Design of Complex Material Systems with Hierarchical Machine Learning
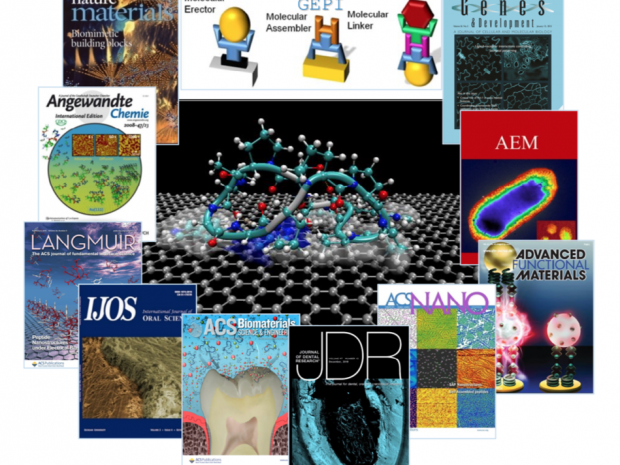
Speaker:
Newell Washburn
Departments of Chemistry and Biomedical Engineering
Carnegie Mellon University
Abstract:
Complex materials – ranging from concentrated colloidal suspensions to 3D-printed constructs to high- performance polymers – are ubiquitous in technological applications. Their properties are determined by multiple competing forces that give rise to a diversity of behaviors, but this also makes their design chal- lenging. Purely computational approaches are often intractable due to the inherently broad spectra of characteristic time-, energy-, and length scales, but traditional data-driven approaches are ineffective due to the relatively small datasets that are generated experimentally which make it challenging to de- termine the couplings between these underlying forces. At CMU, we developed hierarchical machine learning (HML) as a tool for designing and optimizing complex material systems. HML integrates physi- cal modeling in a framework of statistical learning, allowing the algorithm to rapidly determine compli- cated response surfaces based on low-iteration experimentation, and to propose novel solutions to opti- mization problems. In this talk, I will introduce the HML methodology and present applications in three polymer technologies: superplasticizers for low-CO2 cement and concrete, additive manufacturing of soft matter, and predictions of the mechanical properties of crosslinked polyurethane elastomers. I will also discuss my work in the translation of HML in the chemicals and materials industry through a CMU spinoff company, Ansatz AI, which is currently engaging with clients across a range of applications.
- 10:30 Refreshments
- 10:45–12:00 Talk