Fall 2023 ECE Seminars
Date | Time | Speaker | From | Title | Recording |
---|---|---|---|---|---|
Thu, Sep 7 | 11am - 12pm | Dr. Xuan (Sharon) Di | Columbia University | AI for Urban Transportation Digital Twin | |
Mon, Sep 11 | 11am - 12pm | Cosima du Pasquier | Stanford University | Haptic Knits: A New Paradigm in Wearable Technologies | |
Thu, Sep 14 | 2:30pm - 3:30pm | Arthur J. Krener | American Mathematical Society (AMS) | Boundary Stabilization of Polynomial Reaction Diffusion Equations | |
Mon, Sep 26 | 9:30am- 11am | Maria Florina Balcan | Carnegie Mellon University | Modern AI Series: Machine Learning for Algorithm Design | |
Mon, Sep 26 | 11am - 12pm | Jim Kurose | University of Massachusetts, Amherst | Jack Wolf Lecture: The Software-ization of Networking: Protocols, People, Pedagogy | |
Mon, Sep 26 | 2pm - 3pm | Jia Xu | Stevens Institute | Generalization by Diversification | |
Wed, Sep 28 | 11am - 12pm | Ashis G. Banerjee | University of Washington | An Engineer's Foray into Topological Learning | |
Wed, Sep 28 | 3:30pm - 4:30pm | Dr. Sami Haddadin | TUM School of Computation, Information and Technology | Information and Technology | Tactile Robots: Building the Machine and Learning the Self | |
Thu, Sep 29 | 11:30am - 12:30pm | Joseph Bentsman | University of Illinois at Urbana Champaign | Enabling the Energy-based Autonomous Robotic Surgery | |
Mon, Oct 2 | 11am - 12pm | Dr. Qurrat-Ul-Ain Nadeem | University of British Columbia, Canada | Wireless Communication Using Reconfigurable Intelligent Surfaces | |
Thu, Oct 5 | 2pm - 3pm | M. Selim Ünlü | Boston University | Digital Biodetection by Pixel-Diversity IRIS | |
Tue, Oct 10 | 2pm - 3pm | Shirin Jalali | Rutgers University | Mathematical underpinnings of emerging computational imaging | |
Thu, Oct 12 | 10am - 11am | Aydin Aysu | North Carolina State | Machine Learning and Side-Channel Analysis: Happily Ever After | |
Thu, Oct 12 | 12pm - 1pm | Erol Gelenbe | Academia Europaea | Random Neural Networks (RNN) for Accurate CyberAttack Detection | |
Wed, Oct 18 | 1:30pm - 2:30pm | Ki-Uk Kyung | NYU Tandon School of Engineering, KAIST Visiting Professor | Are Soft Actuators Powerful Enough to Revolutionize Robotic Interfaces? | |
Thu, Oct 19 | 11am - 12pm | Raghavendra (Raghav) Pothukuchi | Yale University | Machines that Talk to the Brain and Think Like the Mind | |
Tue, Oct 24 | 9am - 11:30am | Sanja Fidler | University of Toronto | Modern AI Series: Generative AI for 3D Content | |
Tue, Oct 24 | 3pm - 4pm | Baosen Zhang | University of Washington | Safe and Efficient Learning for Power System Operations | |
Thu, Oct 26 | 11am - 12pm | Aditya Ramamoorthy | Iowa State University | Coded Matrix Computation: Numerical Stability, Partial Stragglers | |
Fri, Oct 27 | 12:30m - 1:30m | Rick Parimi | Alexis Networks, Inc. | ECE SEMINAR | |
Mon, Nov 6 | 11am - 12pm | Khalil Ramadi | NYU Abu Dhabi, Assistant Professor of Biomedical Engineering | Ingestible Electronics for Interfacing with the Gastrointestinal Tract | |
Mon, Nov 6 | 12:15pm - 1:15pm | Jovica V Milanović | University of Manchester, United Kingdom | Research Challenges Facing Planning and Operation of Net-zero Power Systems | |
Fri, Nov 10 | 11am - 12pm | Prashant Nair | University of British Columbia, Vancouver Campus | From Bits to Beyond: Crafting Secure and Scalable Memory Architectures for Next-Generation Systems | |
Mon, Nov 13 | 11am - 12pm | Dr. Eleni Kelasidi | Autonomous and Robotic Aquaculture Systems Lab | Autonomous and Robotic Aquaculture Systems Lab | Autonomous Robotic Systems in Aquaculture | |
Tue, Nov 14 | 11am - 12pm | Zhe Sage Chen | New York University, Grossman School of Medicine | A Promising Tale: Machine Learning for Neuroscience | |
Thu, Nov 16 | 11am - 12pm | Dr. YingLi Tian | City College of New York (CCNY) | AI-driven Automated Medical Image Analysis | |
Wed, Dec 6 | 10am - 11am | Weiwen Jiang | George Mason University | QDA: Quantum Design Automation Toward Practical Quantum Computing | |
Thu, Dec 7 | 11am - 12pm | Nizar Touzi | NYU Tandon School of Engineering, Chair of the FRE Department | Mean Field Games approach to Systemic Risk | |
Mon, Dec 18 | 11am - 12pm |
Catie Cuan Ken Goldberg |
Stanford University University of California, Berkeley |
Teaching Robots to Navigate, Grasp, and Dance |
Recording |
AI for Urban Transportation Digital Twin
Speaker: Dr. Xuan (Sharon) Di, Columbia University
Date: Thu, Sep 7
Abstract: Transportation digital twins have become increasingly popular tools to improve traffic efficiency and safety. However, the majority of effort nowadays is focused on the “eyes” of the digital twin, which is object detection using computer vision. I believe the key to empowering the intelligence of a transportation digital twin lies in its “brain,” namely, how to utilize the information extracted from various sensors to infer traffic dynamics evolution and devise optimal control and management strategies with real-time feedback to guide the transportation ecosystem toward a social optimum.
My research aims to employ tools including machine learning and game theory to develop an urban transportation digital twin, leveraging data collected from the NSF PAWR COSMOS city-scale wireless testbed being deployed in West Harlem next to the Columbia campus. In this talk, I will primarily focus on two solutions: (1) scientific machine learning that leverages both domain knowledge and available data, and (2) mean field game that bridges the gap between micro- and macroscopic behaviors of multi-agent dynamical systems. In the first topic, physics-informed deep learning will be introduced and applied to traffic state estimation and uncertainty quantification. In the second topic, I will introduce how to model behaviors of new actors (e.g., a large number of autonomous vehicles) in a transportation system and their interaction with existing actors (e.g., human-driven vehicles).

About the Speaker: Dr. Xuan (Sharon) Di is an Associate Professor in the Department of Civil Engineering and Engineering Mechanics at Columbia University in the City of New York and serves on a committee for the Smart Cities Center in the Data Science Institute. Dr. Di directs the DitecT (Data and innovative technology-driven Transportation) Lab. Her field of research is transportation systems engineering, with a focus on reshaping technological innovation for social good. Her research lies at the intersection of game theory and machine learning. She specializes in autonomous vehicle control in mixed traffic, cyber physical transportation systems, multi-modal mobility optimization, and transportation and health, using innovative tools including physics-informed deep learning and mean-field games. Details about DitecT Lab and her research can be found in the following link: http://sharondi-columbia.wixsite.com/ditectlab.
Dr. Di received her Ph.D. degree from the Department of Civil, Environmental, and Geo-Engineering at the University of Minnesota, Twin Cities. Prior to joining Columbia, she was a Postdoctoral Research Fellow at the University of Michigan Transportation Research Institute (UMTRI). Dr. Di received a number of awards including NSF CAREER, International Data Corporation’s Smart Cities North America Awards (2023), Best Paper Award from ACM SIGKDD Workshop on Urban Computing (2022), Amazon AWS Machine Learning Research Award (2020), Transportation Data Analytics Contest Winner from Transportation Research Board (TRB) (2017), the Dafermos Best Paper Award Honorable Mention from the TRB Network Modeling Committee (2017), Chan Wui & Yunyin Rising Star Workshop Fellowship for Early Career Professionals from TRB, Outstanding Presentation Award from INFORMS (2016), the Best Paper Award (2014) and Best Graduate Student Scholarship (2013) from North-Central Section Institute of Transportation Engineers (ITE). She serves as the Associate Editor for the journals including Transportation Science, Transportation Research Part B, and IEEE on ITS, and won the Transportation Science Meritorious Service Award from INFORMS in 2022. She was a participant in a long program on Mathematical Challenges and Opportunities for Autonomous Vehicles at the Institute for Pure & Applied Mathematics (IPAM) in UCLA in 2020. She has been a visiting research fellow at Bielefeld University’s Center for Interdisciplinary Research (ZiF) in Germany since 2021.
Haptic Knits: A New Paradigm in Wearable Technologies
Speaker: Cosima du Pasquier, Stanford University
Date: Mon, Sep 11
Abstract: Traditional knitting conjures images of manual fabrication with large wooden needles, a thick ball of yarn, and bulky sweaters. In contrast, modern industrial knitting has made incredible advances, using up to 34 different materials simultaneously, a variety of stitches, patterns, numbers of layers; from simple 2D fabric to 3D shapes like whole gloves, molds, and scaffolds. However, the complexity of design and planning increases with every stitch. Modern knitting design requires iteration and a vast know-how. I will present our work using computational modeling, benchtop experiments, and human user studies to create wearable devices we call haptic knits. We are building a modeling and optimization pipeline that can facilitate complex knit design, considering material and geometrical nonlinearity in anything from textiles to high-dimensional 3D structures. Combining elements from soft robotics and knitting, we use multi-material and multi-stitch knitting to achieve variable stiffness textiles that control and direct load transmission from pneumatic actuators. We use this concept in a haptic sleeve to provide sensory feedback and show that it can be applied to a host of exciting applications, from social touch communication to rehabilitation. I will place this work in the context of my prior work in mechanics, soft actuation, and user-centered design.

