Fall 2020 Seminars
A complete listing
Watching IoTs That Watch Us: Empirically Studying IoT Security & Privacy at Scale
Speaker: Danny Y. Huang, New York University
Date: Sept 8
Abstract: Consumers today are increasingly concerned about the security and privacy risks of smart home IoT devices. However, few empirical studies have looked at these problems at scale, partly because a large variety and number of smart-home IoT devices are often closed-source and on private home networks, thus making it difficult for researchers to systematically observe the actual security and privacy issues faced by users in the wild.
In this talk, I describe two methods for researchers to empirically understand these risks to real end-users: (i) crowdsourcing network traffic from thousands of real smart home networks [IMWUT '20], and (ii) emulating user-inputs to study how thousands of smart TV apps track viewers [CCS '19]. Both methods have allowed us to conduct the largest security and privacy studies on smart TVs and other IoT devices to date. Our labeled datasets have also created new opportunities for other research areas, such as machine learning, network management, and healthcare.
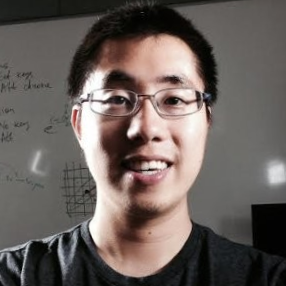
About the Speaker: Danny Y. Huang is an Assistant Professor affiliated with ECE and CUSP. He officially joined NYU 10 days ago. He is broadly interested in the security and privacy of consumer technologies, such as cryptocurrency and IoT. He did a postdoc at Princeton University and obtained his PhD in Computer Science and Engineering from University of California, San Diego. For more information, visit https://mlab.engineering.nyu.edu/.
Physiognomic AI
Speaker: Jevan Hutson, University of Washington School of Law
Date: Oct 20
Abstract: Artificial intelligence (AI) techniques in computer vision and related fields enabled by machine learning (ML) are ushering in a new era of computational physiognomy and phrenology. These scientifically baseless, racist, and socially discredited fields purporting to determine a person character, capability, or future social outcomes based on their facial features, expressions, or other physical or biometric characteristics should be anathema to any researcher or product developer working in computer science today, yet physiognomic and phrenological claims now appear regularly in research papers, at top AI conferences, and in the sales pitches of some digital technology companies. The reanimation of physiognomy and phrenology at scale through computer vision and machine learning is a matter of urgent concern. Physiognomic AI, this talk contends, is the practice of using computer software to infer an individual’s character, natural capabilities, and future social outcomes based on their physical or behavioral characteristics. This talk, which represents an ongoing collaboration with Dr. Luke Stark and contributes to the intersection of critical data studies, consumer protection law, biometric privacy law, and civil rights law, endeavors to conceptualize and problematize physiognomic AI and offer policy recommendations for state and federal lawmakers to forestall its proliferation.

About the Speaker: Jevan Hutson is a lawyer, data justice and privacy advocate, and human-computer interaction researcher, who proposed restrictions on facial recognition technology and AI-enabled profiling in the Washington State Legislature. He recently completed his law degree at the University of Washington School of Law, where he led Facial Recognition & AI Policy at the Technology Law & Public Policy Clinic and served on the External Biometrics Advisory Board of the Port of Seattle. He also holds an M.P.S. information Science and B.A. in History of Art & Visual Studies from Cornell University.
Identifying and Protecting Electricity Vulnerable New Yorkers
Speaker: Yury Dvorkin, New York University
Date: Oct 23
Abstract: Electricity vulnerability is a design factor that has been commonly overlooked in electricity planning, thus putting thousands in danger of serious health implications in case of even short electric power outages. Naturally, the ongoing COVID-19 outbreak has only exacerbated this danger as electricity demand has shifted to residential areas, thus causing unexpected stress, and response times of repair teams are expected to increase due to COVID-19 restrictions. This presentation will describe our ongoing project that collects power outage data in real-time and use social computing and open-source socio-demographic and environmental data to evaluate the severity of each outage for electricity vulnerable population groups, and prioritize outage repairs in the areas with vulnerable population groups.

About the Speaker: Yury is an Assistant Professor and Goddard Junior Faculty Fellow in the Department of Electrical and Computer Engineering at New York University’s Tandon School of Engineering with an affiliated appointment at NYU’s Center for Urban Science and Progress.
Efficient DNN Algorithms, Accelerators, and Automated Tools towards Green AI
Speaker: Yingyan (Celine) Lin, Rice University
Date: Oct 27
Abstract: While machine learning powered intelligence promises to revolutionize the way we live and work by enhancing our ability to recognize, analyze, and classify the world around us, this revolution has yet to be unleashed. First, powerful machine learning algorithms require prohibitive energy consumption (e.g., hundreds of layers and tens of millions of parameters), whereas many daily life devices, such as smartphones, smart sensors, and drones, have limited energy and computation resources since they are battery-powered and have a small form factor. Second, the excellent performance of modern deep neural networks (DNNs) comes at an often exorbitant training cost due to the required vast volume of training data and model parameters, e.g., training a single DNN can cost over $10K US dollars and emit as much carbon as five cars in their lifetimes, raising various environmental concerns. To address the aforementioned gap and challenge, the Efficient and Intelligent Computing (EIC) Lab at Rice University has been developing efficient DNN algorithms, accelerators, and automated tools. In this talk, I will share some promising techniques we recently developed and exciting projects that we are working on.
