Fall 2021 Seminars
A complete listing
Date | Time | Speaker | From | Title | Recording |
---|---|---|---|---|---|
Thur, Sept 23 | 1pm - 2pm | Michael Friedlander | University of British Columbia, Canada | Modern AI Series: Geometric Duality in Optimization | |
Thur, Oct 7 | 11am - 12pm | Sanghamitra Dutta | JPMorgan Chase | Fair and Reliable Machine Learning for High-Stakes Applications: Approaches Using Information Theory | |
Thur, Oct 21 | 11am - 12pm | Karl Friston | University College London, UK | Modern AI Series: Deep Inference | |
Thur, Oct 28 | 11am - 12pm | Panayiotis (Panos) Moutis | Carnegie Mellon University | Modern AI Series: Active Distribution Networks: Clean, robust, self-controlled and profitable | |
Fri, Oct 29 | 2pm - 3pm | Deji Akinwande | University of Texas, Austin | Novel Applications of 2D Materials from Wearable Health to Memory Devices and 5G Switches | |
Thur, Nov 4 | 11am - 12pm | Viveck R. Cadambe | Pennsylvania State University | Resilient and secure distributed computing and learning via coded computing | |
Thur, Nov 18 | 11am - 12pm | Jacob Paul Mays | Cornell University | - | |
Tue, Nov 30 | 10am - 11am | William Rosenbluth, Peter J Sullivan | Automotive Systems Analysis, Inc | Forensic Analysis of Vehicular Malfunction Using On-Board Data | |
Thur, Dec 2 | 11am - 12pm | Fay Cobb Payton | North Carolina State University | Coding, Coded & Counting: A Bias Continuum | |
Thur, Dec 16 | 11am - 12pm | Osvaldo Simeone | King's College London, UK | Free Energy Minimization Learning: Information-Theoretic Fundamentals and Applications |
Geometric Duality in Optimization
Speaker: Michael P. Friedlander, University of British Columbia, Canada
Date: Sept 23
Abstract: Convex duality flows throughout optimization, its algorithms, and its connections to efficient computation. I will describe a fundamental and intuitive form of geometric duality based on convex cones, which provides a lens through which to interpret an important class of algorithms used in statistical learning theory. I'll demonstrate a Julia software package that implements a calculus based on these ideas.
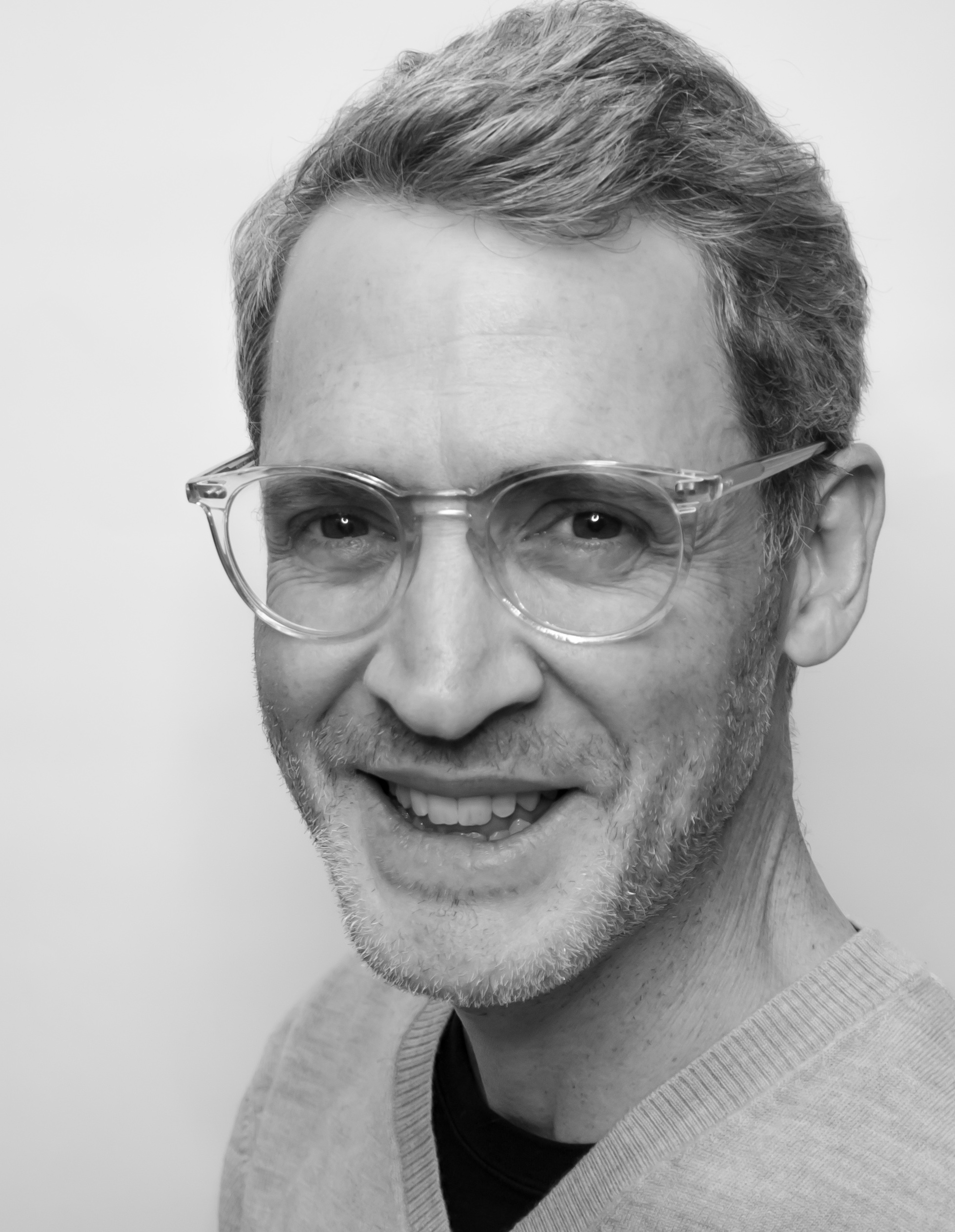
