Private Function Computation: Fundamental Limits and Practical Aspects
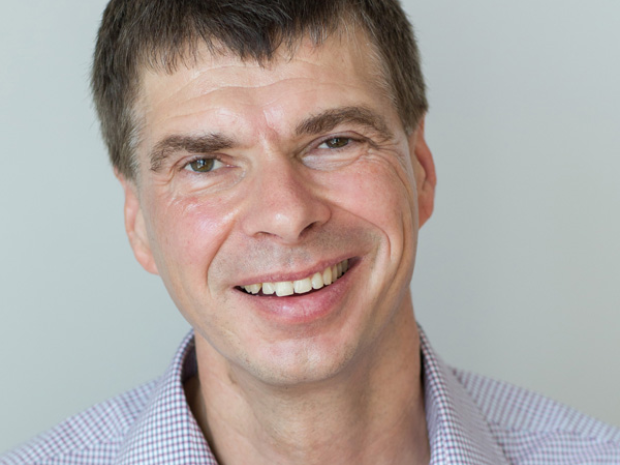
Speaker:
Joerg Kliewer
New Jersey Institute of Technology
Joerg Kliewer received the Dr. Ing. degree (Ph.D.) in electrical engineering from the University of Kiel, Germany, in 1999. He held several postdoc positions from 2000-2006. From 2007 to 2013, he was with New Mexico State University, Las Cruces, NM, USA, most recently as an associate professor. He is currently with the New Jersey Institute of Technology, Newark, NJ, USA, as a professor. His research interests span information theory, security and privacy, machine learning, graphical models, and statistical algorithms. He has been a member of the editorial board of the IEEE Information Theory Society Newsletter since 2014. He is a recipient of a Leverhulme Trust Award, a German Research Foundation Fellowship Award, an IEEE Globecom Best Paper Award, and a Fulbright Scholarship. He was an associate editor of the IEEE Transactions on Communications from 2008 to 2014 and an area editor for the same journal from 2015 to 2021. He has also been an editor for the IEEE Transactions on Information Theory from 2017 to 2020. Since 2021 he has been serving as editor for the IEEE Transactions on Information Forensics & Security.
Abstract:
We consider the problem of private function computation, where a user wishes to compute a function of f messages stored in n non-colluding databases. The goal is to provide a scheme which minimizes the download cost and is private at the same time, i.e., it should not reveal any information about the computation result to the databases. We first employ the computation of a linear function of the messages, where linear codes are used to encode the information on the databases. We show that the private linear computation capacity, which is the ratio of the desired linear function size and the total amount of downloaded information, matches the maximum distance separable coded capacity of private information retrieval for a large class of linear codes. Our capacity expression depends on the rank of the coefficient matrix obtained from all linear combinations. We also present how this approach can be extended to computing arbitrary multivariate polynomials of the messages. Here, the presented schemes yield improved download rates compared to the best known schemes from the literature. Finally, in an attempt to make private information retrieval more practical we propose to relax the privacy and perfect reconstruction requirements. For scenarios where the data statistics is unknown, we propose a new deep learning framework based on generative adversarial networks, which allows to learn schemes minimizing the download cost from the data itself.