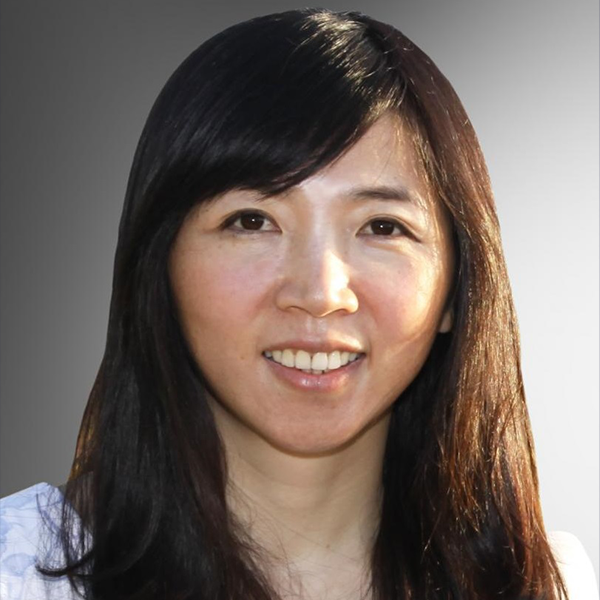
About the Speaker: Yingyan (Celine) Lin is an Assistant Professor in the Department of Electrical and Computer Engineering at Rice University. She leads the Efficient and Intelligent Computing (EIC) Lab at Rice, which focuses on embedded machine learning and aims to develop techniques towards green AI and ubiquitous machine learning powered intelligence. She received a Ph.D. degree in Electrical and Computer Engineering from the University of Illinois at Urbana-Champaign in 2017, a Best Student Paper Award at the 2016 IEEE International Workshop on Signal Processing Systems (SiPS 2016), and the 2016 Robert T. Chien Memorial Award for Excellence in Research at UIUC. She was selected as a Rising Star in EECS by the 2017 Academic Career Workshop for Women at Stanford University. Dr. Lin is currently the lead PI on multiple multi-university projects and her group has been funded by NSF, NIH, ONR, Qualcomm, and Intel.
Hardware-based Acceleration of Homomorphic Encryption
Speaker: Mihalis Maniatakos, NYU-AD
Date: Nov 3
Abstract: The rapid expansion and increased popularity of cloud computing comes with no shortage of privacy concerns about outsourcing computation to semi-trusted parties. While cryptography has been successfully used to solve data-in-transit (e.g., HTTPS) and data-at-rest (e.g., AES encrypted hard disks) concerns, data-in-use protection remains unsolved. Homomorphic encryption, the ability to meaningfully manipulate data while data remains encrypted, has emerged as a prominent solution. The performance degradation compared to non-private computation, however, limits its practicality. In this talk, we will discuss our ongoing efforts towards accelerating homomorphic encryption at the hardware level. We will present the first ASIC implementation of a partially homomorphic encrypted co-processor, as well as discuss the prototype of a fully homomorphic encryption accelerator. The talk will also introduce E3, our framework for compiling C++ programs to their homomorphically encrypted counterparts, as well as E3X, our architectural extensions for accelerating computation on encrypted data demonstrated on an OpenRISC architecture.
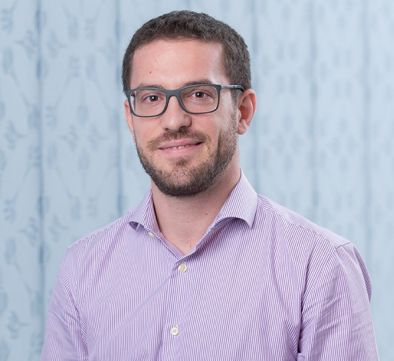
About the Speaker: Mihalis Maniatakos is an Associate Professor of Electrical and Computer Engineering at New York University Abu Dhabi, UAE, and a Global Network University Associate Professor at the NYU Tandon School of Engineering, USA. He is the Director of the MoMA Laboratory (nyuad.nyu.edu/momalab). He received his Ph.D. in Electrical Engineering, as well as M.Sc., M.Phil. degrees from Yale University, New Haven, CT, USA. He also received the B.Sc. and M.Sc. degrees in Computer Science and Embedded Systems, respectively, from the University of Piraeus, Greece. His research interests, funded by industrial partners, the US government, and the UAE government, include privacy-preserving computation, industrial control systems security, and machine learning security. Prof. Maniatakos has authored several publications in IEEE transactions and conferences, holds patents on privacy-preserving data processing and serves in the technical program committee for various international conferences. His cybersecurity work has also been extensively covered by Reuters and BBC.
Towards Reliable and Secure NISQ Systems
Speaker: Samah Saeed, City University of New York
Date: Nov 10
Abstract: Near-term quantum computers referred to as Noisy Intermediate-Scale Quantum (NISQ) computers consist of tens of inherently noisy quantum bits (qubits). They are expected to work as accelerators for solving various problems such as optimization and drug discovery. However, their reliability is very challenging in the presence of limited number of qubits, which cannot support error correction. To reduce the impact of errors, the physical constraints of the quantum hardware should be incorporated into the design process of quantum circuits.
In this talk, we will discuss possible vulnerabilities in the design flow of quantum circuits executed on NISQ systems in addition to their qubits reliability challenges. Then, I will present our ongoing efforts toward reliable and secure NISQ systems. Specifically, I will talk about our recent work on developing online automated test methodologies of quantum circuits for runtime detection of malicious and unexpected changes in the qubit behavior.
