Is AI effective if it isn't equitable and responsible?
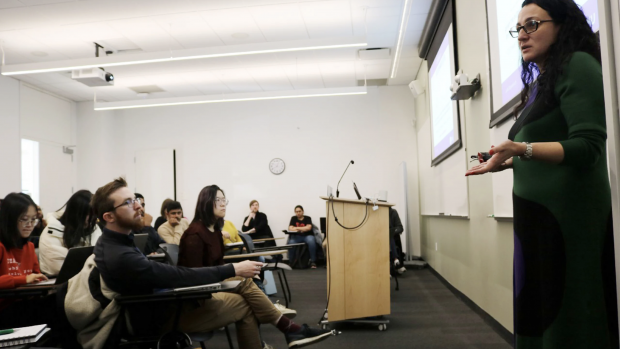
Julia Stoyanovich, professor of computer science and engineering, will teach courses on responsible data science at the Center for Responsible AI.
This article originally appeared in The Chronicle of Higher Education.
In the early days of the COVID-19 pandemic, officials in many states based their decisions about who would get a scarce coronavirus test and where to set up testing facilities on the demographics of the first groups of patients. It was, they asserted, a purely straightforward and data-driven approach that just happened to lead them to focus on affluent, White communities. As a result, poor people and people of color — many with underlying conditions that increased their risk — suffered disproportionately as the virus ravaged their neighborhoods.
Could this have been prevented? The details are in the data, says Julia Stoyanovich, an assistant professor at the NYU Tandon School of Engineering and at the Center for Data Science at NYU, and the founding director of NYU’s Center for Responsible AI.
“AI systems are dependent on the quality and the representativeness of the data being used to train them,” Stoyanovich explains. “If the data includes only certain groups of people, the system isn’t going to perform accurately for other groups.” In the case of COVID-19 testing, focusing on early patients meant focusing on those with ready access to healthcare and the means to seek treatment as soon as they felt ill.
According to Stoyanovich, we must take a hard line on ensuring that automated decision systems (ADS), including those that use Artificial Intelligence (AI), benefit everyone. “Artificially intelligent systems can harness massive datasets to improve medical diagnosis and treatment,” she notes. “There are now breast-cancer detection algorithms, for example, that have analyzed tens of thousands of mammogram slides and learned to detect patterns indicating malignancies.” However, she explains, attempts to create similar algorithms to detect skin cancer have sometimes failed because programmers neglected to include enough slides representing people with darker skin tones.
And medicine isn’t the only field where biased AI-based systems can have a negative impact. These systems are being used by banks to determine who gets loans, by landlords to determine who gets to rent an apartment, by employers to decide whom to invite for job interviews, and by court systems to decide who gets offered bail or parole. And in each of those sectors, massive injustices have been uncovered.
According to Stoyanovich, while it is customary to think that the complexity and opacity of the algorithms, like sophisticated AI methods, is to blame for some of these “bias bugs,” data is the main culprit.
“It is important to keep in mind,” Stoyanovich adds, “that a reflection cannot know whether it is distorted. That is, data alone cannot tell us whether it is a distorted reflection of a perfect world, a perfect reflection of a distorted world, or if these distortions compound. And it is not up to data or algorithms, but rather up to people — individuals, groups, and society at large — to come to consensus about whether the world is how it should be, or if it needs to be improved and, if so, how we should go about improving it.”
To ensure that technology is used in the best interests of society, it’s necessary to take a multipronged approach, which Stoyanovich is doing at the Center for Responsible AI.
An important thread that runs through her work is that we cannot fully automate responsibility. While some of the duties of carrying out the task of, for example, legal compliance can, in principle, be assigned to an algorithm, the accountability for the decisions being made by an ADS always rests with a person. As a technologist, Stoyanovich is building tools that “expose the knobs” or responsibility to people. One recent area of Stoyanovich’s focus is tools for interpretability—for allowing people to understand the process and the decisions of an ADS. Interpretability is needed because it allows people, including software developers, decision-makers, auditors, regulators, individuals who are affected by ADS decisions, and members of the public, to exercise agency by accepting or challenging algorithmic decisions and, in the case of decision-makers, to take responsibility for these decisions.
An example of such a tool is Ranking Facts, a “nutritional label” for rankings, which draws an analogy to the food industry, where simple, standard labels convey information about the ingredients and production processes. Short of setting up a chemistry lab, the consumer would otherwise have no access to this information. Similarly, consumers of data products cannot be expected to reproduce the computational procedures just to understand fitness for their use. Nutritional labels are designed to support specific decisions rather than provide complete information.
Stoyanovich is also advocating for government agencies to guard against potentially unfair, prejudicial systems by implementing regulatory mechanisms, such as a bill currently being considered in New York City that would require employers to inform job applicants when algorithm-based systems were being used to screen them, as well as the qualifications or characteristics being screened. It would also prohibit employers from buying any tools that were not being subjected to yearly bias audits.
Stoyanovich advocates, as well, for the importance of education efforts. For the past few years, she has been developing courses on Responsible Data Science at the Center for Data Science at NYU — an effort that will be scaled up at the Center for Responsible AI. The educational efforts go beyond courses; the latest offering is Volume 1 of the “Data, Responsibly” comic series called Mirror, Mirror, which Stoyanovich created in collaboration with Falaah Arif Khan, a graduate student at NYU.
“Those of us in academia have a responsibility to teach students about the social implications of the technology they build.” she says. “A typical student is driven to develop technical skills and has an engineer's desire to build useful artifacts, such as a classification algorithm with low error rates. A typical student may not have the awareness of historical discrimination, or the motivation to ask hard questions about the choice of a model or of a metric. But that student will soon become a practicing data scientist, influencing how technology companies impact society. It is critical that the students we send out into the world have an understanding of responsible data science and AI.”