Dielectrography: Prospects for Imaging the Brain using Ultra-Wide-Band Microwaves
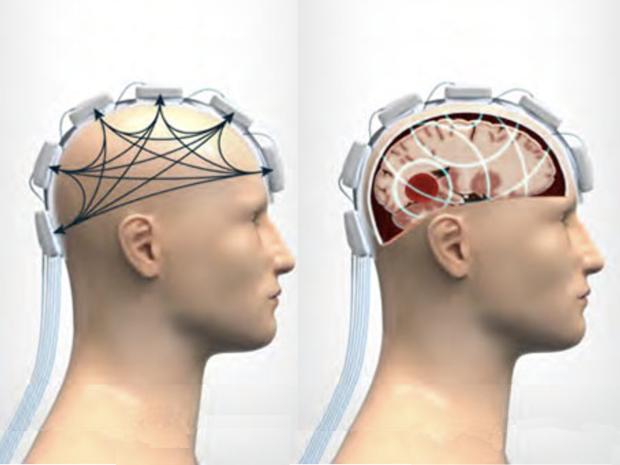
Speaker:
Leoor Alon, PhD
Assistant Professor, Dept. of Radiology
NYU Grossman School of Medicine
Abstract:
Stroke poses an immense public health burden and remains among the primary causes of death and disability worldwide. Emergent therapy is often precluded by late or indeterminate times of onset before initial clinical presentation. Rapid, mobile, safe and low-cost stroke detection technology remains a deeply unmet clinical need. Past studies have explored the use of microwave and other small form-factor strategies for rapid stroke detection; however, widespread clinical adoption remains unrealized. To overcome these challenges, Dr. Alon’s team has developed a new medical imaging technique they call dielectrography. This technique is based on ultra-wide band microwaves and relies on the fact that the dielectric properties of tissues are altered by diseases. In this talk Dr. Alon will present the latest results of his group’s work in which they combine dielectropgraphy with advanced deep-learning approaches. In particular they employed two neural networks (DNNs) for stroke detection (“classification network”), and characterization of the hemorrhage location and size (“discrimination network”). Dielectric signatures were learned on a simulated cohort of 666 hemorrhagic stroke and control subjects using 2D stochastic head models. The classification network yielded a stratified K-fold stroke detection accuracy > 94% with an AUC of 0.996, while the discrimination network resulted in a mean squared error of < 0.004 cm and < 0.02 cm, for the stroke localization and size estimation, respectively.