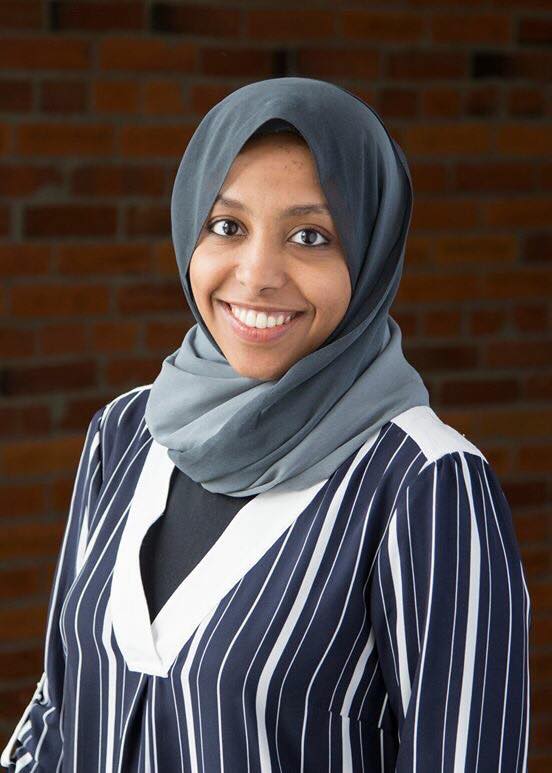
About the Speaker: Samah Saeed is an assistant professor in the electrical engineering department at Grove School of Engineering at CCNY, City University of New York. She received her Ph.D. in the Computer Science and Engineering Department New York University Tandon School of Engineering, NY, USA. Her research interests include the security and the reliability of quantum circuits, hardware security, and testing VLSI circuits. She is the winner of the best paper award at the IEEE VLSI Test Symposium, the Pearl Brownstein Doctoral Research Award by NYU Polytechnic School of Engineering, and the TTTC's E.J. McCluskey Best Doctoral Thesis Award at the IEEE International Test Conference.
Protecting Systems Against Hardware Based Attacks
Speaker: Nicole Fern, Tortuga Logic
Date: Nov 13
Abstract: Hardware is integral to system security, however hardware-focused attacks such as Meltdown and Spectre, Starbleed, and Rowhammer are on the rise. There are many challenges chip vendors face when trying to implement a security strategy on top of aggressive time-to-market schedules and increasing demands for better performance and more features. I will speak to these challenges and emerging solutions from the perspective of a security engineer working for Tortuga Logic, a hardware security startup, and as an academic whose PhD research focus was on pre-silicon security verification. This presentation will provide an overview of “what can go wrong” in hardware, and touch on topics such as security verification versus functional verification and implementing a secure development lifecycle for hardware by leveraging information flow tracking.
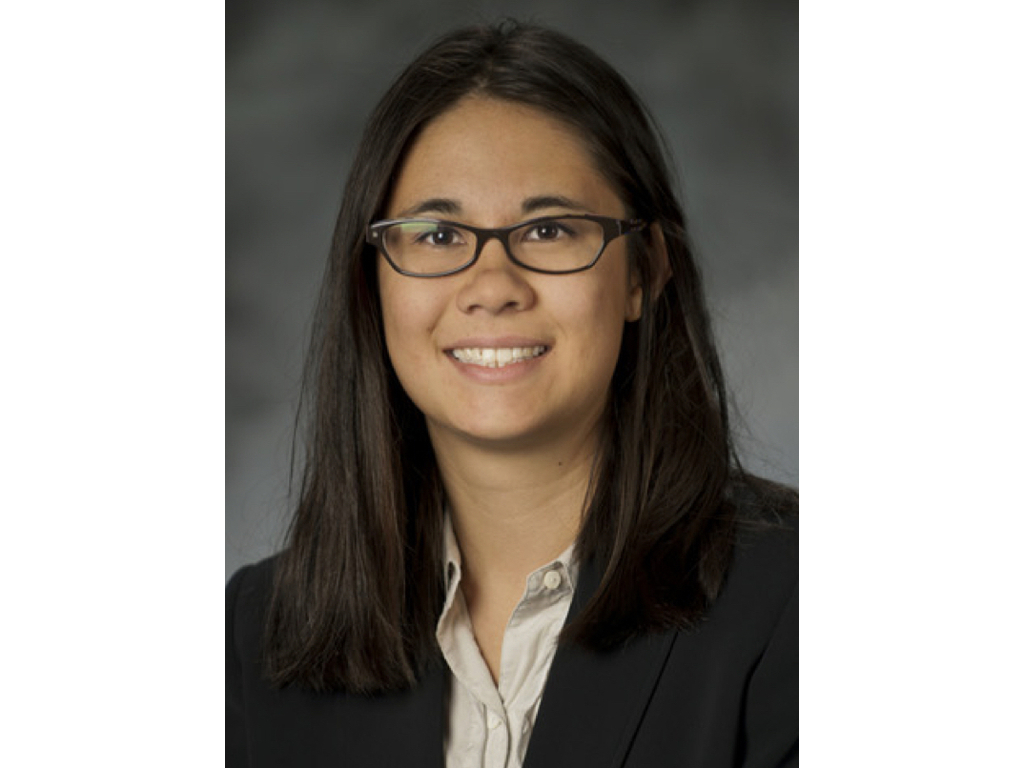
About the Speaker: Nicole Fern is a Senior Hardware Security Engineer at Tortuga Logic whose primary role is providing security expertise and defining future features and applications for the product line. Before joining Tortuga Logic she was a postdoc at UC Santa Barbara. Her research focused on the topics of hardware verification and security.
DNN Training Acceleration through Better Communication-Computation Overlap
Speaker: Sangeetha Abdu Jyothi, University of California, Irvine
Date: Nov 17
Abstract: As deep learning continues to revolutionize a variety of domains, training of Deep Neural Networks (DNNs) is emerging as a prominent workload in data centers. However, the relationship between communication and computation, a key factor that affects the DNN training throughput, is often overlooked in this network- and compute-intensive workload. In this talk, I will cast light on the communication-computation interdependencies that are critical for DNN training acceleration, and present two systems that significantly improve the training performance by leveraging this understanding. I will first discuss the communication paradigms, Parameter Server and AllReduce, and examine scalability challenges in each of them. I will then present TicTac, a system that optimizes training throughput by up to 37% through computation-aware parameter transfer scheduling in Parameter Servers. Next, I will introduce our system, Caramel, which improves training throughput under AllReduce by up to 3.62x using computation scheduling to achieve better communication-computation overlap.
About the Speaker: Sangeetha Abdu Jyothi is an Assistant Professor in the Department of Computer Science at the University of California, Irvine. Her research interests are in the broad areas of computer networking and systems, with a current focus on systems and machine learning. She completed her Ph.D. at the University of Illinois, Urbana-Champaign in 2019, and spent a year at VMware Research as a postdoc, where she continues as an Affiliated Researcher currently. She is a winner of the Facebook Graduate Fellowship (2017) and was invited to attend the Heidelberg Laureate Forum (2019) and the Rising Stars in EECS Workshop at MIT (2018).