About the Speaker: Michael Friedlander is IBM Professor of Computational Mathematics at the University of British Columbia. He was Professor of Mathematics at UC Davis (2014--2016), and has held visiting positions at Berkeley's Simons Institute for the Theory of Computing (2013), and at UCLA's Institute for Pure and Applied Mathematics (2010). From 2002 to 2004 he was the Wilkinson Fellow in Scientific Computing at Argonne National Laboratory. He received his PhD in Operations Research from Stanford University in 2002 under the supervision of Michael A. Saunders. He received his BA in Physics from Cornell University in 1993. He currently serves as Area Editor for Continuous Optimization for the journal Mathematics of Operations Research. His research is primarily in developing numerical methods for large-scale optimization, their software implementation, and applying these to problems in statistical learning.
Fair and Reliable Machine Learning for High-Stakes Applications: Approaches Using Information Theory
Speaker: Sanghamitra Dutta, JP Morgan Chase AI Research
Date: Oct 7
Place: 370 Jay, Room 825
Abstract: How do we make machine learning (ML) algorithms fair and reliable? This is particularly important today as ML enters high-stakes applications such as hiring and education, often adversely affecting people’s lives with respect to gender, race, etc., and also violating anti-discrimination laws. When it comes to resolving legal disputes or even informing policies and interventions, only identifying bias/disparity in a model’s decision is insufficient. We really need to dig deeper into how it arose. E.g., disparities in hiring that can be explained by an occupational necessity (code-writing skills for software engineering) may be exempt by law, but the disparity arising due to an aptitude test may not be (Ref: Griggs v. Duke Power ‘71). This leads us to a question that bridges the fields of fairness, explainability, and law: How can we identify and explain the sources of disparity in ML models, e.g., did the disparity entirely arise due to the critical occupational necessities? In this talk, I propose the first systematic measure of "non-exempt disparity," i.e., the bias which cannot be explained by the occupational necessities. To arrive at a measure for the non-exempt disparity, I adopt a rigorous axiomatic approach that brings together concepts in information theory (in particular, an emerging body of work called Partial Information Decomposition) with causality.
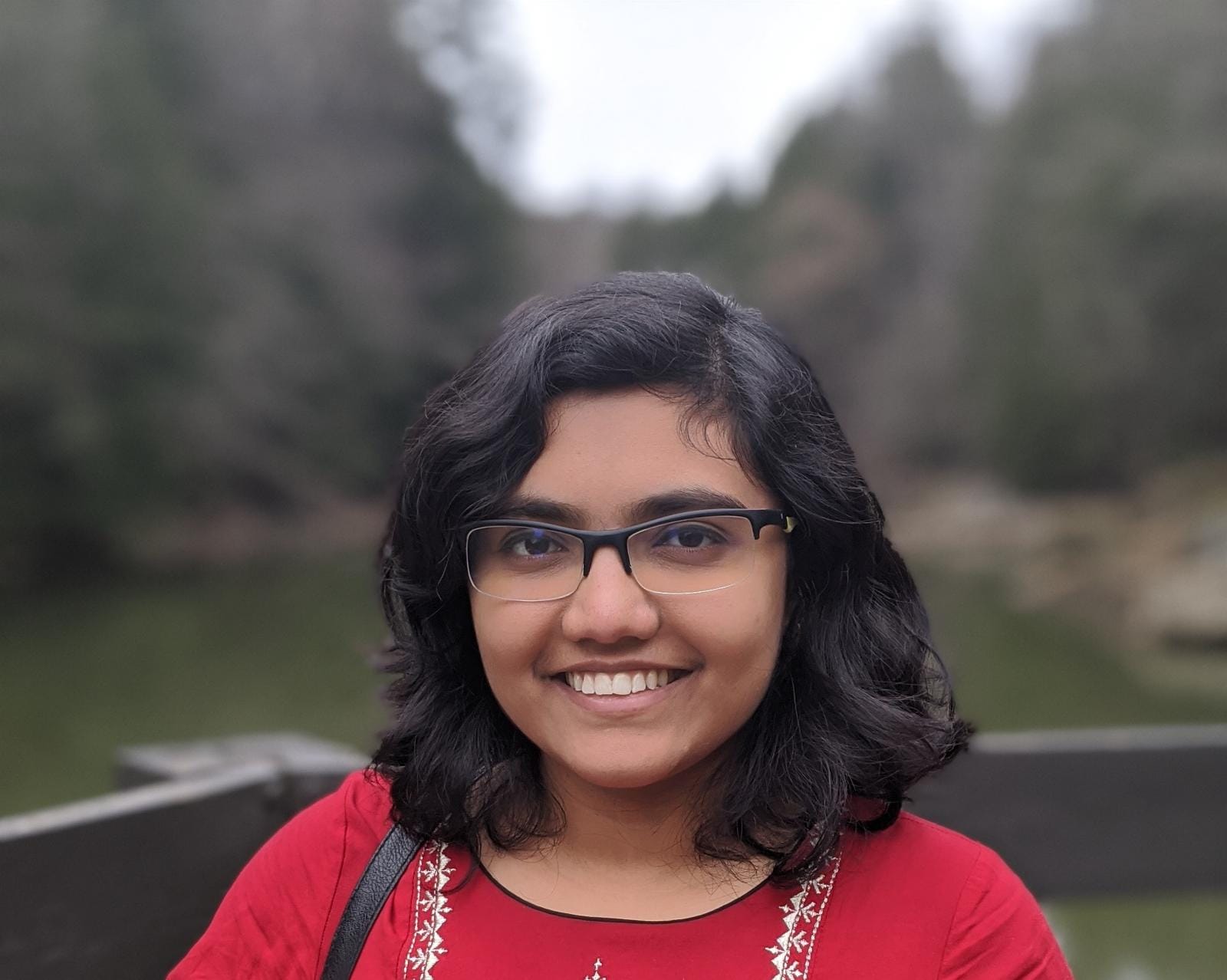