About the Speaker: Cosima du Pasquier is a Postdoc in the Department of Mechanical Engineering at Stanford University. Working with her postdoc sponsor Allison Okamura, as well as academic collaborators from the Massachusetts Institute of Technology (MIT), Georgia Tech, and University of Houston, she focuses on design, modeling, and fabrication of wearable technologies for healthcare. Prior to joining Stanford, Cosima received her BSc, MSc, and PhD in Mechanical Engineering from ETH Zurich in 2014, 2017, and 2022 respectively. Her PhD dissertation was awarded the ETH Medal. Dr. du Pasquier’s research combines structural mechanics, materials, and design. During her PhD at the Engineering Design and Computing (EDAC) Lab at ETH Zurich, she focused on modeling and optimizing deformation of morphing structures using soft printed actuators. In her postdoc, she focuses streamlining the design of assistive wearable technologies, such as rehabilitative devices for stroke survivors and wearable haptic platforms. Dr. du Pasquier’s work has appeared in a number of peer-reviewed journals, including Soft Robotics, Additive Manufacturing, Smart Materials and Structures, and Structural and Multidisciplinary Optimization. During her MSc and PhD, she collaborated with academic partners from MIT, which culminated in the founding of the startup Rapid Liquid Printing Co., for which she is an advisor. Her current work is funded by the U.S. National Science Foundation through the Convergence Accelerator and Human-Centered Computing programs.
Boundary Stabilization of Polynomial Reaction Diffusion Equations
Speaker: Arthur J. Krener, Researcher, American Mathematical Society (AMS)
Date: Thu, Sep 14
Abstract: We show how to stabilize a polynomial reaction diffusion system using boundary control, integration by parts, completing the square and an infinite dimensional extension of Al'brekht's method.

About the Speaker: Arthur J. Krener is a mathematician whose research interests are in developing methods for the control and estimation of nonlinear dynamical systems and stochastic processes. In 1971 he received the PhD in Mathematics from the University of California, Berkeley and joined the faculty of the University of California, Davis. He retired from UCD in 2006 as a Distinguished Professor of Mathematics and he currently is a Distinguished Research Professor of Applied Mathematics at the Naval Postgraduate School in Monterey, CA. He has held visiting positions at Harvard University, Imperial College, NASA Ames Research Center, the University of California, Berkeley, the University of Paris, the University of Maryland, the University of Padua and North Carolina State University.
He is a Fellow of the American Mathematical Society (AMS), a Fellow of the Society for Industrial and Applied Mathematics (SIAM), a Life Fellow of the Institute of Electrical and Electronics Engineers (IEEE) and a Fellow of the International Federation for Automatic Control (IFAC). He was the founding Chair of the SIAM Activity Group on Control and Systems Theory. He has authored or coauthored over 150 scientific papers and has given numerous invited addresses at professional meetings. He has organized several major conferences including the SIAM Conferences on Control and its Applications in 1989 and 2007 both in San Francisco and the IFAC NOLCOS at Lake Tahoe in 1996 and again in Monterey in 2016.
Krener has received numerous honors and awards during his career. These include a John Simon Guggenheim Fellowship in 2002, a Statistical and Applied Mathematical Sciences Institute University Fellow in 2004, the W. T. and Idalia Reid Prize from SIAM “for fundamental contributions to the control and estimation of nonlinear dynamical systems and stochastic processes” in 2004, the IEEE Control System Society Bode Prize Lecture in 2006, the Certificate of Excellent Achievements from the IFAC Technical Committee on Nonlinear Control in 2010, the American Automatic Control Council Richard E. Bellman Control Heritage Award in 2012 and the IEEE Field Award in Control in 2016
Modern AI Series: Machine Learning for Algorithm Design
Speaker: Maria Florina Balcan, Carnegie Mellon University
Date: Mon, Sep 26
Abstract: The classic textbook approach to designing and analyzing algorithms assumes worst-case instances of the problem, about which the algorithm designer has absolutely no information at all. While highly desirable when achievable, such worst-case guarantees — either for solution quality or running time or other performance measures — are often weak for many algorithmic problems. Consequently, rather than using off the shelf algorithms with weak worst-case guarantees, practitioners often employ a data-driven algorithm design approach; specifically, given an application, they use machine learning and instances of the problem from the specific domain to learn a method that works best in that domain. A major question is what provable guarantees do these learning augmented algorithmic techniques enjoy. In this talk, I will describe general analysis techniques developed in my group for data-driven algorithms. I will discuss both for the batch and online scenarios where a collection of typical problem instances from the given application are presented either all at once or in an online fashion, respectively.

About the Speaker: Maria Florina Balcan is the Cadence Design Systems Professor of Computer Science in the School of Computer Science at Carnegie Mellon University. Her main research interests are machine learning, artificial intelligence, theory of computing, and algorithmic game theory. She is a Simons Investigator, a Sloan Fellow, a Microsoft Research New Faculty Fellow, and the recipient of the ACM Grace Murray Hopper Award, NSF CAREER award, and several best paper awards. She has co-chaired major conferences in the field: the Conference on Learning Theory (COLT) 2014, the International Conference on Machine Learning (ICML) 2016, and Neural Information Processing Systems (NeurIPS) 2020. She has also been the general chair for the International Conference on Machine Learning (ICML) 2021, a board member of the International Machine Learning Society, and a co-organizer for the Simons semester on Foundations of Machine Learning.
Jack Wolf Lecture: The Software-ization of Networking: Protocols, People, Pedagogy
Speaker: Jim Kurose, University of Massachusetts, Amherst
Date: Mon, Sep 26
Abstract: It has been said that “software is eating the world.” With the arrival of software-defined networking (SDN), software is “eating” networking as well. In this talk, we consider the impact of SDN on the evolution of network protocols, on network management and “people in the loop”, and on how and what we will teach to future generations of networking students.
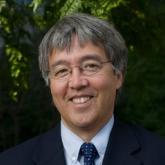
About the Speaker: Jim Kurose is a Distinguished University Professor of Computer Science Emeritus at the University of Massachusetts Amherst. His research interests include computer network architecture and protocols, network measurement, sensor networks, and multimedia communication. From 2015 to 2019, Jim served as Assistant Director at the US National Science Foundation, where he led the Directorate of Computer and Information Science and Engineering, and in 2018, served as the Assistant Director for Artificial Intelligence in the White House Office of Science and Technology Policy.
He has also served as a department chair, dean and association chancellor at UMass. He has received a number of awards for his research, teaching and service, including the IEEE Infocom Award, the ACM SIGCOMM Lifetime Achievement Award, the ACM Sigcomm Test of Time Award, the IEEE Computer Society Taylor Booth Education Medal, and the CRA Distinguished Service Award.
With Keith Ross, he is the co-author of the best-selling textbook, Computer Networking: a Top Down Approach (Pearson), now in its 8th edition. He is amember of the US National Academy of Engineering and a Fellow of the ACM, IEEE and AAAS.
Generalization by Diversification
Speaker: Jia Xu, Stevens Institute
Date: Mon, Sep 26
Abstract: Over the last decade, Deep Learning (DL) has risen to prominence, revolutionizing the field of machine learning. However, the journey to achieve robust generalization remains incomplete, with issues like out-of-domain or noisy data persistently haunting the progress. Conventional strategies for enhancing generalization often involve trade-offs, either sacrificing accuracy in specific domains or relying on a priori knowledge of the target domain, an unrealistic condition in real-world scenarios. This talk will delve into the heart of these challenges by posing two pivotal questions: (Q1) "Is it feasible to train a more generalized machine learning model using a smaller dataset?" and (Q2) "Can we forge a universal language representation to elevate Natural Language Processing (NLP) applications?" I will unveil a novel concept, "semantic diversity", embracing data simplification by identifying representative terms. The core idea is that a concise yet expressive data representation fosters a profound comprehension of linguistic structures and meanings, ultimately leading to lower generalization errors.
Our contributions encompass the following two aspects: (Q1) Data Selection for Generalization: I will introduce cutting-edge deep reinforcement learning strategies bolstered by inventive diversity-measure-based reward functions for data selection. These approaches result in remarkable performance improvements, boasting up to +40% accuracy gains over state-of-the-art methods in tasks such as out-of-domain language modeling and sentiment analysis. (Q2) Language Representation Enhancement: I will discuss the development of a suite of word-coding algorithms by grouping words with semantic diversity dedicated to elevating language representation. Empirical results demonstrate the superiority of these coding methods, consistently outperforming subword/word-based multilingual machine translation baselines across twelve language pairs, particularly beneficial for scarce-resource languages with up to +18.5 BLEU points gain, an +840% relative improvement. By diversifying data and representation units, we elucidate an intriguing yet promising pathway toward generalization.

