S. from Tsinghua University and Ph.D. from Purdue University. At Duke, she co-directs Duke University Center for Computational Evolutionary Intelligence and NSF IUCRC for Alternative Sustainable and Intelligent Computing (ASIC). Her research interests include machine learning acceleration and security, neuromorphic circuit and system for brain-inspired computing, conventional and emerging memory, and software and hardware co-design. She received the NSF CAREER Award, the DARPA Young Faculty Award, TUM-IAS Hans Fischer Fellowship from Germany, ELATE Fellowship, night best paper awards and another nine best paper nominations. Dr. Li is a fellow of IEEE and a distinguished member of ACM. For more information, please see her webpage at http://cei.pratt.duke.edu/.
Building Reproducible, Reusable, and Robust Deep Reinforcement Learning Systems
Speaker: Joelle Pineau, Facebook AI Research
Date: Feb 10
Abstract: We have seen amazing achievements with machine learning in recent years. Yet reproducing results for state-of-the-art deep learning methods is seldom straightforward. Results can vary significantly given minor perturbations in the task specification, data or experimental procedure. This is of major concern for anyone interested in using machine learning in real-world applications. In this talk, I will review challenges that arise in experimental techniques and reporting procedures in deep learning, with a particular focus on reinforcement learning and applications to healthcare. I will also describe several recent results and guidelines designed to make future results more reproducible, reusable and robust.
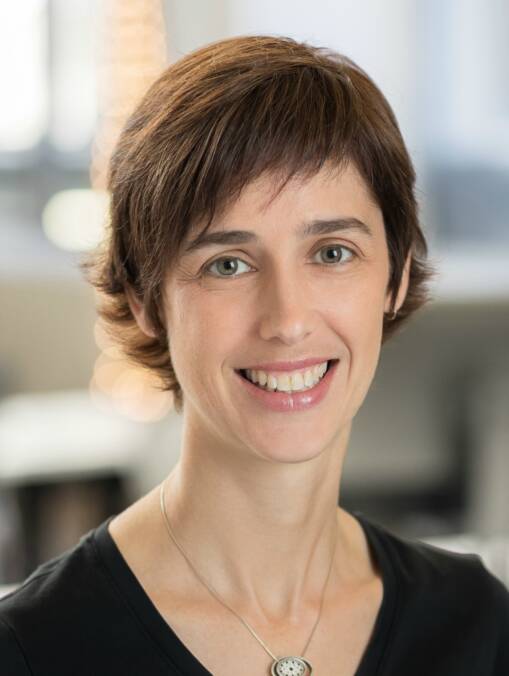
About the Speaker: Joelle Pineau is the co-Managing Director of Facebook AI Research, where she oversees the Montreal, Seattle, Pittsburgh, and Menlo Park labs. She is also a faculty member at Mila and an Associate Professor and William Dawson Scholar at the School of Computer Science at McGill University, where she co-directs the Reasoning and Learning Lab. She holds a BASc in Engineering from the University of Waterloo, and an MSc and PhD in Robotics from Carnegie Mellon University. Dr. Pineau's research focuses on developing new models and algorithms for planning and learning in complex partially-observable domains.
Establishing the Essential Hardware Primitives for Quantum-Proof Secure Computer Systems
Speaker: Michel A. Kinsy, Texas A&M University
Date: Feb 11
Abstract: In the last three years, we have witnessed a raft of breakthroughs and several key milestones towards the development of general quantum computers. These advances do bring with them critical challenges to classical cryptosystems like RSA (Rivest-Shamir-Adleman), ECC (Elliptic Curve Cryptography), and ElGamal. The strength of these algorithms rests on the hardness of integer factorization and discrete logarithm problems under the classic computing paradigm, but not under quantum computing approaches. Thus, researchers have been actively investigating new algorithms and designs for cryptosystems for the post-quantum era. Among these techniques, designs based on Ring-learning with errors (Ring-LWE) thus far have proven to be the most promising approach. In this talk, I will introduce a set of highly-optimized, parameterizable hardware modules to serve as post-quantum primitives for faster design space exploration of post-quantum cryptosystems, especially, cryptosystems using Ring-LWE algorithms. This post-quantum primitive set consist of the four frequently-used security components: the public key cryptosystem (PKC), key exchange (KEX), oblivious transfer (OT), and zero-knowledge proof (ZKP). The PKC and KEX form the basis of most modern cryptographic systems. The OT is used in many privacy-preserving applications, e.g., DNA database and machine learning. Similarly, ZKP is used in a number of applications, for example, it has been proposed as an ideal candidate for next generation blockchain algorithms. These primitives will serve as the fundamental building blocks and aid hardware designers in constructing quantum-proof secure systems in the post-quantum era.

About the Speaker: Michel A. Kinsy Michel A. Kinsy is an Associate Professor in the Department of Electrical and Computer Engineering Texas A&M University (TAMU), where he directs the Adaptive and Secure Computing Systems (ASCS) Laboratory. Dr. Kinsy is also the Associate Director of TAMU Cybersecurity Center. He focuses his research on computer architecture, hardware-level security, and efficient hardware design and implementation of post-quantum cryptography systems. Dr. Kinsy is an MIT Presidential Fellow and an Inaugural Skip Ellis Career Award recipient. He earned his PhD in Electrical Engineering and Computer Science in 2013 from the Massachusetts Institute of Technology (MIT). Before joining the TAMU faculty, Dr. Kinsy was an assistant professor in the Department of Electrical and Computer Engineering at Boston University (BU). Prior to BU, he was an assistant professor in the Department of Computer and Information Systems at the University of Oregon, where he directed the Computer Architecture and Embedded Systems (CAES) Laboratory. From 2013 to 2014, he was a Member of the Technical Staff at the MIT Lincoln Laboratory. His research contributions have been recognized with several awards, including the 2020 GLVLSI Best Paper Award, 2018 IEEE MWSCAS Myril B. Reed Best Paper Award, 2017 DFT Best Student Paper Award, and 2011 FPL Tools and Open-Source Community Service Award.
Accelerating Biology and Medicine with Hardware Specialization
Speaker: Yatish Turakhia, UC Santa Cruz
Date: Feb 18
Abstract: Genome sequencing data is rising exponentially at a rate (125%/year) that is far higher than our current transistor performance scaling (currently 3%/year). New medical and comparative genomics applications have emerged that ensure that the demand for more sequencing will continue to rise, which threatens to overwhelm our current computing capacities. Domain-specific acceleration (DSA), i.e. using specialized hardware for accelerating a narrow domain of algorithms, will enable us to tap the vast potential of this data by providing massive gains in performance efficiency.