About the Speaker: Sanghamitra Dutta is a researcher at JP Morgan Chase AI Research, and an incoming faculty at the University of Maryland, College Park (from Fall 2022). She received her Ph.D. and Masters from Carnegie Mellon University and B. Tech. (honors) from IIT Kharagpur, all in Electrical and Computer Engineering. Her research interests revolve around machine learning, information theory, and statistics. She is currently focused on addressing the emerging issues in machine learning concerning fairness, explainability, and law with publications in both machine learning as well information theory venues (also featured in New Scientist). In her prior work, she has also examined problems in reliable computing, proposing novel algorithmic solutions for large-scale distributed machine learning in the presence of faults and failures, using tools from coding theory (an emerging area called “coded computing”). Her results on coded computing address long-standing foundational challenges in large-scale distributed machine learning and have received substantial attention from across communities (leading to several publications and two pending patents). She is a recipient of the 2021 AG Milnes Dissertation Award, 2020 Cylab Presidential Fellowship, 2019 K&L Gates Presidential Fellowship, 2019 Axel Berny Presidential Graduate Fellowship, 2017 Tan Endowed Graduate Fellowship, 2016 Prabhu and Poonam Goel Graduate Fellowship, 2015 Best Undergraduate Project Award at IIT Kharagpur, and the 2014 HONDA Young Engineer and Scientist Award. She has also pursued research internships at IBM Research and Dataminr.
Deep Inference
Speaker: Karl J. Friston, University College London, UK
Date: Oct 21
Abstract: In the cognitive neurosciences and machine learning, we have formal ways of understanding and characterizing perception and decision-making; however, the approaches appear very different: current formulations of perceptual synthesis call on theories like predictive coding and Bayesian brain hypothesis. Conversely, formulations of decision-making and choice behavior often appeal to reinforcement learning and the Bellman optimality principle. On the one hand, the brain seems to be in the game of optimizing beliefs about how its sensations are caused; while, on the other hand, our choices and decisions appear to be governed by value functions and reward. Are these formulations irreconcilable, or is there some underlying information theoretic imperative that renders perceptual inference and decision- making two sides of the same coin?
