Modern signal processing tools for nonstationary biomedical signals in a decentralized world
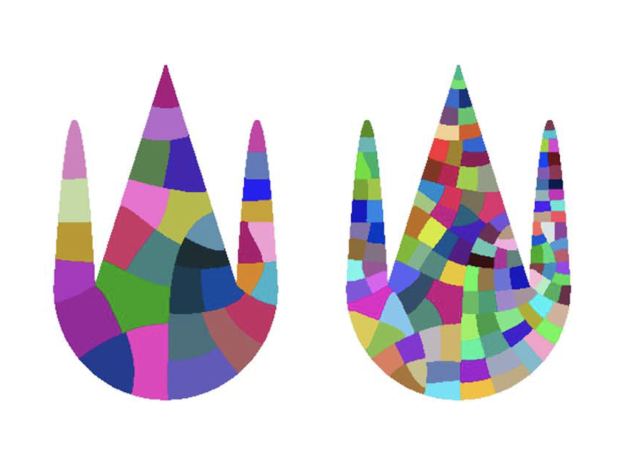
Speaker:
Han-Tieng Wu, MD, Ph.D.
Associate Professor
Depts. of Mathematics and Statistical Sciences
Duke University
Abstract:
Long-term, high-frequency and nonstationary physiological time series contain rich information. However, extracting useful information from these time series for clinical usage is challenging. This challenge is more pronounced in a decentralized world enabled by digital health technologies, particularly in our post-COVID era, where usually few channels with low signal quality are available. In this talk, I will show some signal processing tools designed to handle such challenges. The tools are mainly based on time-frequency analysis and manifold learning. Examples include single channel blind source separation for the transabdominal fetal electrocardiogram, mobile electroence-phalogram analysis and photoplethysmography response to fluid withdrawal status.
Dr. Wu received his PhD in mathematics from Princeton University in 2011 under the supervision of Professor Ingrid Daubechies. After being a postdoctoral researcher at UC Berkeley and Stanford, he joined the Dept. of Mathematics at the University of Toronto. as an assistant professor in 2014. Three years later he accepted an offer to join the Departments of Mathematics and Statistical Science at Duke University as a tenured Associate Professor. Before coming to mathematics, Dr. Wu earned a medical degree from the National Yang Ming University in Taipei, Taiwan, and practiced as a resident at the Taipei Veterans General Hospital for 3 years. Dr. Wu has been recognized for his pioneer work with several awards, including a Sloan Research Fellowship in 2015 and the PIMS Early Career Award from the Canadian Applied and Industrial Society (CAIMS) in 2017
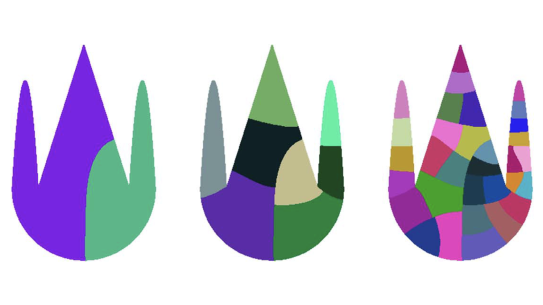