Tolerable Delay: Augmenting Cloud Connectivity with Opportunistic Communication for Entity Centered Applications
Speaker: Corey Baker, University of Kentucky
Date: Nov 18
Abstract: Reliance on Internet connectivity is detrimental where modern networking technology is lacking, power outages are frequent, or network connectivity is expensive, sparse, or non-existent (i.e., developing countries, natural disasters, and rural areas). Though there have been discussions for years about 5G serving as the conduit for connecting any and everything; scalability issues are a major concern and deployments have been limited. Realization of the limitations resulting from reliance on Internet and cellular connectivity are prevalent in mHealth applications where remote patient monitoring has improved the timeliness of clinical decision making, decreased the length of hospital stays, and reduced mortality rates everywhere in the nation except in rural communities like Appalachian Kentucky where chronic disease is approximately 20% more prevalent than other areas. As an alternative, deploying resilient networking technology can facilitate the flow of information in resource-deprived environments to disseminate life saving data. In addition, leveraging opportunistic communication can supplement cellular networks to assist with keeping communication channels open during high-use and extreme situations, along with keeping network connectivity costs at a minimum. This talk will discuss the pragmatic applications of opportunistic communication; specifically applied to healthcare and empowering low-cost smart cities, permitting any community to become smart and connected.

About the Speaker: Corey E. Baker is an Assistant Professor in the Department of Computer Science in the College of Engineering at the University of Kentucky (UK). He directs the Network Reconnaissance (NetRecon) Lab where his research interests are in the area of Cyber Physical Systems (CPS) with emphasis in: opportunistic wireless communication for the Internet of Things (IoT), smart cities, smart homes, and mobile health environments. Professor Baker received a B.S. degree in Computer Engineering from San Jose State University (SJSU), a M.S. in Electrical and Computer Engineering from California State University, Los Angeles (CSULA), and M.S. and Ph.D. degrees in Electrical and Computer Engineering from the University of Florida (UF) under the supervision of Professor Janise McNair. After completion of his graduate studies, Baker was a University of California Presidents Postdoctoral Fellow in the Electrical and Computer Engineering department at the University of California San Diego under the mentorship of Tara Javidi and Ramesh Rao. Baker was later a Visiting Scholar in the Electrical Engineering department at the University of Southern California under the mentorship of Bhaskar Krishnamachari.
Reinforcement learning control of a robotic knee prosthesis with a human in the loop
Speaker: Jennie Si, Arizona State University
Date: Nov 24
Abstract: Reinforcement learning control has broadened the theory, design, especially application of the classic or contemporary concept of control. This data-driven approach iteratively and adaptively solves an optimal control problem by interacting with the system under control. As such, it has further opened up the horizon of the application fields of traditional control. In this talk, I will motivate the discussion by first introducing a new control design challenge. The problem calls for real-time control inputs to a robotic knee prosthesis to enable human-robot integration for restoring amputees’ lost function of locomotion. The problem is challenging because the human-robot system is tightly coupled, actions of both human and robot immediately impact on the system performance or even destabilize the system, additionally, it is difficult or impossible to accurately model or predict the interacting dynamics involving an individual person and a robotic prosthesis. In this talk, I will introduce two classes of reinforcement learning control designs that our team has developed, discuss theoretical feasibility of these methods and evaluate their usefulness in applications. I will provide demonstrations of reinforcement learning based, automatic robotic knee prosthesis tuning to enable safe and continuous level ground walking.
About the Speaker: Dr. Jennie Si received the B.S. and M.S. degrees from Tsinghua University, Beijing, China, and the Ph.D. degree from the University of Notre Dame, Notre Dame, IN, USA. She has been a faculty member in the Department of Electrical Engineering at Arizona State University since 1991. Her research focuses reinforcement learning based adaptive optimal control. She is also interested in fundamental learning control mechanisms in the mammalian frontal cortex. Dr. Si is a recipient of the NSF/White House Presidential Faculty Fellow Award and the Motorola Engineering Excellence Award in 1995. She is a Distinguished Lecturer of the IEEE Computational Intelligence Society and an IEEE Fellow. She has served on several professional organizations’ executive boards, international conference committees, and editorial boards of IEEE transactions.
Accurate, Real-time Energy-efficient Scene Perception through Hardware Acceleration
Speaker: R. Iris Bahar, Brown University
Date: Dec 1
Abstract: Technological advancements have led to a proliferation of robots using machine learning systems to assist humans in a wide range of tasks. However, we are still far from accurate, reliable, and resource-efficient operations of these systems. Despite the strengths of convolutional neural networks (CNNs) for object recognition, these discriminative techniques have several shortcomings that leave them vulnerable to exploitation from adversaries. In addition, the computational cost incurred to train these discriminative models can be quite significance. Discriminative-generative approaches offers a promising avenue for robust perception and action. Such methods combine inference by deep learning with sampling and probabilistic inference models to achieve robust and adaptive understanding. The focus is now on implementing a computationally efficient generative inference stage that can achieve real-time results in an energy efficient manner. In this talk, I will present our work on Generative Robust Inference and Perception (GRIP), a discriminative-generative approach for pose estimation that offers high accuracy especially in unstructured and adversarial environments. I will then describe how we have designed an all-hardware implementation of this algorithm to obtain real-time performance with high energy-efficiency.