About the Speaker: Jia Xu is an assistant professor at the Stevens Institute of Technology, and previously, she was a faculty member and Ph.D. advisor at Tsinghua University. Her research interests are Machine Learning and Natural Language Processing (NLP), focusing on highly competitive AI systems. She has more than 40 papers and regularly publishes in mainstream venues in NLP and machine learning (e.g., AAAI, ICML, ACL, EMNLP, NAACL) with 1220 citations. Professor Xu holds a Diploma from TU-Berlin and a Doctorate degree from RWTH Aachen University in Germany. During this time, she had industrial Internships at IBM in Watson and Microsoft Research (MSR) Redmond. Professor Xu has a unique record of winning over ten NLP competition awards with her team, including WMT and NIST open machine translation. Her team, with members from five nationalities, was selected for the final five of AlexaPrize social bot challenge 5.
An Engineer's Foray into Topological Learning: Addressing Challenges in Mobile Robot Perception and Mapping
Speaker: Ashis G. Banerjee, University of Washington
Date: Wed, Sep 28
Abstract: Topological learning (TL), referring to a synergy of computational topology and machine learning, has recently emerged as an effective pattern recognition framework for noisy, high-dimensional problems. The recognition happens by first identifying the topological structures that encode the shape and connectedness information among the observations (sample), and then characterizing the structures based on their relative persistence over a wide range of spatial and/or temporal scales. In this talk, I discuss successful demonstrations of TL for challenging mobile robot perception and mapping problems in unseen environments. Our novel adaptation of TL recognizes occluded objects and anomalies significantly more accurately than other state-of-the-art shape or learning-based methods without requiring real-world training samples. I conclude by pointing out future research directions for active multi-robot coordination.

About the Speaker: Ashis G. Banerjee is an Associate Professor of Industrial & Systems Engineering and Mechanical Engineering at the University of Washington (UW). Prior to joining UW, he was a Research Scientist at GE Global Research and a Postdoctoral Associate at MIT. He obtained his Ph.D. and M.S. in Mechanical Engineering from the University of Maryland (UMD), College Park, and B.Tech. in Manufacturing Science and Engineering from IIT Kharagpur. Dr. Banerjee has authored sixty peer-reviewed publications on a broad range of research topics spanning AI-enabled autonomous robotics, machine learning-driven decision making, and smart manufacturing. His research has been well supported by a large number of organizations, including the DOE, MxD, NAVSEA, ONR, WA State, Amazon Robotics, Boeing, and PACCAR. He has received several honors including the 2019 Amazon Research Award, 2012 Most Cited Paper Award from the Computer-Aided Design journal, and 2009 Best Mechanical Engineering Dissertation Award at UMD. He serves as a Senior Editor of the IEEE Robotics and Automation Letters, and an Associate Editor of the ASME Journal of Mechanisms and Robotics and the Springer Journal of Micro-Bio Robotics.
Tactile Robots: Building the Machine and Learning the Self
Speaker: Dr. Sami Haddadin, TUM School of Computation, Information and Technology
Date: Wed, Sep 28
Abstract: The development of robots that can learn to interact with the world and manipulate its objects has emerged as one of the greatest and, so far, largely unsolved challenges in robotics research. In this talk, I will argue that developing such advanced machines requires transitioning from classical manual design with purely model-based control to a novel paradigm. We must allow the machine to autonomously develop its own blueprint and generate its topological, kinematic, and dynamic self. Building on this, it shall develop controls for its own body as it moves, learns to manipulate objects in a controlled way, and sensitively interacts with the world.
Drawing from our work in torque-controlled lightweight robots towards human-safe tactile robots that can manipulate, fly, or drive, I explain the technological quantum leaps that have recently taken place. In particular, this progress was made possible by human-centered design, soft and force-sensitive control, contact reflexes, and model-based machine learning. In the real world, by enabling human-robot coexistence, collaboration, and interaction for the first time, this robotic technology has proven transformative to traditional manufacturing already around the globe. It increasingly impacts professional services, domestic applications, medicine, and healthcare.
Then, I will use our current work to chart the path toward the next generation of tactile machines. We have taken the first steps towards increasingly autonomous designing and building machines that can learn their self and thus adapt to changes in body topology and, ultimately, their entire dynamics. Finally, I will present recent results on designing modular control and learning architectures that achieve complex behaviors for challenging manipulation problems while being probably stable.

About the Speaker: Sami Haddadin is the Director of the Munich Institute of Robotics and Machine Intelligence at the Technical University of Munich (TUM) and holds the Chair of Robotics and Systems Intelligence. His research interests include human-centered robotics, embodied AI, collective intelligence, and human-robot symbiosis. He is best known for his contributions to tactile mechatronics, contact-aware robots, safety methods in human-robot interaction, and autonomous manipulation learning. Before joining TUM, he was Chair of the Institute of Automatic Control at Gottfried Wilhelm Leibniz University Hannover from 2014 to 2018. Before that, he held various research positions at the German Aerospace Center DLR. He holds electrical engineering, computer science, and technology management degrees from the Technical University of Munich and the Ludwig Maximilian University of Munich. He received his PhD with summa cum laude from RWTH Aachen University and published more than 200 scientific articles in international journals and conferences, many of them award-winning. He has received numerous awards for his scientific work, including the George Giralt PhD Award (2012), the RSS Early Career Spotlight (2015), the IEEE/RAS Early Career Award (2015), the Alfried Krupp Award for Young Professors (2015), the German President’s Award for Innovation in Science and Technology (2017) and the Leibniz Prize (2019), Germany’s most important science award.
Enabling the Energy-based Autonomous Robotic Surgery
Speaker: Joseph Bentsman, University of Illinois at Urbana Champaign
Date: Thu, Sep 29
Abstract: We first recap the current approaches to robotic surgery and discuss the technology gap that exists in robot-assisted surgery (DaVinci system). We then state what is needed to develop fully-autonomous surgical robots, introduce energy-based surgery, with focus on electrosurgery, and argue why energy-based surgery might be more suited for autonomous systems. Then we loop- back to the need for smart autonomous reasoning and a predictive model, giving a short overview of mathematical modeling tasks. We then introduce sensors, and focus on the potential of thermal sensing with parameter estimation. We end the seminar with the discussion of the real-time robust adaptive control with fast adaptation to changes in the tissue, as well as unexpected behavior (e.g., bleeding.)

