Informing Mechanisms of Human Disease using Computational Multiomics
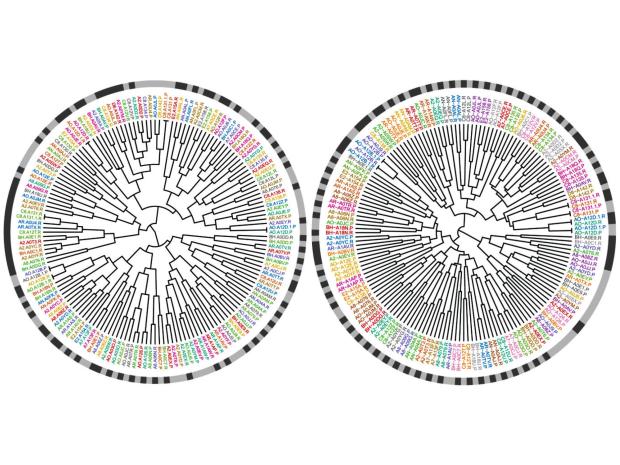
Speaker:
Kelly V. Ruggles, PhD
Associate Professor, Dept. of Medicine
NYU Grossman School of Medicine
Abstract:
Dr. Ruggles’ laboratory focuses on understanding human health and biology using data science, data visualization and predictive modeling. A primary goal of this research is in analyzing and integrating diverse data modalities including bulk and single cell sequencing, phospho- and global- proteomics, metagenomics, flow cytometry, imaging, and clinical data. The goal is to develop novel statistical and data integrative approaches to uncover important biological insights. As part of the Clinical Proteomic Tumor Analysis Consortium (CPTAC) much of Dr. Ruggles’ work has focused on the development of such methods to analyze phospho- and global-proteomics, transcriptomics, and genomics data that leads to a comprehensive understanding of cancer biology. Within this project, her team has developed computational tools that have been used extensively to identify new potentially clinically relevant targets in proteogenomic studies of breast and endometrial cancers. In addition to their work in cancer, a portion of the lab now focuses on other areas of multi-omic integration, including studying genetic and environmental factors in immune variation and stable ischemic heart disease..
Dr. Ruggles started her career with a BS degree in biological engineering from Cornell University. Subsequently she enrolled at Columbia University, where she received an MS degree in human nutrition and a PhD degree in nutritional and metabolic biology. After completing two postdoctoral fellowships in computational proteogenomics and mathematical modeling of HIV, respectively, she joined NYU’s Dept. of Medicine as an Assistant Professor in 2015. In 2022 she was promoted to Associate Professor. Since 2019 she has been the Assistant Director of Bioinformatics at the Institute for Innovations in Medical Education (IIME).
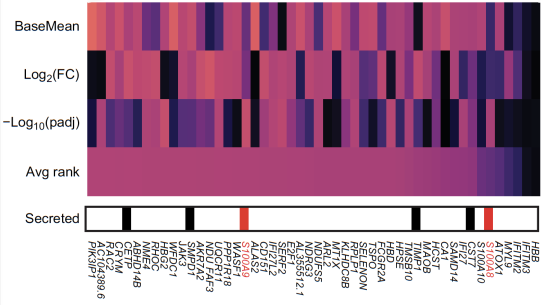