Fall 2022 ECE Seminars
Date | Time | Speaker | From | Title | Recording |
---|---|---|---|---|---|
Wed, Sep 21 | 11am - 12pm | Robert Schapire | Microsoft Research | Modern AI Series: Convex Analysis at Infinity: An Introduction to Astral Space | |
Thu, Sep 22 | 11am - 12pm | Johannes Ballé | 5 Years of Learned Compression - What's Next? |
Unavailable |
|
Fri, Sep 23 | 12pm - 1pm | Yuval E. Yaish | Technion, Israel | Mode coupling, bi-stability, and spectral broadening in buckled carbon nanotube mechanical resonators | |
Fri, Sep 23 | 2pm - 3pm | Guillermo Gallego | Berlin Institute of Technology | ||
Thu, Oct 20 | 2:30pm - 3:30pm | Joerg Kliewer | New Jersey Institute of Technology |
Private Function Computation: Fundamental Limits and Practical Aspects |
|
Wed, Oct 26 | 11am - 12pm | John Langford | Microsoft | Modern AI Series: Discovering an agent's controllable latent state | |
Thu, Nov 10 | 11am - 12pm | Jacob Chakareski |
College of Computing, NJIT |
NextG Wireless Systems at the Nexus of ML, Mobile XR, and UAV-IoT |
|
Thu, Nov 17 | 11am - 12pm | Soumalya Sarkar | Raytheon Technologies Research Center (RTRC) |
Physics-informed AI-enhanced Engineering Design and Simulation Ecosystem |
|
Fri, Nov 18 | 2pm - 3pm | Ken Perlin | New York University | ||
Thu, Dec 1 | 11am - 12pm | Chris Wiggins | The New York Times | Modern AI Series: Data Science at The New York Times | |
Thu, Dec 6 | 11am - 12pm | Vasilis Maglaris | National Technical University of Athens | DDoS Attack Detection via Privacy-aware Federated Machine-Learning and Collaborative Mitigation in Multi-domain Cyber Infrastructures | |
Thu, Dec 15 | 11am - 12pm | Dr. Douglas A. Grose | The American Semiconductor Innovation Coalition (ASIC) | The American Semiconductor Innovation Coalition (ASIC) |
Unavailable |
Convex Analysis at Infinity: An Introduction to Astral Space
Speaker: Robert Schapire, Microsoft Research
Date: Wed, Sep 21
Abstract: Not all convex functions have finite minimizers; some can only be minimized by a sequence as it heads to infinity. In this work, we aim to develop a theory for understanding such minimizers at infinity. We study astral space, a compact extension of Euclidean space to which such points at infinity have been added. Astral space is constructed to be as small as possible while still ensuring that all linear functions can be continuously extended to the new space. Although not a vector space, nor even a metric space, astral space is nevertheless so well-structured as to allow useful and meaningful extensions of such concepts as convexity, conjugacy, and subdifferentials. We develop these concepts and analyze various properties of convex functions on astral space, including the detailed structure of their minimizers, exact characterizations of continuity, and convergence of descent algorithms.
This is joint work with Miroslav Dudík, Ziwei Ji, and Matus Telgarsky.

About the Speaker: Robert Schapire is a Partner Researcher at Microsoft Research in New York City. He received his PhD from MIT in 1991. After a short postdoc at Harvard, he joined the technical staff at AT&T Labs (formerly AT&T Bell Laboratories) in 1991. In 2002, he became a Professor of Computer Science at Princeton University. He joined Microsoft Research in 2014. His awards include the 1991 ACM Doctoral Dissertation Award, the 2003 Gödel Prize, and the 2004 Kanelakkis Theory and Practice Award (both of the last two with Yoav Freund). He is a fellow of the AAAI, and a member of both the National Academy of Engineering and the National Academy of Sciences. His main research interest is in theoretical and applied machine learning.
5 Years of Learned Compression - What's Next?
Speaker: Johannes Ballé, Google
Date: Thu, Sep 22
Abstract: Since its emergence about half a decade ago, the field of learned data compression has attracted considerable attention. Using machine learning in source coding promises faster innovation cycles, as well as better adaptation to novel data modalities and nonlinear distortion metrics. For example, image codecs can now be end-to-end optimized to perform best for specific types of images, by simply replacing the training set. They may be designed to minimize a given perceptual metric, or in fact any differentiable perceptual loss function, without the need to evaluate it during encoding. In this talk, I will first review the current state of learned data compression, and then discuss one of the next challenges in learned image compression: developing better models of human perception, and improving realism of reconstructed images.