In this talk, I will first present the designs of our past co-processors as case-studies for how domain-specific hardware can provide massive speedup (1,000-10,000x) for genomic applications and the various sources of increased efficiency. In the second half of my talk, I will show how this approach would be indispensable to solve a multitude of emerging problems in biology and medicine (including our fight against the current and future pandemics), many of which we will be tackling at my future lab at UC San Diego (UCSD).
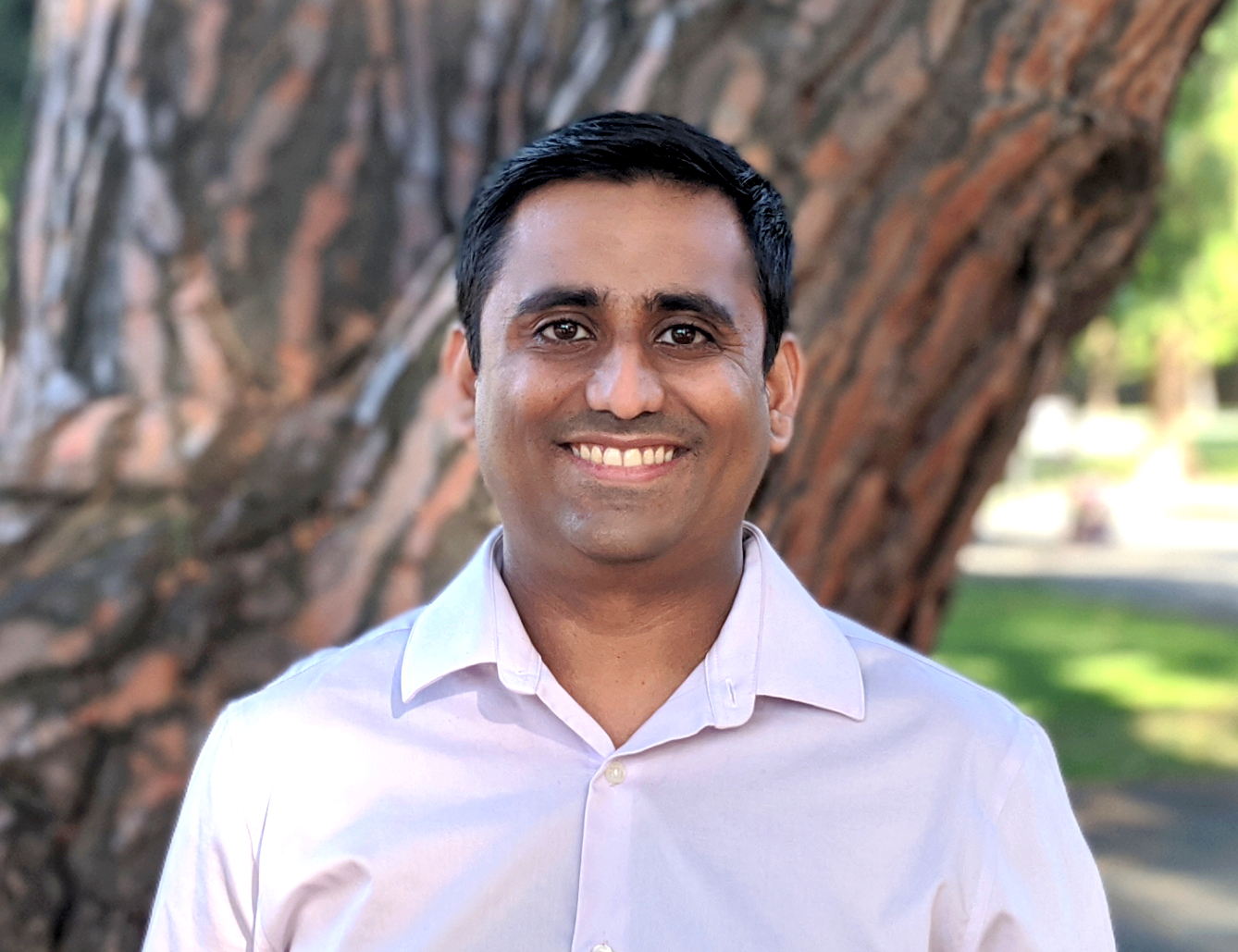
About the Speaker: Dr. Yatish Turakhia is a postdoctoral researcher at Genomics Institute, UC Santa Cruz, where he is jointly advised by Prof. David Haussler and Prof. Benedict Paten. He will be joining the University of California San Diego (UCSD) in July 2021 as an Assistant Professor in the Department of Electrical and Computer Engineering (ECE). His research interests are in developing hardware accelerators and algorithms for faster and cheaper genomic analysis. Dr.Turakhia obtained his Ph.D. from Stanford University in 2019, where he was jointly advised by Prof. Bill Dally and Prof. Gill Bejerano. His work has won the best paper award at ASPLOS 2018 and IEEE Micro Top Picks award 2018. He is also a past recipient of the NVIDIA Graduate Fellowship.
Towards scalable integration of distributed energy resources with packetized energy management
Speaker: Mads Almassalkhi, University of Vermont
Date: Feb 18
Abstract: This talk presents recent results on modeling and control for integrating distributed energy resources (DERs), such as kW-scale smart appliances, into T&D grid operations to enable reliable grid operation under high penetrations of renewables. The focus of the talk will be on a bottom-up/device-driven scheme called Packetized Energy Management (PEM) for aggregating and coordinating DERs, such as electric water heaters, electric vehicles, and battery storage. PEM leverages key methods from communication networks that already enable billions of people to access the internet and adapts these methods to managing fleets of smart loads. For example, in the same way that a bulky data file gets split up into smaller data packets, PEM delivers an electric water heater’s energy need in multiple small "energy packets” rather than a single “bulky"; delivery. Under PEM, local “packetizing” control enables the DERs to asynchronously request energy packets from a demand coordinator, who can then choose to accept or deny the packet requests in real time. By modulating the rate of accepting packet requests from a fleet of water heaters, the demand coordinator can then dispatch the aggregate demand as if it was a bulk energy storage resource. With PEM’s bottom-up framework, we overcome complications with modeling and estimating the complex end-consumer usage patterns and can guarantee privacy for the end-consumer, which makes PEM particularly promising for managing a large, diverse, and heterogeneous fleet of resources across different timescales.
