Spring 2019 Seminars
A complete listing
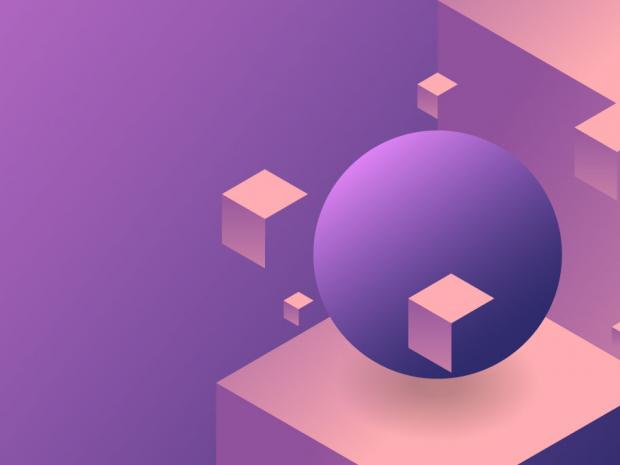
Spectrum Scarcity through Hybrid Optical and Radio-Frequency Wireless Networks
Speaker: Mohamed-Slim Alouini, King Abdullah University of Science and Technology (KAUST)
Time: 11:00 am - 12:00 pm Jan 17, 2019
Location: 2MTC, 10.099, Brooklyn, NY
Abstract: Rapid increase in the use of wireless services over the last two decades has led the problem of the radio-frequency (RF) spectrum exhaustion. More specifically, due to this RF spectrum scarcity, additional RF bandwidth allocation, as utilized in the recent past, is not anymore, a viable solution to fulfill the demand for more wireless applications and higher data rates. The talk goes first over the potential offered by optical wireless (OW) communication systems to relieve spectrum scarcity. It then summarizes some of the challenges that need to be surpassed before such kind of systems can be deployed. Finally, the talk offers two recent studies illustrating how supplementing OW networks with RF backup access points increases these networks reliability and coverage while maintain their high capacity.

About the Speaker: Mohamed-Slim Alouini was born in Tunis, Tunisia. He received the Ph.D. degree in Electrical Engineering from the California Institute of Technology (Caltech), Pasadena, CA, USA, in 1998. He served as a faculty member in the University of Minnesota, Minneapolis, MN, USA, then in the Texas A&M University at Qatar, Education City, Doha, Qatar before joining King Abdullah University of Science and Technology (KAUST), Thuwal, Makkah Province, Saudi Arabia as a Professor of Electrical Engineering in 2009.
Jack Keil Wolf Lecture Series: Guessing Random Additive Noise Decoding (GRAND)
Speaker: Muriel Medard, MIT
Time: 2:00 pm - 3:00 pm Jan 24, 2019
Location: 2MTC Room 9.009, Brooklyn, NY
Abstract: We introduce a new algorithm for Maximum Likelihood (ML) decoding based on guessing noise. The algorithm is based on the principle that the receiver rank orders noise sequences from most likely to least likely. Subtracting noise from the received signal in that order, the first instance that results in an element of the code-book is the ML decoding. For common additive noise channels, we establish that the algorithm is capacity achieving for uniformly selected code-books, providing an intuitive alternate approach to the channel coding theorem. When the code-book rate is less than capacity, we identify exact asymptotic error exponents as the block-length becomes large. We illustrate the practical usefulness of our approach in terms of speeding up decoding for existing codes.
Joint work with Ken Duffy, Kishori Konwar, Jiange Li, Prakash Narayana Moorthy, Amit Solomon.
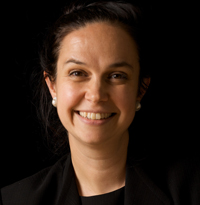
About the Speaker: Muriel Medard is the Cecil H. Green Professor in the Electrical Engineering and Computer Science (EECS) Department at MIT and leads the Network Coding and Reliable Communications Group at the Research Laboratory for Electronics at MIT. She has co-founded three companies to commercialize network coding, CodeOn, Steinwurf and Chocolate Cloud. She has served as editor for many publications of the Institute of Electrical and Electronics Engineers (IEEE), of which she was elected Fellow, and she has served as Editor in Chief of the IEEE Journal on Selected Areas in Communications. She was President of the IEEE Information Theory Society in 2012, and served on its board of governors for eleven years. She has served as technical program committee co-chair of many of the major conferences in information theory, communications and networking. She received the 2009 IEEE Communication Society and Information Theory Society Joint Paper Award, the 2009 William R. Bennett Prize in the Field of Communications Networking, the 2002 IEEE Leon K. Kirchmayer Prize Paper Award, the 2018 ACM SIGCOMM Test of Time Paper Award and several conference paper awards. She was co-winner of the MIT 2004 Harold E. Edgerton Faculty Achievement Award, received the 2013 EECS Graduate Student Association Mentor Award and served as Housemaster for seven years. In 2007 she was named a Gilbreth Lecturer by the U.S. National Academy of Engineering. She received the 2016 IEEE Vehicular Technology James Evans Avant Garde Award, the 2017 Aaron Wyner Distinguished Service Award from the IEEE Information Theory Society and the 2017 IEEE Communications Society Edwin Howard Armstrong Achievement Award.
Accelerating Graph Analytics with Novel Architectures
Speaker: David Bader, Professor & Chair- School of Computational Science & Engineering, Georgia Tech College of Computing
Time: 11:00 am - 12:00 pm Feb 7, 2019
Location: 2MTC, 10.099, Brooklyn, NY
Abstract: The need to sift through massive datasets from applications in cybersecurity, social media, financial transactions, and sensor feeds, is driving the design of novel architectures. There are few programming models and generalized processor architectures that can support the irregular memory accesses and fine grained concurrency requirements of graph analytics well while also providing accelerated run-time support. In this talk, Bader presents the hardware-software co-design of a graph analytics chip, such as in the DARPA HIVE program. In HIVE, Bader leads the Software Toolkit for Accelerating GrapH AlgoRithms on HIVE Processors (SHARP) project (joint with Georgia Tech and USC) to design a graph processing framework with Intel and Qualcomm. Unlike traditional high performance computing applications, solving analytics problems at scale often raises new challenges because of the sparsity and lack of locality in the data, the need for research on scalable algorithms and architectures, and development of frameworks for solving these real-world problems on high performance computers. SHARP will overcome two key challenges of the HIVE program: 1) platform independent, supporting high levels of algorithm acceleration for diverse set of HIVE processor designs which are expected to include technologies such as near-memory computing, systems-in-package, scratchpad and flash memories, accelerated processing, and vector processing and 2) scalability for variety of problem areas for extremely large static and dynamic graphs with millions of vertices and edges. SHARP will exploit the data structures, memory layout, and graph primitive implementations synergistically with a novel data flow representation and optimizations to deliver 1000x performance improvement.

About the Speaker: David A. Bader is Professor and Chair of the School of Computational Science and Engineering at Georgia Institute of Technology. He is a Fellow of the IEEE and AAAS and advises the White House, most recently on the National Strategic Computing Initiative (NSCI). Dr. Bader is a leading expert in solving global grand challenges in science, engineering, computing, and data science. His interests are at the intersection of high-performance computing and real-world applications, including cybersecurity, massive-scale analytics, and computational genomics, and he has co-authored over 230 articles in peer-reviewed journals and conferences. Dr. Bader served as Editor-in-Chief of the IEEE Transactions on Parallel and Distributed Systems and is on the editorial board of several leading journals. Dr. Bader has served as a lead scientist in several DARPA programs including High Productivity Computing Systems (HPCS) with IBM, Ubiquitous High Performance Computing (UHPC) with NVIDIA, Anomaly Detection at Multiple Scales (ADAMS), Power Efficiency Revolution For Embedded Computing Technologies (PERFECT), Hierarchical Identify Verify Exploit (HIVE), and Software-Defined Hardware (SDH). He has also served as Director of the Sony-Toshiba-IBM Center of Competence for the Cell Broadband Engine Processor. Bader is a cofounder of the Graph500 List for benchmarking "Big Data" computing platforms. Bader is recognized as a "RockStar" of High Performance Computing by InsideHPC and as HPCwire's People to Watch in 2012 and 2014.
Mean Field Behavior of Random Networks and Systems with Applications to Cloud Computing and Social Networks
Speaker: Ravi R. Mazumdar, University of Waterloo, Canada
Time: 1:00 pm - 2:00 pm Feb 8, 2019
Location: 2MTC, Room 10.099, Brooklyn, NY
Abstract: This talk will highlight the use of mean-field techniques that help in the analysis of complex interacting stochastic models arising in cloud computing and social networks. To highlight their use I will discuss two different classes of problems.
The first their use in the context of load balancing among a large number of servers in order to minimize
the latency or sojourn of jobs in the servers. I will begin by discussing the classical randomized Join the
Shortest Queue (JSQ) where an incoming job is routed to the server with the least number of on-going jobs
based on a finite random sample of servers. Mean field techniques are useful from two pounts of view: the
first is that they enable us to obtain insights on the stationary occupancy distributions at an arbitrary server,
and the second is that we can show that in large systems with processor sharing the stationary distribution
is insensitive to the job length distribution. In my talk I will consider more general policies in which SQ(d)
is a special case and show insensitivity carries over when systems are large while insensitivity does not hold
in small systems.
Next I will discuss an application motivated by information dissemination in social networks with inter-
actions among a large number of users. We consider a network of interacting agents where each agent exists in one of two possible states {0, 1} and the agents update their states through local interactions. We assume that agents in different states show different propensity towards updating their states( the acceptance of
influence). In particular, through such a mechanism we can model the presence of stubborn agents that are
not affected by interactions.
We will consider majority rule based opinion dynamics with many agents. We also study the situation
where there are stubborn agents who do not change their opinion. This can result in metastability in that
the network can oscillate between multiple stable equilibria.
The analysis is via mean field methods. Joint work with Thirupathiah Vasantam (Waterloo) , Arpan Mukhopadhyay (EPFL, Warwick) and Rahul
Roy (ISI, New Delhi).
About the Speaker: The speaker was educated at the Indian Institute of Technology, Bombay (B.Tech, 1977), Imperial College, London (MSc, DIC, 1978) and obtained his PhD in Control Theory under A. V. Balakrishnan at UCLA in 1983. He is currently a University Research Chair Professor in the Dept. of ECE at the University of Waterloo, Ont., Canada where he has been since September 2004. He has served on the faculties of Columbia University (NY), INRS- Telecommunications (University of Quebec), the University of Essex (UK) and Purdue University (USA). Since 2012 he is a D.J. Gandhi Distinguished Visiting Professor at the Indian Institute of Technology, Bombay, India. He is a Fellow of the IEEE and the Royal Statistical Society. His is the recipient of best paper awards at INFOCOM 2006 , the ITC-27 2015, Performance 2015, and a finalist at INFOCOM 1998. He is the author of a monograph entitled Performance Modeling, Stochastic Networks, and Statistical Multiplexing published by Morgan and Claypool, San Francisco in 2013. His research interests are in stochastic modelling and analysis applied to complex networks and systems, and in issues of network science.
AI Seminar Series: Research challenges in using computer vision in robotics systems
Speaker: Martial Hebert, Carnegie Mellon University
Time: 11:00 am - 12:00 pm Feb 8, 2019
Location: MakerSpace Event Space (6MTC/Rogers Hall), Brooklyn, NY
Abstract: The past decade has seen a remarkable increase in the level of performance of computer vision techniques, including with the introduction of effective deep learning techniques. Much of this progress is in the form of rapidly increasing performance on standard, curated datasets. However, translating these results into operational vision systems for robotics applications remains a formidable challenge. This talk with explore some of the fundamental questions at the boundary between computer vision and robotics that need to be addressed. This includes introspection/self-awareness of performance, anytime algorithms for computer vision, multi-hypothesis generation, rapid learning and adaptation. The discussion will be illustrated by examples from autonomous air and ground robots.