About the Speaker: Joseph Bentsman received the Ph.D. degree in electrical engineering from the Illinois Institute of Technology in 1984. He spent a year as a Lecturer and a Postdoctoral Research Fellow in the Department of Electrical Engineering and Computer Science, University of Michigan, Ann Arbor, MI. At present, he is Professor in the Departments of Mechanical Science and Engineering and Electrical and Computer Engineering, and in Carle-Illinois Medical School, University of Illinois at Urbana-Champaign. He is the author of two books, over 75 journals and over 150 conference papers in various areas of control theory and practice, and the recipient of several major awards. He led the NSF/Industry supported controller design effort in continuous casting that resulted in two key control systems currently in production at Nucor Steel and Clifford Cliffs. He is an ASME Fellow.
Wireless Communication Using Reconfigurable Intelligent Surfaces: Fundamentals, Challenges and Use-Cases
Speaker: Dr. Qurrat-Ul-Ain Nadeem, University of British Columbia, Canada
Date: Mon, Oct 2
Abstract: Programmable metasurface has emerged as a low-cost energy-efficient enabler of several potential next generation wireless communication technologies due to its ability to control the wavefront of the electromagnetic wave by imparting local gradient phase shifts. In this talk, we will cover two key applications of programmable metasurfaces: reconfigurable intelligent surface (RIS) assisted communication systems and stacked intelligent metasurface (SIM) enabled radio transceivers. RIS is essentially a programmable metasurface deployed in the propagation environment to assist the conventional radio transceivers in controlling the direction in which non-line-of-sight propagation paths are created through reflect beamforming. In this context, we will identify the good use cases of this technology from a communication performance viewpoint, and also present our results on a recently developed RIS prototype to support wide band operation. In the second half of the talk, we will introduce our results on the new SIM-enabled radio transceiver architecture that integrates multiple stacked layers of programmable metasurfaces with an antenna array employing a small number of radio frequency chains, as an efficient implementation of holographic MIMO communications systems. The transmit and receive antennas directly radiate and recover the data streams, with precoding and combining implemented in the native electromagnetic wave domain within the SIM layers at the speed of light, paving the way for an exciting paradigm shift from digital beamforming to fully wave-based beamforming.
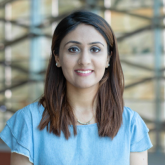
About the Speaker: Dr. Qurrat-Ul-Ain Nadeem received her M.S. and Ph.D. degrees in electrical engineering from King Abdullah University of Science and Technology (KAUST), Saudi Arabia in 2015 and 2019 respectively. She is currently a Postdoctoral Research and Teaching Fellow in the Electrical Engineering Department at the University of British Columbia (UBC), Canada. Her research focus is on the modeling, design and performance analysis of next-generation wireless communication systems, and her expertise lies in the areas of communication theory, information theory, signal processing, and electromagnetics and antenna theory. Dr. Nadeem was the recipient of the prestigious Paul Baron Young Scholar Award from The Marconi Society in 2018, and the Natural Sciences and Engineering Research Council of Canada (NSERC)’s Postdoctoral Fellowship Award in 2021. Dr Nadeem has served as the General Chair of the Workshop on Reconfigurable Intelligent and Holographic surfaces in IEEE PIMRC 2023 and serves as the General Chair of the Workshop on Integrated, Intelligent and Ubiquitous Connectivity for 6G in IEEE ICC 2024.
Digital Biodetection by Pixel-Diversity IRIS
Speaker: M. Selim Ünlü, Boston University
Date: Thu, Oct 5
Abstract: Interferometric Reflectance Imaging Sensor (IRIS) technology defies the conventional wisdom that calls for enhancing the signal through complex optical resonances. Instead, we exploit light interference from an optically transparent thin film—the same phenomenon that gives rainbow colors to a soap film when illuminated by white light. One of the most exciting applications of IRIS is nanoparticle counting or digital detection, that provides resolution and sensitivity beyond the reach of ensemble measurements. As a kinetic (real-time) assay with single-molecule readout, IRIS can measure analytes at attomolar concentrations. IRIS detection relies on interference of light reflected from the sensor surface being modified by the presence of particles producing a distinct signal that correlates to the polarizability (or size) of the particle. For smaller nanoparticles, discerning the optical signatures becomes particularly challenging with a single image capture. Thus, conventional IRIS utilizes z-scan acquisition (a stack of images taken from different focal positions) to capture the defocus signature unique to sub-diffraction limited scattering objects (nanoparticles on the surface). Recently, we demonstrated a new modality of digital detection, ‘pixel-diversity’ IRIS (PD-IRIS), that introduces a paradigm shift for encoding the necessary optical signature of target particles as it compresses the relevant optical information within a single image frame rather than an image stack. This is achieved by using camera sensors that simultaneously record multiple spectral or polarization channels. Therefore, a single image can record distinct spectral responses of target particles with respect to different excitation wavelengths (multi- spectral PD-IRIS) or the distinct scattering characteristics with respect to polarization (polarization PD-IRIS).
We will present experimental results on single image detection of spherical nanoparticles as well as gold nanorods. It is important to note that while polarization PD-IRIS is based on a straightforward mathematical formulation, spectral PD-IRIS is based on empirical observations due to chromatic aberrations that could vary for each instrument. Thus, we consider that Machine Learning Tools would be a powerful alternative method for nanoparticle detection in this case. With the practical implementation of single-molecule detection and counting, ‘digital detection – the new frontier in biomarker analysis’ will be accessible to all research laboratories.

About the Speaker: M. Selim Ünlü received the B.S. degree from the Middle East Technical University, Ankara, Turkey, in 1986, and the M.S.E.E. (1988) and Ph.D. (1992) degrees from the University of Illinois at Urbana-Champaign, all in electrical engineering. Since 1992, he has been a professor at Boston University. He is currently a Distinguished Professor of Engineering appointed in electrical and computer engineering, biomedical engineering, physics, materials science and engineering, and graduate medical sciences. His research interests are in the areas of nanophotonics and biophotonics focusing development of biological detection and imaging techniques, particularly in high-throughput digital biosensors based on detection of individual biological nanoparticles, viruses, and single molecule counting. Dr. Ünlü has authored and co-authored >200 journal articles with >13,000 citations (h-index of 59) and holds 22 US/international patents. He was the recipient of the NSF CAREER and ONR Young Investigator Awards in 1996. He has been selected as a Photonics Society Distinguished Lecturer for 2005-2007 and Australian Research Council Nanotechnology Network (ARCNN) Distinguished Lecturer for 2007. He is a fellow of IEEE, Optica, and AIMBE. He was awarded the Science Award (2008) by the Turkish Scientific Foundation. In 2021, he was selected as Boston University Innovator of the Year. His past professional service includes serving as the Editor-in-Chief for IEEE Journal of Quantum Electronics and General Chair of IEEE Photonics Society Annual Meeting.
Mathematical underpinnings of emerging computational imaging inverse problems
Speaker: Shirin Jalali, Rutgers University
Date: Tue, Oct 10
Abstract: Advanced computational imaging techniques have undergone a transformative evolution in modern imaging systems, surpassing the limitations of conventional approaches and unlocking diverse imaging capabilities. Developing efficient solutions for these imaging systems requires a comprehensive, multidimensional approach, involving mathematics, signal processing, machine learning, and proficient engineering. In this presentation, I will mainly focus on two specific categories of imaging systems: i) snapshot compressive imaging and ii) coherent imaging in the presence of speckle noise. By delving into these systems, I intend to underscore their distinctive challenges and the abundant opportunities they offer. Moreover, I will elucidate our advancements in these domains, with a primary focus on foundational mathematical models and algorithmic solutions grounded in theoretical principles. Additionally, a comprehensive examination of an array of challenges and open problems will be undertaken, emphasizing the essential strides required to fully actualize the potential of these innovative imaging systems.

About the Speaker: Shirin Jalali is an Assistant Professor at the ECE department at Rutgers University. Prior to joining Rutgers in 2022, she was a research scientist at the AI Lab at Nokia Bell Labs. She has also held positions as a Research Scholar at Princeton University and as a Faculty Fellow at NYU Tandon School of Engineering. She obtained her M.Sc. in Statistics and Ph.D. in Electrical Engineering from Stanford University. She has been serving as an Associate Editor of IEEE Transactions on Information Theory since 2021 and is a recipient of 2023 NSF CAREER award. Her research interests primarily lie in information theory, statistical signal processing, and machine learning. She applies these disciplines to tackle computational imaging inverse problems and explore the fundamental limits of structure learning.
Machine Learning and Side-Channel Analysis: Happily Ever After or Bitter Divorce?
Speaker: Aydin Aysu, North Carolina State
Date: Thu, Oct 12
Abstract: While machine learning helps automating and improving certain classification/regression tasks, side-channel analysis allows extracting secret information from systems that are mathematically secure. Although these were two fairly distinct research domains, they have recently been getting closer. Yet it is unclear how they would couple. In this talk, I will explain my research group's efforts in using machine learning for side channels, and side channels for machine learning. I will first demonstrate new side-channel attacks on machine-learning hardware that can steal valuable machine-learning models and methods for protection. Then, I will show the use of novel machine-learning techniques to outperform classical side-channel attacks and break cryptographic systems that cannot be broken with classical attacks.