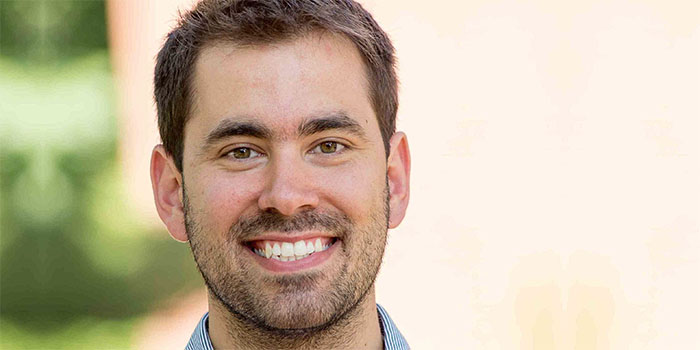
About the Speaker: Since 2014, Mads Almassalkhi has been Assistant Professor in the Department of Electrical and Biomedical Engineering at the University of Vermont. He is also co-founder of clean-tech startup Packetized Energy that is commercializing technology related to PEM. His research interests lie at the intersection of power systems, mathematical optimization, and control systems and focus on developing scalable algorithms that improve responsiveness and resilience of power systems with a recent focus on advanced distribution system operations. He is the Chair of the IEEE PES Smart Buildings, Loads, and Customer Systems (SBLC) technical subcommittee on Loads. He was awarded the NSF CAREER in 2021 and was named the Outstanding Junior Faculty award in his college in 2016. Prior to joining the University of Vermont, he was lead systems engineer at another energy startup company Root3 Technologies. Before that, he received his PhD from the University of Michigan in Electrical Engineering (EE): Systems in 2013 and a dual major in Electrical Engineering and Applied Mathematics at the University of Cincinnati in Ohio in 2008. When he is not working on energy problems or teaching, he is spending his time with his amazing wife and their three small children.
Advancements and Challenges for Deep Learning in Medical Imaging
Speaker: Pranav Rajpurkar, Stanford University
Date: Feb 24
Abstract: There have been rapid advances at the intersection of AI and medicine over the last few years, especially for the interpretation of medical images. In this talk, I will describe three key directions that present challenges and opportunities for the development of deep learning technologies for medical image interpretation. First, I will discuss the development of transfer learning and self-supervised learning algorithms designed to work in low labeled medical data settings. Second, I will discuss the design and curation of large, high-quality datasets and their roles in advancing algorithmic developments. Third, I will discuss the real-world impact of AI technologies on clinicians’ decision making and subtleties for the promise of expert-AI collaboration. Altogether I will summarize key recent contributions and insights in each of these directions with key applications across medical specialties.
About the Speaker: Pranav Rajpurkar is a final year PhD candidate in Computer Science at Stanford, where he works on building reliable artificial intelligence (AI) technologies for medical decision making. Pranav’s work has been published in 30+ peer-reviewed publications in both scientific journals and AI conferences (receiving over 7000 citations) and has been covered by media outlets including NPR, The Washington Post, and WIRED. Pranav founded the AI for Healthcare Bootcamp at Stanford, where he has worked closely with and mentored over 100 Stanford students and collaborated with 18 faculty members on various research projects. He designed and instructed the Coursera course series on AI for Medicine, now with 40,000+ students. Pranav’s PhD was jointly advised by Dr. Andrew Ng and Dr. Percy Liang at Stanford University, where Pranav also received both his Bachelors and Masters Degrees in Computer Science.
STEPS TOWARDS MAKING MACHINE LEARNING MORE NATURAL
Speaker: Mengye Ren, University of Toronto
Date: Mar 1
Abstract: Over the past decades, we have seen machine learning making great strides in AI applications. Yet, most of its success relies on training models offline on a massive amount of data and evaluating them in a similar test environment. By contrast, humans can learn new concepts and skills with very few examples, and can easily generalize to novel tasks. In this talk, I will highlight three key steps towards making machines learning more human-like, and these steps will unlock the next generation of technologies. The first step is to make machines learn new concepts continually and incrementally using limited labeled data. The second step is to develop flexible representations that can generalize well to novel concepts under different contexts. Finally, I’ll show how to make abstract and compositional reasoning given visual inputs. I will then conclude with an outlook of future directions towards building a more general and flexible AI.
About the Speaker: Mengye Ren is a PhD student in the machine learning group of the Department of Computer Science at the University of Toronto. He was also a research scientist at Uber ATG working on self-driving cars from 2017 to 2021. His research focuses on making machines learn in more naturalistic environments with less labeled data. He has won a number of awards including two NVIDIA research pioneer awards and the Alexander Graham Bell Canada Graduate Fellowship.
Hardware Security in Three Dimensional (3D) Integrated Circuits and Systems
Speaker: Qiaoyan Yu, University of New Hampshire
Date: Mar 4
Abstract: Three-dimensional (3D) integration is emerging as promising techniques for high-performance and low-power integrated circuit (IC) design. As 3D chips require more manufacturing phases than conventional planar ICs, more fabrication foundries are involved in the supply chain of 3D ICs. Due to the globalized semiconductor business model, the extended IC supply chain could incur more security challenges on maintaining the integrity, confidentiality, and reliability of integrated circuits and systems. In this talk, we analyze the potential security threats induced by the integration techniques for stacked 3D and monolithic 3D (M3D) ICs and propose effective attack detection and mitigation methods. More specifically, we first propose a comprehensive characterization models for 3D hardware Trojans in the 3D stacking structure. Practical experiment based quantitative analyses have been to assess the impact of 3D Trojans on computing systems. Next, we develop two 3D Trojan detection methods. The proposed frequency-based Trojan-activity identification (FTAI) method can differentiate the frequency changes induced by Trojans from those caused by process variation noise, outperforming the existing time-domain Trojan detection approaches. Our invariance checking based Trojan detection method leverages the invariance among the 3D communication infrastructure, 3D network-on-chips (NoCs), to tackle the cross-tier 3D hardware Trojans. Furthermore, this work investigates another type of common security threats, side-channel attacks. We first demonstrate the impact of the noise in power distribution network (PDN) on the resilience against correlation power analysis (CPA) attack. Then, we propose to utilize the supply voltages of different 3D tiers to jointly drive the crypto unit such that the induced supply noise can obfuscate the original power trace and thus mitigate CPA attacks.

About the Speaker: Dr. Qiaoyan Yu is Associate Professor of Electrical and Computer Engineering at the University of New Hampshire, where she also directs the Reliable & Secure VLSI Systems Laboratory. Dr. Yu’s research expertise includes hardware Trojan detection, side-channel attack mitigation, 3DIC and embedded system security, cybersecurity, and VLSI fault tolerance. Dr. Yu received her B.S. from Xidian University (2002), M.S. in Communication and Information Engineering from Zhejiang University (2005), and the Ph.D. in Electrical and Computer Engineering from the University of Rochester (2011). Dr. Yu’s research expertise includes hardware security with special emphases on integrated circuit security, FPGA security, embedded system security, Internet-of-Things (IoT) security, approximate computing security, and Networks-on-Chip architecture for fault tolerance and error management.