About the Speaker: Martial Hebert is a Professor of Robotics at Carnegie-Mellon University and Director of the Robotics Institute. His research interests include computer vision and robotics, especially recognition in images and video data, model building and object recognition from 3D data, and perception for mobile robots and for intelligent vehicles. His group has developed approaches for object recognition and scene analysis in images, 3D point clouds, and video sequences. In the area of machine perception for robotics, his group has developed techniques for people detection, tracking, and prediction, and for understanding the environment of ground vehicles from sensor data. He currently serves as Editor-in-Chief of the International Journal of Computer Vision.
Robust Computing Systems: From Today to the N3XT 1,000X
Speaker: Subhasish Mitra, Stanford University
Time: 11:00 am - 12:00 pm Feb 14, 2019
Location: 2MTC Room 10.009, Brooklyn, NY
Abstract: Future computing systems require research breakthroughs in the following areas:
• Robustness: Existing validation and test methods barely cope with today’s complexity. In advanced silicon technologies, reliability failures, largely benign in the past, are becoming visible at the system level. Security is a major concern at both hardware and
software levels.
• Performance: Energy benefits of silicon technologies have plateaued (power wall). Coming generations of abundant-data applications (e.g., deep learning, graph analytics) are dominated by off-chip memory accesses (memory wall).
• New applications: Neuro- and bio-sciences create tremendous opportunities for new computing systems, from implants to understanding brain functions.
This talk presents an overview of my group’s research in the above areas, and particularly emphasizes complexity and performance:
• QED (Quick Error Detection) and Symbolic QED dramatically improve pre-silicon verification and post-silicon validation of digital systems. Difficult bugs can now be detected and localized automatically, in a few minutes to a few hours. In contrast, existing approaches might take weeks (or months) of intense manual work with limited success. Results on commercial hardware designs demonstrate the effectiveness of the presented techniques.
• N3XT (Nano-Engineered Computing Systems Technology) leverages unique properties of emerging nanotechnologies to create new architectures that overcome the memory wall and the power wall. N3XT promises 1,000x energy efficiency improvements for abundant-data applications. Such massive benefits enable new frontiers of applications for a wide range of computing systems, from embedded systems to the cloud. N3XT hardware prototypes represent leading examples of transforming scientifically-interesting nanomaterials and nanodevices into actual
nanosystems.
About the Speaker: Subhasish Mitra is Professor of Electrical Engineering and of Computer Science at Stanford University, where he directs the Stanford Robust Systems Group and co-leads the Computation focus area of the Stanford SystemX Alliance. He is also a faculty member of the Stanford Neurosciences Institute. Prof. Mitra holds the Carnot Chair of Excellence in Nanosystems at CEA-LETI in Grenoble, France. Before joining the Stanford faculty, he was a Principal Engineer at Intel Corporation.
Prof. Mitra's research interests range broadly across robust computing, nanosystems, VLSI design, validation, test and electronic design automation, and neurosciences. He, jointly with his students and collaborators, demonstrated the first carbon nanotube computer and the first three-dimensional nanosystem with computation immersed in data storage. These demonstrations received wide-spread recognitions (cover of NATURE, Research Highlight to the United States Congress by the National Science Foundation, highlight as "important, scientific breakthrough" by the BBC, Economist, EE Times, IEEE Spectrum, MIT Technology Review, National Public Radio, New York Times, Scientific American, Time, Wall Street Journal, Washington Post and numerous others worldwide). His earlier work on X-Compact test compression has been key to cost-effective manufacturing and high-quality testing of almost all electronic systems. X-Compact and its derivatives have been implemented in widely-used commercial Electronic Design Automation tools.
Prof. Mitra's honors include the ACM SIGDA/IEEE CEDA A. Richard Newton Technical Impact Award in Electronic Design Automation (a test of time honor), the Semiconductor Research Corporation's Technical Excellence Award, the Intel Achievement Award (Intel’s highest corporate honor), and the Presidential Early Career Award for Scientists and Engineers from the White House (the highest United States honor for early-career outstanding scientists and engineers). He and his students published several award-winning papers at major venues: ACM/IEEE Design Automation Conference, IEEE International Solid-State Circuits Conference, ACM/IEEE International Conference on Computer-Aided Design, IEEE International Test Conference, IEEE Transactions on CAD, IEEE VLSI Test Symposium, and the Symposium on VLSI Technology. At Stanford, he has been honored several times by graduating seniors "for being important to them during their time at Stanford."
Prof. Mitra served on the Defense Advanced Research Projects Agency's (DARPA) Information Science and Technology Board as an invited member. He is a Fellow of the Association for Computing Machinery (ACM) and the Institute of Electrical and Electronics Engineers (IEEE).
Secure Computer Hardware in the Age of Pervasive Security Attacks
Speaker: Mengjia Yan, University of Illinois at Urbana-Champaign
Time: 11:00 am - 12:00 pm Feb 19, 2019
Location: 370 Jay, Room 1201, Brooklyn, NY
Abstract: Recent attacks such as Spectre and Meltdown have shown how vulnerable modern computer hardware is. The root cause of the problem is that computer architects have traditionally focused on performance and energy efficiency. Security has never been a first-class requirement. Moving forward, however, this has to radically change: we need to rethink computer architecture from the ground-up for security.
As an example of this vision, in this talk, I will focus on speculative execution in out-of-order processors --- a core computer architecture technology that is the target of the recent attacks. I will describe InvisiSpec, the first robust hardware defense mechanism against speculative (a.k.a transient) execution attacks. The idea is to make loads invisible in the cache hierarchy, and only reveal their presence at the point when they are safe. Once an instruction is deemed safe, our hardware is able to cheaply modify the cache coherence state in a consistent manner. Further, to reduce the cost of InvisiSpec and increase its protection coverage, I propose Speculative Taint Tracking (STT). This is a novel form of information flow tracking that is specifically designed for speculative execution. It reduces cost by allowing tainted instructions to become safe early, and by effectively leveraging the predictor hardware that is ubiquitous in modern processors. Further improvements of InvisiSpec-STT can be attained with new compiler techniques. Finally, I will conclude my talk by describing ongoing and future directions towards designing secure processors.

About the Speaker: Mengjia Yan is a Ph.D. student at the University of Illinois at Urbana-Champaign (UIUC), working with Professor Josep Torrellas. Her research interest lies in the areas of computer architecture and hardware security, with a focus on defenses against transient execution attacks and cache-based side channel attacks. Her work has appeared in some of the top venues in computer architecture and security, and has sparked a large research collaboration initiative between UIUC and Intel. Mengjia received the UIUC College of Engineering Mavis Future Faculty Fellow, the Computer Science W.J. Poppelbaum Memorial Award, a MICRO TopPicks in Computer Architecture Honorable Mention, and was invited to participate in two Rising Stars workshops.
RSimplex: Towards A Robust CPS Framework
Speaker: Xiaofeng Wang, University of South Carolina (UofSC)
Time: 11:00 am - 12:00 pm Feb 21, 2019
Location: 2MTC, 10.099, Brooklyn, NY
Abstract: Cyber-physical systems (CPS) are ubiquitous in a number of application areas, including aircraft and air-traffic control, highway transportation, manufacturing, medicine and healthcare, to name a few. Such systems consist of two main components: physical elements modeling the systems to be controlled and cyber elements representing the communication links and software. An essential concept in CPS is co-stability. It indicates the ability to maintain system stability in spite of concurrent complex control software failures and physical failures, which is particularly important for safety-critical applications. Unfortunately, the existing literature cannot meet the needs of co-stability. The state-of-the-art approaches only focus on one aspect of the problem, which either address physical failures with the assumption that the software is fault-free, or vice versa. Systematic methodologies for co-stability are still missing.
To close such a gap, we present the RSimplex architecture, which can simultaneously deal with physical failures and software failures. The framework adopts an extended Simplex architecture and integrates a robust fault-tolerant controller (RFTC). On one hand, the RFTC is able to detect, isolate, and compensate for physical failures, and also provide envelope determination and protection control schemes to ensure that the system stays within the desired stability envelope. On the other hand, the extended Simplex makes the system robust to software failures by switching to a safe-mode controller (the robust high assurance controller, RHAC). With the RSimplex architecture, the verification and validation (V&V) complexity can be largely reduced for two reasons. First, the number of components that need to be completely certified are reduced. Second, because of the power of RHAC in compensating uncertainties, it can cover a large range of physical failures without reconfiguring the system parameters. It leads to a significant reduction of the number of states in software. Both of these characters lower down the system complexity from a certification perspective and therefore enable us to simplify the V&V process.