About the Speaker: Johannes Ballé is a Staff Research Scientist at Google. They defended their master's and doctoral theses on signal processing and image compression under the supervision of Jens-Rainer Ohm at RWTH Aachen University in 2007 and 2012, respectively. This was followed by a brief collaboration with Javier Portilla at CSIC in Madrid, Spain, and a postdoctoral fellowship at New York University’s Center for Neural Science with Eero P. Simoncelli to study the relationship between perception and image statistics. While there, they pioneered the use of variational Bayesian models and deep learning techniques for end-to-end optimized image compression. They joined Google in 2017 to continue working in this line of research. Johannes has served as a reviewer for top-tier publications in both machine learning and image processing, such as NeurIPS, ICLR, ICML, Picture Coding Symposium, and several IEEE Transactions journals. They have been a co-organizer of the annual Workshop and Challenge on Learned Image Compression (CLIC) since 2018.
Mode coupling, bi-stability, and spectral broadening in buckled carbon nanotube mechanical resonators
Speaker: Yuval E. Yaish, Technion, Israel
Date: Fri, Sep 23
Abstract: Bi-stable mechanical resonators play a significant role in various applications, such as sensors, memory elements, quantum computing and mechanical parametric amplification. While carbon nanotube (CNT) based resonators have been widely investigated as promising NEMS devices, a bi-stable CNT resonator has never been demonstrated. In this seminar we present a new class of CNT resonators in which the nanotube is buckled upward. We show that a small upward buckling yields record electrical frequency tunability, whereas larger buckling can achieve Euler-Bernoulli (EB) bi-stability, the smallest mechanical resonator with two stable configurations to date. Furthermore, we present a three-dimensional theoretical analysis revealing significant nonlinear coupling between the in-plane and out-of-plane static and dynamic modes of motion, and a unique three-dimensional EB snap-through transition. We utilize this coupling to provide a conclusive explanation for the low-quality factor in CNT resonators at room temperature, key in understanding dissipation mechanisms at the nano scale.

About the Speaker: Dr. Yaish is faculty at the ECE and the Zisapel Nano-Electronics Center at the Technion – Israel Institute of Technology. He earned his B.Sc. and M.Sc. in Physics from Tel-Aviv University and received his Ph.D. in Experimental Physics in Condensed Matter from the Technion. Dr. Yaish did his postdoc in Physics at Cornell University, and since then his research is focused on electrical, thermal, and mechanical properties of 1- and 2-dimensional nano scale materials such as Silicon Nanowires, Carbon Nanotubes, Graphene, and 2-dimensional van der Waals materials.
Event cameras, their working principle and applications
Speaker: Guillermo Gallego, Berlin Institute of Technology
Date: Fri, Sep 23
Abstract: Event cameras, also called neuromorphic cameras or silicon retinas, are novel vision sensors that mimic functions from the human retina and offer potential advantages over traditional cameras (low latency, high speed, high dynamic range, bandwidth savings, low power, etc.). This talk will provide an overview of how event-based cameras work and the computer vision and robotics applications where they are being explored (monitoring, tracking, motion estimation, recognition, etc.). I will also present recent advances from the Robotic Interactive Perception Lab at TU Berlin on processing event data in specific topics of interest (optical flow estimation, 3D reconstruction, animal monitoring, etc.) as the discussion unfolds.

About the Speaker: Guillermo Gallego is Associate Professor at TU Berlin and the Einstein Center Digital Future, Berlin, Germany. He is also a PI of the Science of Intelligence Excellence Cluster. He received the PhD degree in Electrical and Computer Engineering from the Georgia Institute of Technology, USA, in 2011. From 2011 to 2014 he was a Marie Curie researcher with Universidad Politecnica de Madrid, Spain, and from 2014 to 2019 he was a postdoctoral researcher with the Robotics and Perception Group at the University of Zurich, Switzerland. He serves as Associate Editor for IEEE Transactions on Pattern Analysis and Machine Intelligence and IEEE Robotics and Automation Letters.
Private Function Computation: Fundamental Limits and Practical Aspects
Speaker: Joerg Kliewer, New Jersey Institute of Technology
Date: Thu, Oct 20
Abstract: We consider the problem of private function computation, where a user wishes to compute a function of f messages stored in n non-colluding databases. The goal is to provide a scheme which minimizes the download cost and is private at the same time, i.e., it should not reveal any information about the computation result to the databases. We first employ the computation of a linear function of the messages, where linear codes are used to encode the information on the databases. We show that the private linear computation capacity, which is the ratio of the desired linear function size and the total amount of downloaded information, matches the maximum distance separable coded capacity of private information retrieval for a large class of linear codes. Our capacity expression depends on the rank of the coefficient matrix obtained from all linear combinations. We also present how this approach can be extended to computing arbitrary multivariate polynomials of the messages. Here, the presented schemes yield improved download rates compared to the best known schemes from the literature. Finally, in an attempt to make private information retrieval more practical we propose to relax the privacy and perfect reconstruction requirements. For scenarios where the data statistics is unknown, we propose a new deep learning framework based on generative adversarial networks, which allows to learn schemes minimizing the download cost from the data itself.