Dr. Yu received the NSF CAREER Award and the Air Force Research Lab Faculty Fellowship in 2017. Her work was also supported Semiconductor Research Corporation (SRC) and UNH NSF Nanomanufacturing Center. She received the Best Poster Award at ISVLSI’16, Best Paper Award Finalist in MWSCAS’15, Best Paper Award Finalist in NOCS’11, and the Best ECE Ph.D. Dissertation Award at the University of Rochester in 2011. She received the Excellence in Teaching Award at UNH in 2015. She has served on the technical program committees of HOST, Asian HOST, DAC, ASP-DAC, GLSVLSI, ISVLSI, DFT, ISCAS, MWSCAS, and ICCD.
Machine Learning for Personalised Healthcare: Opportunities, Challenges and Insights
Speaker: Danielle Belgrave and Niranjani Prasad, Microsoft Research Cambridge
Date: Mar 10
Abstract: Machine learning advances are opening new routes to more precise healthcare, from the discovery of disease subtypes for stratified interventions to the development of tailored sequences of interaction. These methods offer an exciting opportunity to have a meaningful impact on the delivery of healthcare. In this talk, we will present some of the inroads of machine learning for understanding and learning personalised interventions. Taking examples from mental health, respiratory disease and critical care settings, we present some of the opportunities and inherent challenges to leveraging machine learning in healthcare towards actionable insights.

About the Speaker: Dr Danielle Belgrave is a machine learning researcher in the Healthcare Intelligence group at Microsoft Research, in Cambridge (UK) where she works on Project Talia. Her research focuses on integrating medical domain knowledge, probabilistic graphical modelling and causal modelling frameworks to help develop personalized treatment and intervention strategies for mental health. Mental health presents one of the most challenging and under-investigated domains of machine learning research. In Project Talia, we explore how a human-centric approach to machine learning can meaningfully assist in the detection, diagnosis, monitoring, and treatment of mental health problems. She obtained a BSc in Mathematics and Statistics from London School of Economics, an MSc in Statistics from University College London and a PhD in the area of machine learning in health applications from the University of Manchester. Prior to joining Microsoft, she was a tenured Research Fellow at Imperial College London.

Dr Niranjani Prasad is a senior researcher in the Health Intelligence team at Microsoft Research Cambridge, developing methods to guide personalized interventions in online mental health services. Her research draws on machine learning frameworks for automated decision support such as reinforcement learning and causal inference. She obtained her undergraduate degree (BA,MEng) in Information and Computer Engineering from the University of Cambridge. Prior to joining Microsoft, she completed her PhD in Computer Science at Princeton University, advised by Professor Barbara Engelhardt, where her work centred on clinician-in-loop sequential decision-making in the critical care setting.
Data-powered smart grids and communities
Speaker: Kyri A. Baker, University of Colorado Boulder
Date: Mar 11
Abstract: In this talk, we discuss how machine learning can be used to operate large-scale electric power grids more effectively, and how reinforcement learning can be used to design dynamic prices for price-responsive communities. In the first part of the talk, a deep neural network is designed to optimize power grids in real-time, providing feasible and near-optimal solutions on faster timescales than off-the-shelf optimization solvers. Solving these large scale optimization problems faster is important as increasing amounts of quickly fluctuating renewable energy is introduced to our energy supply. The second part of the talk considers a community that does not share any preferences or information other than smart meter readings from the distribution substation with the aggregator, which uses reinforcement learning to intelligently design dynamic electricity prices.

About the Speaker: Dr. Kyri Baker received her B.S., M.S., and Ph.D. in Electrical and Computer Engineering from Carnegie Mellon University in 2009, 2010, and 2014, respectively. From 2015 to 2017, she worked at the National Renewable Energy Laboratory. Since Fall 2017, she has been an Assistant Professor at the University of Colorado Boulder, and is a Fellow of the Renewable and Sustainable Energy Institute (RASEI). Her research focuses on renewable energy integration by changing the way the electric power grid operates. In particular, she develops computationally efficient optimization and learning algorithms for energy systems ranging from building-level assets to transmission grids.
Embracing Low Inertia in Power System Frequency Control: A Dynamic Droop Approach
Speaker: Enrique Mallada, Johns Hopkins University
Date: Mar 25
Abstract: The transition into renewable energy sources -with limited or no inertia- is seen as potentially threatening to classical methods for achieving grid synchronization. A widely embraced approach to mitigate this problem is to mimic inertial response using grid-connected inverters. That is, introduce virtual inertia to restore the stiffness that the system used to enjoy. In this talk, we seek to challenge this approach and advocate towards taking advantage of the system’s low inertia to restore frequency steady-state without incurring excessive control efforts. With this aim in mind, we develop an analysis and design framework for inverter-based frequency control. We define several performance metrics of practical relevance for power engineers and systematically evaluate the performance of standard control strategies, such as virtual inertia and droop control, in the presence of power disturbances. Our analysis unveils the relatively limited role of inertia on improving performance as well as the inability of droop control to improve performance without incurring large steady-state control efforts. To solve this problem, we propose a novel dynamic droop control for grid-connected inverters -exploiting classical lead/lag compensation and model matching techniques from control theory- that can significantly outperform existing solutions with comparable control efforts.
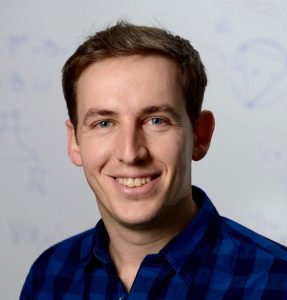
About the Speaker: Enrique Mallada is an assistant professor of electrical and computer engineering at Johns Hopkins University since 2016. Before joining Hopkins, he was a post-doctoral fellow at the Center for the Mathematics of Information at the California Institute of Technology from 2014 to 2016. He received his ingeniero en telecomunicaciones (telecommunications engineering) degree from Universidad ORT, Uruguay, in 2005 and his Ph.D. degree in electrical and computer engineering with a minor in applied mathematics from Cornell University in 2014. Dr. Mallada was awarded the Catalyst Award and Discovery Award from Johns Hopkins in 2020 and 2019, the NSF CAREER award in 2018, the ECE Director's Ph.D. Thesis Research Award for his dissertation in 2014, the Cornell University's Jacobs Fellowship in 2011, and the Organization of American States scholarship from 2008 to 2010. His research interests lie in the areas of control, networked dynamics, and optimization, with applications to power networks and the Internet.