About the Speaker: Xiaofeng Wang is assistant professor in the Department of Electrical Engineering at the University of South Carolina (UofSC), Columbia. He earned his B.S. degree in Applied Mathematics and M.S. in Operation Research and Control Theory from East China Normal University, China, in 2000 and 2003, respectively, and obtained his PhD degree in Electrical Engineering at the University of Notre Dame in 2009. After that, he worked as postdoctoral research associate at the University of Illinois at Urbana and Champaign before he joined UofSC. His research interests include robotics, cyber-physical systems, autonomous systems, multi-agent systems, networked and real-time control systems, robust fault-tolerant control, and optimization. He is associate editor of Journal of The Franklin Institute. He was the recipient of the best paper award in the Annual Conference of the Prognostics and Health Management Society in 2014.
Deep learning accelerators: a proving ground for specialized computing
Speaker: Brandon Reagen, Facebook
Time: 11:00 am - 12:00 pm Feb 22, 2019
Location: 5MTC, LC400, Brooklyn, NY
Abstract: The computing industry has a power problem: the days of ideal power-process scaling are over, and chips now have more devices than can be fully powered simultaneously, limiting performance. To continue scaling performance in light of these power-constraints requires creative solutions. Specialized hardware accelerators are one viable solution. While accelerators promise to provide orders of magnitude more performance per watt, several challenges have limited their wide-scale adoption and fueled skepticism.
Deep learning has emerged as a sort of proving ground for hardware acceleration. With extremely regular compute patterns and wide-spread use, if accelerators can’t work here, there’s little hope elsewhere. To motivate accelerators as the way to continue scaling compute performance, accelerators must enable computation that cannot be done today and demonstrate mechanisms for performance scaling, such that they are not a one-off solution. In this talk I will propose deep learning algorithm-hardware co-design to answer these questions and identify the efficiency gap between standard ASIC design practices and full-stack co-design to enable these powerful models to be used with little restriction. To push the efficiency limits of deep learning inference this talk will introduce principled unsafe optimizations. A principled unsafe optimization changes how a program executes without impacting accuracy. By breaking the contract between the algorithm, architecture, and circuits, efficiency can be greatly improved. To conclude, future research directions centering around hardware specialization will be presented: accelerator-centric architectures and privacy-preserving cloud computing.

About the Speaker: Brandon Reagen is a computer architect with a focus on specialized hardware (i.e., accelerators) and low-power design with applications in deep learning. He received his PhD from Harvard in May of 2018. Over the course of his PhD, Brandon made several research contributions to lower the barrier of using accelerators as general architectural constructs including benchmarking, simulation infrastructure, and SoC design. Using his knowledge of accelerator design, he led the way in highly-efficient and accurate deep learning accelerator design with his work on principled unsafe optimizations. In his thesis, he found that for DNN inference intricate, full-stack co-design between the robust nature of the algorithm and the circuits they execute on can result in nearly an order of magnitude more power-efficiency compared to standard ASIC design practices. His work has been published in conferences ranging from architecture, ML, CAD, and circuits. Brandon is now a Research Scientist at Facebook in the AI Infrastructure team.
Photonics and optoelectronics with carbon nanotube crystalline films
Speaker: Weilu Gao, Rice University
Time: 12:00 pm - 1:15 pm Feb 25, 2019
Location: 2 MTC, Room 10.099, Brooklyn, NY
Abstract: One of the grand challenges in nanoengineering and nanoscience today is how to create macroscopic materials and devices by assembling nano-objects while preserving their rich variety of unprecedented properties that are promising for new applications. Carbon nanotubes make an ideal one-dimensional material platform for the exploration of exotic physical phenomena under extremely strong quantum confinement. Although extraordinary electronic, thermal, and optical properties in individual carbon nanotubes have been continuing to attract interest in various disciplines, including chemistry, materials science, physics, and engineering, the macroscopic manifestation of such properties is still limited, despite significant efforts for decades. In this talk, I will first introduce a new method, controlled vacuum filtration, to address the long-standing problem of preparing wafer-scale films of crystalline chirality-enriched carbon nanotubes. Such films immediately enable exciting new fundamental studies and applications. I will then summarize recent discoveries in optical spectroscopy studies and optoelectronic device applications using films prepared by this technique.

About the Speaker: Dr. Weilu Gao received his B.S. degree in electrical engineering from Shanghai Jiao Tong University in 2011 and his Ph.D. degree in electrical and computer engineering from Rice University in 2016. He is currently a postdoctoral researcher in the group of Prof. Junichiro Kono in the Department of Electrical and Computer Engineering at Rice University. Dr. Gao was a recipient of the National Scholarship for Outstanding Self-Financed Students Abroad, Chinese Government in 2016. His research interests are in photonics and optoelectronics of nanomaterials, including single-wall carbon nanotubes and two-dimensional materials, spanning fundamental research to applications in health, energy, imaging, sensing, computing and communication. He has more than 30 publications, and they have been cited over 1800 times in total.
Confluence of Electromagnetics, Circuits and Systems Enables The Third Wireless Revolution
Speaker: Harish Krishnaswamy, Columbia University
Time: 11:00 am - 12:00 pm Feb 27, 2019
Location: 370 Jay street, Room 1201, Brooklyn, NY
Abstract: Integrated circuits have fueled several revolutions that have deeply impacted modern society, including the computing revolution, the internet and the first two wireless revolutions. We are at the dawn of the third wireless revolution, which I call the Wireless Mobile Reality revolution. Over the next fifteen years, new wireless paradigms spanning from radio frequencies to millimeter-waves and terahertz will change the way in which we interact with the real world, through applications such as mobile virtual and augmented reality, vision quality imaging, gesture recognition and bio- and materials-sensing.
However, at the same time, integrated circuits are starting to run out of steam - technology scaling is no longer yielding better transistors that are faster and lower power. Therefore, circuit design needs to be refreshed with new tools and techniques that draw inspiration from the layers below (electromagnetics and device physics) and the layers above (communication systems and networking).
In this talk, I will describe research along these lines from the CoSMIC lab at Columbia University. I will start by describing a new approach to breaking Lorentz reciprocity to engineer high-performance non-reciprocal components, such as gyrators, isolators and circulators. I will then talk about how these integrated non-reciprocal circulators enable practical integrated full-duplex wireless radios. Finally, I will talk about the FlexICoN project at Columbia which is taking a holistic and cross-layer view of full-duplex networks from the physical layer to the networking layer. I will also briefly touch upon other work from CoSMIC lab in the same vein related to high-power, high-efficiency millimeter-wave radios, MIMO radios, opto-electronic LIDARs and city-scale wireless testbeds.

About the Speaker: Harish Krishnaswamy (S’03–M’09) received the B.Tech. degree in electrical engineering from IIT Madras, Chennai, India, in 2001, and the M.S. and Ph.D. degrees in electrical engineering from the University of Southern California (USC), Los Angeles, CA, USA, in 2003 and 2009, respectively. In 2009, he joined the Electrical Engineering Department, Columbia University, New York, NY, USA, where he is currently an Associate Professor and the Director of the Columbia High-Speed and Millimeter-Wave IC Laboratory (CoSMIC).
In 2017, he co-founded MixComm Inc., a venture-backed startup, to commercialize CoSMIC Laboratory’s advanced wireless research. His current research interests include integrated devices, circuits, and systems for a variety of RF, mmWave, and sub-mmWave applications. Dr. Krishnaswamy was a recipient of the IEEE International Solid-State Circuits Conference Lewis Winner Award for Outstanding Paper in 2007, the Best Thesis in Experimental Research Award from the USC Viterbi School of Engineering in 2009, the Defense Advanced Research Projects Agency Young Faculty Award in 2011, the 2014 IBM Faculty Award, the Best Demo Award at the 2017 IEEE ISSCC, and Best Student Paper Awards (First Place) at the 2015 and 2018 IEEE Radio Frequency Integrated Circuits Symposium . He has been a member of the technical program committee of several conferences, including the IEEE International Solid-State Circuits Conference since 2015 and the IEEE Radio Frequency Integrated Circuits Symposium since 2013. He currently serves as a Distinguished Lecturer for the IEEE Solid-State Circuits Society and as a member of the DARPA Microelectronics Exploratory Council.
AI Seminar Series: Machine Learning for Personalization
Speaker: Tony Jebara, Netflix
Time: 11:00 am - 12:00 pm Feb 28, 2019
Location: 370 Jay, Seminar Room 1201, Brooklyn, NY
Abstract: For many years, the main goal of the Netflix recommendation system has been to get the right titles in front of each member at the right time. For instance, the 2006 Netflix Challenge helped spur new research in low-rank matrix decomposition and collaborative filtering. Today, we use nonlinear, probabilistic, and deep learning approaches to make even better rankings of our movies and TV shows for each user. But the job of recommendation does not end there. The homepage should be able to convey to the member enough evidence of why this is a good title for her, especially for shows that the member has never heard of. One way to address this challenge is to personalize the way we portray the titles on our service. Our image personalization engine is driven by online learning and contextual bandits to reliably handle over 20 million personalized image requests per second. Finally, while machine learning is great at learning to make accurate predictions, predictions must be made in order to take actions in the real world. Currently, we are working on integrating causality and fairness into many of Netflix's machine learning and personalization systems.
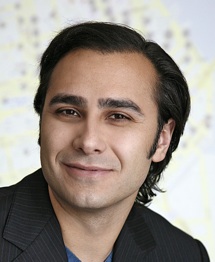
About the Speaker: Tony Jebara is director of machine learning at Netflix and professor on leave from Columbia University. He has published over 100 peer-reviewed papers in leading conferences and journals across machine learning, computer vision, social networks and recommendation. His work has been recognized with best paper awards from the International Conference on Machine Learning and from the Pattern Recognition Society. He is the author of the book Machine Learning: Discriminative and Generative. Jebara is the recipient of the Career award from the National Science Foundation as well as faculty awards from Google, Yahoo and IBM. He has co-founded and advised multiple startup companies in the domain of artificial intelligence. Jebara has served as general chair and program chair for the International Conference on Machine Learning. In 2006, he co-founded the NYAS Machine Learning Symposium and has served on its steering committee since then. He obtained a PhD from MIT in 2002.
TBA
Speaker: Bruno Siciliano, University of Naples, Italy
Time: 11:00 am - 12:00 pm Feb 28, 2019
Location: 2MTC, Room 10.099, Brooklyn, NY
Abstract:TBA
Determination of constitutive properties for Electrical Engineering materials
Speaker: Romain Corcolle, NYU Shanghai
Time: 11:00 am - 12:15 pm Mar 1, 2019
Location: 2MTC, 10.099, Brooklyn, NY

Abstract: Over the past decades, numerical simulation tools (such as Finite Element Method for example) received much attention from researchers in Engineering fields. Nowadays, the numerical modelling of systems with very complex geometries is achievable for reasonable costs (both in time and computation power). However, the models or equations
that describe the material behavior (constitutive laws) in numerical simulation tools did not improve at the same pace; relatively simple constitutive laws are often used for different reasons.
The poorness of constitutive laws is a major scientific problem for achieving a fine modelling of electromagnetic devices. Devices with better performance could be designed if more accurate constitutive laws were available.
In this talk, I will present some modelling techniques for the determination of constitutive properties of Electrical Engineering materials. I will first present some homogenization models for Soft Magnetic Composites, which are composite materials designed to exhibit lower levels of Eddy Current losses than classical laminated iron cores. In the second part of my talk, I will present a constitutive model that is able to predict the effect of mechanical stress on the piezoelectric coefficients of ferroelectric materials.
Cramming More Radios: Wavelength-Scale mm-Wave Integrated Transceivers
Speaker: Arun Natarajan, Oregon State University
Time: 11:00 am - 12:00 pm Mar 7, 2019
Location: 370 Jay Room 202 Auditorium, Brooklyn, NY
Abstract: Wireless systems continue to drive and be driven by emerging data and sensing applications across billions of connected devices. Communication and sensing systems are evolving to higher center frequencies at mm-wave and beyond, leveraging larger available spectrum, ultra-dense MIMO transceivers, heterogenous cell sizes(macro, pico, femto) and increased spatial spectrum reuse. Realizing such systems will require innovations across architectures and circuits for large-scale arrays to achieve multi-Gb/s data rates, high-resolution imaging and low latencies that are critical to real-time applications. These approaches must also enabling integration in silicon-based process technologies to enable mass consumer applications. Interestingly, higher operating frequencies and large-element arrays imply that ICs occupy wavelength-scale dimensions. In this talk, I will present research in the High-Speed Integrated Circuits Lab at Oregon State University on scalable transceivers, demonstrating efficient, reconfigurable architectures across circuits and antennas to realize scalable MIMO arrays. I will discuss spatial filtering techniques in the context of interferer-tolerance in MIMO arrays as well as array synchronization for scalability. Finally, I will present related research in the HSIC lab on low-power transceivers at RF and mm-wave for short-range links and sensing applications.

