Large-Scale Traffic Modeling, Monitoring and Control in Urban Networks
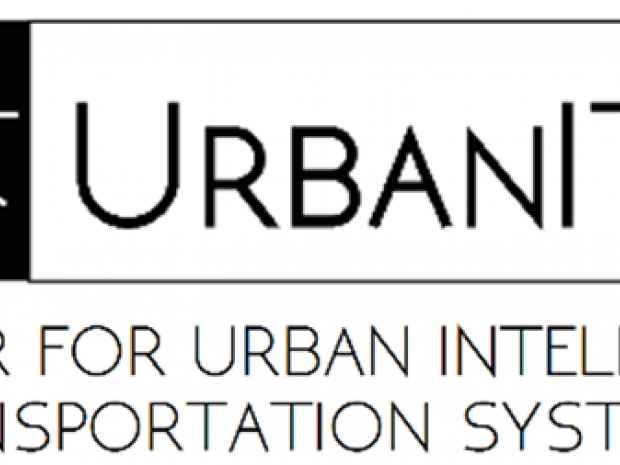
Presenter:
Mohsen Ramezani
The University of Sydney
Abstract
Accurate monitoring and efficient control of transportation systems remain a big challenge, due to the complexity and extent of transportation networks, unpredictability of travelers choices (in terms of route, departure time, and mode of transport), and the uncertainty in their reactions to the control. Over decades, control strategies based on disaggregated traffic flow models, which model behavior of every vehicle, have been proposed and applied for isolated intersections and arterials. However, largescale network control schemes based on microscopic modeling require a tremendous amount of data and thus are computationally intractable. Instead, macroscopic models that only describe the aggregated behavior of traffic networks, and relying on less information, are well-suited for large-scale transportation system analysis. An instance of such models is the macroscopic fundamental diagram (MFD) that aims at simplifying the micro-modeling of urban networks where the collective traffic flow dynamics of subnetworks capture the main characteristics of traffic congestion.
Furthermore, monitoring of operating conditions on signalized arterials and urban networks requires estimates of performance measures for both system operators and travelers. These tools can help traffic managers to monitor the traffic performance, provide travelers with information, and optimize signal timing plans and other control strategies. Emergence of smart cities during the last decade offers massive data sets concerning human mobility, fostered by the widespread distribution of sensors, such as Global Positioning System (GPS) devices in many modes of transport (probe vehicles) and smart phones (crowdsourcing). This talk will discuss (i) network-level control of urban traffic utilizing the MFD modeling and (ii) novel urban traffic monitoring methods based on probe vehicle data.