Decarbonisation and its discontents: A critical justice perspective on four low-carbon transitions
Speaker: Benjamin K. Sovacool, Johns Hopkins University
Date: Apr 1
Abstract: What are the types of injustices associated with low-carbon transitions? Relatedly, in what ways do low-carbon transitions worsen social risks or vulnerabilities? Lastly, what policies might be deployed to make these transitions more just? The presentation answers these questions by first elaborating an “energy justice” framework consisting of four distinct dimensions—distributive justice (costs and benefits), procedural justice (due process), cosmopolitan justice (global externalities), and recognition justice (vulnerable groups). It then examines four European low-carbon transitions—nuclear power in France, smart meters in Great Britain, electric vehicles in Norway, and solar energy in Germany—through this critical justice lens. In doing so, it draws from original data collected from 64 semi-structured interviews with expert participants as well as five public focus groups and the monitoring of twelve internet forums. It documents 120 distinct energy injustices across these four transitions. It then explores two exceedingly vulnerable groups to European low-carbon transitions, those recycling electronic waste flows in Ghana, and those mining for cobalt in the Democratic Republic of the Congo. The presentation aims to show how when low-carbon transitions unfold, deeper injustices related to equity, distribution, and fairness invariably arise.
About the Speaker: Dr. Benjamin K. Sovacool is Professor of Energy Policy at the Science Policy Research Unit (SPRU) at the University of Sussex Business School in the United Kingdom. There he serves as Director of the Sussex Energy Group. He is also University Distinguished Professor of Business & Social Sciences at Aarhus University in Denmark. Professor Sovacool works as a researcher and consultant on issues pertaining to energy policy, energy justice, energy security, climate change mitigation, and climate change adaptation. More specifically, his research focuses on renewable energy and energy efficiency, the politics of large-scale energy infrastructure, the ethics and morality of energy decisions, designing public policy to improve energy security and access to electricity, and building adaptive capacity to the consequences of climate change. With much coverage of his work in the international news media, he is one of the most highly cited global researchers on issues bearing on controversies in energy and climate policy.
Decision Making for Many Mobile Objects
Speaker: Jingjin Yu, Rutgers University
Date: Apr 8
Abstract: Systems composed of many mobile bodies (e.g., robots or movable objects) are often highly complex, due to interactions among the bodies, and between the bodies and the environment. For example, in a multi-robot motion planning scenario, applicable to warehouse/port automation, biomedical research, and autonomous driving settings, as the density of robots increases, it becomes increasingly difficult to coordinate robots’ collision-free motion while simultaneously seeking to optimize the overall system throughput. Alternatively, in a setting where many objects must be manipulated and rearranged, many types of object-object interdepencies arise, causing combinatorial explosions that must be dealt with. In this talk, I will discuss several practical decision-making problems for systems containing many mobile objects, examine the involved computational challenges, and highlight state-of-the-art algorithmic solutions for dealing with these challenges.
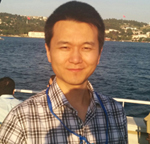
About the Speaker: Jingjin Yu is an Assistant Professor in the Department of Computer Science at Rutgers University. He received his B.S. from the University of Science and Technology of China (USTC), and obtained his M.S. in Computer Science and Ph.D. in Electrical and Computer Engineering, both from the University of Illinois, where he briefly stayed as a postdoctoral researcher. Before joining Rutgers, he was a postdoctoral researcher at the Massachusetts Institute of Technology. He is broadly interested in the area of algorithmic robotics and control, focusing on issues related to optimality, complexity, and the design of efficient decision-making methods. He is a recipient of the NSF CAREER award.
Elections, Online Chatter and Content Moderation
Speaker: Mutale Nkonde, AI for the People
Date: Apr 13
Abstract: The talk centers on the work done by AI for the People on racially targeted disinformation on Twitter during the 2020 Election and the challenges we faced communicating this to trust and safety teams because of their lack of how to read online culture through speech. The talk will introduce listeners to how the environment changed from 2016 to 2020, our findings detailed here and end with recommendations on how to increase the racial literacy of computer scientists working in industry settings.
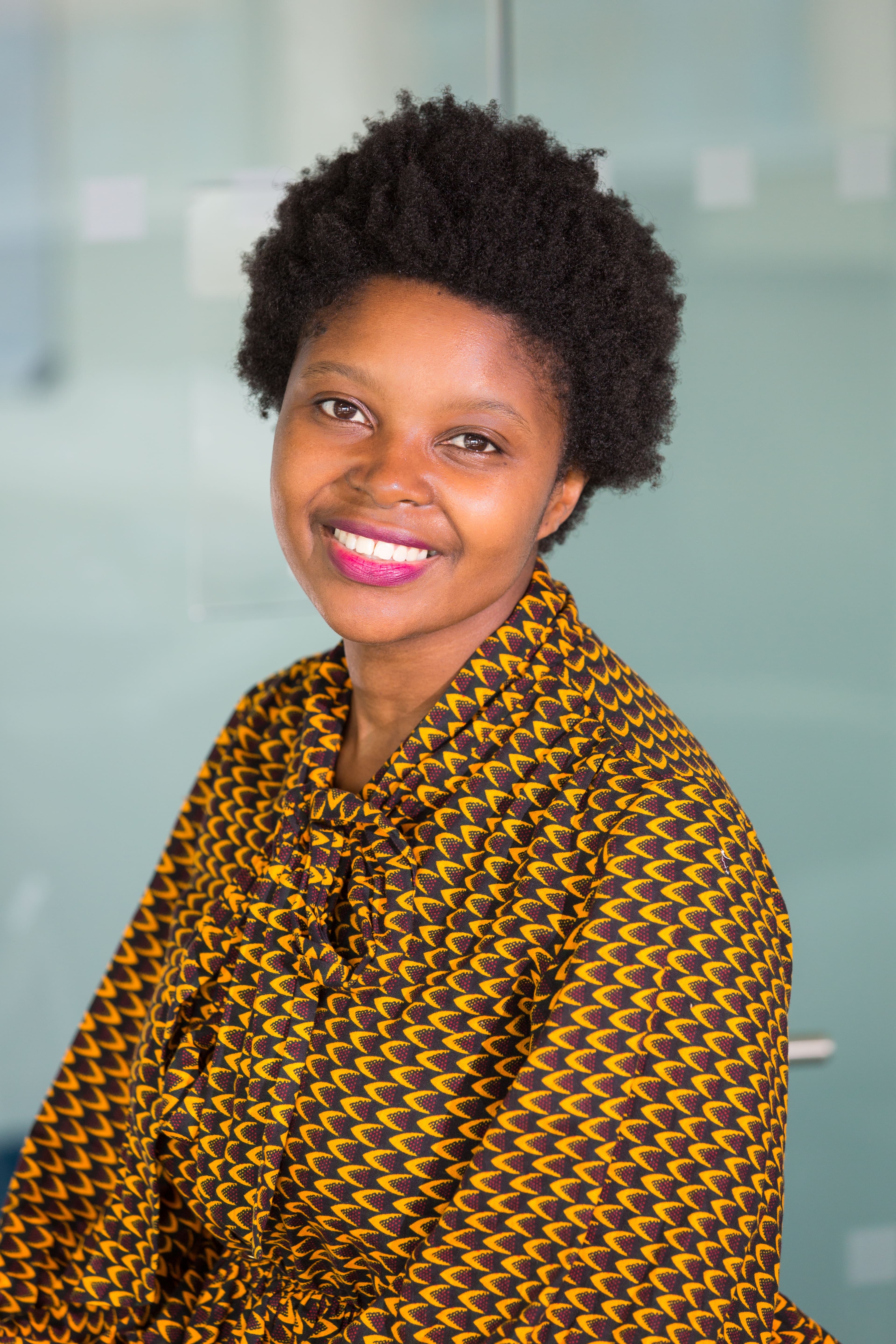
About the Speaker: Mutale Nkonde is the founding director of AI for the People, a non profit communications firm that uses journalism, arts and culture to advance racial justice in tech. During the 2020 presidential election her team identified a disinformation network targeting Black voters in the Philadelphia news ecosystem, and published the findings in the Harvard Kennedy School's Misinformation Review, read it here . In 2021 AI for the People launched their biometric justice vertical by producing a film supporting a ban of facial recognition in New York State, in partnership with Amnesty International, watch it here . Nkonde writes widely on racial impacts of advanced technical systems, is a widely sought after media commentator and seeks to create a safe space for Black technologists who feel marginalized within the wider tech sector.
