NYU Tandon professor of Finance and Risk Engineering wins patent for new method of boosting transparency in AI systems
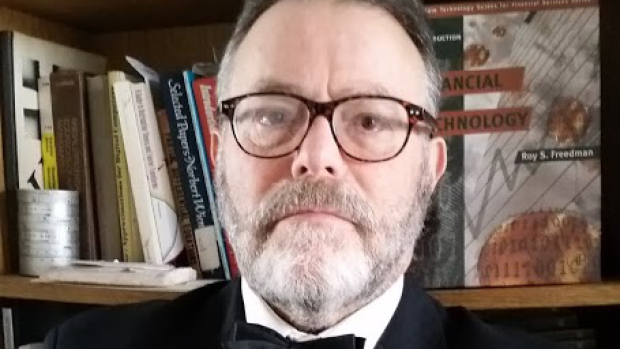
It may be a cliché at this point to assert that someone “wrote the book” on a particular topic, but in the case of Professor of Finance and Risk Engineering Roy Freedman and FinTech, that claim is literally true. His Introduction to Financial Technology delves into a dynamic, ever-evolving discipline that involves massive databases, networked computer clusters, back-office operations, regulation and compliance, bootstrap statistics, optimization, and risk management.
The term FinTech, he explains, refers to the practical, nuts-and-bolts systems that allow financial professionals to do their jobs effectively. “The concept of options and futures has been around since at least the 1600s, when the Amsterdam Stock Exchange was established,” he says. “The difference is that back then, everything was recorded by hand in a book, whereas now, of course, we have the internet and cloud computing.”
Long before authoring the seminal volume, Freedman had worked on the cutting edge of the field, helping spearhead a series of international conferences on Artificial Intelligence Applications on Wall Street in the 1990s. As program chair, Freedman aimed to provide a serious, wide-ranging forum at which the newest technologies for trading, asset allocation, and regulation could be explored. “That was during an era of legacy AI,” he recalls. “It was a time when systems were developed with the goal of getting the computer to think and solve problems like a human being would. Today, the approach is more theoretical. Researchers program computers that induce (“learn”) mathematical functions from various input and output patterns and embed the resulting algorithm in a black-box system.
Sometimes, however, as Freedman points out, patterns don’t always remain consistent and the unexpected occurs, leading to serious glitches in the system. He uses an automotive analogy: if a cow (for example) wonders in front of a moving vehicle driven by a human, the driver knows to stop immediately; a self-driving car that has been trained using modern machine learning, on the other hand, might not recognize a cow as an obstacle unless it has been taught to recognize what a cow looks like.
Freedman, who has taught a variety of FinTech and RegTech (Regulatory Technology) courses at Tandon for decades, warns that if the datasets used to train AI systems are either too small or biased in any way, serious problems can ensue — from the self-driving cars in the analogy operating unsafely to consumers being denied loans. (He regularly serves as an expert witness in court cases and points out that there are instances when a black-box system can actually be sued, such as investors challenging their robo-advisor regarding their portfolio allocations, or, as in the well-known case of Loomis v. Wisconsin, which challenged a jail sentence computed by a proprietary black-box system.)
Freedman is continuing to work on improving such systems, and along with co-inventor Isidore Sobkowski, recently received a patent for a way of boosting the transparency and explainability of AI by coupling non-interpretable black-box systems to interpretable systems.
In their method, the output results of the black-box, non-interpretable systems are used as the training targets for the interpretable systems — allowing for the development of explanations, justifications, and rationalizations for systems previously non-explainable or non-interpretable and solving the transparency and bias problems of those systems. As Freedman — who is also the founder of Inductive Solutions, a consulting company that develops commercial AI software for genetic algorithms, neural networks, and case-based reasoning — explains, it’s vital to be able to fully explain to someone denied bail or a loan why that decision was made.
In addition to his research and teaching activities, Freedman’s CV includes stints as the principal AI consultant to the Chief Information Officer of New York City Health & Human Services during the Bloomberg administration, an editor and reviewer for multiple financial-industry publications, and a member of the technical committee on Computational Finance and Economics for the IEEE Computational Intelligence Society, among other such groups. One facet of his background is cause for special pride here at NYU Tandon: besides being a faculty member, he is also a triple-alum, earning a B.S. and M.S. in Mathematics, an M.S. in Electrical Engineering, and a Ph.D. in Mathematics here.