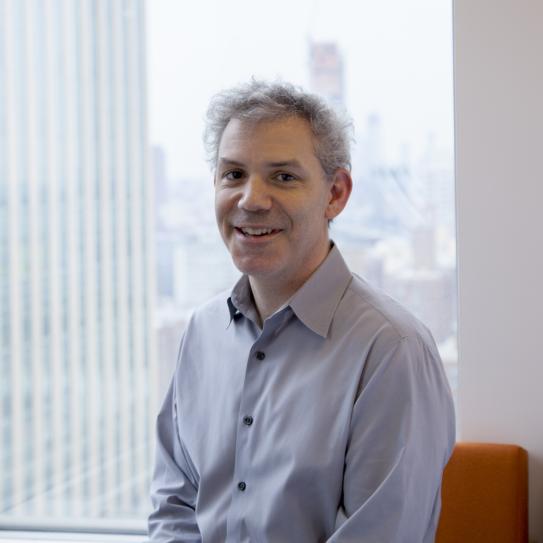
Ivan Selesnick is a Professor of Electrical and Computer Engineering and Professor of Biomedical Engineering, and Radiology at NYU Tandon School of Engineering. He received the BS, MEE, and PhD degrees in Electrical Engineering from Rice University in Houston, Texas. He joined Polytechnic University in 1997 (now NYU Tandon School of Engineering).
He received an Alexander von Humboldt Fellowship in 1997 and a National Science Foundation Career award in 1999. In 2003, he received the Jacobs Excellence in Education Award from Polytechnic University. He became an IEEE Fellow in 2016.
His research interests are in signal and image processing, wavelet-based signal processing, sparsity techniques, and biomedical signal processing. He has been an associate editor for the IEEE Transactions on Image Processing, IEEE Signal Processing Letters, IEEE Transactions on Signal Processing, and IEEE Transactions on Computational Imaging.
Education
Rice University, 1996
PhD, Electrical Engineering
Rice University, 1991
MS, Electrical Engineering
Rice University, 1990
Bachelor of Science, Electrical Engineering
Publications
Selected Journal Articles
R. Soleymani, I. W. Selesnick, and D. M. Landsberger, “SEDA: a tunable Q-factor wavelet-based noise reduction algorithm for multi-talker babble,” Speech Communication, vol. 96, pp. 102–115, 2018. doi: 10.1016/j.specom.2017.11.004.
I. Selesnick, “Sparse regularization via convex analysis,” IEEE Trans. Signal Process., vol. 65, no. 17, pp. 4481–4494, Sep. 2017. doi: 10.1109/TSP.2017.2711501.
W. Dai, I. Selesnick, J.-R. Rizzo, J. C. Rucker, and T. E. Hudson, “A nonlinear generalization of the Savitzky-Golay filter and the quantitative analysis of saccades,” J. Vision, vol. 17, no. 9, pp. 10, 1–15, Aug. 2017. doi: 10.1167/17.9.10.
I. Selesnick, “Total variation denoising via the Moreau envelope,” IEEE Signal Processing Letters, vol. 24, no. 2, pp. 216–220, Feb. 2017. doi: 10.1109/LSP.2017.2647948.
A. Lanza, S. Morigi, I. Selesnick, and F. Sgallari, “Nonconvex nonsmooth optimization via convex– nonconvex majorization–minimization,” Numerische Mathematik, vol. 136, no. 2, pp. 343–381, 2017. doi: 10.1007/s00211-016-0842-x.
Y. Ding, W. He, B. Chen, Y. Zi, and I. W. Selesnick, “Detection of faults in rotating machinery using periodic time-frequency sparsity,” Journal of Sound and Vibration, vol. 382, pp. 357–378, 2016. doi: 10.1016/j.jsv.2016.07.004.
F. Uysal, I. Selesnick, and B. M. Isom, “Mitigation of wind turbine clutter for weather radar by signal separation,” IEEE Trans. on Geoscience and Remote Sensing, vol. 54, no. 5, pp. 2925–2934, May 2016. doi: 10.1109/TGRS.2015.2508380.
A. Parekh and I. W. Selesnick, “Enhanced low-rank matrix approximation,” IEEE Signal Processing Letters, vol. 23, no. 4, pp. 493–497, Apr. 2016. doi: 10.1109/LSP.2016.2535227.
A. Parekh and I. W. Selesnick, “Convex denoising using non-convex tight frame regularization,” IEEE Signal Processing Letters, vol. 22, no. 10, pp. 1786–1790, Oct. 2015. doi: 10.1109/LSP. 2015.2432095.
A. Parekh, I. W. Selesnick, D. M. Rapoport, and I. Ayappa, “Detection of K-complexes and sleep spindles (DETOKS) using sparse optimization,” Journal of Neuroscience Methods, vol. 251, pp. 37–46, Aug. 2015. doi: 10.1016/j.jneumeth.2015.04.006.
Y. Ding and I. W. Selesnick, “Artifact-free wavelet denoising: non-convex sparse regularization, convex optimization,” IEEE Signal Processing Letters, vol. 22, no. 9, pp. 1364–1368, Sep. 2015. doi: 10.1109/LSP.2015.2406314.
I. W. Selesnick, A. Parekh, and I. Bayram, “Convex 1-D total variation denoising with non-convex regularization,” IEEE Signal Processing Letters, vol. 22, no. 2, pp. 141–144, Feb. 2015. doi: 10.1109/LSP.2014.2349356.
I. W. Selesnick, H. L. Graber, Y. Ding, T. Zhang, and R. L. Barbour, “Transient artifact reduction algorithm (TARA) based on sparse optimization,” IEEE Trans. Signal Process., vol. 62, no. 24, pp. 6596–6611, Dec. 2014. doi: 10.1109/TSP.2014.2366716.