About the Speaker: Joerg Kliewer received the Dr. Ing. degree (Ph.D.) in electrical engineering from the University of Kiel, Germany, in 1999. He held several postdoc positions from 2000-2006. From 2007 to 2013, he was with New Mexico State University, Las Cruces, NM, USA, most recently as an associate professor. He is currently with the New Jersey Institute of Technology, Newark, NJ, USA, as a professor. His research interests span information theory, security and privacy, machine learning, graphical models, and statistical algorithms. He has been a member of the editorial board of the IEEE Information Theory Society Newsletter since 2014. He is a recipient of a Leverhulme Trust Award, a German Research Foundation Fellowship Award, an IEEE Globecom Best Paper Award, and a Fulbright Scholarship. He was an associate editor of the IEEE Transactions on Communications from 2008 to 2014 and an area editor for the same journal from 2015 to 2021. He has also been an editor for the IEEE Transactions on Information Theory from 2017 to 2020. Since 2021 he has been serving as editor for the IEEE Transactions on Information Forensics & Security.
Discovering an agent's controllable latent state
Speaker: John Langford, Microsoft
Date: Wed, Oct 26
Abstract: Given rich sensors (like cameras), how can you learn what an agent can do? This is challenging in highly stateful environments where accomplishing tasks requires complex plans, since randomly exploring may never discover some capabilities of the agent. It turns out however that some of the core principles of discovering controllable latent state are straightforward, enabling us to discover the controllable latent state after a few thousand steps even in environments where random exploration is not viable. Furthermore, this forms a good foundational representation for optimizing reward functions.

About the Speaker: John Langford studied Physics and Computer Science at the California Institute of Technology, earning a double bachelor’s degree in 1997, and received his Ph.D. from Carnegie Mellon University in 2002. Since then, he has worked at Yahoo!, Toyota Technological Institute, and IBM‘s Watson Research Center. He is also the primary author of the popular Machine Learning weblog, hunch.net and the principle developer of Vowpal Wabbit. Previous research projects include Isomap, Captcha, Learning Reductions, Cover Trees, and Contextual Bandit learning.
NextG Wireless Systems at the Nexus of ML, Mobile XR, and UAV-IoT
Speaker: Jacob Chakareski, College of Computing, NJIT
Date: Thu, Nov 10
Abstract: The talk reflects the recent paradigm shift in wireless networks research from the traditional objective of enabling ever higher transmission rates at the physical layer to enabling for the network system higher resilience to attacks, higher robustness to system components’ failures, closer vertical integration with key emerging applications and their quality of experience needs, and intelligent self-coordination. The talk will comprise three stories of related recent research (the number three is good J). I will first talk about multi-connectivity enabled NextG wireless multi-user VR systems. Then, I will outline our advances in domain-aware fast RL for IoT systems. Third, I will talk about enabling real-time human AR streaming in NextG classrooms featuring real and virtual participants. The presentation of each of these studies will comprise a brief outline of the overall NSF project in which they are embedded. Next, I will highlight an interdisciplinary NIH R01 study I lead at the nexus of VR and AI aimed at addressing the societal need of low-vision rehabilitation. Finally, I will highlight prospective collaboration avenues with NYU ECE faculty and students, and leave the floor open for questions and discussions.