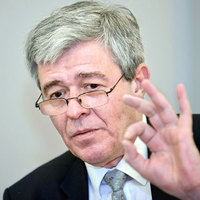
About the Speaker: Karl Friston is a theoretical neuroscientist and authority on brain imaging. He invented statistical parametric mapping (SPM), voxel-based morphometry (VBM) and dynamic causal modelling (DCM). These contributions were motivated by schizophrenia research and theoretical studies of value-learning, formulated as the dysconnection hypothesis of schizophrenia. Mathematical contributions include variational Laplacian procedures and generalized filtering for hierarchical Bayesian model inversion. Friston currently works on models of functional integration in the human brain and the principles that underlie neuronal interactions. His main contribution to theoretical neurobiology is a free-energy principle for action and perception (active inference). Friston received the first Young Investigators Award in Human Brain Mapping (1996) and was elected a Fellow of the Academy of Medical Sciences (1999). In 2000 he was President of the international Organization of Human Brain Mapping. In 2003 he was awarded the Minerva Golden Brain Award and was elected a Fellow of the Royal Society in 2006. In 2008 he received a Medal, College de France and an Honorary Doctorate from the University of York in 2011. He became of Fellow of the Royal Society of Biology in 2012, received the Weldon Memorial prize and Medal in 2013 for contributions to mathematical biology and was elected as a member of EMBO (excellence in the life sciences) in 2014 and the Academia Europaea in (2015). He was the 2016 recipient of the Charles Branch Award for unparalleled breakthroughs in Brain Research and the Glass Brain Award, a lifetime achievement award in the field of human brain mapping. He holds Honorary Doctorates from the University of Zurich and Radboud University.
Active Distribution Networks: Clean, robust, self-controlled and profitable
Speaker: Panayiotis (Panos) Moutis, Carnegie Mellon University
Date: Oct 28
Abstract: Although black-outs which are widely spread through a transmission system are relatively rare, faults and disconnections at the distribution level are notably more frequent and add up to service interruptions of – at least – a few hours per customer every year. At the same time, the restructuring of electricity markets and the calls for the clean energy transition have led policy initiatives, standardization and encouraged the industry to develop energy resources and equipment suited for the distribution grid, its needs and its customers. Finally, from a practical aspect, distribution networks (DNs) are intricate to operate, because they are geographically dispersed with hundreds of buses over dozens of feeders. Hence, the reliability, resilience and quality of service at DNs is a challenging and socially critical task that remains very timely. This task requires accurate monitoring and multi-objective control of DNs, based on suitable hardware, decentralized algorithms (if not fully distributed) and appropriate economies to benefit society in a fairer manner. In this talk, the presenter will review experiences and research outcomes from his works in the industry and the academia, including the digital twin of distribution transformers, distributed optimization of storage systems in DNs with on-roof photovoltaics, and others.

About the Speaker: Panayiotis (Panos) Moutis, PhD, has been Special Faculty with the Scott Institute for Energy Innovation at Carnegie Mellon University (CMU) since August 2018 (postdoc at Electrical & Computer Engineering, CMU, 2016-18). His recent grants include one from the national system operator of Portugal, REN, for the development of a transmission expansion planning platform, and another from the moonshot factory of Google, X, for the digital twin of the electrical grid. Between 2018-20 he served as a Marie Curie Research Fellow with DEPsys, Switzerland, on distribution grid synchronized measurements and state estimation. In 2014 he was awarded a fellowship by Arup UK (through the University of Greenwich), on the “Research Challenge of Balancing Urban Microgrids in Future Planned Communities”. In 2013 he won the “IEEE Sustainability 360o Contest” on the topic of Power. Throughout 2007-15, as part of Prof. Nikos Hatziargyriou’s research group he contributed to over a dozen R&D projects funded by the European Commission. Panos received both his diploma (2007) and his PhD (2015) degrees in Electrical & Computer Engineering at the National Technical University of Athens, Greece, and has published more than 30 papers and contributed to 5 book chapters. He has accumulated over 10 years of industry experience on projects of Renewable Energy Sources and Energy Efficiency, and serves in energy start-ups as advisor and executive. He is a senior member of multiple IEEE societies, member of the IEEE-USA Energy Policy Committee and NASPI, associate editor of IEEE & IET scientific journals, active contributor to IEEE standards working groups, chair of the IEEE Smart Grid Publications Committee and editor-in-chief of the “IEEE Smart Grid Newsletter”.
Novel Applications of 2D Materials from Wearable Health to Memory Devices and 5G Switches
Speaker: Deji Akinwande, University of Texas – Austin
Date: Oct 29
Abstract: This talk will present our latest research adventures on 2D nanomaterials towards greater scientific understanding and advanced engineering applications. In particular, the talk will highlight our work on flexible electronics, zero-power devices, single-atom monolayer memory, non-volatile RF/5G/6G switches, and wearable tattoo sensors for mobile health. Non-volatile memory devices based on 2D materials are an application of defects and is a rapidly advancing field with rich physics that can be attributed to metal adsorption into vacancies. The memory devices can be used for neuromorphic computing and operate as switches up to 500GHz. Likewise, from a practical point, electronic tattoos based on graphene have ushered a new material platform that has highly desirable practical attributes including optical transparency, mechanical imperceptibility, and is the thinnest conductive electrode sensor that can be integrated on skin for physiological measurements including blood pressure monitoring with Class A performance. Much of these research achievements have been published in leading journals.