About the Speaker: Dr. Aysu is currently an assistant professor and Bennett Faculty Fellow at the Electrical and Computer Engineering Department of North Carolina State University, where he leads HECTOR: Hardware Cybersecurity Research Lab. He got his M.S. from Sabanci University in Istanbul, Turkey, and his Ph.D. from Virginia Tech. Before joining NC State, he was a post-doctoral researcher at the University of Texas at Austin. Dr. Aysu's interests are broadly in hardware security research and cybersecurity education. His hardware security research has won NSF CAREER, NSF CRII, Google RSP, and Goodnight Innovation Fellow awards, six best paper nominations (IACR TCHES, IEEE HOST, DATE, GLS-VLSI), two best paper awards, one hardware security top picks (IEEE CEDA), and one publicity paper award (DAC). He is an IEEE senior member.
Random Neural Networks (RNN) for Accurate CyberAttack Detection and Mitigation at the Edge
Speaker: Erol Gelenbe, Academia Europaea
Date: Thu, Oct 12
Abstract: Even simple cyberattacks can impair the operation and performance of network systems substantially for many hours and sometimes days, and also increase the system's energy consumption. Their impact on data security, and the effects of the malware that they convey and install, are also well known. Thus there is a widespread need for accurate cyberattack detection, and rapid reaction and mitigation when attacks occur. On the other hand, the detection must avoid false alarms, to avoid impairing the smooth operation of a system which is not under attack. Thus considerable research has been conducted in this important field. Our presentation will briefly introduce the subject, and then focus on some recent results from the last 4-5 years, that are based on the Random Neural Network (RNN). The mathematical model will be described, and its extensions and deep learning algorithms will be discussed in the context of cyberattack detection and mitigation. The presentation will then focus on practical applications illustrated with different cyberattack datasets, showing the high accuracy and low false alarm rates that can be achieved. Measurements of active control schemes for attack mitigation will also be shown. Finally we will also show how the RNN can be used with Reinforcement Learning and SDN (Software Defined Networks), to dynamically control an Edge System that optimizes Security, QoS and Energy Consumption.
Note. The talk will be based on our publications in the following journals and conferences: Proceedings of the IEEE (2020), Sensors (2021, 2023), ACM SIGCOMM Flexnets (2021), ICC (2016, 2022), IEEE Access (2022, 2023), Performance Evaluation (2022.)
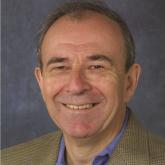
About the Speaker: Institute of Theoretical & Applied Informatics, Polish Academy of Sciences, & University Côte d'Azur I3S CNRS, 06100 Nice, France
Erol Gelenbe FIEEE FACM FIFIP FRSS received his MS and PhD degrees at Brooklyn Poly, and won the Tandon School Distinguished Alumnus Award in 2010. He has held named personal chairs at NJIT (USA), Duke University (USA), University of Central Florida (USA), Imperial College (UK), and full professorships at the University of Liege, University Paris Saclay, and University Paris Descartes. He served as Department Head at University Paris Descartes (1986-92), Duke University (1993-1998), and Director of the School of EECS at UCF (1993-1998). His research focuses on QoS, Security and Energy, and was funded by Industry, DoD and NSF in the USA, EPSRC and MoD in the UK, and numerous EU FP5, FP6, FP7, and Horizon 2020 projects since 2003. Currently Professor at the Institute of Theoretical & Applied Informatics, Polish Academy of Sciences, since 2017, he also cooperates with the CNRS I3S Laboratory of University Côte d'Azur (Nice, France), and Yasar University (Izmir, Turkey), and his work is now supported by grants from H2020 Horizon and UKRI. He is ranked among the top 25 PhD advisors in mathematical sciences by the American Mathematical Society Math. Genealogy Project and has graduated 24 women PhDs. Winner of the Grand Prix France Telecom 1996 (French Academy of Sciences), the ACM SIGMETRICS 2008 Life-Time Award, and the Mustafa Prize 2017, he was awarded the high honors of Commander of the Order of the Crown, Belgium (2022), Commander of the Order of Merit, France (2019), Knight of the Legion of Honour, France (2014), Commander of the Order of Merit, Italy (2005), Grand Officer of the Order of the the Star, Italy (2007). Fellow of the French National Academy of Engineering, and of the Science Academies of Belgium, Poland and Turkey, he is also Honorary Fellow of the Hungarian and Islamic Academy of Sciences. He currently chairs the Informatics Section of Academia Europaea.
Are Soft Actuators Powerful Enough to Revolutionize Robotic Interfaces?
Speaker: Ki-Uk Kyung, NYU Tandon School of Engineering, KAIST Visiting Professor
Date: Wed, Oct 18
Abstract: In recent years, the emergence of flexible technologies, including stretchable and bendable wearables, consumer electronics, and soft electromechanical devices like soft robots, has driven the demand for soft body elements and user interfaces in these systems to bolster their mechanical resilience against deformation. Electroactive polymers (EAPs) have surfaced as one of the most promising materials for implementing flexible actuation mechanisms due to their lightweight nature, inherent flexibility, freedom from geometric constraints, cost-effectiveness, and ease of miniaturization. Nonetheless, the primary drawback of these actuators lies in their inherent softness. To address this limitation, ongoing efforts are dedicated to enhancing the mechanical power of soft EAP actuators while preserving their advantageous characteristics. When configured as thin films, soft EAP actuators exhibit the potential to broaden their applications, particularly in the realm of tactile feedback interfaces for flexible touchscreens, Braille devices, and wearable tactile technology. This versatility positions them as prime candidates for wearable haptic devices. Furthermore, soft actuators have catalyzed a paradigm shift in artificial muscles, biomimetic robots, and miniature mechanical devices, transcending their role as tactile interfaces, thanks to substantial advancements in their output capabilities. One notable strength of soft components lies in their exceptional reconfigurability, exemplified by deformable fingers in robotic grippers, showcasing how soft components excel in handling objects of varying shapes. This discussion also delves into the existing challenges hindering the practical integration of soft actuators into innovative soft human-machine interfaces.
In summary, this exploration underscores the potential of soft actuators as transformative elements in robotic interfaces, shedding light on their evolution from mere tactile devices to powerful drivers of innovation in various fields.
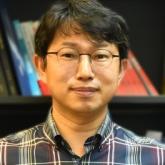
About the Speaker: Ki-Uk Kyung received BS, MS, and Ph.D. degrees in mechanical engineering from the Korea Advanced Institute of Science and Technology (KAIST) in 1999, 2001, and 2006, respectively. In 2006, he joined the Electronics and Telecommunications Research Institute and had been the Director of the Smart UI/UX Device Research Section. He had been a co-chair of the IEEE Technical Committee on Haptics (TCH) from 2018 to 2021. He received the IEEE TCH Early Career Award in 2015 and the Academic Career Award at Active Materials and Soft Mechatronics 2019. He is currently an associate professor of Mechanical Engineering and the director of the Human-Robot Interaction Research Center at KAIST. His research interests are soft sensors and actuators, haptics, soft robots, and human-robot interaction.
Machines that Talk to the Brain and Think Like the Mind
Speaker: Raghavendra (Raghav) Pothukuchi, Yale University
Date: Thu, Oct 19
Abstract: Communicating with the brain enables us to advance our understanding of brain function, treat disorders, restore lost function, and when combined with artificial cognitive frameworks, can push the frontier of human capabilities. Key to realizing brain communication and replicating cognition are new computer architectures– systems that directly sense and stimulate the brain, and those capable of running complex cognitive frameworks. In this talk, I will present recent work on the first brain-computer interfacing platform, SCALO, that can sense, process and stimulate neural activity from multiple regions of the brain with millisecond latency and milliwatts of power. SCALO flexibly supports many neuroscientific applications, enabling for the first time, study of brain-wide behavior and diseases. I will also share how new quantum and classical platforms are needed to accelerate cognitive neuroscience modeling, and outline an end to end design that connects such platforms with brain interfaces.

About the Speaker: Raghavendra (Raghav) Pothukuchi is an Associate Research Scientist at Yale University. He is an NSF/CRA Computing Innovation Fellow with Profs. Abhishek Bhattacharjee and Jonathan D. Cohen (Princeton, neuroscience). He received his Ph. D. in Computer Science (CS) from the University of Illinois at Urbana-Champaign (UIUC) with Prof. Josep Torrellas. His research is on brain-computer interfaces, quantum and classical frameworks to accelerate cognitive models, and biologically inspired computer architectures. He also has interdisciplinary work on building intelligent and secure computer systems using control theory and machine learning. Raghav has been selected as a young researcher at the Heidelberg Laureate Forum, rising star in computer architecture, and his work has been recognized with a best paper award at ISCA, an IEEE Micro Top Picks selection, best paper nomination at PACT, and other honors.
Modern AI Series: Generative AI for 3D Content
Speaker: Sanja Fidler, University of Toronto
Date: Tue, Oct 24
Abstract: 3D content is key in several domains such as architecture, film, gaming, robotics, and lies in the heart of the metaverse applications. However, creating 3D content can be very time consuming -- the artists need to sculpt high quality 3d assets, compose them into large worlds, and bring these worlds to life by writing behaviour models that "drive" the characters around in the world. For applications such as metaverse which feature extremely large 3D virtual worlds, A.I. that can help automate, scale up and democratize 3D content creation is existential. In this talk, I'll present some of our ongoing efforts on 3D Generative AI.
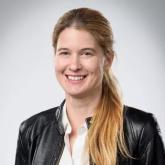
About the Speaker: Sanja Fidler is an Associate Professor at the University of Toronto, affiliated faculty at the Vector Institute (and one of the co-founding members), and VP of AI Research at NVIDIA, leading a research lab in Toronto. Prior to coming to Toronto, in 2014, she was a Research Assistant Professor at Toyota Technological Institute at Chicago, an academic institute located in the campus of University of Chicago. Her main research interests are in the intersection of computer vision, machine learning and graphics.
Safe and Efficient Learning for Power System Operations: An Interior Point Approach
Speaker: Baosen Zhang, University of Washington
Date: Tue, Oct 24
Abstract: To integrate the growing amount of renewable resources in the grid, power system operation problems are increasing in size and complexity. In recent years, machine learning or AI-based approaches have gained significant attention both in academia and industry. A main challenge in adopting these algorithms arises from the constraints in power system problems, which are hard limits that must be satisfied at all times. Tools like neural networks, can limit their outputs to be in simple regions, but there is no obvious way of forcing complex constraint satisfaction at the output. In this talk, we show a neural network architecture that guarantees constraint satisfaction by construction, without relying on steps like projections. By exploring the interior of the feasible set, we demonstrate improved efficiency and closed-loop performance for optimal power flow and model predictive control problems.