About the Speaker: Jacob Chakareski is an associate professor in the College of Computing at NJIT, where he holds the Panasonic Chair of Sustainability and directs the Lab for AI-Enabled Wireless XR Network Systems and Societal Applications. He completed his PhD degree in electrical and computer engineering at Rice University and Stanford University. Dr. Chakareski organized the first National Science Foundation (NSF) visioning workshop on future XR communications and network systems in 2018. His research interests include NextG wireless XR systems, UAV-IoT sensing and networking, domain-aware fast reinforcement (RL) learning, deep RL for AI-enabled edge networks, 5G edge computing/caching, optical and millimeter wave wireless networking, multi-connectivity-enabled scalable 360° streaming, and societal applications. He has authored over 200 publications (books, book chapters, and IEEE/ACM journal and conference papers), holds 8 US patents that have been integrated into real tech products used widely today, and his Google Scholar -index is 36. Chakareski is a recipient of the Adobe Data Science Faculty Research Award in 2017 and 2018, the Swiss NSF Career Award Ambizione (2009), the AFOSR Faculty Fellowship in 2016 and 2017, and best paper awards at ICC 2017 and MMSys 2021. He has held research appointments with Microsoft, HP Labs, and EPFL, and served on the advisory board of Frame, Inc. (acquired in 2019 by Nutanix, Inc.). His research has been generously supported by the NSF, NIH, AFOSR, Adobe, Tencent Research, NVIDIA, Intel, and Microsoft. For further information, please visit www.jakov.org.
Physics-informed AI-enhanced Engineering Design and Simulation Ecosystem
Speaker: Soumalya Sarkar, Raytheon Technologies Research Center (RTRC)
Date: Thu, Nov 17
Abstract: Aerospace and defense industries are actively developing Artificial Intelligence (AI) solutions to fundamentally transform their design, simulation, and development processes to achieve better productivity, energy savings and safety standards. AI is making impacts in industries involving speech and natural language understanding, e-commerce, social networks, gaming, medicine, and robotics, but it has yet to demonstrate its full potential in revolutionizing the development of core engineering and manufacturing industries. The key challenges include long design cycles, siloed and manual design across scales, lack of learning from noisy heterogeneous knowledge sources, and large design and processing space of safety-critical systems. Recently, researchers have been applying off-the-shelf AI/ML tools to overcome some of these challenges via surrogate-based design optimization, multi-fidelity learning and physics-constrained emulators. Still, large-scale engineering and simulation design problems with AI become prohibitively expensive and non-deployable due to challenges such as, (i) lack of interpretability and trust on black-box ML-surrogate models, (ii) high computational overhead for high-fidelity solvers (ii) enterprise-level heterogeneity of model/software, (iii) non-differentiability and lack of model visibility and compatibility, (iv) data scarcity, (v) multiple data sources with different uncertainties. This talk will discuss a novel multi-X AI (X = source, scale and/or fidelity) ecosystem developed by RTRC, which is built upon the concepts of physics-informed and interpretable ML, multi-fidelity leaning, data-efficient surrogate modeling, Cost-aware blackbox optimization, and non-intrusive physics incorporation into learning. The ecosystem has demonstrated 2-10X speed-up and better solutions in diverse domains including rapid materials discovery, gas turbine engine design, digital twin construction, simulation calibration, optimal scenario generation, micro/power electronics systems design, manufacturing process optimization and in-situ monitoring.