Prior to this she lead a team that introduced the Algorithm and Deepfakes Accountability Acts and the No Biometric Barriers Act to the US House of Representatives in 2019 and started her career as a broadcast journalist before transitioning into the world of tech. She currently sits on the Tik Tok Content Moderation Advisory Board, advises the Center of Media, Technology and Democracy at McGill University and is a key constituent for the UN 3C Table on AI.
Newton: A Language for Describing the Physical World to Hardware Architectures and Programming Language Compilers
Speaker: Phillip Stanley-Marbell, University of Cambridge, UK
Date: Apr 22
Abstract: This talk will describe several research threads (and their results) built on the Newton language and its compiler infrastructure. Newton is a language for specifying invariants about the signals, physical materials properties, and other constraints on physical systems and signals with the objective of making this information usable by computer architectures and programming language compilers. Ongoing research efforts building on the concepts enabled by Newton include new approaches to efficient machine learning model training and inference for models involving physical signals, enabling speedups in both training and inference of existing machine learning methods [Tsoutsouras et al., 2021]. Other examples of research building on Newton include automated synthesis of state estimators (such as both linear and extended Kalman filters) [Kaparounakis et al., 2020], automated controller synthesis for robotics [Pirron et al., 2020], and new ongoing research on materials-inspired program transformations (and data-inspired materials formulation and design) to enable ultra-miniature computing systems embedded in 3D-printed structures [EP/V004654/1].
[Pirron et al., 2020] M. Pirron, D. Zufferey and P. Stanley-Marbell, "Automated Controller and Sensor Configuration Synthesis Using Dimensional Analysis," in IEEE Transactions on Computer-Aided Design of Integrated Circuits and Systems, vol. 39, no. 11, pp. 3227-3238, Nov. 2020.
[Kaparounakis et al., 2020] Kaparounakis, Orestis, Vasileios Tsoutsouras, Dimitrios Soudris, and Phillip Stanley-Marbell. "Automated physics-derived code generation for sensor fusion and state estimation." arXiv preprint arXiv:2004.13873 (2020).
[Tsoutsouras et al., 2021] V. Tsoutsouras, S. Willis, and P. Stanley-Marbell "Deriving Equations from Sensor Data Using Dimensional Function Synthesis”, Communications of the ACM, vol. 64, no. 8, August 2021.
[EP/V004654/1] Programmable Sensing Composites Project, 2020 to 2022. https://gow.epsrc.ukri.org/NGBOViewGrant.aspx?GrantRef=EP/V004654/1
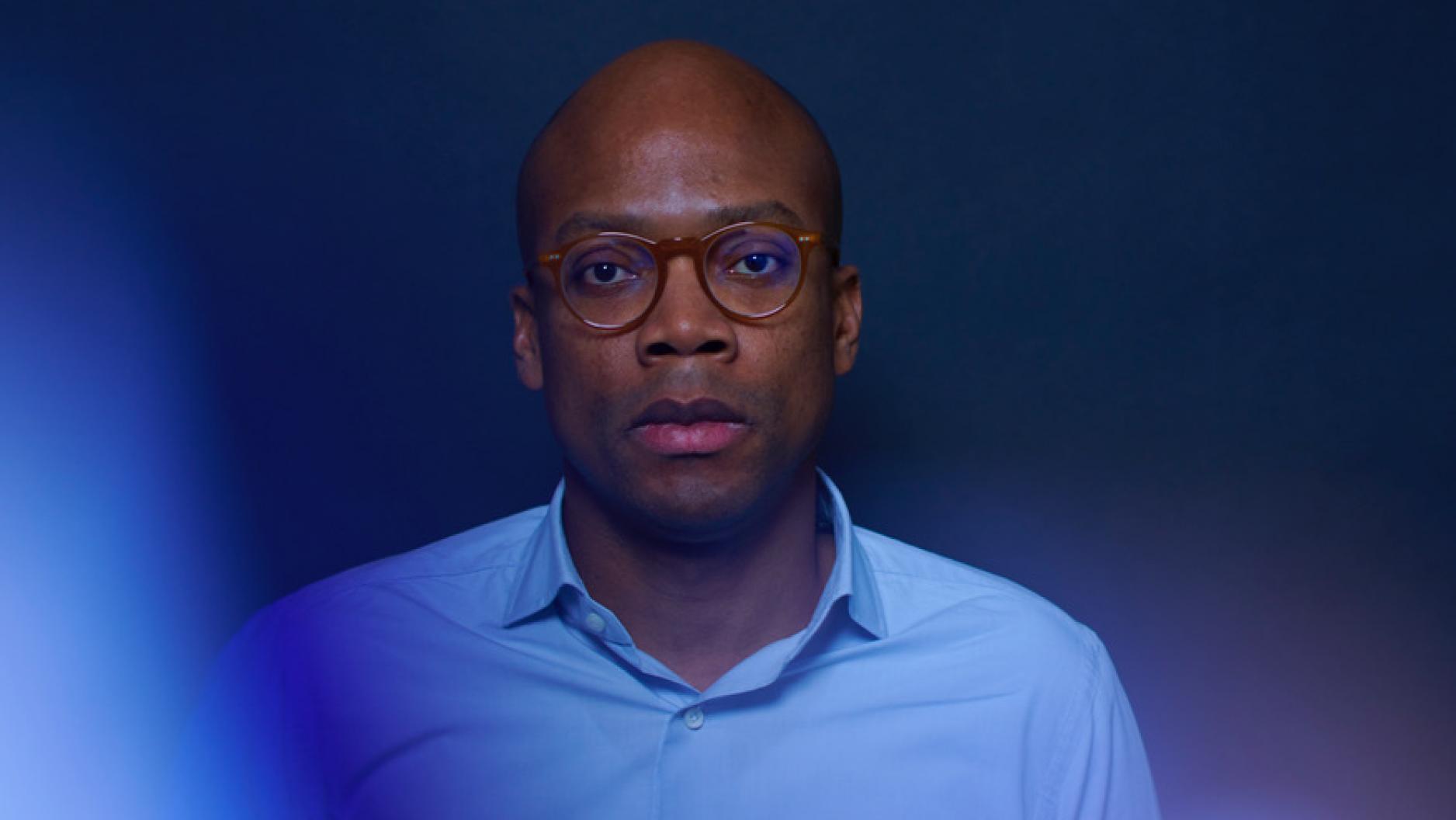
About the Speaker: Phillip Stanley-Marbell is an Associate Professor in the Department of Engineering at the University of Cambridge, UK, where he leads the Physical Computation Laboratory. He also holds an appointment as a Faculty Fellow at the Alan Turing Institute for Artificial Intelligence and Data Science in London. Prior to moving to the UK in 2017, he was a researcher in the Computer Science and Artificial Intelligence Laboratory (CSAIL) at MIT. From 2012 to 2014, he was with the Core OS organization at Apple (Cupertino, USA) where he led the development of new system components for iOS, macOS, and watchOS that enable on-device machine learning. The work is captured in eight granted patents for technologies in Apple products and is incorporated into all Apple’s products shipping today. Prior to Apple, he spent several years (2008–2012) as a permanent research staff member at IBM Research in Zürich, Switzerland. He completed his Ph.D. at Carnegie Mellon University (Pittsburgh, USA) in 2007, spending 2006–2008 at Technische Universiteit Eindhoven in the Netherlands. Before his Ph.D., he spent several summers as an intern or full-time engineer at Bell Labs: in the Microelectronics division with a group that designed ASICs for telephony applications (1995, 1996) and with the Data Networking division (1999), in a project spun out of the group that created UNIX, doing work with the Inferno Operating System. His research focuses on investigating methods to use properties of physical systems to improve the efficiency of computation on data from nature. His research has led to several best paper nominations and awards (IEEE ESWEEK / Transactions on Embedded Computing Systems, ACM Computing Surveys), research highlights in the ACM’s flagship Communications of the ACM journal (CACM, 2021), as well as multiple articles covering his research in the mainstream media (e.g., Fast Company 2019, Wired Magazine 2020). He is the author of over 60 peer-reviewed publications and three textbooks.
