Scaling up knowledge discovery in systems neuroscience: a closed-loop brain-machine-interface (BMI) solution
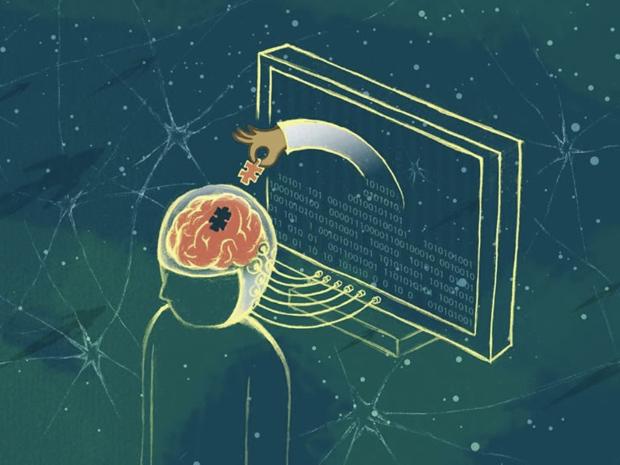
Speaker:
Zhe S. Chen
Associate Professor
Depts. of Psychiatry and Neuroscience & Physiology, Neuroscience Institute
Abstract:
Emerging technologies to acquire data at increasingly greater scales promise to transform discovery in systems neuroscience. However, current exponential growth in the scale of data acquisition is a double-edged sword. Scaling up data acquisition can speed up the cycle of discovery but can also misinterpret the results or possibly slow down the cycle because of challenges presented by the curse of high-dimensional data. In this talk, Dr. Chen will first discuss the trend and bottleneck of data analysis for high-dimensional neural data, touching on the issue of dimensionality reduction, subsampling, active and adaptive closed-loop (AACL) experimental design. In addition, he will use the rodent hippocampus as an example to illustrate the development of scalable methods for analyzing large-scale hippocampal population codes based on multimodal recordings (spikes, local field potentials and calcium imaging). The presentation will demonstrate that AACL experiments and brain-machine interfaces (BMIs) can provide a solution to mitigate the dimensionality bottleneck.
Dr. Chen received his PhD degree in the field of signal processing, machine learning, and neural computation from McMaster University in Hamilton, Ontario, Canada, in 2005. After that he did research at RIKEN Brain Science Institute, MIT and Harvard Medical School. In 2014 Dr. Chen was appointed as an Assistant Professor in the NYU Depts. of Psychiatry and Neuroscience and Physiology. He has published more than 120 research papers and was the author of the book “Correlative Brain” (Wiley, 2007), the editor for the book "Advanced State Space Methods for Neural and Clinical Data" (Cambridge UP, 2015), and the co-editor for the book "Dynamic Neuroscience" (Springer, 2018).
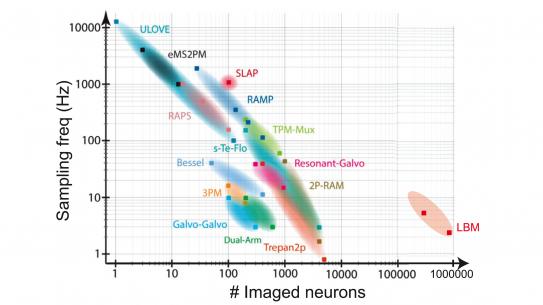