Columbia & NYU Financial Engineering Colloquium: Jose Blanchet & Renyuan Xu
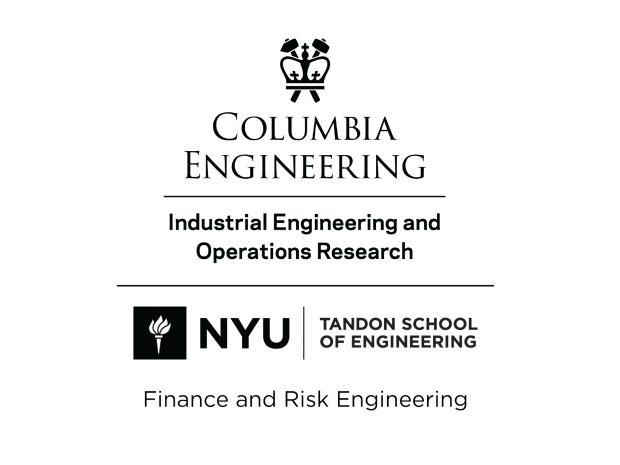
This event is free, but registration is required.
Jose Blanchet
Professor of Management Science and Engineering (MS&E), Stanford University
Title
On Highly Parameterized Controls and Fusion of Generative Diffusions
Abstract
We discuss two recent projects which touch on first-order methods in connection with two very active in artificial intelligence. The first one involves the design of efficient gradient estimators for dynamic optimization problems based on highly parameterized controls. The motivation is the application of stochastic gradient descent for the numerical solution of stochastic control problems using neural networks. Our estimator has at least a linear speed-up in the dimension of the parameter space compared to infinitesimal perturbation analysis and it can be applied to situations in which the likelihood ratio estimator may not be applicable (e.g. If the diffusion matrix depends on the parameter of interest). We show very substantial gains in high-dimensional control problems based on experiments.
The second result involves the development of an efficient approach for merging diffusion-based generative models. We assume the existence of several auxiliary models that have been trained with an abundance of data. These models are assumed to contain features that, combined, can be useful to enhance the training of a generative diffusion model for a target distribution with limited data. We merge the models using a Kullback-Leibler (KL) Barycenter given a set of weights representing the importance of the auxiliaries. In turn, we optimize the weights to improve the overall performance of the fused model. While the double optimization problem (KL Barycenter and optimizing overweights) is challenging to solve, we show that diffusion based generative modeling significantly reduce the complexity of the overall optimization. This approach also provides a mechanistic interpretation of popular fine-tuning approaches used in the literature.
The results are based on two papers, the first one (on gradient estimators) with Peter Glynn and Shengbo Wang, and the second one (on fusion) with Hao Liu, Nian Si, and Tony Ye.
Bio
Jose Blanchet is a Professor of Management Science and Engineering (MS&E) at Stanford University. Before joining Stanford, he was a professor at Columbia University in the Departments of Industrial Engineering and Operations Research, and Statistics (2008-2017). Prior to that, he was a professor in the Statistics Department at Harvard University (2004-2008). In 2010, he received the Presidential Early Career Award for Scientists and Engineers. Jose is the co-winner of the 2010 Erlang Prize, awarded every two years by the INFORMS Applied Probability Society. Several of his papers have been recognized by the biennial Best Publication Award given by the INFORMS Applied Probability Society (2007, 2023). His work has also received the Outstanding Simulation Publication Award from the INFORMS Simulation Society (2021) and other best publication awards from the Operations Management (2019) and Revenue Management Societies (2021) at INFORMS. Jose is an Amazon Scholar and previously has done consulting in areas such as investment banking, risk management, asset management, and online advertising. His research interests include Applied Probability, Stochastic Optimization, and Monte Carlo methods. He is the Area Editor of Stochastic Models in Mathematics of Operations Research and has served on the editorial boards of Advances in Applied Probability, Bernoulli, Extremes, Insurance: Mathematics and Economics, Journal of Applied Probability, Queueing Systems: Theory and Applications, and Stochastic Systems, among others.
Renyuan Xu
Assistant Professor, NYU Tandon School of Engineering
Title
Generative diffusion models: optimization, generalization and fine-tuning
Abstract
Recently, generative diffusion models have outperformed previous architectures, such as GANs, in generating high-quality synthetic data, setting a new standard for generative AI. A key component of these models is learning the associated Stein's score function. Though diffusion models have demonstrated practical success, their theoretical foundations are far from mature, especially regarding whether gradient-based algorithms can provably learn the score function. In this talk, I will present a suite of non-asymptotic theory aimed at understanding the data generation process in diffusion models and the accuracy of score estimation. Our analysis addresses both the optimization and generalization aspects of the learning process, establishing a novel connection to supervised learning and neural tangent kernels.
Building on these theoretical insights, another key challenge arises when fine-tuning pre-trained diffusion models for specific tasks or datasets to improve performance. Fine-tuning requires refining the generated outputs based on particular conditions or human preferences while leveraging prior knowledge from the pre-trained model. In the second part of the talk, we formulate this fine-tuning as a stochastic control problem, establishing its well-definedness through the Dynamic Programming Principle and proving convergence for an iterative Bellman scheme.
This talk is based on joint works with Yinbin Han (NYU) and Meisam Razaviyayn (USC).
Bio
Renyuan Xu is an assistant professor in the Department of Finance and Risk Engineering at New York University. Before joining NYU, she was an assistant professor in the Daniel J. Epstein Department of Industrial and Systems Engineering at the University of Southern California from 2021 to 2024, and a Hooke Research Fellow at the Mathematical Institute at the University of Oxford from 2019 to 2021. She completed her Ph.D. in Industrial Engineering and Operations Research at UC Berkeley in 2019.
Her research interests include stochastic analysis, machine learning theory, and mathematical finance. She is also interested in interdisciplinary topics that integrate methodologies from multiple fields and their applications in addressing high-stakes decision-making problems in large-scale systems, such as financial markets and economic systems. Another recent research interest of hers is the mathematical foundation of generative AI and the simulation of high-dimensional financial scenarios for stress testing and risk management.
She received an NSF CAREER Award in 2024, the SIAM Financial Mathematics and Engineering Early Career Award in 2023, and a JP Morgan AI Faculty Research Award in 2022. She held a Gabilan Assistant Professorship at USC between 2021-2024 and was a finalist at the INFORMS Applied Probability Society Best Paper Competition in 2018.