About the Speaker: Bio: Dr. Natarajan's research is focused on RF and mm-wave integrated circuits and systems for wireless communication and imaging. He received the B.Tech. degree in electrical engineering from the Indian Institute of Technology, Madras, in 2001 and the M.S. and Ph.D. degrees in electrical engineering from the California Institute of Technology (Caltech), Pasadena, in 2003 and 2007, respectively. From 2007 to 2012, he was a Research Staff Member at IBM T. J. Watson Research Center, NY and worked on mm-wave phased arrays for multi-Gb/s data links and airborne radar and on self-healing circuits for increased yield in sub-micron process technologies. Since joining Oregon State University, his research group has focused on low-power RFICs and RF/mm-wave arrays integrated in CMOS/SiGe BiCMOS. Dr. Natarajan received the DARPA Young Faculty Award in 2017, the National Talent Search Scholarship from the Government of India [1995-2000], the Caltech Atwood Fellowship in 2001, the Analog Devices Outstanding Student IC Designer Award in 2004, and the IBM Research Fellowship in 2005, and serves as an Associate Editor for the IEEE Trans. on Microwave Theory and Techniques and on the Technical Program Committee of the IEEE ISSCC and IEEE RFIC conferences.
Learn to Communicate - Communicate to Learn
Speaker: Deniz Gunduz, Imperial College, UK
Time: 2:00 pm - 3:00 pm Mar 7, 2019
Location: 2MTC, 10.099, Brooklyn, NY
Abstract: Machine learning and communications are intrinsically connected. The fundamental problem of communications, as stated by Shannon, "reproducing at one point either exactly or approximately a message selected at another point,” can be considered as a classification problem. With this connection in mind, I will focus on the fundamental joint source-channel coding problem using modern machine learning techniques. I will introduce uncoded "analog” schemes for wireless image transmission, and show their surprising performance both through simulations and practical implementation. This result will be used to motivate unsupervised learning techniques for wireless image transmission, leading to a "deep joint source-channel encoder” architecture, which behaves similarly to analog transmission, and not only improves upon state-of-the-art digital schemes, but also achieves graceful degradation with channel quality, and performs exceptionally well over fading channels despite not utilizing explicit pilot signals or channel state estimation.
In the second part of the talk, I will focus on distributed machine learning, particularly targeting wireless edge networks, and show that ideas from coding and communication theories can help improve their performance. Finally, I will introduce the novel concept of "over-the-air stochastic gradient descent" for wireless edge learning, and show that it significantly improves the efficiency of machine learning across bandwidth and power limited wireless devices compared to the standard digital approach that separates computation and communication. This will close the circle, making another strong case for analog communication in future communication systems.
About the Speaker: Deniz Gunduz received his M.S. and Ph.D. degrees in electrical engineering from NYU Polytechnic School of Engineering (formerly Polytechnic University) in 2004 and 2007, respectively. After his PhD, he served as a postdoctoral research associate at Princeton University, and as a consulting assistant professor at Stanford University. He was a research associate at CTTC in Barcelona, Spain until September 2012, when he joined the Electrical and Electronic Engineering Department of Imperial College London, UK, where he is currently a Reader (Associate Professor) in information theory and communications, and leads the Information Processing and Communications Lab.
His research interests lie in the areas of information theory, machine learning and privacy. Dr. Gunduz is an Editor of the IEEE Transactions on Green Communications and Networking, a Guest Editor for the IEEE Journal on Selected Areas in Communications Special Issue on “Machine Learning for Wireless Communications”, and served as an Editor of the IEEE Transactions on Communications (2013-2018). He is the recipient of the IEEE Communications Society - Communication Theory Technical Committee (CTTC) Early Achievement Award in 2017, a Starting Grant of the European Research Council (ERC) in 2016, IEEE Communications Society Best Young Researcher Award for the Europe, Middle East, and Africa Region in 2014, Best Paper Award at the 2016 IEEE WCNC, and the Best Student Paper Awards at the 2018 IEEE WCNC and the 2007 IEEE ISIT. He is a co-chair of the 2019 London Symposium on Information Theory, and previously served as the co-chair of the 2016 IEEE Information Theory Workshop, and the 2012 IEEE European School of Information Theory.
Interactive Learning and Decision Making with Machines and People
Speaker: Yuxin Chen, Caltech
Time: 11:00 am - 12:00 pm Mar 15, 2019
Location: 1 MetroTech Center, 19th floor, Room 1930 Jacobs Seminar Room, Brooklyn, NY
Abstract: How can we intelligently acquire information for decision making, when facing a large volume of data? In this talk, I will focus on learning and decision making problems that arise in robotics, scientific discovery and human-centered systems, and present how we can develop principled approaches that actively extract information, identify the most relevant data for the learning tasks and make effective decisions under uncertainty. As an example, I will introduce the optimal value of information problem for decision making, and show that for a large class of adaptive information acquisition problems that are known to be NP-hard, one could devise efficient surrogate objectives that are amenable to greedy optimization, while still achieving strong approximation guarantees. I will further talk about a few practical challenges in real-world decision-making systems such as complex constraints, complex action space, and rich interfaces. More concretely, I will elaborate on how to address these practical concerns through a variety of applications, ranging from sequential experimental design for scientific discovery to interactive machine teaching for human learners.

About the Speaker: Yuxin Chen is a postdoctoral scholar in the Department of Computing and Mathematical Sciences at the California Institute of Technology (Caltech). Prior to Caltech, he received his Ph.D. in computer science from ETH Zurich in 2017. His research interest lies broadly in probabilistic reasoning and machine learning. He was a recipient of the Google European Doctoral Fellowship in Interactive Machine Learning, the Swiss SNSF Early Postdoc.Mobility Fellowship, and the PIMCO Postdoctoral Fellowship in Data Science. He currently focuses on developing interactive machine learning systems that involve active learning, sequential decision making, interpretable models and machine teaching.
Battling Bandits: Online Learning from Subset-wise Relative Preferences
Speaker: Aditya Gopalan, Indian Institute of Science, India
Time: 2:00 pm - 3:00 pm Mar 19, 2019
Location: 2MTC, Room10.099, Brooklyn, NY
Abstract: We consider the problem of adaptively learning good alternatives from among a pool of alternatives, but with only relative utility feedback from chosen subsets. At each round, the learner adaptively chooses a subset of alternatives and receives (noisy) observations of which ones are preferred over the others in the subset. This type of feedback is natural in several domains,
especially where human preferences are elicited in a repeated fashion, e.g., the design of surveys and expert reviews, web search and recommender systems, ranking in multiplayer games,
etc. Classical online learning approaches such as the multi-armed bandit typically model absolute utility feedback, and are thus inadequate to express relative choices. The dueling bandit problem (Yue-Joachims'09) is a recent attempt to model online learning with pairwise preferences, but the more general, realistic, and combinatorially harder case of working with preferences expressed over subsets has largely been unexplored. We take a step in this direction and formulate what we call the Battling bandit problem, where one seeks to learn an optimal item or ranking of n items by sequentially choosing up to size-k subsets at each round and exploiting relative preferences
arising from a choice model such as the well-known Plackett-Luce
probability model. We study variants of learning objectives from
subsetwise feedback: Identifying the best item, the set of top-k
items, full ranking etc., in both the probably approximately
correct (PAC) or regret optimization setting, and design
algorithms with optimality properties.
(Joint work with Aadirupa Saha (Indian Institute of Science).)
About the Speaker: Aditya Gopalan is an Assistant Professor and INSPIRE Faculty Fellow at the Indian Institute of Science, Dept. of Electrical Communication Engineering. He received the Ph.D. degree in electrical engineering from The University of Texas at Austin, and the B.Tech. and M.Tech. degrees in electrical engineering from the Indian Institute of Technology Madras. He was an Andrew and Erna Viterbi Post-Doctoral Fellow at the Technion-Israel Institute of Technology. His research interests include machine learning and statistical inference, control, and resource allocation algorithms.
Closing the perception-action loop with deep robotic learning
Speaker: Yuke Zhu, Stanford University
Time: 11:00 am - 12:00 pm Mar 25, 2019
Location: 6MTC/RH325, Brooklyn, NY
Abstract: Robots and autonomous systems have been playing a significant role in the modern economy. Custom-built robots have remarkably improved productivity, operational safety, and product quality. However, these robots are usually programmed for specific tasks in well-controlled environments, unable to perform diverse tasks in the real world. In this talk, I will demonstrate how machine learning techniques, such as deep neural networks, offer important computational tools towards building more effective and generalizable robot intelligence. I will discuss my research on learning-based methods that established a tighter coupling between perception and action at three levels of abstraction: 1) learning primitive motor skills from raw sensory data, 2) sharing knowledge between sequential tasks in visual environments, and 3) learning hierarchical task structures from video demonstrations.