Deep Neural Networks that Route VLSI Systems
Speaker: Inna Partin-Vaisband, University of Illinois at Chicago
Date: Apr 29
Abstract: There is a pressing need in modern VLSI systems to shift electronic design automation (EDA) toward “no human in the loop” and “24-hour turnaround time” paradigms. Inspired by recent AI advances, there has been a growing interest in AI enhanced design of integrated circuits (ICs). Yet, reducing the overall EDA turnaround time is limited by the series nature of some traditional EDA algorithms. In this talk, I will focus on global routing – a highly complex and computationally expensive IC design step. First, I will explain the challenges of speeding global routing with traditional algorithms. Then, I will draw an analogy between traditional routing and classical image inpainting and will discuss its speedup opportunities. Finally, I will describe a learning framework to solve such a task with focus on the design of training datasets, deep learning architectures, and cost functions for this fundamentally novel routing approach. I will conclude with a prospect of utilizing AI toward mitigation of exponentially increasing IC design costs.
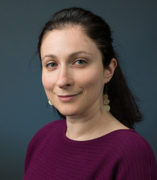
About the Speaker: Inna Partin-Vaisband received her B.Sc. in computer engineering and M.Sc. in electrical engineering from the Technion-Israel Institute of Technology, Haifa, Israel, in, respectively, 2006 and 2009, and the Ph.D. in electrical engineering from the University of Rochester, Rochester, NY in 2015. She is currently with the Department of Electrical and Computer Engineering at the University of Illinois at Chicago, where she is an Assistant Professor and the Director of High-Performance Circuits and Systems Laboratory. Between 2003 and 2009, she held a variety of software and hardware research and development positions at Tower Semiconductor, G-Connect, and IBM, all in Israel. Her research and teaching interests focus on design of digital and mixed-signal microelectronic systems with application to high performance and low-power portable processors, hardware security, and integrated AI systems. Special emphasis has been placed on distributed power delivery and locally intelligent power management, which is described in her book, On-Chip Power Delivery and Management, 4th Edition. She is a member of the editorial board of the Microelectronics Journal.
Towards a Theory of Generalization in Reinforcement Learning
Speaker: Sham Kakade, University of Washington/Microsoft Research
Date: May 4
Abstract: A fundamental question in the theory of reinforcement learning is what properties govern our ability to generalize and avoid the curse of dimensionality. With regards to supervised learning, these questions are well understood theoretically, and, practically speaking, we have overwhelming evidence on the value of representational learning (say through modern deep networks) as a means for sample efficient learning. Providing an analogous theory for reinforcement learning is far more challenging, where even characterizing the representational conditions which support sample efficient generalization is far less well understood.
This work will survey a number of recent advances towards characterizing when generalization is possible in reinforcement learning. We will start by reviewing this question in a simpler context, namely contextual bandits. Then we will move to lower bounds and consider one of the most fundamental questions in the theory of reinforcement learning, namely that of linear function approximation: suppose the optimal Q-function lies in the linear span of a given d dimensional feature mapping, is sample-efficient reinforcement learning (RL) possible? Finally, we will cover a new set of structural and representational conditions which permit generalization in reinforcement learning in a wide variety of settings through the use of function approximation.