X. Ning, I. W. Selesnick, and L. Duval, “Chromatogram baseline estimation and denoising using sparsity (BEADS),” Chemometrics and Intelligent Laboratory Systems, vol. 139, pp. 156–167, Dec. 2014. doi: 10.1016/j.chemolab.2014.09.014.
F. Uysal, I. Selesnick, U. Pillai, and B. Himed, “Dynamic clutter mitigation using sparse optimization,” IEEE Aerospace and Electronic Systems Magazine, vol. 29, no. 7, pp. 37–49, Jul. 2014. doi: 10.1109/MAES.2014.130137.
P.-Y. Chen and I. W. Selesnick, “Group-sparse signal denoising: non-convex regularization, convex optimization,” IEEE Trans. Signal Process., vol. 62, no. 13, pp. 3464–3478, Jul. 2014. doi: 10. 1109/TSP.2014.2329274.
I. W. Selesnick and I. Bayram, “Sparse signal estimation by maximally sparse convex optimization,” IEEE Trans. Signal Process., vol. 62, no. 5, pp. 1078–1092, Mar. 2014. doi: 10.1109/TSP. 2014.2298839.
P.-Y. Chen and I. W. Selesnick, “Translation-invariant shrinkage/thresholding of group sparse signals,” Signal Processing, vol. 94, pp. 476–489, Jan. 2014. doi: 10.1016/j.sigpro.2013.06. 011.
I. W. Selesnick, S. Arnold, and V. R. Dantham, “Polynomial smoothing of time series with additive step discontinuities,” IEEE Trans. Signal Process., vol. 60, no. 12, pp. 6305–6318, Dec. 2012. doi: 10.1109/TSP.2012.2214219.
I. W. Selesnick, “Wavelet transform with tunable Q-factor,” IEEE Trans. Signal Process., vol. 59, no. 8, pp. 3560–3575, Aug. 2011. doi: 10.1109/TSP.2011.2143711.
I. W. Selesnick, “Resonance-based signal decomposition: a new sparsity-enabled signal analysis method,” Signal Processing, vol. 91, no. 12, pp. 2793–2809, 2011. doi: DOI:10.1016/j.sigpro. 2010.10.018.
I. Bayram and I. W. Selesnick, “A subband adaptive iterative shrinkage/thresholding algorithm,” IEEE Trans. Signal Process., vol. 58, no. 3, pp. 1131–1143, Mar. 2010. doi: 10.1109/TSP.2009. 2036064.
I. Bayram and I. W. Selesnick, “Overcomplete discrete wavelet transforms with rational dilation factors,” IEEE Trans. Signal Process., vol. 57, no. 1, pp. 131–145, Jan. 2009. doi: 10.1109/TSP. 2008.2007097.
I. W. Selesnick, “Wavelets, a modern tool for signal processing,” Physics Today, vol. 60, no. 10, pp. 78–79, Oct. 2007. doi: 10.1063/1.2800108.
I. W. Selesnick, R. G. Baraniuk, and N. G. Kingsbury, “The dual-tree complex wavelet transform - A coherent framework for multiscale signal and image processing,” IEEE Signal Processing Magazine, vol. 22, no. 6, pp. 123–151, Nov. 2005. doi: 10.1109/MSP.2005.1550194.
A. F. Abdelnour and I. W. Selesnick, “Symmetric nearly shift-invariant tight frame wavelets,” IEEE Trans. Signal Process., vol. 53, no. 1, pp. 231–239, Jan. 2005. doi: 10.1109/TSP.2004. 838959.
L. Sendur and I. W. Selesnick, “Bivariate shrinkage functions for wavelet-based denoising exploiting interscale dependency,” IEEE Trans. Signal Process., vol. 50, no. 11, pp. 2744–2756, Nov. 2002. doi: 10.1109/TSP.2002.804091.
I. W. Selesnick, “The design of approximate Hilbert transform pairs of wavelet bases,” IEEE Trans. Signal Process., vol. 50, no. 5, pp. 1144–1152, May 2002. doi: 10.1109/78.995070.
D. Czarkowski, D. V. Chudnovsky, G. V. Chudnovsky, and I. W. Selesnick, “Solving the optimal PWM problem for single-phase inverters,” IEEE Trans. on Circuits and Systems I, vol. 49, no. 4, pp. 465–475, Apr. 2002. doi: 10.1109/TCSII.2002.1013869.
I. W. Selesnick, “Hilbert transform pairs of wavelet bases,” IEEE Signal Processing Letters, vol. 8, no. 6, pp. 170–173, Jun. 2001. doi: 10.1109/97.923042.
I. W. Selesnick, “Interpolating multiwavelet bases and the sampling theorem,” IEEE Trans. Signal Process., vol. 47, no. 6, pp. 1615–1621, Jun. 1999. doi: 10.1109/78.765131.
I. W. Selesnick, “The slantlet transform,” IEEE Trans. Signal Process., vol. 47, no. 5, pp. 1304– 1313, May 1999. doi: 10.1109/78.757218.
I. W. Selesnick and C. S. Burrus, “Generalized digital Butterworth filter design,” IEEE Trans. Signal Process., vol. 46, no. 6, pp. 1688–1694, Jun. 1998. doi: 10.1109/78.678493.
I. W. Selesnick, M. Lang, and C. S. Burrus, “Constrained least square design of FIR filters without specified transition bands,” IEEE Trans. Signal Process., vol. 44, no. 8, pp. 1879–1892, Aug. 1996. doi: 10.1109/78.533710.
I. W. Selesnick and C. S. Burrus, “Automatic generation of prime length FFT programs,” IEEE Trans. Signal Process., vol. 44, no. 1, pp. 14–24, Jan. 1996. doi: 10.1109/78.482008.