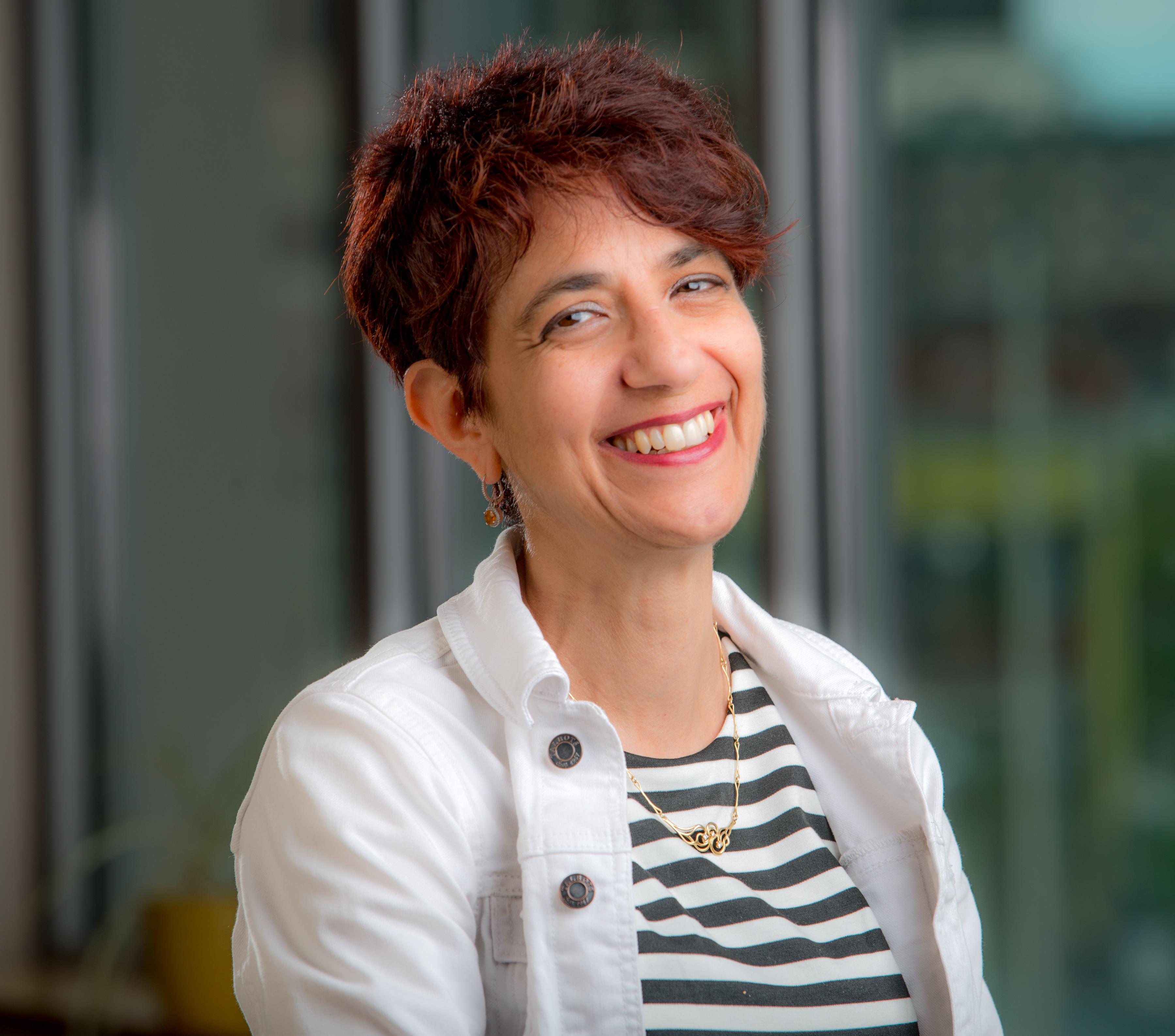
About the Speaker: R. Iris Bahar received the B.S. and M.S. degrees in computer engineering from the University of Illinois, Urbana-Champaign, and the Ph.D. degree in electrical and computer engineering from the University of Colorado, Boulder. Before entering the Ph.D program at CU-Boulder, she worked for Digital Equipment Corporation on their microprocessor designs. She has been on the faculty at Brown University since 1996 and now holds a dual appointment as Professor of Engineering and Professor of Computer Science. Her research interests have centered on energy-efficient and reliable computing, from the system level to device level. Most recently, this includes the design of robotic systems. Recently, she served as the Program Chair and General Chair of the International Conference on Computer-Aided Design (ICCAD) in 2017, 2018 respectively and the General Chair of the International Conference on Architectural Support for Programming Languages and Operating Systems (ASPLOS) in 2019. She is the 2019 recipient of the Marie R. Pistilli Women in Engineering Achievement Award and the Brown University School of Engineering Award for Excellence in Teaching in Engineering. More information about her research can be found at http://cs.brown.edu/people/irisbahar
Enabling Hyperscale Web Services
Speaker: Akshitha Sriraman, University of Michigan
Date: Dec 8
Abstract: Modern hyperscale web service systems introduce trade-offs between performance and numerous features essential for cost- and energy-efficient operation of data centers (e.g., high server utilization, continuous power management, and use of commodity hardware and software). In this talk, I will present two solutions to bridge the performance vs. cost and energy efficiency gap in hyperscale web services (1) a software system that auto-tunes threading models during system run-time to minimize web service tail latency (OSDI 2018) and (2) a system that exploits coarse-grained OS and hardware configuration knobs to tune cost-efficient commodity server processors, to better support their assigned service (ISCA 2019).
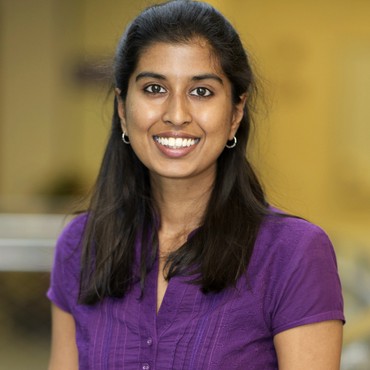
About the Speaker: Akshitha Sriraman is a PhD candidate in Computer Science and Engineering at the University of Michigan. Her dissertation research is on the topic of enabling hyperscale web services. Specifically, her work bridges computer architecture and software systems and demonstrates the importance of that bridge by realizing efficient web services from models on paper to deployment at hyperscale. Sriraman has influenced the design of server architectures both via hardware analysis of production data centers and her subsequent software designs that use data center hardware more efficiently. Sriraman has been recognized with a Facebook Fellowship (Distributed Systems), a Rackham Merit Ph.D. Fellowship, and was selected for the Rising Stars in EECS workshop. She hopes to enter academia after her PhD program, and will be on the academic job market (for tenure-track faculty positions) this upcoming cycle.