About the Speaker: Yuke Zhu is a final year Ph.D. candidate in the Department of Computer Science at Stanford University, advised by Prof. Fei-Fei Li and Prof. Silvio Savarese. His research interests lie at the intersection of machine learning, computer vision, and robotics. His work builds machine learning algorithms for general-purpose robots. He received a Master's degree from Stanford University and dual Bachelor's degrees from Zhejiang University and Simon Fraser University. He also collaborated with research labs including Snap Research, Allen Institute for Artificial Intelligence, and DeepMind.
Device simulation study on transition metal dichalcogenide transistors
Speaker: Akiko Ueda, AIST, Japan
Time: 2:00 pm - 3:00 pm Mar 26, 2019
Location: 2MTC, 9.101 Executive Conference Room., Brooklyn, NY
Abstract: Transition metal dichalcogenide (TMD) is fascinating material which shows intriguing phenomena such as circular dichroism stemmed from the valley and spin degree of
freedom and attracts attention as a candidate for next generation devices in electronics and
spintronics. To bring out rich functionalities and to design the ideal structure of TMD, the
device simulation takes an important role. In this presentation, we talk about two recent
works of simulations we performed on the TMD.
We start with the drift-diffusion (DD) study on the ion-gated TMD transistors. Ion gating is
known as a powerful tool to access electronic functionalities with low voltage operation.
Though many interesting experimental studies have been reported, the device simulation
had never been performed before. In this work, we developed a 2D layer transistor model
including an ionic liquid (IL) as a gate dielectric based on the DD method. We reproduced
the ambipolar behavior reported in several experiments and explained the transport
mechanism using the band profile and spatial distribution obtained by the calculation.
Next, we talk about the Monte-Carlo study of the self-heating effect on electron transport in
monolayer TMD. The heat dissipation is one of the severe problems to cause degradation
in electronics. We studied the impact of heat induced by electrons on I-V characteristics
using the Monte Carlo method. We show that under the strong electric field, the self-
heating enhances the inter-valley phonon scattering and results in a negative conductance.
About the Speaker: Dr. Akiko Ueda received her Ph.D. from Keio University (2007) on the topic of nonequilibrium transport in quantum dots and Aharonov-Bohm interferometers. From 2007- 09, she was an assistant professor in the Faculty of Business and Commerce at Keio University (limited-term) studying physics education for liberal art students. From 2009-11, she was a postdoctoral fellow at Ben Gurion University in Israel, researching transport and vibrational effects in molecular junctions. In 2011, she returned to Japan as a tenure-track assistant professor at University of Tsukuba, receiving tenure in 2016, and studying transport characteristics of Si nanowire transistors, and topological superconductors. In 2018, she moved to the National Institute of Advanced Industrial Science and Technology (AIST) in Japan as a senior researcher and works on device simulation on next-generation devices.
Unsupervised Neural Network Learning via an Algorithmic Lens
Speaker: Chinmay Hegde, Iowa State University
Time: 11:00 am - 12:00 pm Mar 28, 2019
Location: 370 Jay, Room 1201 Seminar Room, Brooklyn, NY
Abstract: The tremendous success of deep learning in applications compels us to revisit its theoretical foundations. While an overarching rigorous theory for deep learning algorithms remains elusive, recent breakthroughs may pave the way towards such a theory. However, these results primarily have focused on the supervised setting, which typically relies on the availability of abundant, pristine, labeled training data.
I will first describe several new theoretical results for neural learning algorithms for the unsupervised setting. Our approach rests on two key ideas: (1) the learned representations often themselves obey conciseness assumptions (such as compositionality, sparseness, and/or democracy), and (2) datasets often obey certain natural generative modeling assumptions. Our results can be viewed as formal evidence that (shallow) networks are indeed unsupervised feature learning mechanisms, and may shed insights on how to train larger stacked architectures.
I will then describe an approach for unsupervised learning that succeeds in the setting of limited, coarse, unlabeled data. Our approach rests on a new generative modeling architecture, together an associated training algorithm, that is explicitly physics-aware. We demonstrate this approach in an application in computational material science, and show its benefits over the state of the art.
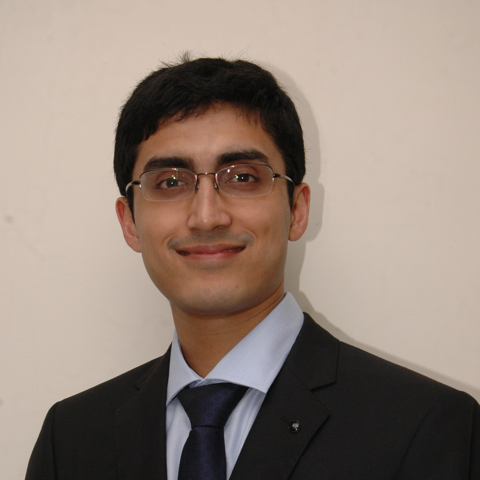
About the Speaker: Chinmay Hegde is with the Electrical and Computer Engineering at Iowa State University in Ames, IA, where he has been an assistant professor since Fall 2015. His research focuses on developing fast and robust algorithms for machine learning and statistical signal processing, with applications to imaging, transportation analytics, and materials informatics. Before coming to Ames, Chinmay received his PhD at Rice University, and was a postdoctoral associate in CSAIL at MIT. He is the recipient of multiple awards, including best paper awards at ICML, SPARS, and MMLS; the Budd Award for Best Engineering PhD Thesis in 2013; the NSF CRII Award in 2016; the Warren Boast Undergraduate Teaching Award in 2016, the Boast-Nilsson Award for Educational Impact in 2018; and the NSF CAREER Award in 2018.
Robotics @ PRISMA Lab
Speaker: Bruno Siciliano, University of Naples Federico II
Time: 11:00 am - 12:00 pm Apr 1, 2019
Location: 370 Jay 1201 Seminar Room, Brooklyn, NY
Abstract: The PRISMA Lab <www.prisma.unina.it> is committed to research in robotics and automation since 30 years at University of Naples Federico II. The team has a track record of successful research projects, mainly at a European level, for a total funding of 12 million € in the last 10 years. This talk is organized in five parts. In the first part, aerial and dynamic manipulation are surveyed along with the main results achieved on modelling, planning, perception and control. The second part of the talk focuses on how to merge learning and model-based strategies to provide autonomy to robotic manipulation. In the third part of the talk, anthropomorphic tools for robotics and prosthetics are presented, requiring advanced sensorimotor skills to reproduce human’s manipulation abilities. The fourth part of the talk deals with human-friendly robots and recent advances on compliant control during interaction with soft tissues with emphasis for a surgical scenario. The final part of the talk is devoted to discuss future perspectives and big challenges of robotics.

About the Speaker: Professor Bruno Siciliano is Director of the Interdepartmental Center for Advances in RObotic Surgery (ICAROS), as well as Coordinator of the Laboratory of Robotics Projects for Industry, Services and Mechatronics (PRISMA Lab), at University of Naples Federico II. His research interests in robotics include manipulation and control, human–robot cooperation, and service robotics. Fellow of the scientific societies IEEE, ASME, IFAC, he received numerous international prizes and awards, and he was President of the IEEE Robotics and Automation Society from 2008 to 2009. Since 2012 he is on the Board of Directors of the European Robotics Association. He has delivered more than 150 keynotes and has published more than 300 papers and 7 books. His book “Robotics” is among the most adopted academic texts worldwide, while his edited volume “Springer Handbook of Robotics” received the highest recognition for scientific publishing: 2008 PROSE Award for Excellence in Physical Sciences & Mathematics. More details are available at http://wpage.unina.it/sicilian/
Understanding and Improving Deep Neural Networks
Speaker: Jeff Clune, University of Wyoming/Uber AI Labs
Time: 3:00 pm - 4:00 pm Apr 3, 2019
Location: 370 Jay, Room 120, Brooklyn, NY
Abstract: Through deep learning, deep neural networks have produced state-of-the-art results in a number of different areas of machine learning, including computer vision, natural language processing, robotics and reinforcement learning. I will summarize three projects on better understanding deep neural networks and improving their performance. First I will describe our sustained effort to study how much deep neural networks know about the images they classify. Our team initially showed that deep neural networks are “easily fooled,” meaning they will declare with near certainty that completely unrecognizable images are everyday objects. These results suggested that deep neural networks do not truly understand the objects they classify. However, our subsequent results reveal that, when augmented with powerful priors, deep neural networks actually have a surprisingly deep understanding of objects, which also enables them to be incredibly effective generative models that can produce a wide diversity of photo-realistic images. Second, I will summarize our Nature paper on learning algorithms that enable robots, after being damaged, to adapt in 1-2 minutes in order to continue performing their mission. This work combines a novel stochastic optimization algorithm with Bayesian optimization to produce state-of-the-art robot damage recovery. Third, I will describe our recent Go-Explore algorithm, which dramatically improves the ability of deep reinforcement learning algorithms to solve previously unsolvable problems wherein reward signals are sparse, meaning that intelligent exploration is required. Go-Explore solves Montezuma’s Revenge, considered by many to be a grand challenge of AI research. I will also very briefly summarize a few other machine learning projects from my career, including our PNAS paper on automatically identifying, counting, and describing wild animals in images taken remotely by motion-sensor cameras.
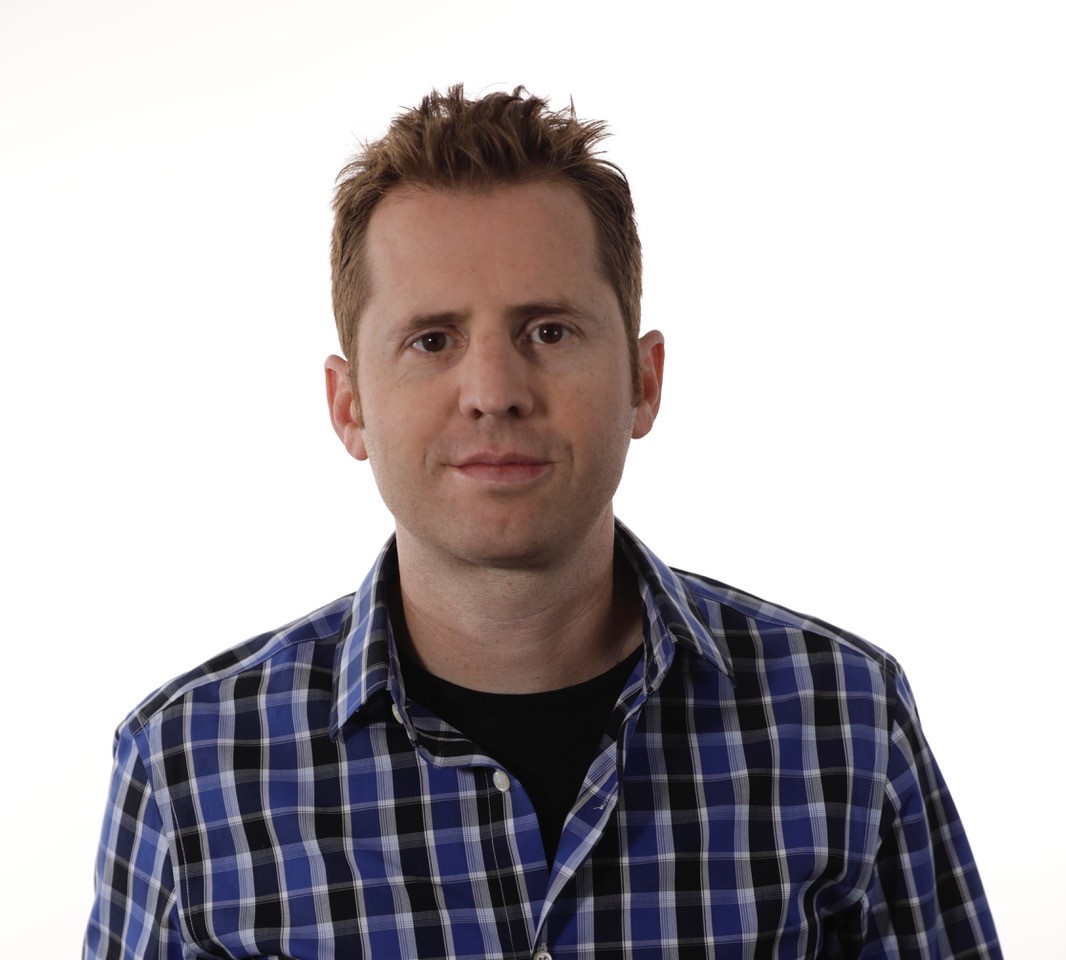
About the Speaker: Jeff Clune is the Loy and Edith Harris Associate Professor in Computer Science at the University of Wyoming and a Senior Research Manager and founding member of Uber AI Labs, which was formed after Uber acquired a startup he helped lead. Jeff focuses on robotics and training deep neural networks via deep learning, including deep reinforcement learning. Since 2015, a robotics paper he co-authored was on the cover of Nature, a deep learning paper from his lab was on the cover of the Proceedings of the National Academy of Sciences, he won an NSF CAREER award, his deep learning papers were awarded honors (best paper awards and/or oral presentations) at the top machine learning conferences (NeurIPS, CVPR, ICLR, and ICML), he was an invited speaker at five ICML and two NeurIPS workshops (including the NeurIPS Deep Reinforcement Learning Workshop), and he was invited to give a forthcoming ICML tutorial. His research is regularly covered in the press, including the New York Times, NPR, NBC, Wired, the BBC, the Economist, Science, Nature, National Geographic, the Atlantic, and the New Scientist. Prior to becoming a professor, he was a Research Scientist at Cornell University and received degrees from Michigan State University (PhD, master’s) and the University of Michigan (bachelor’s).
Towards a Lasting Human-AI Interaction
Speaker: Manuela Veloso, JPMorgan Chase
Time: 11:30 am - 12:30 pm Apr 4, 2019
Location: MakerSpace Event Space (6MTC/Rogers Hall), Brooklyn, NY
Abstract: Artificial intelligence, including extensive data processing, decision making and execution, and learning from experience, offers new challenges for an effective human-AI interaction. This talk delves into multiple roles humans can have in such interaction, as well as the underlying challenges to AI in particular in terms of collaboration and interpretability. The presentation is grounded within the context of autonomous mobile service robots, and applications to other areas.

