Using machine learning and uncertainty quantification to tackle data scarcity in high-resolution disaster simulations
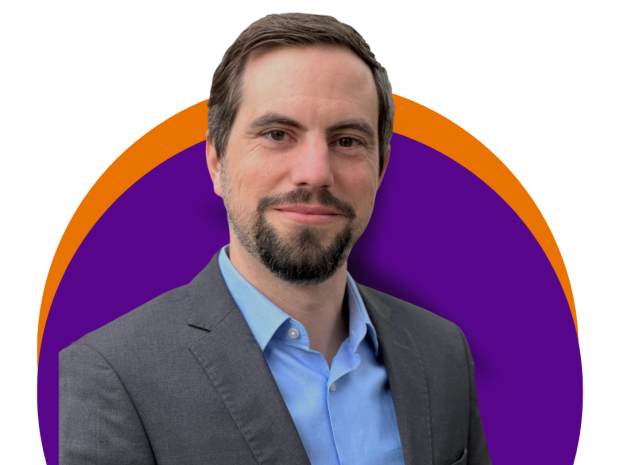
NYU CUSP is pleased to host our annual Research Seminar Series, featuring leading voices in the growing field of urban informatics. The seminars will examine real-world challenges facing cities and urban environments around the world, with topics ranging from citizen and social sciences to smart infrastructure.
Using machine learning and uncertainty quantification to tackle data scarcity in high-resolution disaster simulations
The recent devastating earthquakes in Turkey are a stark reminder of the risk natural hazards pose to many communities around the world despite significant advances in our understanding of the hazards and substantial improvements in design and construction techniques. Such catastrophic events occur more and more frequently due to urbanization and a rapidly changing climate. Mitigating this increasing risk is challenging because a large part of our cities’ building inventory and infrastructure were constructed before the introduction of modern building codes. Since strengthening all vulnerable structures is not economically feasible, cities are incentivized to collect valuable data on their buildings and communities and use it to prioritize traditional retrofits and develop other types of interventions.
Regional simulation of natural hazard events and their impact on cities promises to help us better understand critical vulnerabilities in the built and social environment and design effective interventions to address them. I introduce the components of high-resolution regional disaster simulations and illustrate the insights these calculations can provide today. Data scarcity makes it challenging to develop robust simulations, and – despite the rapidly growing application of remote sensing – certain important building features will remain difficult to identify at scale. I review machine learning and uncertainty quantification methods that researchers can use to infer missing data and characterize the uncertainty in simulation results due to imperfect and incomplete inputs. The presented methods are part of an open-source disaster simulation platform developed and supported by the NSF-funded NHERI SimCenter, and I explain how researchers can access this platform for free and leverage the included tools and resources in their work.
About the Speaker
Adam Zsarnóczay is a Research Engineer at the John A. Blume Earthquake Engineering Center at Stanford University, where his work focuses on disaster simulations that support multi-hazard risk assessment and management at a regional scale. As Associate Director for Research Outreach at the NHERI SimCenter, he connects to researchers and practitioners to monitor the state of the art and foster collaboration in the natural hazards engineering community. Adam obtained his Ph.D. in civil engineering at the Budapest University of Technology and Economics and also completed a graduate program at the University of Tokyo. He has experience working at scales ranging from individual structural members through a building to cities with hundreds of thousands of assets. His research interests include probabilistic natural hazard assessment, model development and calibration for structural response estimation and performance assessment, surrogate modeling and uncertainty quantification in large-scale, regional simulations, and using quantitative disaster simulations to support risk management and mitigation.