Learning Strong Inference Models in Small Data Domains: Towards Robust Human Pose Estimation
Speaker: Sarah Ostadabbas, Northeastern University
Date: Dec 9
Abstract: Recent efforts in machine learning (especially with the new waves of deep learning introduced in the last decade) have obliterated records for regression and classification tasks that have previously seen only incremental accuracy improvements. There are many other fields that would significantly benefit from machine learning (ML)-based inferences where data collection or labeling is expensive, such as healthcare. In these domains (i.e., Small Data domains), the challenge we now face is how to learn efficiently with the same performance with fewer data. Many applications will benefit from a strong inference framework with deep structure that will: (i) work with limited labeled training samples; (ii) integrate explicit (structural or data-driven) domain knowledge into the inference model as editable priors to constrain search space; and (iii) maximize the generalization of learning across domains. In this talk, I explore a generalized ML approach to solve the small data problem in the context of human pose estimation with several medical applications. There are two basic approaches to reduce data needs during model training: (1) decrease inference model learning complexity via data-efficient machine learning, and (2) incorporate domain knowledge in the learning pipeline through the use of data-driven or simulation-based generative models. In this talk, I present my recent work on merging the benefits of these two approaches to enable the training of robust and accurate (i.e., strong) inference models that can be applied on real-world problems dealing with data limitation. My plan to achieve this aim is structured in four research thrusts: (i) introduction of physics- and/or data-driven computational models here referred to as weak generator to synthesize enough labeled data in an adjacent domain; (ii) design and analysis of unsupervised domain adaptation techniques to close the gap between the domain adjacent and domain-specific data distributions; (iii) combined use of the weak generator, a weak inference model and an adversarial framework to refine the domain adjacent dataset by employing a set of unlabeled domain-specific dataset; and (iv) development and analysis of co-labeling/active learning techniques to select the most informative datasets to refine and adapt the weak inference model into a strong inference model in the target application.

About the Speaker: Professor Ostadabbas is an assistant professor in the Electrical and Computer Engineering Department of Northeastern University (NEU), Boston, Massachusetts, USA. Professor Ostadabbas joined NEU in 2016 from Georgia Tech, where she was a post-doctoral researcher following completion of her PhD at the University of Texas at Dallas in 2014. At NEU, Professor Ostadabbas is the director of the Augmented Cognition Laboratory (ACLab) with the goal of enhancing human information-processing capabilities through the design of adaptive interfaces via physical, physiological, and cognitive state estimation. These interfaces are based on rigorous models adaptively parameterized using machine learning and computer vision algorithms. In particular, she has been integrating domain knowledge with machine learning by using physics-based simulation as generative models for bootstrapping deep learning recognizers. Professor Ostadabbas is the co-author of more than 70 peer- reviewed journal and conference articles and her research has been awarded by the National Science Foundation (NSF), Mathworks, Amazon AWS, Biogen, and NVIDIA. She co-organized the Multimodal Data Fusion (MMDF2018) workshop, an NSF PI mini-workshop on Deep Learning in Small Data, the 2019 CVPR workshop on Analysis and Modeling of Faces and Gestures (AMFG2019) and she is the program chair of the Machine Learning in Signal Processing (MLSP2019). Prof. Ostadabbas is an associate editor of the IEEE Transactions on Biomedical Circuits and Systems, on the Editorial Board of the IEEE Sensors Letters and Digital Biomarkers Journal, and has been serving in several signal processing and machine learning conferences as a technical chair or session chair. She is a member of IEEE, IEEE Computer Society, IEEE Women in Engineering, IEEE Signal Processing Society, IEEE EMBS, IEEE Young Professionals, International Society for Virtual Rehabilitation (ISVR), and ACM SIGCHI.
Realistic but not Real: Synthetic electrical distribution models of the future
Speaker: Tarek Elgindy, National Renewable Energy Lab
Date: Dec 10
Abstract: The arpa-e funded SMART-DS project by NREL, Comillas university and MIT has released several synthetic electrical models that span entire cities, the largest of which serves over 4 million customers. These models have been created using a tool called the Reference Network Model (RNM-US) and processed using the Distribution Transformation Tool (DiTTo). They contain detailed electrical information of the distribution system including primary and secondary systems, substation internals, a sub-transmission system and 15 minute timeseries load data for each customer. Several scenarios of solar, battery, EV and demand response uptake have also been produced and attached to our models. Additionally we are currently creating synthetic distribution models for the entire state of Texas, which will be attached to a synthetic transmission model. This T&D dataset will be able to be co-simulated using the Hierarchical Engine for Large-scale Infrastructure CoSimulation (HELICS) tool. This presentation will demonstrate existing integrations with other projects at NREL, describe the methodology that was used to create and validate these models, and discuss future research use-cases for large-scale grid modelling that they can support.