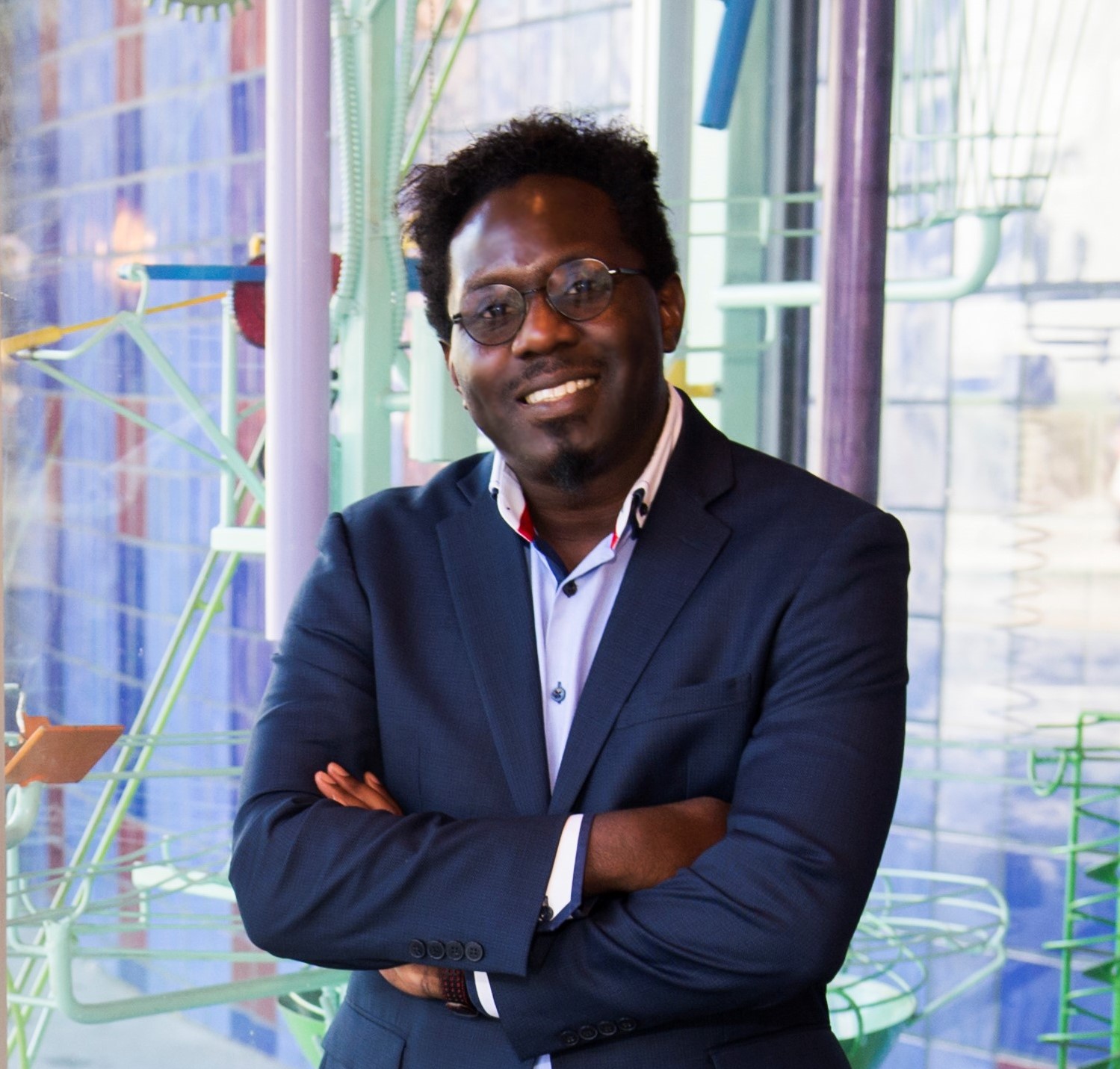
About the Speaker: Deji Akinwande is an Endowed Full Professor at the University of Texas at Austin, and a Fellow of the IEEE and APS. He received the PhD degree from Stanford University in 2009. His research focuses on 2D materials and nanoelectronics/technology, pioneering device innovations from lab towards applications. Prof. Akinwande has been honored with the 2019 Fulbright Specialist Award, 2017 Bessel-Humboldt Research Award, the U.S Presidential PECASE award, the inaugural Gordon Moore Inventor Fellow award, the inaugural IEEE Nano Geim and Novoselov Graphene Prize, the IEEE “Early Career Award” in Nanotechnology, the NSF CAREER award, several DoD Young Investigator awards, and was a past recipient of fellowships from the Kilby/TI, Ford Foundation, Alfred P. Sloan Foundation, 3M, and Stanford DARE Initiative. His research achievements have been featured by Nature news, Time and Forbes magazine, BBC, Discover magazine, Wall Street Journal, and many media outlets. He serves as an Editor for the IEEE Electron Device Letters and Nature NPJ 2D Materials and Applications. He Chairs the 2022 Gordon Research Conference on 2D materials, and was the past chair of the 2019 Device Research Conference (DRC), and the 2018 Nano-device committee of IEEE IEDM Conference.