About the Speaker: Baosen Zhang is currently the Keith and Nancy Endowed Career Development Professor in Electrical and Computer Engineering at the University of Washington, Seattle, WA. He received his Bachelor of Applied Science in Engineering Science degree from the University of Toronto in 2008; and his PhD degree in Electrical Engineering and Computer Sciences from University of California, Berkeley in 2013. He was a Postdoctoral Scholar at Stanford University. His research interests are in control, optimization and learning applied to power and energy systems. He received the NSF CAREER award as well as several best paper awards.
Coded Matrix Computation: Numerical Stability, Partial Stragglers, and Sparse Input Matrices
Speaker: Aditya Ramamoorthy, Iowa State University
Date: Thu, Oct 26
Abstract: The overall execution time of distributed matrix computations is often dominated by slow or failed worker nodes (also known as stragglers). Recently, ideas from coding theory have been adapted to these problems; these allow for recovery of the intended result as long as a minimum number of worker nodes complete their assigned tasks.
In this talk, we will provide an overview of this area, and highlight some important issues with several prior works. These include numerical instability in the recovery of the desired result and ignoring work performed by slow workers (or partial stragglers). Furthermore, several prior schemes can cause an undesirable increase in the worker computation time when the input matrices are sparse. We will discuss some of our recent contributions in this area that address these issues.
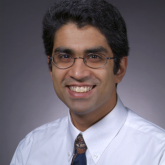
About the Speaker: Aditya Ramamoorthy is the Northrop Grumman Professor of Electrical and Computer Engineering and (by courtesy) of Mathematics at Iowa State University. He received his B. Tech. degree in Electrical Engineering from the Indian Institute of Technology, Delhi and the M.S. and Ph.D. degrees from the University of California, Los Angeles (UCLA). His research interests are in the areas of classical/quantum information theory and coding techniques with applications to distributed computation, content distribution networks and machine learning.
Dr. Ramamoorthy currently serves as an editor for the IEEE Transactions on Information Theory, and served a previous term from 2016 — 2019. In addition, he was an editor for the IEEE Transactions on Communications from 2011 — 2015. He is the recipient of the 2020 College of Engineering Mid-Career Achievement in Research Award, the 2019 Boast-Nilsson Educational Impact Award and the 2012 Early Career Engineering Faculty Research Award from Iowa State University, the 2012 NSF CAREER award, and the Harpole-Pentair professorship in 2009 and 2010. He is a senior member of the IEEE.
ECE SEMINAR: Rick Parimi, Alexis Networks, Inc
Speaker: Rick Parimi, Alexis Networks, Inc.
Date: Fri, Oct 27
Abstract: Alexis Networks, Inc. was created in 2019, initially with an objective to provide One-Click Anomaly Detection software solutions (“apps”) in the commercial sector. Successful track record includes projects with Aegis Foundry (PoS software maker), FINRA, CareCentrix (now part of Walgreens), and Insmed. The nature of projects involved – cloud services, software services and anomaly detection software. Currently, Alexis Networks, Inc. is pursuing Federal Contracts (biding and via GSA). Alexis Networks, Inc. has advanced its software solution specifically tailored for Insider Risk Analytics as a SaaS offering – using our proprietary MLN2 technology and a variety of open-source technologies. In this session, you can also learn about the nature of challenges that the company had to overcome – both from a technology perspective and business perspective. The session will also cover how Generative AI has begun to disrupt the domain of machine learning models and qualitative data analysis. You will also get to hear about the innovation from AlexisPi LCL (software solutions for universities.)
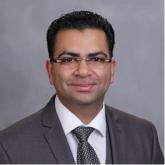
About the Speaker: Rick Parimi, Founder and CEO of AlexisPi LLC, is a renowned figure in the healthcare IT and technology sectors. His impressive academic journey includes a Harvard School of Public Health Fellowship, an Executive MBA from NYU's Stern School of Business, an MS in Computer Science from the University at Albany, and a BE in Computer Science from Amravati University, India. With a track record that boasts seven software patents with IBM, Rick's expertise transcends academia and has been instrumental in shaping the industry.
Throughout his career, Rick has made significant contributions, serving as a Board Member at the College of Emergency Preparedness, Homeland Security, and Cybersecurity at the University at Albany, and leading AlexisPi LLC. His professional journey also includes roles at Alexis Networks, Inc, private equity firms, the Mount Sinai Health System, Cognizant, IBM, and GE R&D. With an enduring passion for technology, Rick's dedication to innovation and leadership continues to redefine the healthcare IT landscape and inspire future generations.
Ingestible Electronics for Interfacing with the Gastrointestinal Tract
Speaker: Khalil Ramadi, New York University (NYU) Abu Dhabi, Assistant Professor of Biomedical Engineering
Date: Mon, Nov 6
Abstract: The gastrointestinal tract is a unique point of intersection of digestive, metabolic, nervous, and immune system. Interfacing with the GI tract is quite challenging, given the harsh, motile, and variable environments of the stomach and intestine. This talk will present a variety of engineering approaches for the design and manufacturing of ingestible devices that can successfully interface with GI tissue. We illustrate a variety of clinical applications for such devices, including 3-dimensional mapping of GI anatomy, assessment of GI motility, and modulation of neural and hormonal circuitry. Such ingestible electronic and electroceutical platforms could be a novel approach towards interrogating physiology, diagnosing disease, and delivering therapy non-invasively through the GI tract.

About the Speaker: Prof. Khalil Ramadi is an Assistant Professor of Bioengineering and Director of the Laboratory for Advanced Neuro-Engineering and Translational Medicine (LANTRN) at New York University (NYU) Abu Dhabi. His work focuses on developing new tools and technologies for treatment a variety of neurologic, endocrine, and immune disorders. Prof. Ramadi has been named a TED junior fellow, CIFAR Global Scholar, and MIT Technology Review Innovator Under 35 (MENA), and received multiple honors including the NIH F32 Ruth Kirschstein Postdoctoral Fellowship, BMES Career Development Award, and a NASA Aeronautics Scholarship. He holds a Ph.D. in Biomedical Engineering and Medical Physics from MIT, a M.S. in Mechanical Engineering from MIT, and B.S. degrees in Mechanical Engineering and Bioengineering from the Pennsylvania State University. He is also a board member and former co-Director of MIT Hacking Medicine, a group dedicated to enabling multi-disciplinary health entrepreneurship worldwide.
Research Challenges Facing Planning and Operation of Net-zero Power Systems
Speaker: Jovica V Milanović, University of Manchester, United Kingdom
Date: Mon, Nov 6
Abstract: Due to the evident climate change and environmental pressures the future power/energy systems will have to operate, sooner rather than later, in a net-zero environment. This will manifest in a mix of wide range of electricity generation, storage and demand technologies (increasingly power electronics interfaced); blurred boundaries between transmission and distribution system, significantly higher reliance on the use of legacy and measurement data including global signals for system identification, characterization and control and Information and Communication Technology embedded within the power system network and its components. The key characteristics of such a complex system would certainly be proliferation of power electronic devices in different shapes and forms and for different purposes, increased uncertainties in system operation and parameters and much larger reliance on the use of measurement and other data collected.
This presentation will first briefly introduce some of the key characteristics of future net-zero power systems and summarize the key challenges associated with operation, modeling and control of such systems. Following these examples of the latest research results in the areas of probabilistic stability studies of uncertain systems, data analytics, risk assessment and complex system analysis, all constituent parts of planning, operation and control of net-zero power systems, will be discussed and unanswered research questions summarized.