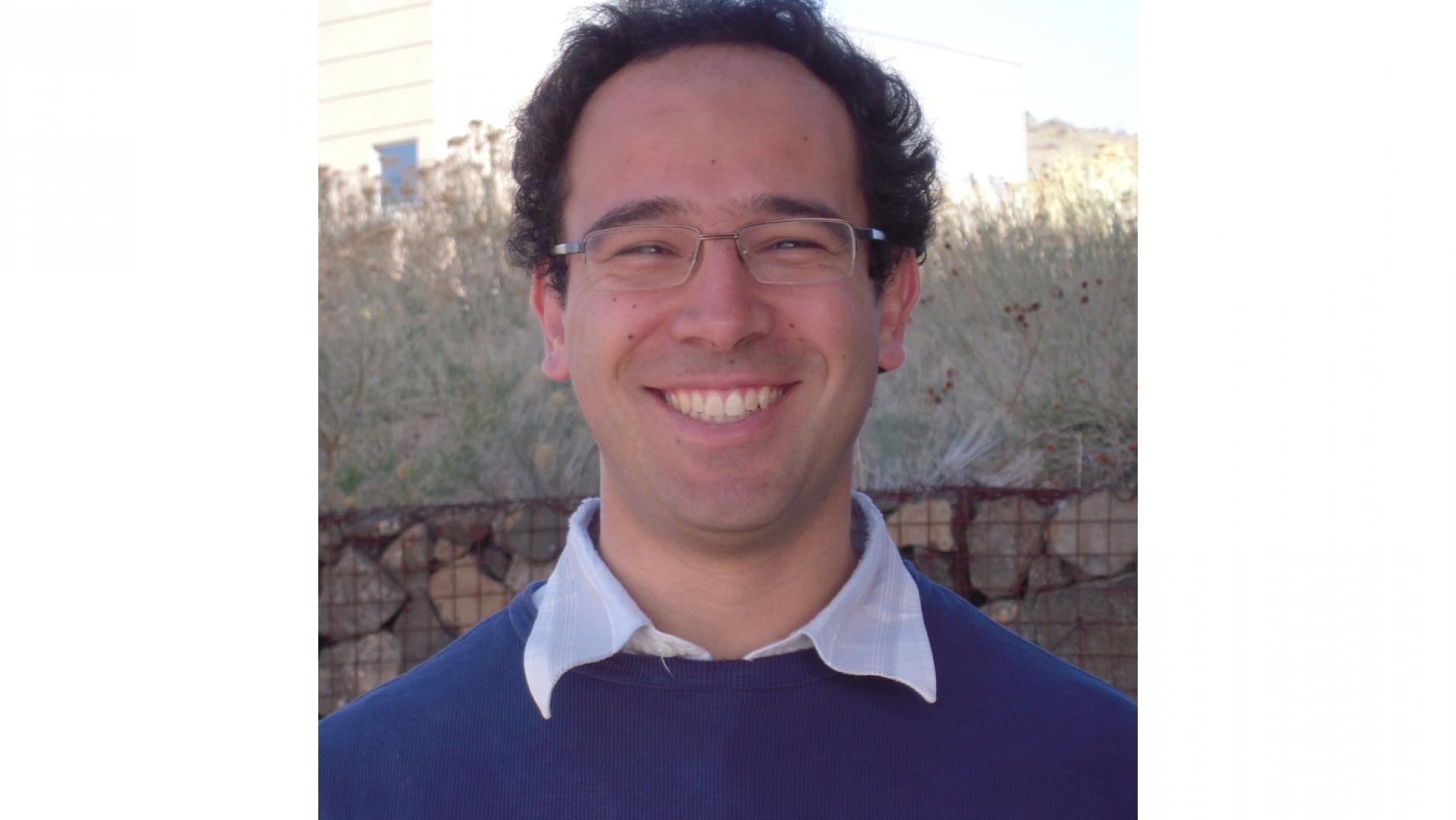
About the Speaker: Tarek Elgindy joined the National Renewable Energy Lab in 2015 after receiving a masters in algorithms, combinatorics and optimization from Carnegie Mellon University. Prior to his time in the US, Tarek worked at CSIRO on applied mathematical optimization with application to the Australian Future Grid Forum. Tarek has worked extensively on managing large electrical distribution datasets and understanding the impact of network structures and designs on power quality – primarily for quasi-static timeseries analysis. His research interests include developing market structures for distribution networks, developing tools for managing and cleaning electrical infrastructure data, and understanding the interactions between distribution and transmission systems with high penetrations of DERs.
Security and Stealth: Fundamental Limits
Speaker: Song Fang, New York University
Date: Dec 15
Abstract: Data security issues are becoming more and more prevalent nowadays, and not only in the cyber world but also in physical systems which are safety-critical in most cases. In this talk, we focus on analyzing the fundamental limits of stealth for data that are subject to attacks, and we consider both static and dynamic data, including data in dynamical systems such as control systems. It is shown in general that fundamental tradeoffs exist between the attack stealth (as quantified by the Kullback-Leibler divergence between the modified data and the original data) and the attack effect (as measured by the data distortion). Additionally, we characterize explicitly the worst-case attacks as well as the optimal defending strategies, in terms of power spectra shaping games.