Resilient and secure distributed computing and learning via coded computing
Speaker: Viveck R. Cadambe, Pennsylvania State University
Date: Nov 4
Abstract: We present ideas of coded computing - an emerging paradigm that uses information and coding theoretic tools to handle bottlenecks in distributed computing and learning. The ideas of coded computing can mitigate straggler bottlenecks in distributed computing, enable secure computation over untrusted devices, and enable scalable data privacy by generalizing classical secure multiparty computing and secret sharing techniques. We present an overview of coding ideas and fundamental limits on the trade-off between resilience, security and redundancy overheads.
We will survey the history of resilient computing for linear algebraic operations starting the 1980s, cast them in a coding-theoretic language, and describe some elegant recent solutions that yield significant improvements in the trade-off between resilience and redundancy. In contrast to classical coding theory that aims that operates over finite fields, we demonstrate some surprising threshold phenomena on the aforementioned trade-off for code development for approximate real-valued computations. We then show the utility of the developed codes to a wide class of computations beyond linear algebraic computations, and describe some promising directions in this regard. Experimental results in the context of resilient distributed training and inference in machine learning will be presented to demonstrate the promise of the ideas.
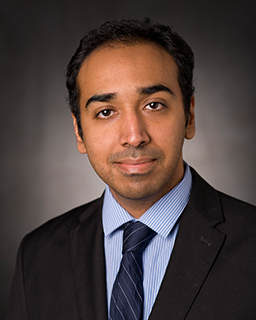
About the Speaker: Viveck R. Cadambe is an Associate Professor in the Electrical Engineering at Pennsylvania State University. He received his Ph.D. from University of California Irvine in 2011, and has done postdoctoral stints at Boston University and MIT. His research interests are in information and coding theory, and he studies applications to distributed computing and machine learning, cloud based data storage services, and wireless communication networks. He has received the 2009 Information Theory Society Best Paper Award, the 2014 IEEE Network Computing and Applications Best Paper Award, an NSF CRII Award in 2015, an NSF Career Award in 2016 and a Google Faculty Award in 2019. He has served as an Associate Editor for IEEE Transactions on Wireless Communications and for the IEEE Journal on Special Areas in Information Theory.
Forensic Analysis of Vehicular Malfunctions Using On-Board Data
Speaker: William Rosenbluth, Peter J Sullivan; Automotive Systems Analysis, Inc
Date: Nov 30
Abstract: The authors are involved with forensic analysis and recording of vehicle dynamic performance tests and investigations. To accomplish these tests and investigations, they needed and developed forensically accurate and reliable test monitoring and recording instruments. And, because the tests were usually sequential and interactive between the operator and the particular exemplar vehicle, those instruments had to allow for real time graphical readouts and displays of parameters from both vehicle data networks and from instrumentation data networks.
It is hoped that the methodologies and examples shown here will encourage students and professionals to explore data analysis designs and solutions beyond basic or textbook procedures by providing examples to show real world data projects and test instruments that are now repeatedly used in many continuing vehicle forensic investigations. Additionally, these working examples have been shown to assist associated skill disciplines such as Reconstruction and Biometric Analysis of these same accidents.
About the Speaker: Willam Rosenbluth is President and Principal Engineer of Automotive Systems Analysis, Inc, Reston, VA. For the past 35 years he has performed technical investigations of automotive electrical and computer control systems. He holds a BEE ‘61 and an MSEE ‘65 from the Polytechnic Institute of Brooklyn.
Peter J Sullivan is President and Principal Engineer of Advanced Analysis Associates, Inc, Houston TX. For the past 26 years he has performed Forensic Expert Witness investigations for clients throughout the US, and when appropriate, he has testified in State and Federal Courts throughout the USA.
Coding, Coded & Counting: A Bias Continuum
Speaker: Viveck R. Cadambe, Pennsylvania State University
Date: Dec 2
Abstract: This presentation will review my research trajectory starting from earlier publications to works in progress. My earlier research examined information sharing and the impacts on health organizations and patients given the increased needs to share clinical information. I have continued this research with the expansion into social media, human computer interaction, content design as well as health disparities via comorbid conditions (including breast cancer, mental health, HIV) and data modeling. A central theme of my research is leveraging, creating and using data to assess society (community) needs and the intersection of disparities which exist as along an “implications” continuum. While much of the data created and used is a direct result of embedded notions of “systems” informing a direct outcome the coding, coded and counting (Web of Cs) does not exist in a vacuum. Rather, the coding and coded influence a counting of decisions used that impact lived experiences. For Black and Brown communities, this web of Cs informed algorithmic bias while overlooking factors including context, place and space.

