NYU Tandon researchers mitigate racial bias in facial recognition technology with demographically diverse synthetic image dataset for AI training
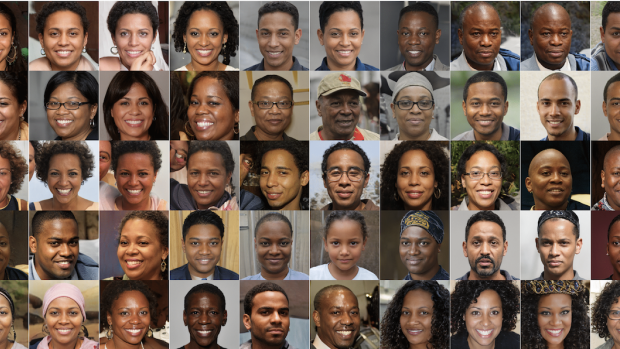
Examples of different identities corresponding to the "African" racial group generated using the approach proposed by the NYU Tandon researchers. These have been randomly selected from the dataset showing different poses and expression.
Facial recognition technology has made great strides in accuracy thanks to advanced artificial intelligence (AI) models trained on massive datasets of face images.
These datasets often lack diversity in terms of race, ethnicity, gender, and other demographic categories, however, causing facial recognition systems to perform worse on underrepresented demographic groups compared to groups ubiquitous in the training data. In other words, the systems are less likely to accurately match different images depicting the same person if that person belongs to a group that was insufficiently represented in the training data.
This systemic bias can jeopardize the integrity and fairness of facial recognition systems deployed for security purposes or to protect individual rights and civil liberties.
Researchers at NYU Tandon School of Engineering are tackling the problem. In a recent paper, a team led by Julian Togelius, Associate Professor of Computer Science and Engineering (CSE) revealed it successfully reduced facial recognition bias by generating highly diverse and balanced synthetic face datasets that can train facial recognition AI models to produce more fair results. The paper’s lead author is Anubhav Jain, Ph.D. candidate in CSE.
The team applied an "evolutionary algorithm" to control the output of StyleGAN2, an existing generative AI model that creates high-quality artificial face images and was initially trained on the Flickr Faces High Quality Dataset (FFHQ). The method is a "zero-shot" technique, meaning the researchers used the model as-is, without additional training.
The algorithm the researchers developed searches in the model’s latent space until it generates an equal balance of synthetic faces with appropriate demographic representations. The team was able to produce a dataset of 13.5 million unique synthetic face images, with 50,000 distinct digital identities for each of six major racial groups: White, Black, Indian, Asian, Hispanic and Middle Eastern.
The researchers then pre-trained three facial recognition models — ArcFace, AdaFace and ElasticFace — on the large, balanced synthetic dataset they generated.
The result not only boosted overall accuracy compared to models trained on existing imbalanced datasets, but also significantly reduced demographic bias. The trained models showed more equitable accuracy across all racial groups compared to existing models exhibiting poor performance on underrepresented minorities.
The synthetic data proved similarly effective for improving the fairness of algorithms analyzing face images for attributes like gender and ethnicity categorization.
By avoiding the need to collect and store real people's face data, the synthetic approach delivers the added benefit of protecting individual privacy, a concern when training AI models on images of actual people’s faces. And by generating balanced representations across demographic groups, it overcomes the bias limitations of existing face datasets and models.
The researchers have open-sourced their code to enable others to reproduce and build upon their work developing unbiased, high-accuracy facial recognition and analysis capabilities. This could pave the way for deploying the technology more responsibly across security, law enforcement and other sensitive applications where fairness is paramount.
This study — whose authors also include Rishit Dholakia (’22) MS in Computer Science, NYU Courant; and Nasir Memon, Dean of Engineering at NYU Shanghai, NYU Tandon ECE professor and faculty member of NYU Center for Cybersecurity — builds upon a paper the researchers shared at the IEEE International Joint Conference on Biometrics (IJCB), September 25-28, 2023.
Anubhav Jain , Rishit Dholakia , Nasir Memon , et al. Zero-shot demographically unbiased image generation from an existing biased StyleGAN. TechRxiv. December 02, 2023