About the Speaker: Manuela Veloso, Ph.D., Managing Director, Head of AI Research, J.P. Morgan & Herbert A. Simon University Professor, School of Computer Science, Carnegie Mellon University (on leave)
Manuela M. Veloso is the Head of J.P. Morgan AI Research, which pursues fundamental research in areas of core relevance to financial services, including data mining and cryptography, machine learning, explainability, and human-AI interaction. J.P. Morgan AI Research partners with applied data analytics teams across the firm as well as with leading academic institutions globally.
Professor Veloso is on leave from Carnegie Mellon University as the Herbert A. Simon University Professor in the School of Computer Science, and the past Head of the Machine Learning Department. With her students, she had led research in AI, with a focus on robotics and machine learning, having concretely researched and developed a variety of autonomous robots, including teams of soccer robots, and mobile service robots. Her robot soccer teams have been RoboCup world champions several times, and the CoBot mobile robots have autonomously navigated for more than 1,000km in university buildings.
Professor Veloso is the Past President of AAAI, (the Association for the Advancement of Artificial Intelligence), and the co-founder, Trustee, and Past President of RoboCup. Professor Veloso has been recognized with a multiple honors, including being a Fellow of the ACM, IEEE, AAAS, and AAAI. She is the recipient of several best paper awards, the Einstein Chair of the Chinese Academy of Science, the ACM/SIGART Autonomous Agents Research Award, an NSF Career Award, and the Allen Newell Medal for Excellence in Research.
See www.cs.cmu.edu/~mmv/Veloso.html for her scientific publications.
Agency in the Era of Learning Systems
Speaker: Jakob Foerster, University of Oxford, UK
Time: 11:00 am - 12:00 pm Apr 5, 2019
Location: 1MTC, 1930 Jacobs Seminar Room, Brooklyn, NY
Abstract: We commonly think of machine learning problems, such as machine translation, as supervised tasks consisting of a static set of inputs and desired outputs. Even reinforcement learning, which tackles sequential decision making, typically treats the environment as a stationary black box. However, as machine learning systems are deployed in the real world, these systems start having impact on each other and their users, turning their decision making into a multi-agent problem. It is time we start thinking of these problems as such, by directly accounting for the agency of other learning systems in the environment. In this talk we look at recent advances in the field of multi-agent learning, where accounting for agency can have drastic effects.
As a case study we present the “Bayesian Action Decoder” (BAD), which allows agents to directly reason over the beliefs of other agents in order to learn communication protocols in settings with limited public knowledge and actions that can be used to share information. BAD can be seen as a step towards a kind of “theory of mind” for AI agents and achieves a new state-of-the-art on the cooperative, partial-information, card-game Hanabi (“Spiel des Jahres” in 2013), an exciting new benchmark for measuring AI progress.
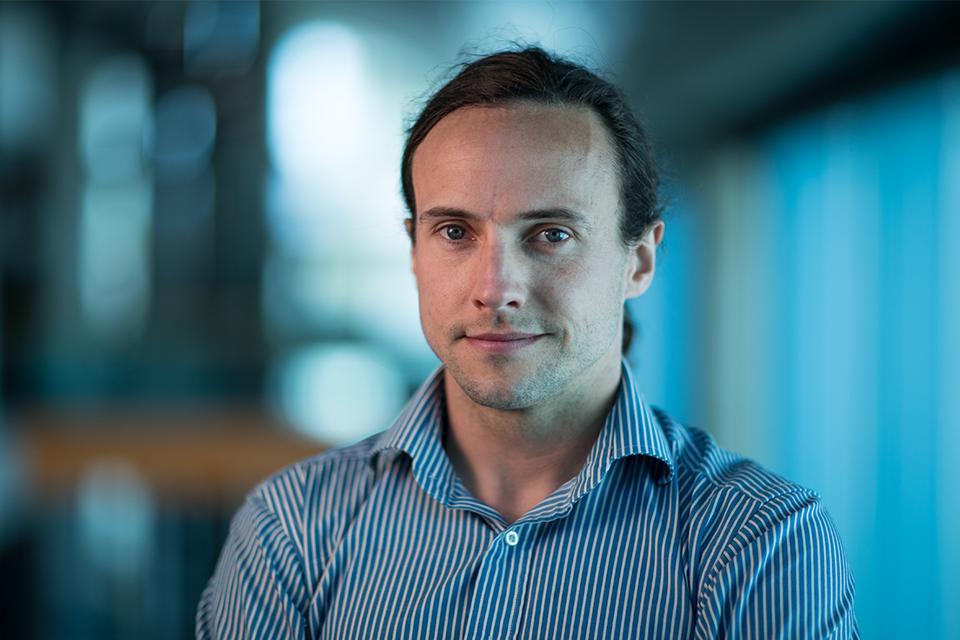
About the Speaker: Jakob Foerster recently obtained his PhD in AI at the University of Oxford, under the supervision of Shimon Whiteson. Using deep reinforcement learning (RL) he studies how accounting for agency can address multi-agent problems, ranging from the emergence of communication to non-stationarity, reciprocity and multi-agent credit-assignment. His papers have gained prestigious awards at top machine learning conferences (ICML, AAAI) and have helped push deep multi-agent RL to the forefront of AI research. During his PhD Jakob interned at Google Brain, OpenAI, and DeepMind. Prior to his PhD Jakob obtained a first-class honours Bachelor’s and Master’s degree in Physics from the University of Cambridge and also spent four years working at Goldman Sachs and Google. Previously he has also worked on a number of research projects in systems neuroscience, including work at MIT and research at the Weizmann Institute.
AI Seminar Series
Speaker: Eric Kandel, Columbia University
Time: 3:00 pm - 4:00 pm May 8, 2019
Location: 370 Jay Seminar Room 1201, Brooklyn, NY
Challenges and Opportunities in Future Wireless Net- works: 5G and Beyond
Speaker: Aissa Ikhlef, Durham University, UK
Time: 11:00 am - 12:00 pm Apr 8, 2019
Location: 370 Jay Room 1201, Brooklyn, NY
Abstract: Future wireless networks, fifth generation (5G) and beyond, are expected to support diverse applications with different quality-of-service (QoS) requirements such as smart cities, autonomous cars, drones, robots, virtual and augmented reality, etc. These networks are required to have high spectral efficiency to meet the predicted exponential growth in wireless data traffic. Additionally, the global energy consumption of wireless networks is expected to double within the next few years. Furthermore, wireless networks are facing increasing security threats in both number and sophistication. Due to the aforementioned challenges, wireless network providers face huge increases in capital and operating expenditures. As a result, the need of developing innovative solutions and strategies to enable wireless networks to meet the ever-increasing demand in wireless data in a secure and sustainable way is more pressing than ever. In this talk, I will first review some of the main challenges in the design of future wireless networks. Then, I will discuss some potential solutions to address these challenges through the use of various advanced technologies such as massive multiple-input multiple-output (MIMO), simultaneous wireless information and power transfer (SWIPT), physical layer security, cloud radio access networks (CRANs), unmanned aerial vehicles (UAVs), and machine learning (ML).