About the Speaker: Dr. Fay Cobb Payton is a Full Professor (with Tenure) of Information Technology/Analytics at North Carolina State University and was named a University Faculty Scholar for her leadership in turning research into solutions to society’s most pressing issues. She is on rotation as a Program Director at the National Science Foundation (NSF) in the Division of Computer and Network Systems. At the NSF, she works with the CISE Minority-Serving Institutions Research Expansion Program (CISE-MSI Program), Smart Health and Biomedical Research in the Era of Artificial Intelligence and Advanced Data Science (SCH), AI-FEAT, Computing In Undergraduate Education, Broadening Participating Alliances in Computing and a number of other cross- directorate programs (INCLUDES, ADVANCE, HBCU Excellence in Research and NSF Research Traineeship). She (along with a group of colleagues) received the 2020 NSF Director’s Award.
She is a full member of Sigma Xi, and serves in the following capacities:
Association of Computing Machinery (ACM) Education Advisory and co-chair of its Diversity, Equity & Inclusion Committee
American Association for the Advancement of Science (AAAS), Science and Technology Fellows Selection Committee
Institute of Industrial & Systems Engineers, Health Systems and Diversity-Equity-Inclusion Committees
Dr. Payton has received the North Carolina Technology Association Tech Educator of the Year, PhD Project Hall of Fame and National Coalition of Women in Information Technology Undergraduate Mentoring Awards. She is a named SAS Institute Fellow for her work in analytics and teaching in the IT classroom, and as received two NC State University Alumni Extension Awards. She is a National Institute of Environmental Health Sciences Fellow where she worked on data management, technology and communications strategies for a health disparities study. As an American Council on Education Fellow, she worked on issues of academic review, interdisciplinary graduate research and education, and institutional economic and community impact.
Her research interests include healthcare IT/informatics/disparities; data quality; bias in AI/information seeking/HCI; access and participation in computing/STEM and entrepreneurship pathways. She has published over 100 peer-reviewed journal articles, conference publications and book chapters. Her research has been published and/or forthcoming in the Journal of the American Medical Informatics Association, Issues in Science and Technology, Communications of the ACM, European Journal of Information Systems, Journal of Medical Internet Research, IIE Transactions on Healthcare Systems Engineering, Information Systems Journal, Journal of the Association for Information Science and Technology, Health Care Management Science, Telemedicine and eHealth, just to name a few. The National Science Foundation, National Institutes of Health, AT&T, Kenan Institute and others have supported Dr. Payton’s research and teaching.
She serves on several local and national boards and has been recognized in NC State and other media outlets for research and mentoring work. She has presented her research at national and international conferences and symposia in China, Nigeria, Netherlands, Canada, Germany, Greece, Ireland, UK, Ghana, just to name a few. Dr. Payton has worked in an advisory and/or volunteer capacity with the PhD Project Information Systems Doctoral Student Association, YMCA, Wake County Public School Systems and the City of Raleigh. She is often the speaker or panelist for corporate career development and leadership programs, and serves as a consultant for leadership development programs for current mid-level corporate African Americans in IT careers.
Free Energy Minimization Learning: Information-Theoretic Fundamentals and Applications
Speaker: Osvaldo Simeone, King's College London, UK
Date: Dec 16
Abstract: The free energy principle in neuroscience postulates that living beings train internal models of the environment that allow them to act in a manner that minimizes surprise -- i.e., that reduces uncertainty. In this talk, free energy minimization (FEM) will be presented as a unifying training criterion encompassing frequentist, Bayesian, variational, as well as modern generalized Bayesian, learning formulations. To this end, the information-theoretic foundations of FEM learning will be first reviewed via the generalization analysis of stochastic training algorithms defined by generalized posterior distributions. Then, the theoretical and algorithmic frameworks of FEM learning will be extended to the higher level of abstraction of meta-learning, also known as learning to learn. Finally, applications of FEM learning to neuromorphic computing will be described along with experimental results. Throughout, directions for further research, along with several applications to wireless communications, will be highlighted.