About the Speaker: Jovica V Milanović received Dipl.Ing. and M.Sc. degrees from the University of Belgrade, Yugoslavia, Ph.D. degree from the University of Newcastle, Australia, and D.Sc. degree from The University of Manchester, UK. Prior to joining The University of Manchester, UK, in 1998, he worked with “Energoproject”, Engineering and Consulting Co. and the University of Belgrade in Yugoslavia, and the Universities of Newcastle and Tasmania in Australia.
Professor Milanović is Head of Department of Electrical and Electronic Engineering at The University of Manchester, UK, Visiting Professor at the University of Novi Sad and the University of Belgrade, Serbia and a Honorary Professor at the University of Queensland, Australia. He was chairman of 6 international conferences, member of 9 (convenor of 3) past IEEE/CIGRE/CIRED WG, participated in or lead numerous research projects with total value of over £80 million, published over 600 research papers and reports, gave over 30 key-note speeches at international conferences and presented over 150 courses/tutorials and lectures to industry and academia around the world.
Professor Milanovic is a Chartered Engineer in the UK, Foreign member of the Serbian Academy of Engineering Sciences, Fellow of the IET, Fellow of the IEEE, Distinguished IEEE PES Lecturer, member of the IEEE PES Industry Technical Support Leadership Committee, member of the IEEE PES Long Range Planning Committee, member of IEEE Fellows Committee and Editor-in-Chief of IEEE Transactions on Power Systems. He was a member of the IEEE PES Governing Board as Regional Representative for Europe, Middle East and Africa for six years, member and vice-chair of IEEE PES Fellows Evaluation Committee and member and Chair of the IEEE Herman Halperin Transmission and Distribution Award Committee.
From Bits to Beyond: Crafting Secure and Scalable Memory Architectures for Next-Generation Systems
Speaker: Prashant Nair, The University of British Columbia, Vancouver Campus
Date: Fri, Nov 10
Abstract: Modern memory systems face scalability, security, and efficiency challenges as applications demand more from them. DRAM cells, the building blocks of memory systems, suffer from reliability issues that expose new attack vectors. One such attack is Row Hammer, which allows adversaries to flip the contents of DRAM cells by repeatedly accessing them. This compromises the security and scalability of memory systems. Moreover, different computing hardware, such as GPUs and CPUs, have different memory capacities, which affects the efficiency of memory systems.
This talk motivates the need for scalable, secure, and efficient memory systems. My presentation will dive deep into our latest HPCA 2023 paper (best paper) and discuss a novel attack called Juggernaut. Juggernaut breaks the state-of-the-art Row Hammer mitigation. My talk will discuss developing scalable and efficient solutions to mitigate Row Hammer. Going beyond security, I will briefly discuss our system-level proposal for enabling scalable memory systems (VLDB 2022) for large-scale ML models. I will conclude the talk by describing how research vectors in my research group, namely Quantum Computing, AI/ML memory system architectures, and security, are critical for developing next-generation systems.

About the Speaker: Prashant Nair is an Assistant Professor at the University of British Columbia (UBC). He is also an Affiliate Fellow at the Quantum Algorithms Institute. His primary interests are Computer Architecture, Quantum Systems, AI/ML Systems, Memory Systems, Reliability, and Security. He leads the "Systems and Architectures (STAR) Lab."
Dr. Nair has published over 25 papers in top-tier venues such as ISCA, MICRO, HPCA, ASPLOS, DSN, SC, and VLDB. He has received several awards, including the best paper award at HPCA 2023, two honourable mentions for IEEE MICRO Top-Picks, and the ECE Graduate Research Assistant Excellence Award for his Ph.D. at Georgia Tech. Before joining UBC, he worked at the T. J. Watson Research Center, developing practical data compression techniques for IBM systems. His work on integrating On-Die ECC and Host ECC (XED @ ISCA-2016) has been adopted by the HBM3 Memory Protocol by JEDEC.
Autonomous Robotic Systems in Aquaculture
Speaker: Dr. Eleni Kelasidi, Senior Research Scientist, Autonomous and Robotic Aquaculture Systems Lab
Date: Mon, Nov 13
Abstract: An increasing world population raises concerns over food security which, consequently, opens new avenues for food production. Agriculture, Fisheries, and Aquaculture are important global contributors to the production of food from land and sea for human consumption. In the last decades, the aquaculture industry has been the faster-growing food-producing sector in the world while also being known for substantially higher Health, Safety, and Environmental (HSE) risks, while still relying heavily on subjective and experience-based decisions and assessments, and intensive manual labor. Therefore, to target current and future challenges in aquaculture with the overall goal of improving efficiency, reducing risks and costs, and increasing production and sustainability, the industry has shown an increased interest in utilizing autonomy and robotic solutions in a variety of operations. Increasing the level of automation in high-risk operations within aquaculture would lead not only to economic but also social and ethical benefits. This talk will discuss the utilization of autonomous robotic solutions in the fish farming industry by presenting challenges, R&D solutions, and industrial applications in this sector. In addition, suggestions and guidelines on how autonomous operations in complex and dynamically changing environments can be realized by enabling underwater robots as a substitute. This is concluded with directions for future research and innovations needed to follow the currently emerging Precision Fish Farming (PFF) concept which outlines how innovative technologies and automation principles may be used to industrialize, digitize, and improve operations in fish farming.

