Chip-Chat: Current Progress and The Path Towards Generated Hardware by Generative AI
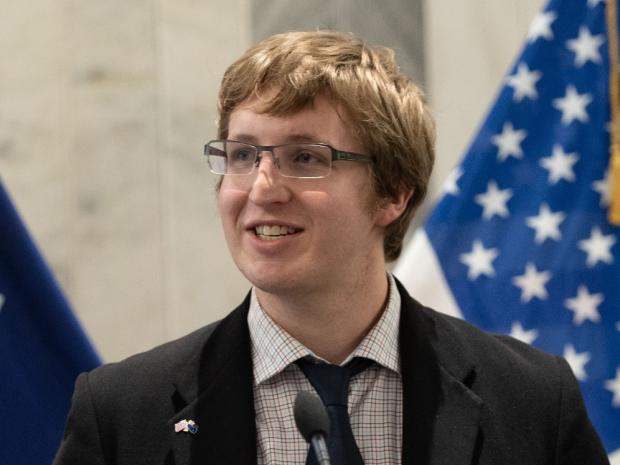
Speaker
Hammond Pearce
Assistant Professor, University of New South Wales in Sydney, Australia
Title
"Chip-Chat: Current Progress and The Path Towards Generated Hardware by Generative AI"
Abstract
Recent advances in Artificial Intelligence (AI) have enabled new techniques for machine-generated code. The most remarkable come from so-called Large Language Models (LLMs), a kind of Generative AI which have demonstrated significant potential for software development tasks such as code authorship, code explanation, and code debugging. This raises the spectre for AI to also assist with hardware development, something particularly important given the shortage of well-trained engineers in the field. Our research group has explored this area, examining different applications for Generative AI in hardware, including for hardware design, test, and bug repair. A major challenge comes from the nature of the AI training process, which relies on considerable volumes of high-quality code and annotated context, data which, unlike in the software domain, simply does not exist for hardware. The talk concludes with proposed research directions in the future which seek to overcome this and related challenges.
About Speaker
Dr. Hammond Pearce is a Lecturer (a.k.a. Assistant Professor) at the University of New South Wales in Sydney, Australia. Previously he worked at NYU's Department of Electrical and Computer Engineering / NYU Center for Cybersecurity as a Research Assistant Professor, and at NASA Ames on a research internship. His research interests lie in the intersections of AI, cybersecurity, and hardware and embedded systems design - in particular, Hammond is passionate about exploring the future of the design process in the hardware and firmware spaces, which involves the investigation of tools like ChatGPT and other Large Language Models and how they impact the development lifecycle. As part of his research work he recently won the Cybersecurity Award 2023 for the Best Machine Learning and Security Paper, the inaugural Efabless AI Generated Open-Source Silicon Design Challenge, and also previously won the Distinguished Paper Award at IEEE Symposium on Security and Privacy in 2022.