Generalization by Diversification
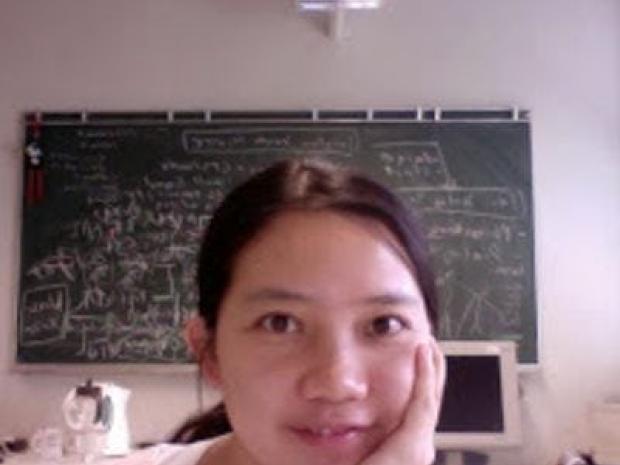
Speaker
Jia Xu, Stevens Institute of Technology
Title
"Generalization by Diversification"
Abstract
Over the last decade, Deep Learning (DL) has risen to prominence, revolutionizing the field of machine learning. However, the journey to achieve robust generalization remains incomplete, with issues like out-of-domain or noisy data persistently haunting the progress. Conventional strategies for enhancing generalization often involve trade-offs, either sacrificing accuracy in specific domains or relying on a priori knowledge of the target domain, an unrealistic condition in real-world scenarios. This talk will delve into the heart of these challenges by posing two pivotal questions: (Q1) "Is it feasible to train a more generalized machine learning model using a smaller dataset?" and (Q2) "Can we forge a universal language representation to elevate Natural Language Processing (NLP) applications?" I will unveil a novel concept, "semantic diversity", embracing data simplification by identifying representative terms. The core idea is that a concise yet expressive data representation fosters a profound comprehension of linguistic structures and meanings, ultimately leading to lower generalization errors.
Our contributions encompass the following two aspects: (Q1) Data Selection for Generalization: I will introduce cutting-edge deep reinforcement learning strategies bolstered by inventive diversity-measure-based reward functions for data selection. These approaches result in remarkable performance improvements, boasting up to +40% accuracy gains over state-of-the-art methods in tasks such as out-of-domain language modeling and sentiment analysis. (Q2) Language Representation Enhancement: I will discuss the development of a suite of word-coding algorithms by grouping words with semantic diversity dedicated to elevating language representation. Empirical results demonstrate the superiority of these coding methods, consistently outperforming subword/word-based multilingual machine translation baselines across twelve language pairs, particularly beneficial for scarce-resource languages with up to +18.5 BLEU points gain, an +840% relative improvement. By diversifying data and representation units, we elucidate an intriguing yet promising pathway toward generalization.
About Speaker
Jia Xu is an assistant professor at the Stevens Institute of Technology, and previously, she was a faculty member and Ph.D. advisor at Tsinghua University. Her research interests are Machine Learning and Natural Language Processing (NLP), focusing on highly competitive AI systems. She has more than 40 papers and regularly publishes in mainstream venues in NLP and machine learning (e.g., AAAI, ICML, ACL, EMNLP, NAACL) with 1220 citations. Professor Xu holds a Diploma from TU-Berlin and a Doctorate degree from RWTH Aachen University in Germany. During this time, she had industrial Internships at IBM in Watson and Microsoft Research (MSR) Redmond. Professor Xu has a unique record of winning over ten NLP competition awards with her team, including WMT and NIST open machine translation. Her team, with members from five nationalities, was selected for the final five of AlexaPrize social bot challenge 5.