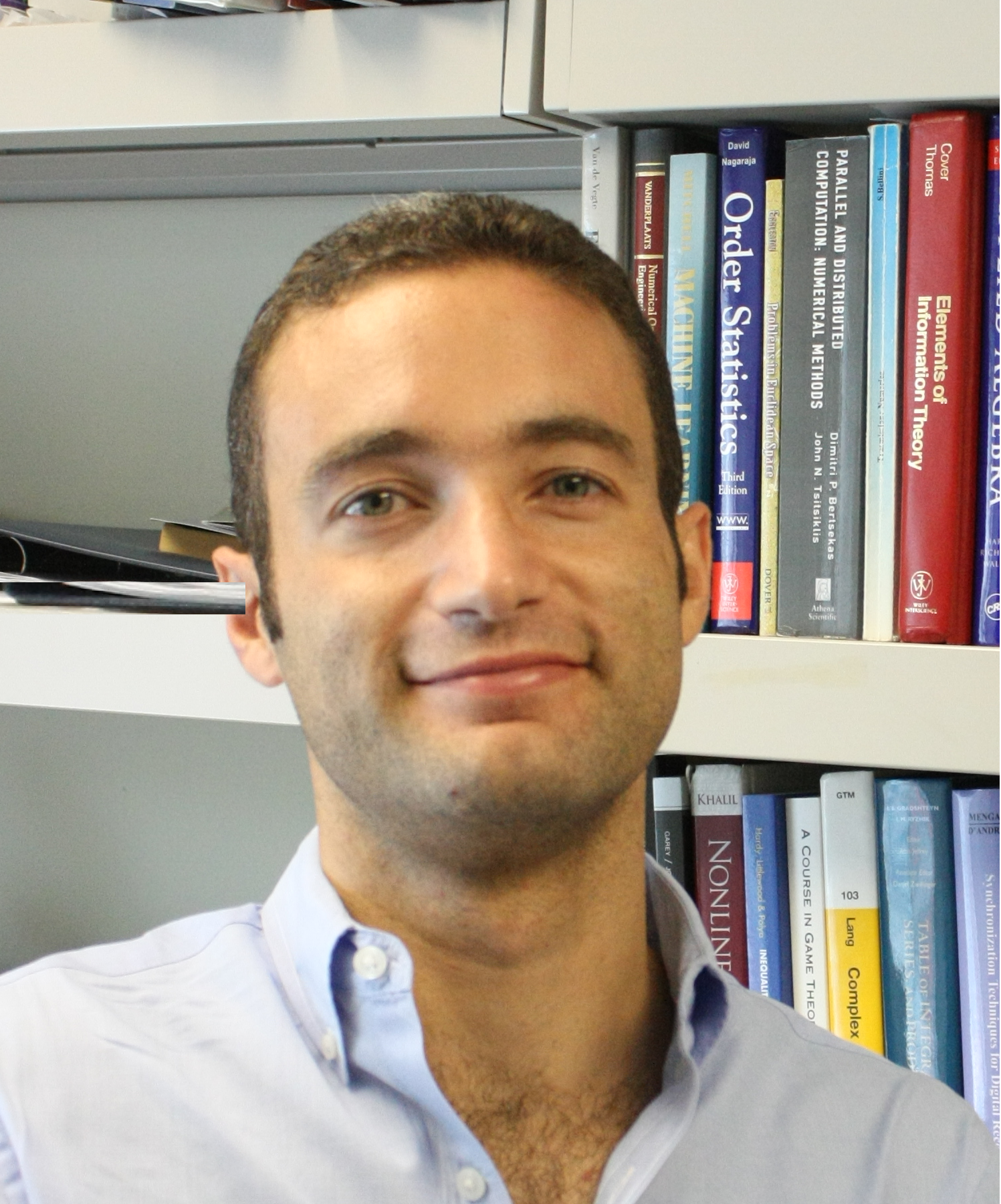
About the Speaker: Osvaldo Simeone is a Professor of Information Engineering with the Centre for Telecommunications Research at the Department of Engineering of King's College London, where he directs the King's Communications, Learning and Information Processing lab. He received an M.Sc. degree (with honors) and a Ph.D. degree in information engineering from Politecnico di Milano, Milan, Italy, in 2001 and 2005, respectively. From 2006 to 2017, he was a faculty member of the Electrical and Computer Engineering (ECE) Department at New Jersey Institute of Technology (NJIT), where he was affiliated with the Center for Wireless Information Processing (CWiP). His research interests include information theory, machine learning, wireless communications, and neuromorphic computing. Dr Simeone is a co-recipient of the IEEE Vehicular Technology Society 2021 Jack Neubauer Memorial Award, 2019 IEEE Communication Society Best Tutorial Paper Award, the 2018 IEEE Signal Processing Best Paper Award, the 2017 JCN Best Paper Award, the 2015 IEEE Communication Society Best Tutorial Paper Award and of the Best Paper Awards of IEEE SPAWC 2007 and IEEE WRECOM 2007. He was awarded a Consolidator grant by the European Research Council (ERC) in 2016. His research has been supported by the U.S. NSF, the ERC, the Vienna Science and Technology Fund, as well as by a number of industrial collaborations. He currently serves in the editorial board of the IEEE Signal Processing Magazine and is the chair of the Signal Processing for Communications and Networking Technical Committee of the IEEE Signal Processing Society. He was a Distinguished Lecturer of the IEEE Information Theory Society in 2017 and 2018, and he is currently a Distinguished Lecturer of the IEEE Communications Society. Dr Simeone is a co-author of a textbook (to be published by Cambridge University Press), two monographs, two edited books, and more than 160 research journal papers. He is a Fellow of the IET and of the IEEE.