About the Speaker: Aissa Ikhlef (M’09-SM’17) received the B.S. degree in electrical engineering from the University of Constantine, Constantine, Algeria, in 2001, and the M.Sc. and Ph.D. degrees in Electrical Engineering from the University of Rennes 1, Rennes, France, in 2004 and 2008, respectively. From 2004 to 2008, he was with Sup ́elec, France, where he received the Ph.D. degree. From 2007 to 2008, he was a Lecturer with the University of Rennes 1. From 2008 to 2010, he was a Post-Doctoral Fellow with the Communication and Remote Sensing Laboratory, Catholic Uni- versity of Louvain, Louvain La Neuve, Belgium. He was a visiting Post-Doctoral Fellow with the University of British Columbia, Vancouver, BC, Canada, in 2009. From 2010 to 2013, he was with the Data Communications Group, University of British Columbia, as a Post-Doctoral Fellow. From 2013 to 2014, he was with Toshiba Research Europe Limited, Bristol, UK, as a Senior Research Engineer. From 2014 to 2016, he was with the School of Electrical and Electronic Engineering, Newcastle University, Newcastle upon Tyne, UK, as a Lecturer (Assistant Professor). Since 2016, he has been an Assistant Professor with the Department of Engineering, Durham University, Durham, UK. He has served as an Editor for the IEEE Communications Letters from 2014 to 2016. He co-organized several workshops and has served as technical program committee (TPC) member for several IEEE flagship conferences. His current research interests include machine learning, energy harvesting communications, physical layer security, unmanned aerial vehicles (UAVs) and massive MIMO.
Why do neural networks learn?
Speaker: Behnam Neyshabur, New York University
Time: 11:00 am - 12:00 pm Apr 15, 2019
Location: 370 Jay, Room 1201, Brooklyn, NY
Abstract: Neural networks used in practice have millions of parameters and yet they generalize well even when they are trained on small datasets. While there exists networks with zero training error and a large test error, the optimization algorithms used in practice magically find the networks that generalizes well to test data. How can we characterize such networks? What are the properties of networks that generalize well? How do these properties ensure generalization?
In this talk, we will develop techniques to understand generalization in neural networks. Towards the end, I will show how this understanding can help us design architectures and optimization algorithms with better generalization performance.

About the Speaker: Behnam Neyshabur is a postdoctoral researcher in Yann LeCun’s group at New York University. Before that, he was a member of Theoretical Machine Learning program lead by Sanjeev Arora at the Institute for Advanced Study (IAS) in Princeton. In summer 2017, he received a PhD in computer science at TTI-Chicago where Nati Srebro was his advisor. He is interested in machine learning and optimization and his primary research is on optimization and generalization in deep learning.
AI and Neuroscience: Bridging the Gap
Speaker: Irina Rish, IBM
Time: 11:00 am - 12:00 pm Apr 16, 2019
Location: 370 Jay, Room 1201, Brooklyn, NY
Abstract: The ultimate objective of understanding and modeling intelligent behavior is at the core of both neuroscience and artificial intelligence. Cross-fertilization between these fields has already proven to be extremely useful, both in terms of neuroscience informing AI, with the most prominent examples including deep learning and reinforcement learning, as well as AI helping to bring neuroscience to a new level. This talk is on overview of some of our projects on the intersection between these two disciplines. We start with a brief summary of "AI for Neuro" (e.g., statistical biomarker discovery from neuroimaging of mental disorders and an automated depression therapy models), and continue with an in-depth overview of "Neuro for AI", i.e. better AI algorithms development which draws inspirations from neuroscience. In particular, I will focus on the continual (lifelong) learning objective, and discuss several examples of more neuro-inspired approaches, including (1) neurogenetic online model adaptation in non-stationary environments, (2) more biologically plausible alternatives to back-propagation, e.g., local optimization for neural net learning via alternating minimization with auxiliary activation variables, and co-activation memory, (3) modeling reward-driven attention and attention-driven reward in contextual bandit setting, as well as (4) modeling and forecasting behavior of coupled nonlinear dynamical systems such as brain (from calcium imaging and fMRI) using a combination of analytical van der Pol model with LSTMs, especially in small-data regimes, where such hybrid approach outperforms both of its components used separately. However, besides bridging the gap between biological computation and AI algorithms, another important open question remains about how to bridge the second gap: between emerging novel AI algorithms and constraints/capabilities of analog neuromorphic hardware.

About the Speaker: Irina Rish is a researcher at the AI Foundations department of the IBM T.J. Watson Research Center. She received MS in Applied Mathematics from Moscow Gubkin Institute, Russia, and PhD in Computer Science from the University of California, Irvine. Her areas of expertise include artificial intelligence and machine learning, with a particular focus on probabilistic graphical models, sparsity and compressed sensing, active learning, and their applications to various domains, ranging from diagnosis and performance management of distributed computer systems (“autonomic computing”) to predictive modeling and statistical biomarker discovery in neuroimaging and other biological data. Irina has published over 70 research papers, several book chapters, two edited books, and a monograph on Sparse Modeling, taught several tutorials and organized multiple workshops at machine-learning conferences, including NIPS, ICML and ECML. She holds over 26 patents and several IBM awards. Irina currently serves on the editorial board of the Artificial Intelligence Journal (AIJ). As an adjunct professor at the EE Department of Columbia University, she taught several advanced graduate courses on statistical learning and sparse signal modeling.
Aerial Robotics: Novel Design Control Methods for Enabling Physical Interactive Tasks in Real World
Speaker: Antonio Franchi, LAAS-CNRS, Toulouse, France
Time: 11:00 am - 12:00 pm Apr 29, 2019
Location: 370 Jay, Room 1201, Brooklyn, NY
Abstract: Physical interactive tasks have been kept for a long time far from the conception and development of robotic flying systems. In recent times a few research groups in the world started to study the problem of elevating aerial vehicles from the condition of pure observers to the one of fully-mature robotic actors able to help humans in manipulating and operating in places that are hardly accessible with other type robots.
In this seminar I will present, from an application driven perspective, the main theoretical and technical challenges in this field. I will introduce the recent results of our group in the design of flying machines that are the most suited for aerial physical interaction — such as multi-directional thrust vehicles — and illustrate the novel concept of flying companion and MAGMaS systems — where one or more aerial robots collaborate with ground robots in order to comanipulate long objects. I will then show how the control of physical interaction can be used to achieve capabilities that are otherwise impossible to standard (contact-free) aerial vehicles, such as stable landing on inclined surfaces, physically inspecting curved pipes with sensor probes, and how a proper design of the aerial manipulator may
greatly simplify the end-effector nonlinear control problem.
I will conclude the seminar with some insights on current and future directions in this exciting domain of robotics.

About the Speaker: Antonio Franchi is a Tenured Researcher of CNRS, the French National Centre for Scientific Research, one of the world's leading research institutions (http://www.cnrs.fr/en/cnrs). He is based at LAAS-CNRS (RIS team) in Toulouse, France, since 2014.
From 2010 to 2013 he was a Research Scientist and then a Senior Research Scientist at the Max Planck Institute for Biological Cybernetics in Germany, and the scientific leader of the group ‘Autonomous Robotics and Human-Machine Systems’.
He received the HDR (French Professorial Habilitation) from the National Polytechnic Institute of Toulouse, the Ph.D. degree in Control and System Theory and master degree in Electronic Engineering from the Sapienza University of Rome, Italy. He was a visiting scholar at the University of California at Santa Barbara.
His main research interests in robotics are motion control, estimation, hardware design, and human-machine systems. His main areas of expertise are aerial robotics and multi-robot systems. He published about 130 articles in international journals, conferences, and books, and in 2010 he was awarded with the ‘IEEE RAS ICYA Best Paper Award’ for one of his works on Multi-robot Exploration.
He is a IEEE Senior Member and Associate Editor of the IEEE Transactions on Robotics. He has been Associate Editor of the IEEE Robotics & Automation Magazine (2013 to 2016), IEEE ICRA (2014 to 2019), IEEE/RSJ IROS (2014 to 2019) and the IEEE Aerospace and Electronic Systems Magazine (2015).
He is currently coordinator of the ANR MuRoPhen project (2018-2021), the CNRS PI of the AEROARMS EU H2020 project (2015-2019), coordinator of the MBZIRC 2020 LAAS team project (2018-2020), and co-coordinator of the FlyCrane Occitanie Pre-Maturation project (2019-2020). He has also a prominent role in the ANR Flying Co-Worker project (2019-2022) and the PRO-ACT EU H2020 project (2019-2021). In the past, he also participated to the ARCAS EU FP7 (2014-2010) project.
He is a co-chair of the IEEE RAS Technical Committee on Multi-Robot systems (400+ members), which he co-founded in 2014, and was the recipient of the IEEE RAS most active TC Award 2018.
He co-funded and was the program co-chair of the IEEE-sponsored biannual International Symposium on Multi-robot and Multi-agent Systems (MRS 2017 in Los Angeles, MRS 2019 in New Brunswick).
RowHammer and Beyond
Speaker: Onur Mutlu, ETH Zurich. Switzerland/CMU
Time: 11:00 am - 12:00 pm Apr 25, 2019
Location: 2 MetroTech Center, Room 10.099, Brooklyn, NY
Abstract: We will discuss the RowHammer problem in DRAM, which is a prime (and likely the first) example of how a circuit-level failure mechanism in Dynamic Random Access Memory (DRAM) can cause a practical and widespread system security vulnerability. RowHammer is the phenomenon that repeatedly accessing a row in a modern DRAM chip predictably causes errors in physically-adjacent rows. It is caused by a hardware failure mechanism called read disturb errors. Building on our initial fundamental work that appeared at ISCA 2014, Google Project Zero demonstrated that this hardware phenomenon can be exploited by user-level programs to gain kernel privileges. Many other recent works demonstrated other attacks exploiting RowHammer, including remote takeover of a server vulnerable to RowHammer. We will analyze the root causes of the problem and examine solution directions. We will also discuss what other problems may be lurking in DRAM and other types of memories, e.g., NAND flash and Phase Change Memory, which can potentially threaten the foundations of reliable and secure systems, as the memory technologies scale to higher densities.
A short accompanying, yet slightly outdated paper, appearing at DATE 2017, can be found here:
https://people.inf.ethz.ch/omutlu/pub/rowhammer-and-other-memory-issues_date17.pdf