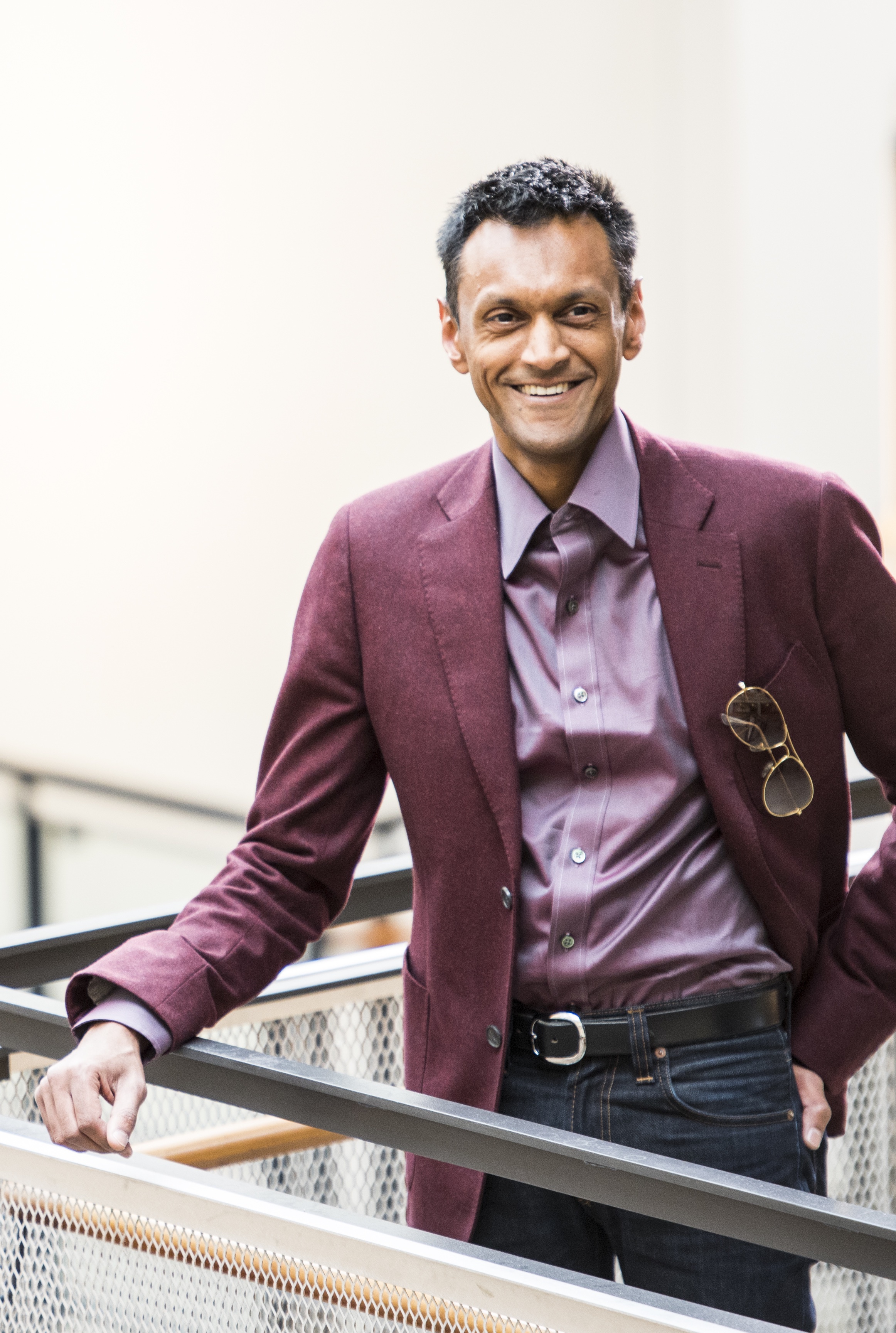
About the Speaker: Sham Kakade is a professor in the Department of Computer Science and the Department of Statistics at the University of Washington and is also a senior principal researcher at Microsoft Research. He works on the mathematical foundations of machine learning and AI. Sham's thesis helped lay the statistical foundations of reinforcement learning. With his collaborators, his additional contributions include: one of the first provably efficient policy search methods in reinforcement learning; developing the mathematical foundations for the widely used linear bandit models and the Gaussian process bandit models; the tensor and spectral methodologies for provable estimation of latent variable models; the first sharp analysis of the perturbed gradient descent algorithm, along with the design and analysis of numerous other convex and non-convex algorithms. He is the recipient of the ICML Test of Time Award, the IBM Pat Goldberg best paper award, and INFORMS Revenue Management and Pricing Prize. He has been program chair for COLT 2011.
Sham was an undergraduate at Caltech, where he studied physics and worked under the guidance of John Preskill in quantum computing. He completed his Ph.D. with Peter Dayan in computational neuroscience at the Gatsby Computational Neuroscience Unit. He was a postdoc with Michael Kearns at the University of Pennsylvania.
The future of climate modeling in the age of artificial intelligence
Speaker: Laure Zanna, NYU
Date: May 11
Abstract: Numerical simulations used for weather and climate predictions solve approximations of the governing laws of fluid motions on a grid. Ultimately, uncertainties in climate predictions originate from the poor or lacking representation of processes, such as turbulence, clouds that are not resolved on the grid of global climate models. The representation of these unresolved processes has been a bottleneck in improving climate predictions.
The explosion of climate data and the power of machine learning algorithms are suddenly offering new opportunities: can we deepen our understanding of these unresolved processes and simultaneously improve their representation in climate models to reduce climate projections uncertainty?
In this talk, I will discuss the current state of climate modeling and projections and its future, focusing on the advantages and challenges of using machine learning for climate modeling. I will present some of our recent work in which we leverage tools from machine learning and deep learning to learn representations of unresolved processes and improve climate simulations. Our work suggests that machine learning could open the door to discovering new physics from data and enhance climate predictions.
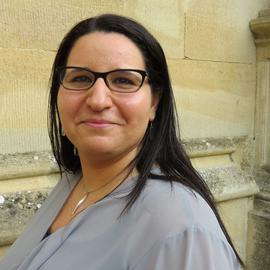
About the Speaker: Laure Zanna is a Professor in Mathematics & Atmosphere/Ocean Science at the Courant Institute, New York University. Her research focuses on the dynamics of the climate system and the main emphasis of her work is to study the influence of the ocean on local and global scales. Prior to NYU, she was a faculty member at the University of Oxford until 2019, and obtained her PhD in 2009 in Climate Dynamics from Harvard University. She was the recipient of the 2020 Nicholas P. Fofonoff Award from the American Meteorological Society “For exceptional creativity in the development and application of new concepts in ocean and climate dynamics”. She is the lead principal investigator of the NSF-NOAA Climate Process Team on Ocean Transport and Eddy Energy, and M2LInES – an international effort to improve climate models with scientific machine learning. She currently serves as an editor for the Journal of Climate, a member on the International CLIVAR Ocean Model Development Panel, and on the CESM Advisory Board.