About the Speaker: Dr. Soumalya Sarkar, Sr. Principal Scientist of the AI Discipline in Raytheon Technologies Research Center (RTRC), has 7 years of experience in Scientific Machine Learning, Physics-informed AI, Deep Learning, NLP and Knowledge graph, Black-box optimization, Multi-modal Sensor Fusion. He serves as the PI/co-PI and ML task lead for government (ARPA-E DIFFERENTIATE, DARPA, and DOE) and corporate-funded research programs in the areas of physics-informed AI, AI-guided engineering simulation, design, materials discovery. He has co-authored 55 peer-reviewed publications including 20 Journal papers, 2 book chapters and 10 US patent applications and 5 awarded patents. Dr. Sarkar has received numerous awards including the 2021 “Technical Excellence Award (TEA)" (highest individual technical award at RTRC), two annual “Outstanding Achievement Award (OAA)" from RTRC in 2018 and 2022, and invitation as one of the 100 early-career engineers/faculties from US industry, universities, and national labs to attend National Academy of Engineering’s (NAE) 2020 US Frontiers of Engineering symposium. He has received PhD on data-driven learning of complex systems and double masters in Math and ME from the Pennsylvania State University.
Jack Wolf Series: The Future of Experiential Computing
Speaker: Ken Perlin, New York University
Date: Fri, Nov 18
Abstract: New kinds of media can change how we interact with each other in unexpected and sometimes radical ways. The World Wide Web led to an unprecedented democratization of knowledge. Smartphones led to vast changes in everything from how we shop to how we socialize to how we travel. In another decade, the combination of ubiquitous smart glasses and 6G wireless connectivity will fundamentally alter everything from how children learn to how work is conducted to the meaning of shared public spaces. It is hard to fully anticipate the impact of such a profound change, but we can make a few predictions.
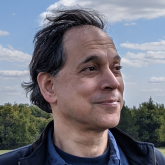
About the Speaker: Ken Perlin, a professor in the Department of Computer Science at New York University, directs the Future Reality Lab, and is a participating faculty member at NYU MAGNET. His research interests include future reality, computer graphics and animation, user interfaces and education. He is chief scientist at Parallux and Tactonic Technologies. He is an advisor for High Fidelity and a Fellow of the National Academy of Inventors. He received an Academy Award for Technical Achievement from the Academy of Motion Picture Arts and Sciences for his noise and turbulence procedural texturing techniques, which are widely used in feature films and television, as well as membership in the ACM/SIGGRAPH Academy, the 2020 New York Visual Effects Society Empire Award the 2008 ACM/SIGGRAPH Computer Graphics Achievement Award, the TrapCode award for achievement in computer graphics research, the NYC Mayor's award for excellence in Science and Technology and the Sokol award for outstanding Science faculty at NYU, and a Presidential Young Investigator Award from the National Science Foundation. He serves on the Advisory Board for the Centre for Digital Media at GNWC. Previously he served on the program committee of the AAAS, was external examiner for the Interactive Digital Media program at Trinity College, general chair of the UIST2010 conference, directed the NYU Center for Advanced Technology and Games for Learning Institute, and has been a featured artist at the Whitney Museum of American Art. He received his Ph.D. in Computer Science from NYU, and a B.A. in theoretical mathematics from Harvard. Before working at NYU he was Head of Software Development at R/GREENBERG Associates in New York, NY. Prior to that he was the System Architect for computer generated animation at MAGI, where he worked on TRON.
Data Science at The New York Times
Speaker: Chris Wiggins, New York University
Date: Thu, Dec 1
Abstract: The Data Science group at The New York Times develops and deploys machine learning solutions to newsroom and business problems. Re-framing real-world questions as machine learning tasks requires not only adapting and extending models and algorithms to new or special cases but also sufficient breadth to know the right method for the right challenge. I'll first outline how - unsupervised, - supervised, and - reinforcement learning methods are increasingly used in human applications for - description, - prediction, and - prescription, respectively.
I'll then focus on the 'prescriptive' cases, showing how methods from the reinforcement learning and causal inference literatures can be of direct impact in - engineering, - business, and - decision-making more generally.

About the Speaker: Chris Wiggins is an associate professor of applied mathematics at Columbia University and the Chief Data Scientist at The New York Times. At Columbia, he is a founding member of the executive committee of the Data Science Institute, and of the Department of Applied Physics and Applied Mathematics as well as the Department of Systems Biology, and is affiliated faculty in Statistics. He is a co-founder and co-organizer of hackNY, a nonprofit that since 2010 has organized once-a-semester student hackathons and the hackNY Fellows Program, a structured summer internship at NYC startups.
Prior to joining the faculty at Columbia he was a Courant Instructor at NYU (1998-2001) and earned his Ph.D. at Princeton University (1993-1998) in theoretical physics. He is a Fellow of the American Physical Society and is a recipient of Columbia's Avanessians Diversity Award.
Books:
"Data Science in Context: Foundations, Challenges, Opportunities" (Cambridge Press 2022)
"How Data Happened: A History from the Age of Reason to the Age of Algorithms" (Norton Press 2023)
DDoS Attack Detection via Privacy-aware Federated Machine-Learning and Collaborative Mitigation in Multi-domain Cyber Infrastructures
Speaker: Vasilis Maglaris, National Technical University of Athens
Date: Thu, Dec 6
Abstract: Interconnected cyber infrastructure, accessible via the Internet, are a common target of DDoS attacks intending to downgrade their operations and services. Collaborative protection mechanisms are prime candidates to defend against massive attacks but, although collaborations were instrumental in the Internet success story, this is largely not extended to multidomain cyber security. Notably, collaborative DDoS detection is hindered by data privacy legislations, while mitigation is limited to operations of stand-alone rigid firewalls. Motivated by these shortcomings, we propose a Federated Learning schema for collaborative privacy-aware DDoS detection. Coordination is orchestrated by a third trusted party that aggregates machine learning models proposed by collaborators based on their private attack and benign traces, without exchanging sensitive data. Attacks detected via the privacy-aware federated model are subsequently mitigated by efficient and scalable firewalls, implemented within the eXpress Data Path (XDP) data plane programmability framework. Our approach was evaluated using production traffic traces in terms of packet classification accuracy and packet processing performance. We conclude that our proposed Federated Learning framework enabled collaborators to accurately classify benign and attack packets, thereby improving individual domain accuracy. Furthermore, our data plane programmable firewalls promptly mitigated large-scale attacks in emulated federated cyber infrastructures.
Index Terms—Federated Machine Learning, Federated Clouds, Multi-domain DDoS Protection, Programmable Data Planes, eXpress Data Path (XDP)