About the Speaker: Dr. Eleni Kelasidi is a Senior Research Scientist and head of SINTEF ACE-RoboticLab - Autonomous and Robotic Aquaculture systems Lab in SINTEF Ocean leading activities on autonomous systems and robotic solutions applied to the aquaculture industry and targeting interdisciplinary research to link the field of technology and biology. Eleni Kelasidi received a Ph.D degree in Engineering Cybernetics from the Norwegian University of Science and Technology (NTNU) in 2015. In 2016, she was honored three-year VISTA (as a collaborative partnership between Statoil and the Norwegian Academy of Science and Letters) fellowship investigating Resident Robot Manipulators for Subsea IMR operations as a PostDoc Researcher (VISTA Scholar) at the CoE Centre for Autonomous Marine Operations and Systems, Department of Engineering Cybernetics at NTNU. She has acquired several projects targeting both fundamental knowledge and applied research and investigated problems towards the realization of autonomous robotic concepts in the aquaculture domain (e.g. Research Council of Norway: Project leader of 5 IPNs, 1 KSP, 1 FRIPRO-Young Researcher Talent, 3 RACE projects, and WP leader of two EU projects). Her research group’s work on robotic applications in the fish farming industry in SINTEF Ocean led to the development of the first robotic lab dedicated to the aquaculture domain.
A Promising Tale: Machine Learning for Neuroscience
Speaker: Zhe Sage Chen, New York University, Grossman School of Medicine
Date: Tue, Nov 14
Abstract: Advances in neurotechnology has allowed us to access large-scale amounts of neural and behavioral data. Statistical challenges remain how to leverage these BigData for understanding healthy and pathological brains and their links to behaviors, varying from episodic memory, decision making, pain, to neurological/psychiatric disorders. Machine learning and AI have played an increasingly important role in this endeavor for basic and clinical neuroscience research, including developing biologically realistic neural circuits for experimental predictions, extracting neural representations from multi-brain regions, reading out neural codes in brain-machine interfaces (BMI), and EEG data augmentation for biomarker discovery. I will outline some research efforts and showcase a few recent projects in my lab, followed by open discussions on new challenges and future research opportunities.
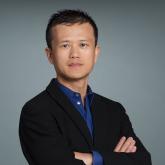
About the Speaker: Zhe Sage Chen is an associate professor (tenured) and principal investigator at New York University (NYU), with joint appointment at the Department of Psychiatry and Department of Neuroscience and Physiology at NYU Grossman School of Medicine, Department of Biomedical Engineering at NYU Tandon School of Engineering. He is the Founding Director of the CN^3 (Computational Neuroscience, Neuroengineering and Neuropsychiatry) Laboratory at NYU, and Director of the Computational Psychiatry program at NYU. The research in his lab covers a wide range of areas in computational neuroscience, neural engineering, machine learning, and brain-machine interfaces, studying fundamental research questions related to sleep and memory, nociception and pain, and cognitive control. His research and collaborative work has been published in high-impact journals such as Nature Biomedical Engineering, Science Translational Medicine, Neuron, Cell, Cell Reports, Nature Reviews Neuroscience, and Nature Communications. He is the lead author of the book Correlative Learning (Wiley, 2007) and the editor of books Advanced State Space Methods for Neural and Clinical Data (Cambridge University Press, 2015), Dynamic Neuroscience: Statistics, Modeling, and Control (Springer, 2018) and Memory and Sleep: A Computational Perspective (World Scientific, forthcoming). He has served in the editorial board and the role of associate editor for Neural Networks, Journal of Neural Engineering, Frontiers in Computational Neuroscience and IEEE Transactions on Neural Systems & Rehabilitation Engineering. He is the principal investigator for multiple research grants funded by the US National Science Foundation (NSF), National Institutes of Health (NIH), the BRAIN Initiatives, and the HEAL Initiatives.
AI-driven Automated Medical Image Analysis
Speaker: Dr. YingLi Tian, City College of New York (CCNY)
Date: Thu, Nov 16
Abstract: Medical imaging has been applied widely in many clinical diagnoses to detect and differentiate abnormalities. Manual analyzing medical images demands attention and is time-consuming, requiring well-trained expertise. The speed, fatigue, and experience may limit the diagnostic performance, leading to delays and even false diagnoses that significantly impact patient treatment. Therefore, developing AI-driven automated medical image analysis systems are crucial for timely clinical diagnosis. In this talk, I will share our research on two topics: 1) Contrast-enhanced medical images to highlight the internal structure of blood vessels and organs; 2) Cancer diagnosis with high sensitivity and specificity to detect, segment, and classify abnormality on medical images. The talk will be concluded with a discussion of potential future research trends.
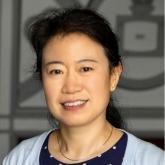
About the Speaker: Dr. YingLi Tian is a CUNY Distinguished Professor in Electrical Engineering Department at the City College of New York (CCNY) and Computer Science Department at Graduate Center of the City University of New York (CUNY). She is a Fellow of the Institute of Electrical and Electronics Engineers (IEEE), as well as a Fellow of International Association of Pattern Recognition (IAPR). She received her PhD from the Department of Electronic Engineering at the Chinese University of Hong Kong in 1996. Her research interests include computer vision, machine learning, artificial intelligence, assistive technology, medical imaging analysis, and remote sensing. She has published more than 240 peer-reviewed papers in journals and conferences in these areas with 24,000+ citations and holds 29 issued patents.
She is a pioneer in automatic facial expression analysis, human activity understanding, and assistive technology. Before joining CCNY in 2008, Dr. Tian was a research staff member at IBM T. J. Watson Research Center and led the video analytics team. She received the IBM Outstanding Innovation Achievement Award in 2007 and the IBM Invention Achievement Awards from 2002 to 2007. She serves as an associate editor for IEEE Trans. on Multimedia (TMM), Computer Vision and Image Understanding (CVIU), Journal of Visual Communication and Image Representation (JVCI), and Machine Vision and Applications (MVAP.)
QDA: Quantum Design Automation Toward Practical Quantum Computing
Speaker: Weiwen Jiang, Ph.D., Assistant Professor, George Mason University
Date: Wed, Dec 6
Abstract: Before the advent of Electronic Design Automation (EDA) in the mid-1970s, integrated circuits were meticulously crafted by hand, relying on manual layout processes. Similarly, the field of quantum computing is presently in its nascent stage, marked by the prevalent use of ad-hoc and manual-design approaches in quantum circuit design. This methodology, reminiscent of the early days of classical integrated circuits, presents a challenge to the evolution of practical quantum computing. In response to this challenge, there is a growing imperative for the development of a quantum analog to EDA --- Quantum Design Automation (QDA). This quantum-centric EDA aims to provide a systematic and automated framework for the design of quantum circuits, marking a crucial step towards overcoming the current impediments in the path to practical quantum computing.
The distinctions between classical bits and quantum qubits --- binary vs. superposition, independent vs. entanglement --- add complexity to QDA development. The inherent instability of quantum noise further compounds challenges. This talk undertakes a comprehensive comparison between classical and quantum computing design stacks, elucidating the intricacies introduced by qubit properties. Addressing the impact of unstable noise becomes central, providing insights into the necessities, obstacles, and opportunities in QDA. The talk outlines automated optimizations at various layers to tailor circuits to current noise, ultimately enhancing runtime fidelity. Finally, preliminary results employing quantum learning for real-world applications are presented, offering a glimpse into the potential advancements facilitated by QDA in the realm of practical quantum computing.

About the Speaker: Dr. Weiwen Jiang is an Assistant Professor in the ECE department at George Mason University. Prior to joining Mason, he served as a Post-Doctoral Researcher at the University of Notre Dame and as a research scholar at the University of Pittsburgh. During this period, Dr. Jiang focused on Electric Design Automation (EDA), earning acclaim with three Best Paper Awards in IEEE TCAD’21, ICCD’17, and NVMSA’15. He also received four Best Paper Nominations in ASP-DAC’16, DAC’19, CODES+ISSS’19, and ASP-DAC’20. Dr. Jiang's contributions extend beyond traditional academia, evidenced by his Top Winning Award at the IEEE Services Hackathon and two Best Demonstrations in IEEE/ACM University Demo.
In 2019, he embarked on a new research adventure in quantum computing. His initial work, published at Nature Communications, pioneered the co-design of quantum circuits and neural architectures. With the ultimate goal of practically using quantum computers for real-world applications, Dr. Jiang is actively involved in Quantum Design Automation, aiming to address interpretability and reproducibility challenges. His recent efforts in this field were recognized with a Best Paper Award at IEEE QuantumWeek’2023 and a Best Poster Award at ORNL’s Quantum Computing User Forum 2023. Dr. Jiang's research in this quantum line is currently supported by Industry, DoE, and NSF.
Mean Field Games approach to Systemic Risk
Speaker: Nizar Touzi, NYU Tandon School of Engineering, Chair of the FRE Department
Date: Thu, Dec 7
Abstract: Connections between economic agents are desirable for risk diversification purposes. However, they may also be responsible for default contagion, a major concern at the heart of the financial stability of the system. We develop a model of system connection based on the strategic interaction between economic agents. By considering the mean field limit, we provide a quasi explicit solution of the mean field game. This allows to provide an approximate Nash equilibrium for the finite population problem.

About the Speaker: Nizar Touzi is Professor at NYU Tandon School of Engineering, Chair of the FRE department. He was previously Professor at Ecole Polytechnique (France), Department of Applied Mathematics. His research expertise is on stochastic optimal control and differential games, with applications in finance and economics.
He holds a PhD from the University Paris Dauphine in January 1994, where he was appointed Assistant Professor. After a passage by University Pantheon Sorbonne, ENSAE as head of the finance and actuarial sciences lab, and Imperial College London, he served as Professor of applied mathematics and head of the financial mathematics group at Ecole Polytechnique from 2006 to 2023, where he took various responsibilities including chair of his department and head of the doctoral school in Mathematics from 2021 to 2023.
Nizar was invited to the International Congress of Mathematicians (Hyderabad 2010) as a session speaker and received the Louis Bachelier prize of the French Academy of Sciences in 2012 and the Paris Europlace prize of Best Young Researcher in Finance in 2007. He obtained many research grants, including the prestigious Advanced ERC grant. He is co-editor and associate editor in various international journals in the fields of financial mathematics, applied probability, and control theory.
Teaching Robots to Navigate, Grasp, and Dance
Speaker: Catie Cuan, Stanford University : Ken Goldberg, University of California, Berkeley
Date: Mon, Dec 18
About the Speakers: A researcher, entrepreneur, and artist, Dr. Catie Cuan is a pioneer in the nascent field of ‘choreorobotics’ and works at the intersection of artificial intelligence, human-robot interaction, and art. She is currently a Postdoc in Computer Science at Stanford University. Catie recently defended her PhD in robotics and AI via the Mechanical Engineering department at Stanford. Her PhD was funded by the National Institutes of Health, Google, and Stanford University. During her PhD, she led the first multi-robot machine learning project at Everyday Robots (Google X) and Robotics at Google (now a part of Google Deepmind). She has held artistic residencies at the Smithsonian, the Exploratorium, Jacob’s Pillow Dance Festival, Everyday Robots (Google X), TED, and ThoughtWorks Arts. www.catiecuan.com
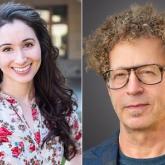
Ken Goldberg is William S. Floyd Jr Distinguished Chair in Engineering at UC Berkeley with appointments in IEOR, EECS, and Art Practice. He is co-founder and Chief Scientist of Ambi Robotics and Jacobi Robotics. He co-founded the Berkeley AI Research (BAIR) Lab and the
IEEE Transactions on Automation Science and Engineering. As Director of UC Berkeley’s AUTOLab, Ken and his students have published 400 peer-reviewed papers and 10 US patents. Ken's artwork has been exhibited internationally and in the 2000 Whitney Bienniel. He founded the Art, Technology, and Culture public lecture series in 1997 and has presented over 600 invited lectures worldwide: http://goldberg.berkeley.edu