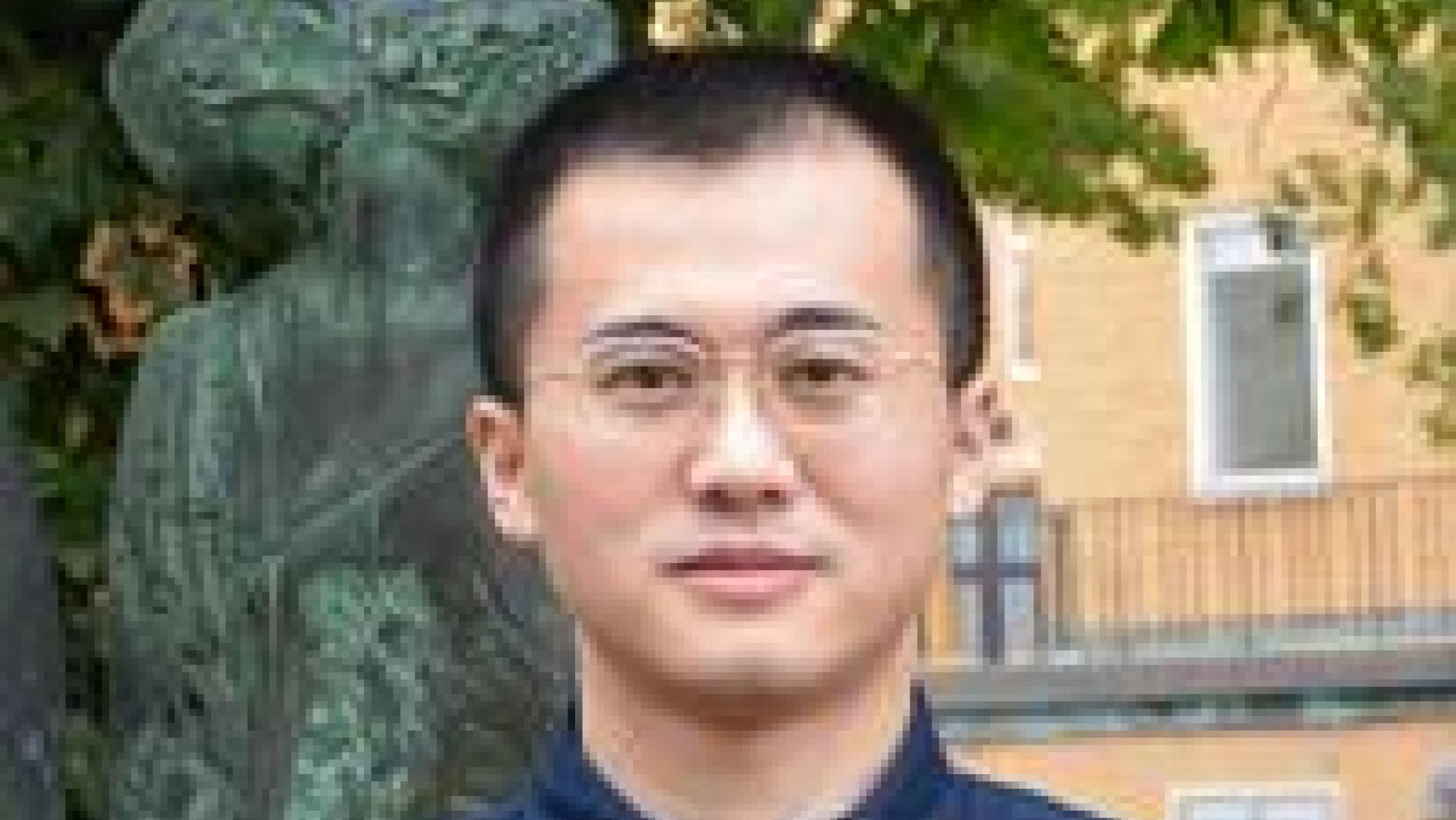
About the Speaker: Song Fang received the B.S. degree from Shandong University, the M.S. degree from Shanghai Jiao Tong University, and the Ph.D. degree from City University of Hong Kong. He was a postdoctoral researcher at Tokyo Institute of Technology and KTH Royal Institute of Technology. Currently, he is a postdoctoral researcher at New York University. He is broadly interested in the interplay of control theory (estimation/prediction theory), information theory (coding theory), and learning theory. He co-authored the book "Towards Integrating Control and Information Theories: From Information-Theoretic Measures to Control Performance Limitations" (Springer, 2017).
Hypothesis Testing by Machine Learning for Localization and Authentication by Wireless Signals
Speaker: Stefano Tomasin, University of Padova, Italy
Date: Dec 17
Abstract: Neural networks (NNs) and support vector machines (SVMs) are among the most popular tools of machine learning for binary hypothesis testing. The talk will investigate their properties, in the light of the Neyman-Pearson Lemma on optimal tests: we will show that, using deep-learning solutions and huge training sets, NNs and SVMs achieve optimality. As an example of application, the problem of deciding if a wireless-connected device is inside or outside a designated area (in-region location verification) will be addressed: here, the wireless channel features feed a decision machine, trained in a supervised fashion with data from both inside and outside the area. Then, NNs and SVMs will be employed also to confuse the decision process, pretending to be inside the area, while actually being outside. In this case, the machines will be trained with channel features taken only from one class. The problem of in-region-localization is also very close to that of user authentication, wherein we aim at verifying that a message received over a wireless channel comes from the declared transmitter. The talk will provide an overview of machine learning approaches for this purpose, and indicate some interesting future research directions on open problems.
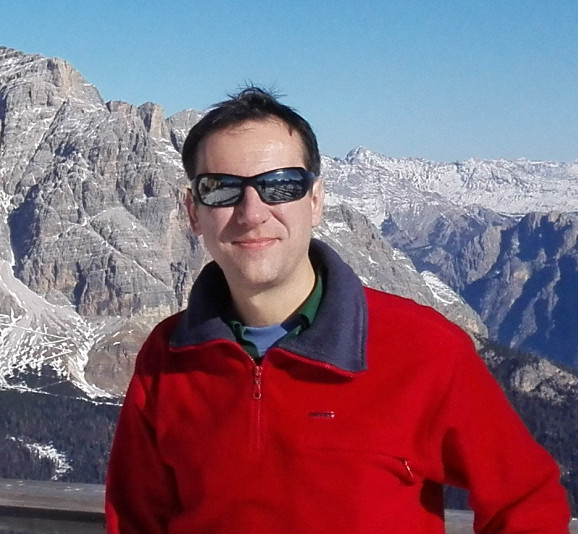
About the Speaker: Stefano Tomasin received the Ph.D. degree in Telecommunications Engineering from the University of Padova, Italy, in 2003. In 2002 he joined the University of Padova where he is now Associate Professor. He has been on leave at Philips Research (Eindhoven, Netherlands) in 2002, Qualcomm Research Laboratories (San Diego, California) in 2004, Polytechnic University (Brooklyn, New York) in 2007, and Huawei Mathematical and Algorithmic Sciences Laboratory (Boulogne-Billancourt, France) in 2015. His current research interests include physical layer security and signal processing for wireless communications, with application to 5th generation cellular systems.