About the Speaker: Onur Mutlu is a Professor of Computer Science at ETH Zurich. He is also a faculty member at Carnegie Mellon University, where he previously held Strecker Early Career Professorship. His current broader research interests are in computer architecture, systems, hardware security, and bioinformatics. A variety of techniques he, along with his group and collaborators, has invented over the years have influenced industry and have been employed in commercial microprocessors and memory/storage systems. He obtained his PhD and MS in ECE from the University of Texas at Austin and BS degrees in Computer Engineering and Psychology from the University of Michigan, Ann Arbor. He started the Computer Architecture Group at Microsoft Research (2006-2009), and held various product and research positions at Intel Corporation, Advanced Micro Devices, VMware, and Google. He received the inaugural IEEE Computer Society Young Computer Architect Award, the inaugural Intel Early Career Faculty Award, US National Science Foundation CAREER Award, Carnegie Mellon University Ladd Research Award, faculty partnership awards from various companies, and a healthy number of best paper or "Top Pick" paper recognitions at various computer systems, architecture, and hardware security venues. He is an ACM Fellow "for contributions to computer architecture research, especially in memory systems", IEEE Fellow for "contributions to computer architecture research and practice", and an elected member of the Academy of Europe (Academia Europaea). For more information, please see his webpage at https://people.inf.ethz.ch/omutlu/.
AI Seminar Series: The Biology of Memory and Age Related Memory Loss
Speaker: Eric R. Kandel, Columbia University
Time: 3:00 pm - 4:00 pm May 8, 2019
Location: 370 Jay Street, Auditorium 1201 Brooklyn, NY
Abstract: I will consider the neural systems and molecular mechanisms that contribute to learning and long-term memory. I will divide my talk into two parts: First, I will consider how different memory systems were identified in the human brain and how they were shown to be involved in two major forms of neural memory storage: 1) simple memory for perceptual and motor skills and 2) complex memory for facts and events. I will then go on to outline studies that demonstrated that long-term memory is reflected in the growth of new synaptic connections. Finally, I will discuss how our insights into memory storage are allowing us to understand the two major forms of age related memory loss.
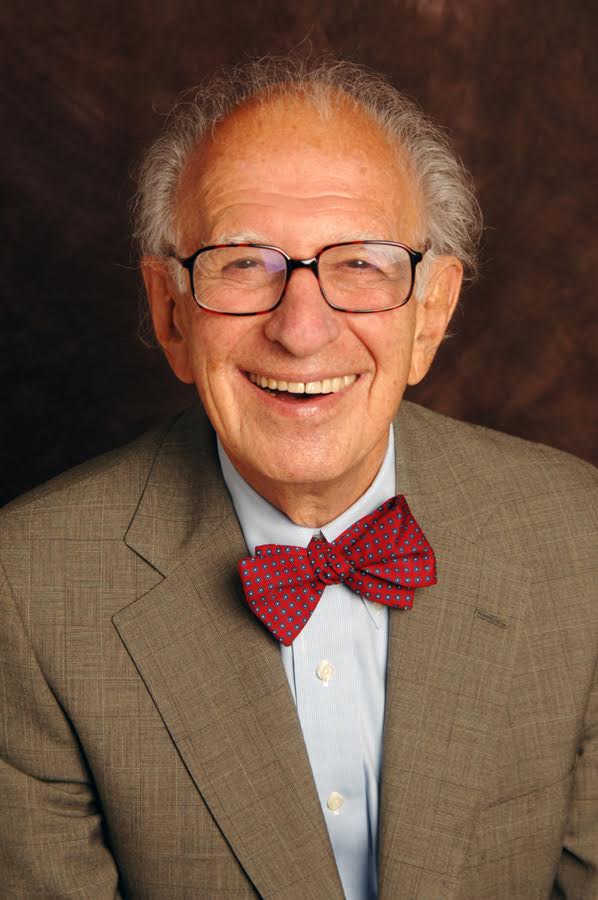
About the Speaker: Eric R. Kandel, M.D., is University Professor at Columbia; Kavli Professor and Director, Kavli Institute for Brain Science; Co-Director, Mortimer B. Zuckerman Mind Brain Behavior Institute; and a Senior Investigator at the Howard Hughes Medical Institute. A graduate of Harvard College and NYU School of Medicine, Dr. Kandel trained in Neurobiology at the NIH and in Psychiatry at Harvard Medical School. He joined the faculty of the College of Physicians and Surgeons
at Columbia University in 1974 as the founding director of the Center for Neurobiology and Behavior. At Columbia Kandel organized the neuroscience curriculum. He is an editor of Principles of Neural Science, the standard textbook in the field now in its 5th edition. In 2006, Kandel wrote a book on the
brain for the general public entitled In Search of Memory: The Emergence of a New Science of Mind, which won both the L.A. Times and U.S. National Academy of Science Awards for best book in Science and Technology in 2008. A documentary film based on that book is also entitled In Search of Memory. In
2012 Kandel wrote The Age of Insight: The Quest to Understand the Unconscious in Art, Mind, and Brain, from Vienna 1900 to the Present, which won the Bruno-Kreisky Award in Literature, Austria’s highest literary award. Kandel’s book entitled, Reductionism in Art and Brain Science: Bridging the Two
Cultures was published in 2016 by Columbia University Press. Kandel’s newest Book, The Disordered Mind: What Unusual Brains Tell Us About Ourselves published by Farrar, Straus and Giroux has just been released.
Eric Kandel’s research has been concerned with the molecular mechanisms of memory storage in Aplysia and mice. More recently, he has studied animal models in mice of memory disorders, mental illness and nicotine addiction. Kandel has received twenty-four honorary degrees, is a member of the
U.S. National Academy of Sciences as well as being a Foreign Member of the Royal Society of London and a member of the National Science Academies of Austria, France, Germany and Greece. He has been recognized with the Albert Lasker Award, the Heineken Award of the Netherlands, the Gairdner Award of Canada, the Harvey Prize and the Wolf Prize of Israel, the National Medal of Science USA and the Nobel Prize for Physiology or Medicine in 2000.
Spiking Neural Networks: A Stochastic Signal Processing Perspectiv
Speaker: Osvaldo Simeone, King's College London, UK
Time: 11:00 am - 12:00 pm Jun 19, 2019
Location: 2MTC, Room 10.099, Brooklyn, NY
Abstract: Spiking Neural Networks (SNNs) are distributed trainable systems whose computing elements, or neurons, are characterized by internal analog dynamics and by digital and sparse synaptic communications. The sparsity of the synaptic spiking inputs and the corresponding event-driven nature of neural processing can be leveraged by hardware implementations that have demonstrated significant energy reductions as compared to conventional Artificial Neural Networks (ANNs). SNNs have been traditionally studied in the field of theoretical neuroscience through the lens of biological plausibility. In contrast, this talk aims at providing an introduction to models, learning rules, and applications of SNNs from the viewpoint of stochastic signal processing. To this end, it adopts discrete-time probabilistic models for networked spiking neurons, and it derives supervised and unsupervised learning rules from first principles by using variational inference. Examples and open research problems are also provided.
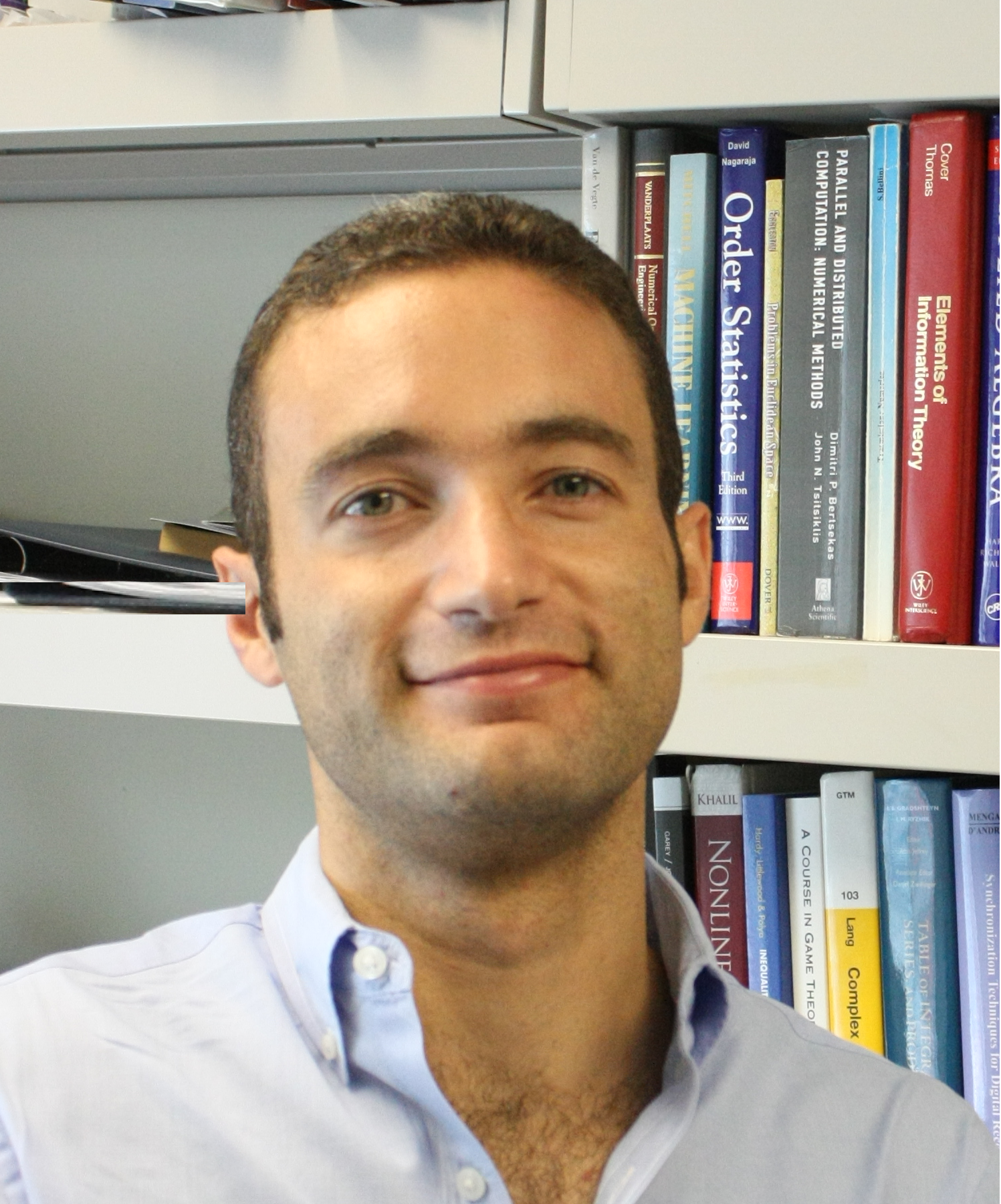
About the Speaker: Osvaldo Simeone is a Professor of Information Engineering with the Centre for Telecommunications Research at the Department of Informatics of King's College London. He received an M.Sc. degree (with honors) and a Ph.D. degree in information engineering from Politecnico di Milano, Milan, Italy, in 2001 and 2005, respectively. From 2006 to 2017, he was a faculty member of the Electrical and Computer Engineering (ECE) Department at New Jersey Institute of Technology (NJIT), where he was affiliated with the Center for Wireless Information Processing (CWiP). His research interests include wireless communications, information theory, optimization and machine learning. Dr Simeone is a co-recipient of the 2019 IEEE Communication Society Best Tutorial Paper Award, the 2018 IEEE Signal Processing Best Paper Award, the 2017 JCN Best Paper Award, the 2015 IEEE Communication Society Best Tutorial Paper Award and of the Best Paper Awards of IEEE SPAWC 2007 and IEEE WRECOM 2007. He was awarded a Consolidator grant by the European Research Council (ERC) in 2016. His research has been supported by the U.S. NSF, the ERC, the Vienna Science and Technology Fund, as well as by a number of industrial collaborations. He currently serves in the editorial board of the IEEE Signal Processing Magazine, and he is a Distinguished Lecturer of the IEEE Information Theory Society. Dr Simeone is a co-author of two monographs, an edited book published by Cambridge University Press, and more than one hundred research journal papers. He is a Fellow of the IET and of the IEEE.