About the Speaker: Professor Vasilis Maglaris holds an Engineering Degree from the National Technical University of Athens – NTUA (Athens, Greece, 1974), an M.Sc. from Polytechnic University (Brooklyn NY, 1975) and a Ph.D. degree from Columbia University (New York, USA, 1979). Between 1979 and 1989 he held industrial and academic positions in the USA, all in advanced electronic communications. From 1981 to 1990 he was with the faculty of EE/CS of Polytechnic University - now NYU Tandon/Poly - Brooklyn NY. From 1990 to 2019 he was with the faculty of the School of Electrical & Computer Engineering at NTUA teaching and performing research on Internet technologies, directing the Network Management & Optimal Design (NETMODE) Laboratory that he established. He was responsible for the development of the NTUA Campus LAN and of GRNET, the National Research & Education Network (NREN) of Greece. From 2004 to 2012 he was the GÉANT Policy Committee Chairman, the governance body of the advanced Internet serving the 37 NRENs of the extended European Research Area. From July 2012 to June 2013 he served as General Secretary for Research & Technology appointed by the Greek coalition Government, on hold from his duties at NTUA. In 2020 the NTUA Senate conferred upon him the title of Professor Emeritus, enabling him to continue his teaching and research activities beyond his retirement in 2019. His current research interest focus on attack protection of cyber-infrastructures via machine learning algorithms, possibly leveraging on data-plane technologies.
The American Semiconductor Innovation Coalition (ASIC)
Speaker: Dr. Douglas A. Grose, The American Semiconductor Innovation Coalition (ASIC)
Date: Thu, Dec 15
Abstract: The CHIPS and Science Act, signed into law by President Biden August 9, 2022, includes significant funding for a once in a generation opportunity to strengthen the U.S. microelectronics ecosystem that is facing significant global competition.
The CHIPS and Science Act provides funding for the Department of Commerce/NIST to invest $11 billion over 5 years in semiconductor R&D, including a National Semiconductor Technology Center (NSTC) and a National Advance Packaging Manufacturing Program (NAPMP). Included in the CHIPS and Science Act is $39 billion financial support for domestic semiconductor manufacturing,
The American Semiconductor Innovation Coalition (ASIC), represents more than 160 businesses, start-ups, universities, national labs, non-profits dedicated to bringing the best research and development to the National Semiconductor Technology Center (NSTC) and the National Advanced Packaging Manufacturing Program (NAPMP).
Dr. Doug Grose, representing ASIC, will discuss the ASIC vision for the NSTC and NAPMP.
Index Terms—Federated Machine Learning, Federated Clouds, Multi-domain DDoS Protection, Programmable Data Planes, eXpress Data Path (XDP)

About the Speaker: Douglas Grose is a technologist who built his early career at IBM for over 20 years.
From 2006 through 2009, he was the Senior Vice President of Technology Development, Manufacturing and Supply Chain at Advanced Micro Devices (AMD).
Dr. Grose helped direct the formation of GLOBALFOUNDRIES. He was the CEO of GLOBALFOUNDRIES from 2009-2011.
Dr. Grose was appointed President of NY CREATES in 2019 and transitioned to Chair of the Board of NY CREATES in 2021.
In semi-retirement, Dr. Grose is supporting the American Semiconductor Innovation Coalition (ASIC), guiding a response for the CHIPS and Science Act, National Semiconductor Technology Center (NSTC) and National Advanced Packaging Manufacturing Program (NAPMP).
Dr. Grose graduated from Rensselaer Polytechnic Institute with an MBA and a BS, MS and PhD in Materials Engineering.